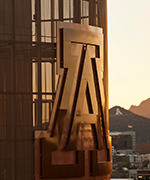
Michael Chertkov
- Professor, Mathematics
- Chair, Applied Mathematics - GIDP
- Professor, Statistics-GIDP
- Member of the Graduate Faculty
Contact
- (520) 621-4664
- Mathematics, Rm. 416
- Tucson, AZ 85721
- chertkov@arizona.edu
Degrees
- Ph.D. Physics
- Weizmann Institute of Science, Rehovot, Israel
Awards
- American Association for the Advancement of Science Fellow
- AAAS, Spring 2023
- Fellow of AAAS
- Fall 2022
Interests
Research
Science Informed AIStatistical HydrodynamicsPower Systems Modeling and ControlGraphical, Network and Agent Based Models Viral and Social Epidemiology
Teaching
Method of Applied Mathematics (core class of Applied Math GIDP)Inference, Learning and Optimization with Graphs and Networks (taught as a topic course in the fall of 2022)Stochastic Control and Learning (propose to teach as a spacial topic course in the fall of 2023)
Courses
2024-25 Courses
-
APPL Research
APPL 900 (Spring 2025) -
Advncd Tpcs in Undergrad Math
MATH 496T (Spring 2025) -
Case Study Applied Math
MATH 586 (Spring 2025) -
Dissertation
APPL 920 (Spring 2025) -
Independent Study
APPL 599 (Spring 2025) -
Research
CSC 900 (Spring 2025) -
Case Study Applied Math
MATH 586 (Fall 2024) -
Dissertation
APPL 920 (Fall 2024) -
Topics In Applied Math
MATH 577 (Fall 2024)
2023-24 Courses
-
Internship
MATH 593 (Summer I 2024) -
Case Study Applied Math
MATH 586 (Spring 2024) -
Dissertation
MATH 920 (Spring 2024) -
Methods Applied Mathematics II
MATH 581B (Spring 2024) -
Research
CSC 900 (Spring 2024) -
Case Study Applied Math
MATH 586 (Fall 2023) -
Dissertation
MATH 920 (Fall 2023) -
Independent Study
APPL 599 (Fall 2023) -
Research
CSC 900 (Fall 2023) -
Research
MATH 900 (Fall 2023) -
Topics In Applied Math
MATH 577 (Fall 2023)
2022-23 Courses
-
Internship
MATH 593 (Summer I 2023) -
Case Study Applied Math
MATH 586 (Spring 2023) -
Dissertation
MATH 920 (Spring 2023) -
Methods Applied Mathematics II
MATH 581B (Spring 2023) -
Research
MATH 900 (Spring 2023) -
Case Study Applied Math
MATH 586 (Fall 2022) -
Dissertation
MATH 920 (Fall 2022) -
Independent Study
CSC 599 (Fall 2022) -
Independent Study
MATH 599 (Fall 2022) -
Research
CSC 900 (Fall 2022) -
Research
MATH 900 (Fall 2022) -
Topics In Applied Math
MATH 577 (Fall 2022)
2021-22 Courses
-
Case Study Applied Math
MATH 586 (Spring 2022) -
Dissertation
MATH 920 (Spring 2022) -
Methods Applied Mathematics II
MATH 581B (Spring 2022) -
Research
MATH 900 (Spring 2022) -
Case Study Applied Math
MATH 586 (Fall 2021) -
Dissertation
MATH 920 (Fall 2021) -
Independent Study
MATH 599 (Fall 2021) -
Methods Applied Mathematics I
MATH 581A (Fall 2021) -
Research
MATH 900 (Fall 2021)
2020-21 Courses
-
Case Study Applied Math
MATH 586 (Spring 2021) -
Independent Study
MATH 599 (Spring 2021) -
Prin+Method Applied Math
MATH 583B (Spring 2021) -
Research
MATH 900 (Spring 2021) -
Case Study Applied Math
MATH 586 (Fall 2020) -
Independent Study
MATH 599 (Fall 2020) -
Independent Study
STAT 599 (Fall 2020) -
Prin+Method Applied Math
MATH 583A (Fall 2020) -
Research
MATH 900 (Fall 2020)
2019-20 Courses
-
Case Study Applied Math
MATH 586 (Spring 2020) -
Independent Study
MATH 599 (Spring 2020) -
Prin+Method Applied Math
MATH 583B (Spring 2020) -
Research
MATH 900 (Spring 2020) -
Case Study Applied Math
MATH 586 (Fall 2019) -
Independent Study
PHYS 599 (Fall 2019) -
Prin+Method Applied Math
MATH 583A (Fall 2019)
2018-19 Courses
-
Case Study Applied Math
MATH 586 (Spring 2019)
Scholarly Contributions
Journals/Publications
- Chertkov, M. (2023). Universality and Control of Fat Tails. IEEE Control Systems Letters, v., 1718-1723.More infoMotivated by applications in hydrodynamics and networks ofthermostatically-control loads in buildings we study control of lineardynamical systems driven by additive and also multiplicative noise of a generalposition. Utilizing mathematical theory of stochastic multiplicative processeswe present a universal way to estimate fat, algebraic tails of the state vectorprobability distributions. This prompts us to introduce and analyzemean-q-power stability criterion, generalizing the mean-square stabilitycriterion, and then juxtapose it to other tools in control.[Journal_ref: IEEE Control Systems Letters, v. 7, p. 1718-1723, 2023]
