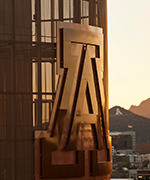
Lifeng Lin
- Associate Professor, Public Health
- Member of the Graduate Faculty
Contact
- (520) 000-0000
- Roy P. Drachman Hall, Rm. 200
- Tucson, AZ 85721
- lifenglin@arizona.edu
Biography
Lifeng Lin is an Associate Professor in the Department of Epidemiology and Biostatistics at the Mel and Enid Zuckerman College of Public Health at the University of Arizona. Prior to joining the UA, he was an Assistant Professor in the Department of Statistics at Florida State University from August 2017 to June 2022. He obtained his Ph.D. in biostatistics from the University of Minnesota in 2017. His research focuses on statistical methods for meta-analysis, network meta-analysis of multiple-treatment comparisons, publication bias, and Bayesian methods. He is also interested in the applications of statistical methods to real-world problems and approaches to improving research reproducibility and replicability.Degrees
- Ph.D. Biostatistics
- University of Minnesota, Minneapolis, Minnesota, United States
- B.S. Statistics
- University of Science and Technology of China, Hefei, China
Work Experience
- Florida State University, Tallahassee, Florida (2017 - 2022)
Awards
- Early Career Award by the Society for Research Synthesis and Methodology
- Society for Research Synthesis and Methodology, Summer 2023
Interests
Teaching
Applied Statistics
Research
Bayesian Analysis; Biostatistics; Meta-Analysis; Network Meta-Analysis; Publication bias
Courses
2024-25 Courses
-
Biostatistics Seminar
BIOS 696S (Fall 2024)
2023-24 Courses
-
Bayesian Stat Thry+Appli
BIOS 574B (Spring 2024) -
Bayesian Stat Thry+Appli
STAT 574B (Spring 2024) -
Biostatistical Methods I
BIOS 680 (Spring 2024) -
Biostatistics Seminar
BIOS 696S (Spring 2024) -
Research
BIOS 900 (Spring 2024) -
Biostatistics Seminar
BIOS 696S (Fall 2023) -
Research
BIOS 900 (Fall 2023)
2022-23 Courses
-
Independent Study
BIOS 699 (Spring 2023) -
Special Topics: Biostatistics
BIOS 685 (Spring 2023)
Scholarly Contributions
Journals/Publications
- Chu, H., Lin, L., Wang, Z., Wang, Z., Chen, Y., & Cappelleri, J. C. (2024). A review and comparison of arm-based versus contrast-based network meta-analysis for binary outcomes—Understanding their differences and limitations. Wiley Interdisciplinary Reviews: Computational Statistics, 16(1), e1639. doi:10.1002/wics.1639
- Xiao, M., Chu, H., Hodges, J. S., & Lin, L. (2024). Quantifying replicability of multiple studies in a meta-analysis. The Annals of Applied Statistics, 18(1), 664--682. doi:10.1214/23-AOAS1806
- Dai, M., Furuya-Kanamori, L., Syed, A., Lin, L., & Wang, Q. (2023). An empirical comparison of the harmful effects for randomized controlled trials and non-randomized studies of interventions. Frontiers in pharmacology, 14, 1064567.More infoRandomized controlled trials (RCTs) are the gold standard to evaluate the efficacy of interventions (e.g., drugs and vaccines), yet the sample size of RCTs is often limited for safety assessment. Non-randomized studies of interventions (NRSIs) had been proposed as an important alternative source for safety assessment. In this study, we aimed to investigate whether there is any difference between RCTs and NRSIs in the evaluation of adverse events. We used the dataset of systematic reviews with at least one meta-analysis including both RCTs and NRSIs and collected the 2 × 2 table information (i.e., numbers of cases and sample sizes in intervention and control groups) of each study in the meta-analysis. We matched RCTs and NRSIs by their sample sizes (ratio: 0.85/1 to 1/0.85) within a meta-analysis. We estimated the ratio of the odds ratios (RORs) of an NRSI against an RCT in each pair and used the inverse variance as the weight to combine the natural logarithm of ROR (lnROR). We included systematic reviews with 178 meta analyses, from which we confirmed 119 pairs of RCTs and NRSIs. The pooled ROR of NRSIs compared to that of RCTs was estimated to be 0.96 (95% confidence interval: 0.87 and 1.07). Similar results were obtained with different sample size subgroups and treatment subgroups. With the increase in sample size, the difference in ROR between RCTs and NRSIs decreased, although not significantly. There was no substantial difference in the effects between RCTs and NRSIs in safety assessment when they have similar sample sizes. Evidence from NRSIs might be considered a supplement to RCTs for safety assessment.
- Davis, J. D., Sanchez-Ramos, L., McKinney, J. A., Lin, L., & Kaunitz, A. M. (2023). Intrapartum amnioinfusion reduces meconium aspiration syndrome and improves neonatal outcomes in patients with meconium-stained fluid: a systematic review and meta-analysis. American journal of obstetrics and gynecology, 228(5S), S1179-S1191.e19.More infoThis study aimed to reassess the effect of prophylactic transcervical amnioinfusion for intrapartum meconium-stained amniotic fluid on meconium aspiration syndrome and other adverse neonatal and maternal outcomes.
- Duan, R., Tong, J., Lin, L., Levine, L., Sammel, M., Stoddard, J., Li, T., Schmid, C. H., Chu, H., & Chen, Y. (2023). PALM: Patient-centered treatment ranking via large-scale multivariate network meta-analysis. Annals of Applied Statistics, 17(1), 815-837. doi:10.1214/22-AOAS1652
- Guo, J., Xiao, M., Chu, H., & Lin, L. (2023). Meta-analysis methods for risk difference: A comparison of different models. Statistical methods in medical research, 32(1), 3-21.More infoRisk difference is a frequently-used effect measure for binary outcomes. In a meta-analysis, commonly-used methods to synthesize risk differences include: (1) the two-step methods that estimate study-specific risk differences first, then followed by the univariate common-effect model, fixed-effects model, or random-effects models; and (2) the one-step methods using bivariate random-effects models to estimate the summary risk difference from study-specific risks. These methods are expected to have similar performance when the number of studies is large and the event rate is not rare. However, studies with zero events are common in meta-analyses, and bias may occur with the conventional two-step methods from excluding zero-event studies or using an artificial continuity correction to zero events. In contrast, zero-event studies can be included and modeled by bivariate random-effects models in a single step. This article compares various methods to estimate risk differences in meta-analyses. Specifically, we present two case studies and three simulation studies to compare the performance of conventional two-step methods and bivariate random-effects models in the presence or absence of zero-event studies. In conclusion, we recommend researchers using bivariate random-effects models to estimate risk differences in meta-analyses, particularly in the presence of zero events.
- Liu, Z., Al Amer, F. M., Xiao, M., Xu, C., Furuya-Kanamori, L., Hong, H., Siegel, L., & Lin, L. (2023). The normality assumption on between-study random effects was questionable in a considerable number of Cochrane meta-analyses. BMC medicine, 21(1), 112.More infoStudies included in a meta-analysis are often heterogeneous. The traditional random-effects models assume their true effects to follow a normal distribution, while it is unclear if this critical assumption is practical. Violations of this between-study normality assumption could lead to problematic meta-analytical conclusions. We aimed to empirically examine if this assumption is valid in published meta-analyses.
