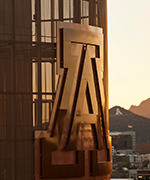
Liang Zhang
- Assistant Professor, Civil Engineering-Engineering Mechanics
- Member of the Graduate Faculty
Contact
- (520) 621-2266
- Civil Engineering, Rm. 206
- Tucson, AZ 85721
- liangzhang1@arizona.edu
Biography
Dr. Liang Zhang is an assistant professor of the Department of Civil and Architectural Engineering and Mechanics at the University of Arizona. He is the director of Building Energy & Sustainability Laboratory (BESLab). He is also a joint appointee at National Renewable Energy Laboratory (NREL). His research lies in the intersection of building decarbonization and physics-informed artificial intelligence. He led and worked on high-profile U.S. Department of Energy (DOE) and National Science Foundation (NSF) projects related to artificial intelligence in buildings, trans-scalar building energy modeling, smart and connected communities, and fault detection & diagnostics.Degrees
- Ph.D. Architectural Engineering
- Drexel University, Philadelphia, Pennsylvania, United States
- Data-driven whole building energy forecasting model for data predictive control
- M.S. Power Engineering
- Tongji University, Shanghai, China
- B.S. Electronic Science and Technology
- East China Normal University, Shanghai, China
Work Experience
- Joint Appointee - National Renewable Energy Laboratory (2023 - Ongoing)
- Research Scientist - National Renewable Energy Laboratory (2019 - 2022)
Interests
Teaching
Building Energy Modeling
Research
Dr. Zhang's research is broadly focused in the area of computing and modeling for smart buildings and cities to promote building energy efficiency, sustainability, and decarbonization. More specifically, his research has emphasized the following topics: 1) grid-interactive efficient building, 2) machine learning control in buildings, 3) fault detection and diagnostics in buildings, 4) smart and connected communities, 5) building energy modeling (building, community, and urban scale)
Courses
2024-25 Courses
-
Dissertation
CE 920 (Spring 2025) -
Spec Top in Bldg Sci
ARCE 497B (Spring 2025) -
Spec Top in Bldg Sci
ARCE 597B (Spring 2025) -
Thesis
CE 910 (Spring 2025) -
Dissertation
CE 920 (Fall 2024) -
Independent Study
CE 599 (Fall 2024) -
Spec Top in Arch Engr
ARCE 597A (Fall 2024)
2023-24 Courses
-
Independent Study
CE 599 (Spring 2024) -
Research Topics
CE 596A (Spring 2024) -
Spec Top in Bldg Sci
ARCE 497B (Spring 2024) -
Spec Top in Bldg Sci
ARCE 597B (Spring 2024) -
Dissertation
CE 920 (Fall 2023)
2022-23 Courses
-
Spec Top in Bldg Sci
ARCE 497B (Spring 2023)
Scholarly Contributions
Books
- Zhang, L. (2018). Data-driven whole building energy forecasting model for data predictive control. Drexel University.
Journals/Publications
- Zhang, L., Ford, V., Chen, Z., & Chen, J. (2025). Automatic building energy model development and debugging using large language models agentic workflow. Energy and Buildings, 327, 115116.
- Zhang, L., Ford, V., Chen, Z., & Chen, J. (2025). Automatic building energy model development and debugging using large language models agentic workflow. Energy and Buildings, 327. doi:10.1016/j.enbuild.2024.115116More infoBuilding energy modeling (BEM) is a complex process that demands significant time and expertise, limiting its broader application in building design and operations. While Large Language Models (LLMs) agentic workflow have facilitated complex engineering processes, their application in BEM has not been specifically explored. This paper investigates the feasibility of automating BEM using LLM agentic workflow. We developed a generic LLM-planning-based workflow that takes a building description as input and generates an error-free EnergyPlus building energy model. Our robust workflow includes four core agents: 1) Building Description Pre-Processing, 2) IDF Object Information Extraction, 3) Single IDF Object Generator Suite, and 4) IDF Debugging Agent. These agents divide the complex tasks into manageable sub-steps, enabling LLMs to generate accurate and reliable results at each stage. The case study demonstrates the successful translation of a building description into an error-free EnergyPlus model for the iUnit modular building at the National Renewable Energy Laboratory. The effectiveness of our workflow surpasses: 1) naive prompt engineering, 2) other LLM-based workflows, and 3) manual modeling, in terms of accuracy, reliability, and time efficiency. The paper concludes with a discussion on the interplay between foundational models and LLM agent planning design, advocating for the use of fine-tuned, specialized models to advance this field.