- Tian, Y., Woodward, M., Stepanov, M., Fryer, C., Hyett, C. M., Livescu, D., & Chertkov, M. (2023). Lagrangian Large Eddy Simulations via Physics Informed Machine Learning. PNAS.
- Woodward, M., Tian, Y., Hyett, C. M., Fryer, C., Livescu, D., Stepanov, M., & Chertkov, M. (2022). Physics-informed machine learning with smoothed particle hydrodynamics: Hierarchy of reduced Lagrangian models of turbulence. Physical Review Fluids.
- , A. A., & , M. C. (2022). Which Neural Network to Choose for Post-Fault Localization, Dynamic State Estimation and Optimal Measurement Placement in Power Systems?.More infoWe consider a power transmission system monitored with Phasor MeasurementUnits (PMUs) placed at significant, but not all, nodes of the system. Assumingthat a sufficient number of distinct single-line faults, specifically pre-faultstate and (not cleared) post-fault state, are recorded by the PMUs and areavailable for training, we, first, design a comprehensive sequence of NeuralNetworks (NNs) locating the faulty line. Performance of different NNs in thesequence, including Linear Regression, Feed-Forward NN, AlexNet, GraphicalConvolutional NN, Neural Linear ODE and Neural Graph-based ODE, orderedaccording to the type and amount of the power flow physics involved, arecompared for different levels of observability. Second, we build a sequence ofadvanced Power-System-Dynamics-Informed and Neural-ODE based Machine Learningschemes trained, given pre-fault state, to predict the post-fault state andalso, in parallel, to estimate system parameters. Finally, third, andcontinuing to work with the first (fault localization) setting we design a(NN-based) algorithm which discovers optimal PMU placement.[Journal_ref: ]
- , F. C., & , M. C. (2022). Message Passing Descent for Efficient Machine Learning.More infoWe propose a new iterative optimization method for the {\bf Data-Fitting}(DF) problem in Machine Learning, e.g. Neural Network (NN) training. Theapproach relies on {\bf Graphical Model} (GM) representation of the DF problem,where variables are fitting parameters and factors are associated with theInput-Output (IO) data. The GM results in the {\bf Belief Propagation}Equations considered in the {\bf Large Deviation Limit} corresponding to thepractically important case when the number of the IO samples is much largerthan the number of the fitting parameters. We suggest the {\bf Message PassageDescent} algorithm which relies on the piece-wise-polynomial representation ofthe model DF function. In contrast with the popular gradient descent andrelated algorithms our MPD algorithm rely on analytic (not automatic)differentiation, while also (and most importantly) it descents through therugged DF landscape by \emph{making non local updates of the parameters} ateach iteration. The non-locality guarantees that the MPD is not trapped in thelocal-minima, therefore resulting in better performance than locally-updatedalgorithms of the gradient-descent type. We illustrate superior performance ofthe algorithm on a Feed-Forward NN with a single hidden layer and apiece-wise-linear activation function.[Journal_ref: ]
- , I. L., , D. M., , H. O., & , M. C. (2022). Super-relaxation of space-time-quantized ensemble of energy loads to curtail their synchronization after demand response perturbation. Applied Energy vol..More infoEnsembles of thermostatically controlled loads (TCL) provide a significantdemand response reserve for the system operator to balance power grids.However, this also results in the parasitic synchronization of individualdevices within the ensemble leading to long post-demand-response oscillationsin the integrated energy consumption of the ensemble. The synchronization iseventually destructed by fluctuations, thus leading to the (pre-demandresponse) steady state; however, this natural desynchronization, or relaxationto a statistically steady state, is too long. A resolution of this problemconsists in measuring the ensemble's instantaneous consumption and using it asa feedback to stochastic switching of the ensemble's devices between on- andoff- states. A simplified continuous-time model showed that carefully tunednonlinear feedback results in a fast (super-) relaxation of the ensemble energyconsumption. Since both state information and control signals are discrete, theactual TCL devices operation is space-time quantized, and this must beconsidered for realistic