- Meng, Z., Wu, C., & Lin, L. (2023). THE EFFECT DIRECTION SHOULD BE TAKEN INTO ACCOUNT WHEN ASSESSING SMALL-STUDY EFFECTS. The journal of evidence-based dental practice, 23(1), 101830.More infoStudies with statistically significant results are frequently more likely to be published than those with non-significant results. This phenomenon leads to publication bias or small-study effects and can seriously affect the validity of the conclusion from systematic reviews and meta-analyses. Small-study effects typically appear in a specific direction, depending on whether the outcome of interest is beneficial or harmful, but this direction is rarely taken into account in conventional methods.
- Murad, M. H., Lin, L., Chu, H., Hasan, B., Alsibai, R. A., Abbas, A. S., Mustafa, R. A., & Wang, Z. (2023). The association of sensitivity and specificity with disease prevalence: analysis of 6909 studies of diagnostic test accuracy. CMAJ : Canadian Medical Association journal = journal de l'Association medicale canadienne, 195(27), E925-E931.More infoSensitivity and specificity are characteristics of a diagnostic test and are not expected to change as the prevalence of the target condition changes. We sought to evaluate the association between prevalence and changes in sensitivity and specificity.
- Murad, M. H., Verbeek, J., Schwingshackl, L., Filippini, T., Vinceti, M., Akl, E. A., Morgan, R. L., Mustafa, R. A., Zeraatkar, D., Senerth, E., Street, R., Lin, L., Falck-Ytter, Y., Guyatt, G., Schünemann, H. J., & , G. W. (2023). GRADE GUIDANCE 38: Updated guidance for rating up certainty of evidence due to a dose-response gradient. Journal of clinical epidemiology, 164, 45-53.More infoThis updated guidance from the Grading of Recommendations Assessment, Development, and Evaluation addresses rating up certainty of evidence due to a dose-response gradient (DRG) observed in synthesis of intervention and exposure studies.
- Murad, M. H., Wang, Z., Chu, H., Lin, L., El Mikati, I. K., Khabsa, J., Akl, E. A., Nieuwlaat, R., Schuenemann, H. J., & Riaz, I. B. (2023). Proposed triggers for retiring a living systematic review. BMJ evidence-based medicine, 28(5), 348-352.More infoLiving systematic reviews (LSRs) are systematic reviews that are continually updated, incorporating relevant new evidence as it becomes available. LSRs are critical for decision-making in topics where the evidence continues to evolve. It is not feasible to continue to update LSRs indefinitely; however, guidance on when to retire LSRs from the living mode is not clear. We propose triggers for making such a decision. The first trigger is to retire LSRs when the evidence becomes conclusive for the outcomes that are required for decision-making. Conclusiveness of evidence is best determined based on the GRADE certainty of evidence construct, which is more comprehensive than solely relying on statistical considerations. The second trigger to retire LSRs is when the question becomes less pertinent for decision-making as determined by relevant stakeholders, including people affected by the problem, healthcare professionals, policymakers and researchers. LSRs can also be retired from a living mode when new studies are not anticipated to be published on the topic and when resources become unavailable to continue updating. We describe examples of retired LSRs and apply the proposed approach using one LSR about adjuvant tyrosine kinase inhibitors in high-risk renal cell carcinoma that we retired from a living mode and published its last update.
- Murad, M. H., Wang, Z., Zhu, Y., Saadi, S., Chu, H., & Lin, L. (2023). Methods for deriving risk difference (absolute risk reduction) from a meta-analysis. BMJ (Clinical research ed.), 381, e073141.
- Sanchez-Ramos, L., Lin, L., & Romero, R. (2023). Beware of references when using ChatGPT as a source of information to write scientific articles. American journal of obstetrics and gynecology, 229(3), 356-357.
- Tong, J., Luo, C., Sun, Y., Duan, R., Saine, E., Lin, L., Peng, Y., Lu, Y., Batra, A., Pan, A., Wang, O., Li, R., Marks-Anglin, A., Yang, Y., Zuo, X., Liu, Y., Bian, J., Kimmel, S. E., Hamilton, K., , Cuker, A., et al. (2023). Confidence Score: A Data-Driven Measure for Inclusive Systematic Reviews Considering Unpublished Preprints. Journal of the American Medical Informatics Association : JAMIA.More infoCOVID-19, since its emergence in December 2019, has globally impacted research. Over 360,000 COVID-19-related manuscripts have been published on PubMed and preprint servers like medRxiv and bioRxiv, with preprints comprising about 15% of all manuscripts. Yet, the role and impact of preprints on COVID-19 research and evidence synthesis remain uncertain.
- Wang, Z., Murray, T. A., Xiao, M., Lin, L., Alemayehu, D., & Chu, H. (2023). Bayesian hierarchical models incorporating study-level covariates for multivariate meta-analysis of diagnostic tests without a gold standard with application to COVID-19. Statistics in medicine, 42(28), 5085-5099.More infoWhen evaluating a diagnostic test, it is common that a gold standard may not be available. One example is the diagnosis of SARS-CoV-2 infection using saliva sampling or nasopharyngeal swabs. Without a gold standard, a pragmatic approach is to postulate a "reference standard," defined as positive if either test is positive, or negative if both are negative. However, this pragmatic approach may overestimate sensitivities because subjects infected with SARS-CoV-2 may still have double-negative test results even when both tests exhibit perfect specificity. To address this limitation, we propose a Bayesian hierarchical model for simultaneously estimating sensitivity, specificity, and disease prevalence in the absence of a gold standard. The proposed model allows adjusting for study-level covariates. We evaluate the model performance using an example based on a recently published meta-analysis on the diagnosis of SARS-CoV-2 infection and extensive simulations. Compared with the pragmatic reference standard approach, we demonstrate that the proposed Bayesian method provides a more accurate evaluation of prevalence, specificity, and sensitivity in a meta-analytic framework.
- Xing, A., & Lin, L. (2023). Empirical assessment of fragility index based on a large database of clinical studies in the Cochrane Library. Journal of evaluation in clinical practice, 29(2), 359-370.More infoThe fragility index (FI) and fragility quotient (FQ) are increasingly used measures for assessing the robustness of clinical studies with binary outcomes in terms of statistical significance. The FI is the minimum number of event status modifications that can alter a study result's statistical significance (or nonsignificance), and the FQ is calculated as the FI divided by the study's total sample size. The literature has no widely recognized criteria for interpreting the fragility measures' magnitudes. This article aims to provide an empirical assessment for the FI and FQ based on a large database of clinical studies in the Cochrane Library.
- Xu, C., Furuya-Kanamori, L., Lin, L., Zorzela, L., Yu, T., & Vohra, S. (2023). Measuring the impact of zero-cases studies in evidence synthesis practice using the harms index and benefits index (Hi-Bi). BMC medical research methodology, 23(1), 61.More infoIn evidence synthesis practice, dealing with studies with no cases in both arms has been a tough problem, for which there is no consensus in the research community. In this study, we propose a method to measure the potential impact of studies with no cases for meta-analysis results which we define as harms index (Hi) and benefits index (Bi) as an alternative solution for deciding how to deal with such studies.
- Zhou, J., Yang, J., S. Hodges, J., Lin, L., & Chu, H. (2023).