- Jiang, G., Ma, Z., Zhang, L., & Chen, J. (2024). EPlus-LLM: A large language model-based computing platform for automated building energy modeling. Applied Energy, 367, 123431.
- Jiang, G., Ma, Z., Zhang, L., & Chen, J. (2024). EPlus-LLM: A large language model-based computing platform for automated building energy modeling. Applied Energy, 367. doi:10.1016/j.apenergy.2024.123431More infoEstablishing building energy models (BEMs) for building design and analysis poses significant challenges due to demanding modeling efforts, expertise to use simulation software, and building science knowledge in practice. These make building modeling labor-intensive, hindering its widespread adoptions in building development. Therefore, to overcome these challenges in building modeling with enhanced automation in modeling practice, this paper proposes Eplus-LLM (EnergyPlus-Large Language Model) as the auto-building modeling platform, building on a fine-tuned large language model (LLM) to directly translate natural language description of buildings to established building models of various geometries, occupancy scenarios, and equipment loads. Through fine-tuning, the LLM (i.e., T5) is customized to digest natural language and simulation demands from users and convert human descriptions into EnergyPlus modeling files. Then, the Eplus-LLM platform realizes the automated building modeling through invoking the API of simulation software (i.e., the EnergyPlus engine) to simulate the auto-generated model files and output simulation results of interest. The validation process, involving four different types of prompts, demonstrates that Eplus-LLM reduces over 95% modeling efforts and achieves 100% accuracy in establishing BEMs while being robust to interference in usage, including but not limited to different tones, misspells, omissions, and redundancies. Overall, this research serves as the pioneering effort to customize LLM for auto-modeling purpose (directly build-up building models from natural language), aiming to provide a user-friendly human-AI interface that significantly reduces building modeling efforts. This work also further facilitates large-scale building model efforts, e.g., urban building energy modeling (UBEM), in modeling practice.
- Khadka, S. (2024). Interpretable Machine Learning Control in Building Energy Systems. ASHRAE Transactions, 130, 544--551.
- Khadka, S., & Zhang, L. (2024). Scaling Data-Driven Building Energy Modelling using Large Language Models. arXiv preprint arXiv:2407.03469.
- Zhang, L., & Chen, Z. (2024). Large Language Model-Based Interpretable Machine Learning Control in Building Energy Systems. arXiv preprint arXiv:2402.09584.
- Zhang, L., & Chen, Z. (2024). Large language model-based interpretable machine learning control in building energy systems. Energy and Buildings, 313, 114278.
- Zhang, L., Chen, Z., & Ford, V. (2024). Advancing Building Energy Modeling with Large Language Models: Exploration and Case Studies. arXiv preprint arXiv:2402.09579.
- Zhang, L., Haroon, S. M., & Ryan, A. (2024). Py-Cosim: Python-Based Building Energy Co-Simulation Infrastructure. SSRN.
- Zhang, L., Kaufman, Z., & Leach, M. (2024). Physics-informed hybrid modeling methodology for building infiltration. Energy and Buildings, 320, 114580.
- Zhang, L., Kaufman, Z., & Leach, M. (2024). Physics-informed hybrid modeling methodology for building infiltration. Energy and Buildings, 320. doi:10.1016/j.enbuild.2024.114580More infoInfiltration is responsible for one-third to one-half of the space conditioning load of a typical residential home, but the modeling of infiltration for building energy modeling is either represented by over-simplified equations or dependent on over-generalized rules of thumb. This paper develops a physics-informed data-driven methodology for modeling infiltration using building-specific empirical measurements. The developed hybrid methodology combines machine-learning categorization and grey-box sub-modeling to improve the accuracy and generalization of commonly used grey-box infiltration models. The developed methodology excels at predicting infiltration by improving the ability to predict infiltration under unseen environmental conditions using machine learning algorithms with physical significance. In a case study conducted using the iUnit, a modular studio apartment experimental test facility located at the National Renewable Energy Laboratory, we use empirical airtightness measurements to fit an infiltration model using the developed methodology. We find that the developed methodology can improve the overall model accuracy by 43% and improve extrapolation by 38%, compared with the model based on the common grey-box infiltration equation. We also notice that the selected features can improve the performance of a pure machine-learning model, indicating that our methodology identifies the features with the most physical significance to infiltration modeling.