TCL ensemble modelling. Here, assuming that states arecharacterized by indoor temperature (quantifying comfort) and air conditionerregime (on, off), we construct a discrete model based on the probabilisticdescription of state transitions. We demonstrate that super-relaxation holds insuch a more realistic setting, and that while it is stable against randomnessin the stochastic matrix of the quantized model, it remains sensitive to thetime discretization scheme. Aiming to achieve a balance betweensuper-relaxation and customer's comfort, we analyze the dependence ofsuper-relaxation on details of the space-time quantization, and provide asimple analytical criterion to avoid undesirable oscillations in consumption.[Journal_ref: Applied Energy vol. 285, 116419 (2021)]
- , L. P., & , M. C. (2022). Embedding Power Flow into Machine Learning for Parameter and State Estimation.More infoModern state and parameter estimations in power systems consist of twostages: the outer problem of minimizing the mismatch between networkobservation and prediction over the network parameters, and the inner problemof predicting the system state for given values of the parameters. The standardsolution of the combined problem is iterative: (a) set the parameters, e.g. topriors on the power line characteristics, (b) map input observation toprediction of the output, (c) compute the mismatch between predicted andobserved output, (d) make a gradient descent step in the space of parameters tominimize the mismatch, and loop back to (a). We show how modern MachineLearning (ML), and specifically training guided by automatic differentiation,allows to resolve the iterative loop more efficiently. Moreover, we extend thescheme to the case of incomplete observations, where Phasor Measurement Units(reporting real and reactive powers, voltage and phase) are available only atthe generators (PV buses), while loads (PQ buses) report (via SCADA controls)only active and reactive powers. Considering it from the implementationperspective, our methodology of resolving the parameter and state estimationproblem can be viewed as embedding of the Power Flow (PF) solver into thetraining loop of the Machine Learning framework (PyTorch, in this study). Weargue that this embedding can help to resolve high-level optimization problemsin power system operations and planning.[Journal_ref: ]
- , L. P., & , M. C. (2022). Physics-Informed Graphical Neural Network for Parameter & State Estimations in Power Systems.More infoParameter Estimation (PE) and State Estimation (SE) are the most wide-spreadtasks in the system engineering. They need to be done automatically, fast andfrequently, as measurements arrive. Deep Learning (DL) holds the promise oftackling the challenge, however in so far, as PE and SE in power systems isconcerned, (a) DL did not win trust of the system operators because of the lackof the physics of electricity based, interpretations and (b) DL remainedillusive in the operational regimes were data is scarce. To address this, wepresent a hybrid scheme which embeds physics modeling of power systems intoGraphical Neural Networks (GNN), therefore empowering system operators with areliable and explainable real-time predictions which can then be used tocontrol the critical infrastructure. To enable progress towards trustworthy DLfor PE and SE, we build a physics-informed method, named Power-GNN, whichreconstructs physical, thus interpretable, parameters within Effective PowerFlow (EPF) models, such as admittances of effective power lines, and NNparameters, representing implicitly unobserved elements of the system. In ourexperiments, we test the Power-GNN on different realistic power networks,including these with thousands of loads and hundreds of generators. We showthat the Power-GNN outperforms vanilla NN scheme unaware of the EPF physics.[Journal_ref: ]