Estimating Causal Effects using Bayesian Methods with the R Package BayesCACE
. The R Journal, 15(1), 297-315. doi:10.32614/rj-2023-038 - Zhu, Y., Ren, P., Doi, S. A., Furuya-Kanamori, L., Lin, L., Zhou, X., Tao, F., & Xu, C. (2023). Data extraction error in pharmaceutical versus non-pharmaceutical interventions for evidence synthesis: Study protocol for a crossover trial. Contemporary clinical trials communications, 35, 101189.More infoData extraction is the foundation for research synthesis evidence, while data extraction errors frequently occur in the literature. An interesting phenomenon was observed that data extraction error tend to be more common in trials of pharmaceutical interventions compared to non-pharmaceutical ones. The elucidation of which would have implications for guidelines, practice, and policy.
- Aloe, A. M., Thompson, C. G., Liu, Z., & Lin, L. (2022). Estimating Partial Standardized Mean Differences from Regression Models. The Journal of Experimental Education, 90(4), 898-915. doi:10.1080/00220973.2021.1966605
- Atieh, M. A., Almutairi, Z., Amir-Rad, F., Koleilat, M., Tawse-Smith, A., Ma, S., Lin, L., & Alsabeeha, N. H. (2022). A Retrospective Analysis of Biological Complications of Dental Implants. International journal of dentistry, 2022, 1545748.More infoA retrospective analysis of patients aged ≥18 years and having dental implants placed at Dubai Health Authority in 2010. Relevant information related to systemic-, patient-, implant-, site-, surgical- and prosthesis-related factors were collected. The strength of association between the prevalence of peri-implant mucositis and peri-implantitis and each variable was measured by chi-square analysis. A binary logistic regression analysis was performed to identify possible risk factors.
- Atieh, M. A., Alnaqbi, M., Abdunabi, F., Lin, L., & Alsabeeha, N. H. (2022). Alveolar ridge preservation in extraction sockets of periodontally compromised teeth: A systematic review and meta-analysis. Clinical oral implants research, 33(9), 869-885.More infoAlveolar ridge preservation (ARP) procedures can limit bone changes following tooth extraction. However, the role of ARP in periodontally compromised socket lacks strong scientific evidence. The aim of this systematic review and meta-analysis was to evaluate the outcomes of ARP following extraction of periodontally compromised teeth in comparison with extraction alone in terms of hard tissue changes, need for additional augmentation at the time of implant placement, and patient-reported outcomes.
- Doi, S. A., Furuya-Kanamori, L., Xu, C., Chivese, T., Lin, L., Musa, O. A., Hindy, G., Thalib, L., & Harrell, F. E. (2022). The Odds Ratio is "portable" across baseline risk but not the Relative Risk: Time to do away with the log link in binomial regression. Journal of clinical epidemiology, 142, 288-293.More infoIn a recent paper we suggest that the relative risk (RR) be replaced with the odds ratio (OR) as the effect measure of choice in clinical epidemiology. In response, Chu, and colleagues raise several points that argue for the status quo. In this paper, we respond to their response.
- Doi, S. A., Furuya-Kanamori, L., Xu, C., Lin, L., Chivese, T., & Thalib, L. (2022). Controversy and Debate: Questionable utility of the relative risk in clinical research: Paper 1: A call for change to practice. Journal of clinical epidemiology, 142, 271-279.More infoIn clinical trials, the relative risk or risk ratio (RR) is a mainstay of reporting of the effect magnitude for an intervention. The RR is the ratio of the probability of an outcome in an intervention group to its probability in a control group. Thus, the RR provides a measure of change in the likelihood of an event linked to a given intervention. This measure has been widely used because it is today considered a measure with "portability" across varying outcome prevalence, especially when the outcome is rare. It turns out, however, that there is a much more important problem with this ratio, and this paper aims to demonstrate this problem.
- Furuya-Kanamori, L., Lin, L., & Doi, S. A. (2022). Comment on a review of methods to assess publication and other reporting biases in meta-analysis. Research synthesis methods, 13(4), 390-391.
- Furuya-Kanamori, L., Lin, L., Kostoulas, P., Clark, J., & Xu, C. (2023). Limits in the search date for rapid reviews of diagnostic test accuracy studies. Research synthesis methods, 14(2), 173-179. doi:10.1002/jrsm.1598More infoLimiting the search date is a common approach utilised in therapeutic/interventional rapid reviews. Yet the accuracy of pooled estimates is unknown when applied to rapid reviews of diagnostic test accuracy studies. Data from all systematic reviews of diagnostic test accuracy studies published in the Cochrane Database of Systematic Reviews, until February 2022 were collected. Meta-analyses with at least five studies were included to emulate rapid reviews by limiting the search to the recent 1, 2, 3, 4, 5, 10, 15, 20, 25, 30, 35 and 40 years. The magnitude of the pooled area under the curve (AUC), sensitivity and specificity for the full meta-analysis and the rapid reviews were compared. A total of 846 diagnostic meta-analyses were included. When the search date was limited to the recent 10 and 15 years, more than 75% and 80% of meta-analyses presented less than 5% difference between the pooled AUC, sensitivity and specificity of the full meta-analysis and the rapid review. There was little gain in the precision of the pooled estimates when the emulated rapid reviews included more than 15 years in the search. Rapid reviews restricted by search date are a valid and reliable approach for diagnostic test accuracy studies. Robust evidence can be achieved by restricting the search date to the recent 10-15 years. Future studies need to examine the reduction in workload and time to finish the rapid reviews under different search date limits.
- Jing, Y., Murad, M. H., & Lin, L. (2022). A Bayesian model for combining standardized mean differences and odds ratios in the same meta-analysis. Journal of biopharmaceutical statistics, 1-24.More infoIn meta-analysis practice, researchers frequently face studies that report the same outcome differently, such as a continuous variable (e.g., scores for rating depression) or a binary variable (e.g., counts of patients with depression dichotomized by certain latent and unreported depression scores). For combining these two types of studies in the same analysis, a simple conversion method has been widely used to handle standardized mean differences (SMDs) and odds ratios (ORs). This conventional method uses a linear function connecting the SMD and log OR; it assumes logistic distributions for (latent) continuous measures. However, the normality assumption is more commonly used for continuous measures, and the conventional method may be inaccurate when effect sizes are large or cutoff values for dichotomizing binary events are extreme (leading to rare events). This article proposes a Bayesian hierarchical model to synthesize SMDs and ORs without using the conventional conversion method. This model assumes exact likelihoods for continuous and binary outcome measures, which account for full uncertainties in the synthesized results. We performed simulation studies to compare the performance of the conventional and Bayesian methods in various settings. The Bayesian method generally produced less biased results with smaller mean squared errors and higher coverage probabilities than the conventional method in most cases. Nevertheless, this superior performance depended on the normality assumption for continuous measures; the Bayesian method could lead to nonignorable biases for non-normal data. In addition, we used two case studies to illustrate the proposed Bayesian method in real-world settings.
- Li, L., Asemota, I., Liu, B., Gomez-Valencia, J., Lin, L., Arif, A. W., Siddiqi, T. J., & Usman, M. S. (2022). AMSTAR 2 appraisal of systematic reviews and meta-analyses in the field of heart failure from high-impact journals. Systematic reviews, 11(1), 147.More infoThe Measurement Tool to Assess systematic Reviews (AMSTAR) 2 is a critical appraisal tool for systematic reviews (SRs) and meta-analyses (MAs) of interventions. We aimed to perform the first AMSTAR 2-based quality assessment of heart failure-related studies.