- Zhang, L., & Chen, Z. (2023). Opportunities and Challenges of Applying Large Language Models in Building Energy Efficiency and Decarbonization Studies: An Exploratory Overview. arXiv preprint arXiv:2312.11701.
- Zhang, L., & Wen, J. (2017). A Systematic Feature Selection Procedure for Data-driven Building Energy Forecasting Model Development. ASHRAE Annual Conference.
- Zhang, L., Chen, J., & Zou, J. (2023). Taxonomy, Semantic Data Schema, and Schema Alignment for Open Data in Urban Building Energy Modeling. arXiv preprint arXiv:2311.08535.
- Zhang, L., Leach, M., Chen, J., & Hu, Y. (2023). Sensor cost-effectiveness analysis for data-driven fault detection and diagnostics in commercial buildings. Energy, 263, 125577.
- Chen, J., Zhang, L., Li, Y., Shi, Y., Gao, X., & Hu, Y. (2022). A review of computing-based automated fault detection and diagnosis of heating, ventilation and air conditioning systems. Renewable and Sustainable Energy Reviews, 161, 112395.
- Zhang, L., & Leach, M. (2022). Evaluate the impact of sensor accuracy on model performance in data-driven building fault detection and diagnostics using Monte Carlo simulation. Building Simulation, 15(5).
- Zhang, L., Chen, Z., Zhang, X., Pertzborn, A., & Jin, X. (2023). Challenges and Opportunities of Machine Learning Control in Building Operations. Building SImulation, TBD.
- Bae, Y., Bhattacharya, S., Cui, B., Lee, S., Li, Y., Zhang, L., Im, P., Adetola, V., Vrabie, D., Leach, M., & others, . (2021). Sensor impacts on building and HVAC controls: A critical review for building energy performance. Advances in Applied Energy, 4, 100068.
- Li, Y., O'Neill, Z., Zhang, L., Chen, J., Im, P., & DeGraw, J. (2021). Grey-box modeling and application for building energy simulations-A critical review. Renewable and Sustainable Energy Reviews, 146, 111174.
- Zhang, L. (2021). Data-driven building energy modeling with feature selection and active learning for data predictive control. Energy and Buildings, 252, 111436.
- Zhang, L., & Wen, J. (2021). Active learning strategy for high fidelity short-term data-driven building energy forecasting. Energy and Buildings, 244, 111026.
- Zhang, L., Alahmad, M., & Wen, J. (2021). Comparison of time-frequency-analysis techniques applied in building energy data noise cancellation for building load forecasting: A real-building case study. Energy and Buildings, 231, 110592.
- Zhang, L., Leach, M., Bae, Y., Cui, B., Bhattacharya, S., Lee, S., Im, P., Adetola, V., Vrabie, D., & Kuruganti, T. (2021). Sensor impact evaluation and verification for fault detection and diagnostics in building energy systems: A review. Advances in Applied Energy, 3, 100055.
- Zhang, L., Plathottam, S., Reyna, J., Merket, N., Sayers, K., Yang, X., Reynolds, M., Parker, A., Wilson, E., Fontanini, A., & others, . (2021). High-resolution hourly surrogate modeling framework for physics-based large-scale building stock modeling. Sustainable Cities and Society, 75, 103292.
- Zhang, L., Wen, J., Li, Y., Chen, J., Ye, Y., Fu, Y., & Livingood, W. (2021). A review of machine learning in building load prediction. Applied Energy, 285, 116452.
- Bianchi, C., Zhang, L., Goldwasser, D., Parker, A., & Horsey, H. (2020). Modeling occupancy-driven building loads for large and diversified building stocks through the use of parametric schedules. Applied Energy, 276, 115470.
- Im, P., Bae, Y., Cui, B., Lee, S., Bhattacharya, S., Adetola, V., Vrabie, D., Zhang, L., & Leach, M. (2020). Literature Review for Sensor Impact Evaluation and Verification Use Cases-Building Controls and Fault Detection and Diagnosis (FDD).
- Zhang, L. (2020). A pattern-recognition-based ensemble data imputation framework for sensors from building energy systems. Sensors, 20(20), 5947.