- , L. P., , M. C., , J. F., & , P. J. (2022). Model Reduction of Swing Equations with Physics Informed PDE.More infoThis manuscript is the first step towards building a robust and efficientmodel reduction methodology to capture transient dynamics in a transmissionlevel electric power system. Such dynamics is normally modeled onseconds-to-tens-of-seconds time scales by the so-called swing equations, whichare ordinary differential equations defined on a spatially discrete model ofthe power grid. We suggest, following Seymlyen (1974) and Thorpe, Seyler andPhadke (1999), to map the swing equations onto a linear, inhomogeneous PartialDifferential Equation (PDE) of parabolic type in two space and one timedimensions with time-independent coefficients and properly defined boundaryconditions. The continuous two-dimensional spatial domain is defined by ageographical map of the area served by the power grid, and associated with thePDE coefficients derived from smoothed graph-Laplacian of susceptances, machineinertia and damping. Inhomogeneous source terms represent spatially distributedinjection/consumption of power. We illustrate our method on PanTaGruEl(Pan-European Transmission Grid and ELectricity generation model). We showthat, when properly coarse-grained, i.e. with the PDE coefficients and sourceterms extracted from a spatial convolution procedure of the respective discretecoefficients in the swing equations, the resulting PDE reproduces faithfullyand efficiently the original swing dynamics. We finally discuss futureextensions of this work, where the presented PDE-based reduced modeling willinitialize a physics-informed machine learning approach for real-time modeling,$n-1$ feasibility assessment and transient stability analysis of power systems.[Journal_ref: ]
- , M. K., , A. M., , A. E., , V. P., & , M. C. (2022). Prediction and Prevention of Pandemics via Graphical Model Inference and Convex Programming.More infoHard-to-predict bursts of COVID-19 pandemic revealed significance ofstatistical modeling which would resolve spatio-temporal correlations overgeographical areas, for example spread of the infection over a city with censustract granularity. In this manuscript, we provide algorithmic answers to thefollowing two inter-related public health challenges. (1) Inference Challenge:assuming that there are $N$ census blocks (nodes) in the city, and given aninitial infection at any set of nodes, what is the probability for a subset ofcensus blocks to become infected by the time the spread of the infection burstis stabilized? (2) Prevention Challenge: What is the minimal control action onecan take to minimize the infected part of the stabilized state footprint? Toanswer the challenges, we build a Graphical Model of pandemic of the attractiveIsing (pair-wise, binary) type, where each node represents a census track andeach edge factor represents the strength of the pairwise interaction between apair of nodes. We show that almost all attractive Ising Models on dense graphsresult in either of the two modes for the most probable state: either all nodeswhich were not infected initially became infected, or all the initiallyuninfected nodes remain uninfected. This bi-modal solution of the InferenceChallenge allows us to re-state the Prevention Challenge as the followingtractable convex programming: for the bare Ising Model with pair-wise and biasfactors representing the system without prevention measures, such that the MAPstate is fully infected for at least one of the initial infection patterns,find the closest, in $l_1$ norm, set of factors resulting in all the MAP statesof the Ising model, with the optimal prevention measures applied, to becomesafe.[Journal_ref: ]
- , M. W., , Y. T., , C. H., , C. F., , D. L., , M. S., & , M. C. (2022). Physics Informed Machine Learning of SPH: Machine Learning Lagrangian Turbulence.More infoSmoothed particle hydrodynamics (SPH) is a mesh-free Lagrangian method forobtaining approximate numerical solutions of the equations of fluid dynamics;which has been widely applied to weakly- and strongly compressible turbulencein astrophysics and engineering applications. We present a learn-able hierarchyof parameterized and "physics-explainable" SPH informed fluid simulators usingboth physics based parameters and Neural Networks (NNs) as universal functionapproximators. Our learning algorithm develops a mixed mode approach, mixingforward and reverse mode automatic differentiation with forward and adjointbased sensitivity analyses to efficiently perform gradient based optimization.We show that our physics informed learning method is capable of: (a) solvinginverse problems over the physically interpretable parameter space, as well asover the space of NN parameters; (b) learning Lagrangian statistics ofturbulence (interpolation); (c) combining Lagrangian trajectory based,probabilistic, and Eulerian field based loss functions; and (d) extrapolatingbeyond training sets into more complex regimes of interest. Furthermore, thishierarchy of models gradually introduces more physical structure, which we showimproves interpretability, generalizability (over larger ranges of time scalesand Reynolds numbers), preservation of physical symmetries, and requires lesstraining data.[Journal_ref: ]