- Lin, L., & Chu, H. (2022). Assessing and visualizing fragility of clinical results with binary outcomes in R using the fragility package. PloS one, 17(6), e0268754.More infoWith the growing concerns about research reproducibility and replicability, the assessment of scientific results' fragility (or robustness) has been of increasing interest. The fragility index was proposed to quantify the robustness of statistical significance of clinical studies with binary outcomes. It is defined as the minimal event status modifications that can alter statistical significance. It helps clinicians evaluate the reliability of the conclusions. Many factors may affect the fragility index, including the treatment groups in which event status is modified, the statistical methods used for testing for the association between treatments and outcomes, and the pre-specified significance level. In addition to assessing the fragility of individual studies, the fragility index was recently extended to both conventional pairwise meta-analyses and network meta-analyses of multiple treatment comparisons. It is not straightforward for clinicians to calculate these measures and visualize the results. We have developed an R package called "fragility" to offer user-friendly functions for such purposes. This article provides an overview of methods for assessing and visualizing the fragility of individual studies as well as pairwise and network meta-analyses, introduces the usage of the "fragility" package, and illustrates the implementations with several worked examples.
- Lin, L., Xing, A., Chu, H., Murad, M. H., Xu, C., Baer, B. R., Wells, M. T., & Sanchez-Ramos, L. (2022). Assessing the robustness of results from clinical trials and meta-analyses with the fragility index. American journal of obstetrics and gynecology.More infoThe fragility index has been increasingly used to assess the robustness of the results of clinical trials since 2014. It aims at finding the smallest number of event changes that could alter originally statistically significant results. Despite its popularity, some researchers have expressed several concerns about the validity and usefulness of the fragility index. It offers a comprehensive review of the fragility index's rationale, calculation, software, and interpretation, with emphasis on application to studies in obstetrics and gynecology. This article presents the fragility index in the settings of individual clinical trials, standard pairwise meta-analyses, and network meta-analyses. Moreover, this article provides worked examples to demonstrate how the fragility index can be appropriately calculated and interpreted. In addition, the limitations of the traditional fragility index and some solutions proposed in the literature to address these limitations were reviewed. In summary, the fragility index is recommended to be used as a supplemental measure in the reporting of clinical trials and a tool to communicate the robustness of trial results to clinicians. Other considerations that can aid in the fragility index's interpretation include the loss to follow-up and the likelihood of data modifications that achieve the loss of statistical significance.
- Lin, L., Xu, C., & Chu, H. (2022). Empirical Comparisons of 12 Meta-analysis Methods for Synthesizing Proportions of Binary Outcomes. Journal of general internal medicine, 37(2), 308-317.More infoMeta-analysis is increasingly used to synthesize proportions (e.g., disease prevalence). It can be implemented with widely used two-step methods or one-step methods, such as generalized linear mixed models (GLMMs). Existing simulation studies have shown that GLMMs outperform the two-step methods in some settings. It is, however, unclear whether these simulation settings are common in the real world. We aim to compare the real-world performance of various meta-analysis methods for synthesizing proportions.
- Luo, C., Marks-Anglin, A., Duan, R., Lin, L., Hong, C., Chu, H., & Chen, Y. (2022). Accounting for publication bias using a bivariate trim and fill meta-analysis procedure. Statistics in medicine, 41(18), 3466-3478.More infoIn research synthesis, publication bias (PB) refers to the phenomenon that the publication of a study is associated with the direction and statistical significance of its results. Consequently, it may lead to biased (commonly optimistic) estimates of treatment effects. Visualization tools such as funnel plots have been widely used to investigate PB in univariate meta-analyses. The trim and fill procedure is a nonparametric method to identify and adjust for PB. It is popular among applied scientists due to its simplicity. However, most visualization tools and PB correction methods focus on univariate outcomes. For a meta-analysis with multiple outcomes, the conventional univariate trim and fill method can only account for different outcomes separately and thus may lead to inconsistent conclusions. In this article, we propose a bivariate trim and fill procedure to simultaneously account for PB in the presence of two outcomes that are possibly associated. Based on a recently developed galaxy plot for bivariate meta-analysis, the proposed procedure uses a data-driven imputation algorithm to detect and adjust PB. The method relies on the symmetry of the galaxy plot and assumes that some studies are suppressed based on a linear combination of outcomes. The method projects bivariate outcomes along a particular direction, uses the univariate trim and fill method to estimate the number of trimmed and filled studies, and yields consistent conclusions about PB. The proposed approach is validated using simulated data and is applied to a meta-analysis of the efficacy and safety of antidepressant drugs.
- Rosenberger, K. J., Chu, H., & Lin, L. (2022). Empirical comparisons of meta-analysis methods for diagnostic studies: a meta-epidemiological study. BMJ open, 12(5), e055336.More infoSeveral methods are commonly used for meta-analyses of diagnostic studies, such as the bivariate linear mixed model (LMM). It estimates the overall sensitivity, specificity, their correlation, diagnostic OR (DOR) and the area under the curve (AUC) of the summary receiver operating characteristic (ROC) estimates. Nevertheless, the bivariate LMM makes potentially unrealistic assumptions (ie, normality of within-study estimates), which could be avoided by the bivariate generalised linear mixed model (GLMM). This article aims at investigating the real-world performance of the bivariate LMM and GLMM using meta-analyses of diagnostic studies from the Cochrane Library.
- Sanchez-Ramos, L., & Lin, L. (2022). Cerclage placement in twin pregnancies with short or dilated cervix does not prevent preterm birth: a fragility index assessment. American journal of obstetrics and gynecology, 227(2), 338-339.
- Wang, Y., Lin, L., Thompson, C. G., & Chu, H. (2022). A penalization approach to random-effects meta-analysis. Statistics in medicine, 41(3), 500-516.More infoSystematic reviews and meta-analyses are principal tools to synthesize evidence from multiple independent sources in many research fields. The assessment of heterogeneity among collected studies is a critical step when performing a meta-analysis, given its influence on model selection and conclusions about treatment effects. A common-effect (CE) model is conventionally used when the studies are deemed homogeneous, while a random-effects (RE) model is used for heterogeneous studies. However, both models have limitations. For example, the CE model produces excessively conservative confidence intervals with low coverage probabilities when the collected studies have heterogeneous treatment effects. The RE model, on the other hand, assigns higher weights to small studies compared to the CE model. In the presence of small-study effects or publication bias, the over-weighted small studies from a RE model can lead to substantially biased overall treatment effect estimates. In addition, outlying studies may exaggerate between-study heterogeneity. This article introduces penalization methods as a compromise between the CE and RE models. The proposed methods are motivated by the penalized likelihood approach, which is widely used in the current literature to control model complexity and reduce variances of parameter estimates. We compare the existing and proposed methods with simulated data and several case studies to illustrate the benefits of the penalization methods.
- Xu, C., Doi, S. A., Zhou, X., Lin, L., Furuya-Kanamori, L., & Tao, F. (2022). Data reproducibility issues and their potential impact on conclusions from evidence syntheses of randomized controlled trials in sleep medicine. Sleep medicine reviews, 66, 101708.More infoIn this study, we examined the data reproducibility issues in systematic reviews in sleep medicine. We searched for systematic reviews of randomized controlled trials published in sleep medicine journals. The metadata in meta-analyses among the eligible systematic reviews were collected. The original sources of the data were reviewed to see if the components used in the meta-analyses were correctly extracted or estimated. The impacts of the data reproducibility issues were investigated. We identified 48 systematic reviews with 244 meta-analyses of continuous outcomes and 54 of binary outcomes. Our results suggest that for continuous outcomes, 20.03% of the data used in meta-analyses cannot be reproduced at the trial level, and 43.44% of the data cannot be reproduced at the meta-analysis level. For binary outcomes, the proportions were 14.14% and 40.74%. In total, 83.33% of the data cannot be reproduced at the systematic review level. Our further analysis suggested that these reproducibility issues would lead to as much as 6.52% of the available meta-analyses changing the direction of the effects, and 9.78% changing the significance of the P-values. Sleep medicine systematic reviews and meta-analyses face serious issues in terms of data reproducibility, and further efforts are urgently needed to improve this situation.