- Zhang, L., Frank, S., Kim, J., Jin, X., & Leach, M. (2020). A systematic feature extraction and selection framework for data-driven whole-building automated fault detection and diagnostics in commercial buildings. Building and Environment, 186, 107338.
- Zhang, L., & Wen, J. (2019). A systematic feature selection procedure for short-term data-driven building energy forecasting model development. Energy and Buildings, 183, 428--442.
- Zhang, L., & Wen, J. (2018). Apply Active Learning in Short-term Data-driven Building Energy Modeling.
- Zhang, L., Wen, J., Cui, C., Li, X., & Wu, T. (2016). Experiment Design and Training Data Quality of Inverse Model for Short-term Building Energy Forecasting.
- Zhang, L., Xu, P., Mao, J., Tang, X. u., Li, Z., & Shi, J. (2015). A low cost seasonal solar soil heat storage system for greenhouse heating: Design and pilot study. Applied Energy, 156, 213--222.
Proceedings Publications
- Sharma, A., Li, X., Guan, H., Sun, G., Zhang, L., Wang, L., Wu, K., Cao, L., Zhu, E., Sim, A., & others, . (2023). Automatic Data Transformation Using Large Language Model-An Experimental Study on Building Energy Data. In 2023 IEEE International Conference on Big Data (BigData).
- Pradhan, O., Pertzborn, A., Zhang, L., & Wen, J. (2020). Development and Validation of a Simulation Testbed for the Intelligent Building Agents Laboratory (IBAL) Using TRNSYS (VC-20-C056). In 2020 ASHRAE Virtual Conference.
- Zhang, L., Wen, J., & Chen, Y. (2017). Systematic Feature Selection Process Applied in Short-Term Data-Driven Building Energy Forecasting Models: A Case Study of a Campus Building. In Dynamic Systems and Control Conference, 58295.
- Liu, C., Xu, P., Chen, W., Zhang, L., & Li, W. (2015). Study of Urban Patterns Optimization Employing CFD Method: A Case Study of Chenjiazhen Experimental Ecological Community, Chongming, Shanghai. In Building Resilient Cities in China: The Nexus between Planning and Science: Selected Papers from the 7th International Association for China Planning Conference, Shanghai, China, June 29--July 1, 2013.
- Zhang, L., Xu, P., & Li, Z. W. (2014). Relationship between Energy Consumption and Service Level: A Survey of Class A Office Buildings in Shanghai. In Advanced Materials Research, 953.
- Zhang, L., Xu, P., Mao, J. C., & Tang, X. u. (2014). Design and application of a seasonal solar soil heat storage system applied in greenhouse heating. In Applied Mechanics and Materials, 672.
Others
- Horsey, H., Parker, A., Farthing, A., Dahlhausen, M., Praprost, M., Bianchi, C., Robertson, J., Horowitz, S., Zhang, L., Ringold, E., & others, . (2025). ComStock™ 2024 Release 1 [SWR-19-33 and SWR-20-32].
- Bae, Y., Cui, B., Joe, J., Im, P., Adetola, V., Zhang, L., Leach, M., & Kuruganti, T. (2020, 1). Sensor Impact on Building Controls and Automatic Fault Detection and Diagnosis (AFDD).
- Frank, S. M., Lin, G., Jin, X., Singla, R., Farthing, A., Zhang, L., & Granderson, J. (2019, 1). Metrics and methods to assess building fault detection and diagnosis tools.
- Horsey, H., Parker, A., Farthing, A., Dahlhausen, M., Praprost, M., Bianchi, C., Robertson, J., Horowitz, S., & Zhang, L. (2020, 1). ComStock™.
- Im, P., Bae, Y., Cui, B., Lee, S., Bhattacharya, S., Adetola, V., Vrabie, D., Zhang, L., & Leach, M. (2020, 1). Sensor Impacts Evaluation and Verification: Expert Interview Responses.
- Wilson, E. J., Parker, A., Fontanini, A., Present, E., Reyna, J. L., Adhikari, R., Bianchi, C., CaraDonna, C., Dahlhausen, M., Kim, J., & others, . (2022, March). End-Use Load Profiles for the US Building Stock: Methodology and Results of Model Calibration, Validation, and Uncertainty Quantification.