- , N. S., & , M. C. (2022). Neural Particle Image Velocimetry.More infoIn the past decades, great progress has been made in the field of optical andparticle-based measurement techniques for experimental analysis of fluid flows.Particle Image Velocimetry (PIV) technique is widely used to identify flowparameters from time-consecutive snapshots of particles injected into thefluid. The computation is performed as post-processing of the experimental datavia proximity measure between particles in frames of reference. However, thepost-processing step becomes problematic as the motility and density of theparticles increases, since the data emerges in extreme rates and volumes.Moreover, existing algorithms for PIV either provide sparse estimations of theflow or require large computational time frame preventing from on-line use. Thegoal of this manuscript is therefore to develop an accurate on-line algorithmfor estimation of the fine-grained velocity field from PIV data. As the dataconstitutes a pair of images, we employ computer vision methods to solve theproblem. In this work, we introduce a convolutional neural network adapted tothe problem, namely Volumetric Correspondence Network (VCN) which was recentlyproposed for the end-to-end optical flow estimation in computer vision. Thenetwork is thoroughly trained and tested on a dataset containing both syntheticand real flow data. Experimental results are analyzed and compared to that ofconventional methods as well as other recently introduced methods based onneural networks. Our analysis indicates that the proposed approach providesimproved efficiency also keeping accuracy on par with other state-of-the-artmethods in the field. We also verify through a-posteriori tests that our newlyconstructed VCN schemes are reproducing well physically relevant statistics ofvelocity and velocity gradients.[Journal_ref: ]
- , Y. T., , D. L., & , M. C. (2022). Physics-Informed Machine Learning of the Lagrangian Dynamics of Velocity Gradient Tensor.More infoReduced models describing the Lagrangian dynamics of the Velocity GradientTensor (VGT) in Homogeneous Isotropic Turbulence (HIT) are developed under thePhysics-Informed Machine Learning (PIML) framework. We consider VGT at bothKolmogorov scale and coarse-grained scale within the inertial range of HIT.Building reduced models requires resolving the pressure Hessian and sub-filtercontributions, which is accomplished by constructing them using the integritybases and invariants of VGT. The developed models can be expressed using theextended Tensor Basis Neural Network (TBNN). Physical constraints, such asGalilean invariance, rotational invariance, and incompressibility condition,are thus embedded in the models explicitly. Our PIML models are trained on theLagrangian data from a high-Reynolds number Direct Numerical Simulation (DNS).To validate the results, we perform a comprehensive out-of-sample test. Weobserve that the PIML model provides an improved representation for themagnitude and orientation of the small-scale pressure Hessian contributions.Statistics of the flow, as indicated by the joint PDF of second and thirdinvariants of the VGT, show good agreement with the "ground-truth" DNS data. Anumber of other important features describing the structure of HIT arereproduced by the model successfully. We have also identified challenges inmodeling inertial range dynamics, which indicates that a richer modelingstrategy is required. This helps us identify important directions for futureresearch, in particular towards including inertial range geometry into TBNN.[Journal_ref: ]
Presentations
- Stepanov, M., & Chertkov, M. (2019, November). Towards (Machine) Learning of Large Eddy Lagrangian Models (of Turbulence). 72nd Annual Meeting of the APS Division of Fluid Dynamics. Seattle, WA, USA.More infoPresentation (by Michael Chertkov) of our joint work at APS meeting.