- Xu, C., Furuya-Kanamori, L., & Lin, L. (2022). Synthesis of evidence from zero-events studies: A comparison of one-stage framework methods. Research synthesis methods, 13(2), 176-189.More infoIn evidence synthesis, dealing with zero-events studies is an important and complicated task that has generated broad discussion. Numerous methods provide valid solutions to synthesizing data from studies with zero-events, either based on a frequentist or a Bayesian framework. Among frequentist frameworks, the one-stage methods have their unique advantages to deal with zero-events studies, especially for double-arm-zero-events. In this article, we give a concise overview of the one-stage frequentist methods. We conducted simulation studies to compare the statistical properties of these methods to the two-stage frequentist method (continuity correction) for meta-analysis with zero-events studies when double-zero-events studies were included. Our simulation studies demonstrated that the generalized estimating equation with unstructured correlation and beta-binomial method had the best performance among the one-stage methods. The random intercepts generalized linear mixed model showed good performance in the absence of obvious between-study variance. Our results also showed that the continuity correction with inverse-variance heterogeneous (IVhet) analytic model based on the two-stage framework had good performance when the between-study variance was obvious and the group size was balanced for included studies. In summary, the one-stage framework has unique advantages to deal with studies with zero events and is not susceptive to group size ratio. It should be considered in future meta-analyses whenever possible.
- Xu, C., Ju, K., Lin, L., Jia, P., Kwong, J. S., Syed, A., & Furuya-Kanamori, L. (2022). Rapid evidence synthesis approach for limits on the search date: How rapid could it be?. Research synthesis methods, 13(1), 68-76.More infoRapid reviews have been widely employed to support timely decision-making, and limiting the search date is the most popular approach in published rapid reviews. We assessed the accuracy and workload of search date limits on the meta-analytical results to determine the best rapid strategy. The meta-analyses data were collected from the Cochrane Database of Systematic Reviews (CDSR). We emulated the rapid reviews by limiting the search date of the original CDSR to the recent 40, 35, 30, 25, 20, 15, 10, 7, 5, and 3 years, and their results were compared to the full meta-analyses. A random sample of 10% was drawn to repeat the literature search by the same timeframe limits to measure the relative workload reduction (RWR). The relationship between accuracy and RWR was established. We identified 21,363 meta-analyses of binary outcomes and 7683 meta-analyses of continuous outcomes from 2693 CDSRs. Our results suggested that under a maximum tolerance of 5% and 10% on the bias of magnitude, a limit on the recent 20 years can achieve good accuracy and at the same time save the most workload. Under the tolerance of 15% and 20% on the bias, a limit on the recent 10 years and 15 years could be considered. Limiting the search date is a valid rapid method to produce credible evidence for timely decisions. When conducting rapid reviews, researchers should consider both the accuracy and workload to make an appropriate decision.
- Xu, C., Lin, L., & Vohra, S. (2022). Evidence synthesis practice: why we cannot ignore studies with no events?. Journal of general internal medicine, 37(14), 3744-3745.
- Xu, C., Yu, T., Furuya-Kanamori, L., Lin, L., Zorzela, L., Zhou, X., Dai, H., Loke, Y., & Vohra, S. (2022). Validity of data extraction in evidence synthesis practice of adverse events: reproducibility study. BMJ (Clinical research ed.), 377, e069155.More infoTo investigate the validity of data extraction in systematic reviews of adverse events, the effect of data extraction errors on the results, and to develop a classification framework for data extraction errors to support further methodological research.
- Yu, T., Lin, L., Furuya-Kanamori, L., & Xu, C. (2022). Synthesizing evidence from the earliest studies to support decision-making: To what extent could the evidence be reliable?. Research synthesis methods, 13(5), 632-644.More infoIn evidence-based practice, new topics generally only have a few studies available for synthesis. As a result, the evidence of such meta-analyses raised substantial concerns. We investigated the robustness of the evidence from these earliest studies. Real-world data from the Cochrane Database of Systematic Reviews (CDSR) were collected. We emulated meta-analyses with the earliest 1 to 10 studies through cumulative meta-analysis from eligible meta-analyses. The magnitude and the direction of meta-analyses with the earliest few studies were compared to the full meta-analyses. From the CDSR, we identified 20,227 meta-analyses of binary outcomes and 7683 meta-analyses of continuous outcomes. Under the tolerable difference of 20% on the magnitude of the effects, the convergence proportion ranged from 24.24% (earliest 1 study) to 77.45% (earliest 10 studies) for meta-analyses of few earliest studies with binary outcomes. For meta-analyses of continuous outcomes, the convergence proportion ranged from 13.86% to 56.52%. In terms of the direction of the effects, even when only three studies were available at the earliest stage, the majority had the same direction as full meta-analyses; Only 19% for binary outcomes and 12% for continuous outcomes changed the direction as further evidence accumulated. Synthesizing evidence from the earliest studies is feasible to support urgent decision-making, and in most cases, the decisions would be reasonable. Considering the potential uncertainties, it is essential to evaluate the confidence of the evidence of these meta-analyses and update the evidence when necessary.
- Zhao, Y., Slate, E. H., Xu, C., Chu, H., & Lin, L. (2022). Empirical comparisons of heterogeneity magnitudes of the risk difference, relative risk, and odds ratio. Systematic reviews, 11(1), 26.
- Zhou, T., Zhou, J., Hodges, J. S., Lin, L., Chen, Y., Cole, S. R., & Chu, H. (2022). Estimating the Complier Average Causal Effect in a Meta-Analysis of Randomized Clinical Trials With Binary Outcomes Accounting for Noncompliance: A Generalized Linear Latent and Mixed Model Approach. American journal of epidemiology, 191(1), 220-229.More infoNoncompliance, a common problem in randomized clinical trials (RCTs), can bias estimation of the effect of treatment receipt using a standard intention-to-treat analysis. The complier average causal effect (CACE) measures the effect of an intervention in the latent subpopulation that would comply with their assigned treatment. Although several methods have been developed to estimate the CACE in analyzing a single RCT, methods for estimating the CACE in a meta-analysis of RCTs with noncompliance await further development. This article reviews the assumptions needed to estimate the CACE in a single RCT and proposes a frequentist alternative for estimating the CACE in a meta-analysis, using a generalized linear latent and mixed model with SAS software (SAS Institute, Inc.). The method accounts for between-study heterogeneity using random effects. We implement the methods and describe an illustrative example of a meta-analysis of 10 RCTs evaluating the effect of receiving epidural analgesia in labor on cesarean delivery, where noncompliance varies dramatically between studies. Simulation studies are used to evaluate the performance of the proposed method.
- Al Amer, F. M., & Lin, L. (2021). Empirical assessment of prediction intervals in Cochrane meta-analyses. European journal of clinical investigation, 51(7), e13524.
- Al Amer, F. M., Thompson, C. G., & Lin, L. (2021). Bayesian Methods for Meta-Analyses of Binary Outcomes: Implementations, Examples, and Impact of Priors. International journal of environmental research and public health, 18(7).More infoBayesian methods are an important set of tools for performing meta-analyses. They avoid some potentially unrealistic assumptions that are required by conventional frequentist methods. More importantly, meta-analysts can incorporate prior information from many sources, including experts' opinions and prior meta-analyses. Nevertheless, Bayesian methods are used less frequently than conventional frequentist methods, primarily because of the need for nontrivial statistical coding, while frequentist approaches can be implemented via many user-friendly software packages. This article aims at providing a practical review of implementations for Bayesian meta-analyses with various prior distributions. We present Bayesian methods for meta-analyses with the focus on odds ratio for binary outcomes. We summarize various commonly used prior distribution choices for the between-studies heterogeneity variance, a critical parameter in meta-analyses. They include the inverse-gamma, uniform, and half-normal distributions, as well as evidence-based informative log-normal priors. Five real-world examples are presented to illustrate their performance. We provide all of the statistical code for future use by practitioners. Under certain circumstances, Bayesian methods can produce markedly different results from those by frequentist methods, including a change in decision on statistical significance. When data information is limited, the choice of priors may have a large impact on meta-analytic results, in which case sensitivity analyses are recommended. Moreover, the algorithm for implementing Bayesian analyses may not converge for extremely sparse data; caution is needed in interpreting respective results. As such, convergence should be routinely examined. When select statistical assumptions that are made by conventional frequentist methods are violated, Bayesian methods provide a reliable alternative to perform a meta-analysis.
- Atieh, M. A., Baqain, Z. H., Tawse-Smith, A., Ma, S., Almoselli, M., Lin, L., & Alsabeeha, N. H. (2021). The influence of insertion torque values on the failure and complication rates of dental implants: A systematic review and meta-analysis. Clinical implant dentistry and related research, 23(3), 341-360.More infoThe influence of using different insertion torque values on clinical and radiographic outcomes of implant therapy is unclear in the current literature. The aim of this systematic review and meta-analysis was to evaluate the implant outcomes and complications rates using high insertion torque values compared with those using regular insertion torque value levels.
- Jia, P., Lin, L., Kwong, J. S., & Xu, C. (2021). Many meta-analyses of rare events in the Cochrane Database of Systematic Reviews were underpowered. Journal of clinical epidemiology, 131, 113-122.More infoMeta-analysis is a statistical method with the ability to increase the power for statistical inference, while it may still face the problem of being underpowered. In this study, we investigated the power to detect certain true effects for published meta-analyses of rare events.
- Lin, L. (2021). Evidence inconsistency degrees of freedom in Bayesian network meta-analysis. Journal of biopharmaceutical statistics, 31(3), 317-330.More infoNetwork meta-analysis (NMA) is a popular tool to synthesize direct and indirect evidence for simultaneously comparing multiple treatments, while evidence inconsistency greatly threatens its validity. One may use the inconsistency degrees of freedom (ICDF) to assess the potential that an NMA might suffer from inconsistency. Multi-arm studies provide intrinsically consistent evidence and complicate the ICDF's calculation; they commonly appear in NMAs. The existing ICDF measure may not feasibly handle multi-arm studies. Motivated from the effective numbers of parameters of Bayesian hierarchical models, we propose new ICDF measures in generic NMAs that may contain multi-arm studies. Under the fixed- or random-effects setting, the new ICDF measure is the difference between the effective numbers of parameters of the consistency and inconsistency NMA models. We used artificial NMAs created based on an illustrative example and 39 empirical NMAs to evaluate the performance of the existing and new measures. In NMAs with two-arm studies only, the proposed ICDF measure under the fixed-effects setting was nearly the same with the existing measure. Among the empirical NMAs, 27 (69%) contained at least one multi-arm study. The existing measure was not applicable to them, while the proposed measures led to interpretable ICDFs in all NMAs.
- Lin, L. (2021). Factors that impact fragility index and their visualizations. Journal of evaluation in clinical practice, 27(2), 356-364.More infoAs the recent literature has growing concerns about research replicability and the misuse and misconception of P-values, the fragility index (FI) has been an attractive measure to assess the robustness (or fragility) of clinical study results with binary outcomes. It is defined as the minimum number of event status modifications that can alter a study result's statistical significance (or non-significance). Owing to its intuitive concept, the FI has been applied to assess the fragility of clinical studies of various specialties. However, the FI may be limited in certain settings. As a relatively new measure, more work is needed to examine its properties.
- Lin, L., & Aloe, A. M. (2021). Evaluation of various estimators for standardized mean difference in meta-analysis. Statistics in medicine, 40(2), 403-426.More infoMeta-analyses of a treatment's effect compared with a control frequently calculate the meta-effect from standardized mean differences (SMDs). SMDs are usually estimated by Cohen's d or Hedges' g. Cohen's d divides the difference between sample means of a continuous response by the pooled standard deviation, but is subject to nonnegligible bias for small sample sizes. Hedges' g removes this bias with a correction factor. The current literature (including meta-analysis books and software packages) is confusingly inconsistent about methods for synthesizing SMDs, potentially making reproducibility a problem. Using conventional methods, the variance estimate of SMD is associated with the point estimate of SMD, so Hedges' g is not guaranteed to be unbiased in meta-analyses. This article comprehensively reviews and evaluates available methods for synthesizing SMDs. Their performance is compared using extensive simulation studies and analyses of actual datasets. We find that because of the intrinsic association between point estimates and standard errors, the usual version of Hedges' g can result in more biased meta-estimation than Cohen's d. We recommend using average-adjusted variance estimators to obtain an unbiased meta-estimate, and the Hartung-Knapp-Sidik-Jonkman method for accurate estimation of its confidence interval.
- Rosenberger, K. J., Duan, R., Chen, Y., & Lin, L. (2021). Predictive P-score for treatment ranking in Bayesian network meta-analysis. BMC medical research methodology, 21(1), 213.More infoNetwork meta-analysis (NMA) is a widely used tool to compare multiple treatments by synthesizing different sources of evidence. Measures such as the surface under the cumulative ranking curve (SUCRA) and the P-score are increasingly used to quantify treatment ranking. They provide summary scores of treatments among the existing studies in an NMA. Clinicians are frequently interested in applying such evidence from the NMA to decision-making in the future. This prediction process needs to account for the heterogeneity between the existing studies in the NMA and a future study.
- Rosenberger, K. J., Xing, A., Murad, M. H., Chu, H., & Lin, L. (2021). Prior Choices of Between-Study Heterogeneity in Contemporary Bayesian Network Meta-analyses: an Empirical Study. Journal of general internal medicine, 36(4), 1049-1057.More infoNetwork meta-analysis (NMA) is a popular tool to compare multiple treatments in medical research. It is frequently implemented via Bayesian methods. The prior choice of between-study heterogeneity is critical in Bayesian NMAs. This study evaluates the impact of different priors for heterogeneity on NMA results.
- Rosenberger, K. J., Xu, C., & Lin, L. (2021). Methodological assessment of systematic reviews and meta-analyses on COVID-19: A meta-epidemiological study. Journal of evaluation in clinical practice, 27(5), 1123-1133.More infoCOVID-19 has caused an ongoing public health crisis. Many systematic reviews and meta-analyses have been performed to synthesize evidence for better understanding this new disease. However, some concerns have been raised about rapid COVID-19 research. This meta-epidemiological study aims to methodologically assess the current systematic reviews and meta-analyses on COVID-19.
- Rott, K. W., Lin, L., Hodges, J. S., Siegel, L., Shi, A., Chen, Y., & Chu, H. (2021). Bayesian meta-analysis using SAS PROC BGLIMM. Research synthesis methods, 12(6), 692-700.More infoMeta-analysis is commonly used to compare two treatments. Network meta-analysis (NMA) is a powerful extension for comparing and contrasting multiple treatments simultaneously in a systematic review of multiple clinical trials. Although the practical utility of meta-analysis is apparent, it is not always straightforward to implement, especially for those interested in a Bayesian approach. This paper demonstrates that the recently-developed SAS procedure BGLIMM provides an intuitive and computationally efficient means for conducting Bayesian meta-analysis in SAS, using a worked example of a smoking cessation NMA data set. BGLIMM gives practitioners an effective and simple way to implement Bayesian meta-analysis (pairwise and network, either contrast-based or arm-based) without requiring significant background in coding or statistical modeling. Those familiar with generalized linear mixed models, and especially the SAS procedure GLIMMIX, will find this tutorial a useful introduction to Bayesian meta-analysis in SAS.
- Wang, Z., Lin, L., Hodges, J. S., MacLehose, R., & Chu, H. (2021). A variance shrinkage method improves arm-based Bayesian network meta-analysis. Statistical methods in medical research, 30(1), 151-165.More infoNetwork meta-analysis is a commonly used tool to combine direct and indirect evidence in systematic reviews of multiple treatments to improve estimation compared to traditional pairwise meta-analysis. Unlike the contrast-based network meta-analysis approach, which focuses on estimating relative effects such as odds ratios, the arm-based network meta-analysis approach can estimate absolute risks and other effects, which are arguably more informative in medicine and public health. However, the number of clinical studies involving each treatment is often small in a network meta-analysis, leading to unstable treatment-specific variance estimates in the arm-based network meta-analysis approach when using non- or weakly informative priors under an unequal variance assumption. Additional assumptions, such as equal (i.e. homogeneous) variances for all treatments, may be used to remedy this problem, but such assumptions may be inappropriately strong. This article introduces a variance shrinkage method for an arm-based network meta-analysis. Specifically, we assume different treatment variances share a common prior with unknown hyperparameters. This assumption is weaker than the homogeneous variance assumption and improves estimation by shrinking the variances in a data-dependent way. We illustrate the advantages of the variance shrinkage method by reanalyzing a network meta-analysis of organized inpatient care interventions for stroke. Finally, comprehensive simulations investigate the impact of different variance assumptions on statistical inference, and simulation results show that the variance shrinkage method provides better estimation for log odds ratios and absolute risks.
- Wang, Z., Lin, L., Murray, T., Hodges, J. S., & Chu, H. (2021). BRIDGING RANDOMIZED CONTROLLED TRIALS AND SINGLE-ARM TRIALS USING COMMENSURATE PRIORS IN ARM-BASED NETWORK META-ANALYSIS. The annals of applied statistics, 15(4), 1767-1787.More infoNetwork meta-analysis (NMA) is a powerful tool to compare multiple treatments directly and indirectly by combining and contrasting multiple independent clinical trials. Because many NMAs collect only a few eligible randomized controlled trials (RCTs), there is an urgent need to synthesize different sources of information, e.g., from both RCTs and single-arm trials. However, single-arm trials and RCTs may have different populations and quality, so that assuming they are exchangeable may be inappropriate. This article presents a novel method using a (CPV) to borrow variance (rather than mean) information from single-arm trials in an arm-based (AB) Bayesian NMA. We illustrate the advantages of this CPV method by reanalyzing an NMA of immune checkpoint inhibitors in cancer patients. Comprehensive simulations investigate the impact on statistical inference of including single-arm trials. The simulation results show that the CPV method provides efficient and robust estimation even when the two sources of information are moderately inconsistent.
- Wu, C., Wu, L., Wang, J., Lin, L., Li, Y., Lu, Q., & Deng, H. W. (2021). Systematic identification of risk factors and drug repurposing options for Alzheimer's disease. Alzheimer's & dementia (New York, N. Y.), 7(1), e12148.More infoSeveral Mendelian randomization studies have been conducted that identified multiple risk factors for Alzheimer's disease (AD). However, they typically focus on a few pre-selected risk factors.
- Xiao, M., Lin, L., Hodges, J. S., Xu, C., & Chu, H. (2021). Double-zero-event studies matter: A re-evaluation of physical distancing, face masks, and eye protection for preventing person-to-person transmission of COVID-19 and its policy impact. Journal of clinical epidemiology, 133, 158-160.
- Xu, C., Furuya-Kanamori, L., Zorzela, L., Lin, L., & Vohra, S. (2021). A proposed framework to guide evidence synthesis practice for meta-analysis with zero-events studies. Journal of clinical epidemiology, 135, 70-78.More infoIn evidence synthesis practice, researchers often face the problem of how to deal with zero-events. Inappropriately dealing with zero-events studies may lead to research waste and mislead healthcare practice. We propose a framework to guide researchers to better deal with zero-events in meta-analysis.
- Xu, C., Zhou, X., Zorzela, L., Ju, K., Furuya-Kanamori, L., Lin, L., Lu, C., Musa, O. A., & Vohra, S. (2021). Utilization of the evidence from studies with no events in meta-analyses of adverse events: an empirical investigation. BMC medicine, 19(1), 141.More infoZero-events studies frequently occur in systematic reviews of adverse events, which consist of an important source of evidence. We aimed to examine how evidence of zero-events studies was utilized in the meta-analyses of systematic reviews of adverse events.
- Yang, J., Lin, L., & Chu, H. (2021). BayesSenMC: an R package for Bayesian Sensitivity Analysis of Misclassification. The R Journal, 13(2), 228-238. doi:10.32614/RJ-2021-097
- Zhao, Y., & Lin, L. (2021). Good Statistical Practices for Contemporary Meta-Analysis: Examples Based on a Systematic Review on COVID-19 in Pregnancy. BioMedInformatics, 1(2), 64-76. doi:10.3390/biomedinformatics1020005
- Zhou, X., Li, L., Lin, L., Ju, K., Kwong, J. S., & Xu, C. (2021). Methodological quality for systematic reviews of adverse events with surgical interventions: a cross-sectional survey. BMC medical research methodology, 21(1), 223.More infoAn increasing number of systematic reviews assessed the safety of surgical interventions over time. How well these systematic reviews were designed and conducted determines the reliability of evidence. In this study, we aimed to assess the methodological quality of systematic reviews on the safety of surgical interventions.
- Furuya-Kanamori, L., Xu, C., Lin, L., Doan, T., Chu, H., Thalib, L., & Doi, S. A. (2020). P value-driven methods were underpowered to detect publication bias: analysis of Cochrane review meta-analyses. Journal of clinical epidemiology, 118, 86-92.More infoThe aim of the study was to investigate the effect of number of studies in a meta-analysis on the detection of publication bias using P value-driven methods.
- Ju, K., Lin, L., Chu, H., Cheng, L. L., & Xu, C. (2020). Laplace approximation, penalized quasi-likelihood, and adaptive Gauss-Hermite quadrature for generalized linear mixed models: towards meta-analysis of binary outcome with sparse data. BMC medical research methodology, 20(1), 152.More infoIn meta-analyses of a binary outcome, double zero events in some studies cause a critical methodology problem. The generalized linear mixed model (GLMM) has been proposed as a valid statistical tool for pooling such data. Three parameter estimation methods, including the Laplace approximation (LA), penalized quasi-likelihood (PQL) and adaptive Gauss-Hermite quadrature (AGHQ) were frequently used in the GLMM. However, the performance of GLMM via these estimation methods is unclear in meta-analysis with zero events.
- Lin, L. (2020). Comparison of four heterogeneity measures for meta-analysis. Journal of evaluation in clinical practice, 26(1), 376-384.More infoHeterogeneity is a critical issue in meta-analysis, because it implies the appropriateness of combining the collected studies and impacts the reliability of the synthesized results. The Q test is a traditional method to assess heterogeneity; however, because it does not have an intuitive interpretation for clinicians and often has low statistical power, many meta-analysts alter to use some measures, such as the I statistic, to quantify the extent of heterogeneity. This article aims at providing a summary of available tools to assess heterogeneity and comparing their performance.
- Lin, L. (2020). Hybrid test for publication bias in meta-analysis. Statistical methods in medical research, 29(10), 2881-2899.More infoPublication bias frequently appears in meta-analyses when the included studies' results (e.g., -values) influence the studies' publication processes. Some unfavorable studies may be suppressed from publication, so the meta-analytic results may be biased toward an artificially favorable direction. Many statistical tests have been proposed to detect publication bias in recent two decades. However, they often make dramatically different assumptions about the cause of publication bias; therefore, they are usually powerful only in certain cases that support their particular assumptions, while their powers may be fairly low in many other cases. Although several simulation studies have been carried out to compare different tests' powers under various situations, it is typically infeasible to justify the exact mechanism of publication bias in a real-world meta-analysis and thus select the corresponding optimal publication bias test. We introduce a hybrid test for publication bias by synthesizing various tests and incorporating their benefits, so that it maintains relatively high powers across various mechanisms of publication bias. The superior performance of the proposed hybrid test is illustrated using simulation studies and three real-world meta-analyses with different effect sizes. It is compared with many existing methods, including the commonly used regression and rank tests, and the trim-and-fill method.
- Lin, L., & Chu, H. (2020). Meta-analysis of Proportions Using Generalized Linear Mixed Models. Epidemiology (Cambridge, Mass.), 31(5), 713-717.More infoEpidemiologic research often involves meta-analyses of proportions. Conventional two-step methods first transform each study's proportion and subsequently perform a meta-analysis on the transformed scale. They suffer from several important limitations: the log and logit transformations impractically treat within-study variances as fixed, known values and require ad hoc corrections for zero counts; the results from arcsine-based transformations may lack interpretability. Generalized linear mixed models (GLMMs) have been recommended in meta-analyses as a one-step approach to fully accounting for within-study uncertainties. However, they are seldom used in current practice to synthesize proportions. This article summarizes various methods for meta-analyses of proportions, illustrates their implementations, and explores their performance using real and simulated datasets. In general, GLMMs led to smaller biases and mean squared errors and higher coverage probabilities than two-step methods. Many software programs are readily available to implement these methods.
- Lin, L., & Xu, C. (2020). Arcsine-based transformations for meta-analysis of proportions: Pros, cons, and alternatives. Health science reports, 3(3), e178.More infoMeta-analyses have been increasingly used to synthesize proportions (eg, disease prevalence) from multiple studies in recent years. Arcsine-based transformations, especially the Freeman-Tukey double-arcsine transformation, are popular tools for stabilizing the variance of each study's proportion in two-step meta-analysis methods. Although they offer some benefits over the conventional logit transformation, they also suffer from several important limitations (eg, lack of interpretability) and may lead to misleading conclusions. Generalized linear mixed models and Bayesian models are intuitive one-step alternative approaches, and can be readily implemented via many software programs. This article explains various pros and cons of the arcsine-based transformations, and discusses the alternatives that may be generally superior to the currently popular practice.
- Lin, L., Chu, H., & Hodges, J. S. (2020). On evidence cycles in network meta-analysis. Statistics and its interface, 13(4), 425-436.More infoAs an extension of pairwise meta-analysis of two treatments, network meta-analysis has recently attracted many researchers in evidence-based medicine because it simultaneously synthesizes both direct and indirect evidence from multiple treatments and thus facilitates better decision making. The Bayesian hierarchical model is a popular method to implement network meta-analysis, and it is generally considered more powerful than conventional pairwise meta-analysis, leading to more precise effect estimates with narrower credible intervals. However, the improvement of effect estimates produced by Bayesian network meta-analysis has never been studied theoretically. This article shows that such improvement depends highly on evidence cycles in the treatment network. When all treatment comparisons are assumed to have different heterogeneity variances, a network meta-analysis produces posterior distributions identical to separate pairwise meta-analyses for treatment comparisons that are not contained in any evidence cycles. However, this equivalence does not hold under the commonly-used assumption of a common heterogeneity variance for all comparisons. Simulations and a case study are used to illustrate the equivalence of the Bayesian network and pairwise meta-analyses in certain networks.
- Lin, L., Shi, L., Chu, H., & Murad, M. H. (2020). The magnitude of small-study effects in the : an empirical study of nearly 30 000 meta-analyses. BMJ evidence-based medicine, 25(1), 27-32.More infoPublication bias, more generally termed as small-study effect, is a major threat to the validity of meta-analyses. Most meta-analysts rely on the p values from statistical tests to make a binary decision about the presence or absence of small-study effects. Measures are available to quantify small-study effects' magnitude, but the current literature lacks clear rules to help evidence users in judging whether such effects are minimal or substantial. This article aims to provide rules of thumb for interpreting the measures. We use six measures to evaluate small-study effects in 29 932 meta-analyses from the They include Egger's regression intercept and the skewness under both the fixed-effect and random-effects settings, the proportion of suppressed studies, and the relative change of the estimated overall result due to small-study effects. The cut-offs for different extents of small-study effects are determined based on the quantiles in these distributions. We present the empirical distributions of the six measures and propose a rough guide to interpret the measures' magnitude. The proposed rules of thumb may help evidence users grade the certainty in evidence as impacted by small-study effects.
- Shao, Y., MacLehose, R. F., Lin, L., Hwang, J., Alexander, B. H., Mandel, J. H., & Ramachandran, G. (2020). A Bayesian Approach for Determining the Relationship Between Various Elongate Mineral Particles (EMPs) Definitions. Annals of work exposures and health, 64(9), 993-1006.More infoA variety of dimensions (lengths and widths) of elongate mineral particles (EMPs) have been proposed as being related to health effects. In this paper, we develop a mathematical approach for deriving numerical conversion factors (CFs) between these EMP exposure metrics and applied it to the Minnesota Taconite Health Worker study which contains 196 different job exposure groups (28 similar exposure groups times 7 taconite mines). This approach comprises four steps: for each group (i) obtain EMP dimension information using ISO-TEM 10312/13794 analysis; (ii) use bivariate lognormal distribution to characterize overall EMP size distribution; (iii) use a Bayesian approach to facilitate the formation of the bivariate lognormal distribution; (iv) derive conversion factors between any pair of EMP definitions. The final CFs allow the creation of job exposure matrices (JEMs) for alternative EMP metrics using existing EMP exposures already characterized according to the National Institute of Occupational Safety and Health (NIOSH)-defined EMP exposure metric (length >5 µm with an aspect ratio ≥3.0). The relationships between the NIOSH EMP and other EMP definitions provide the basis of classification of workers into JEMs based on alternate definitions of EMP for epidemiological studies of mesothelioma, lung cancer, and non-malignant respiratory disease.
- Shi, L., Chu, H., & Lin, L. (2020). A Bayesian approach to assessing small-study effects in meta-analysis of a binary outcome with controlled false positive rate. Research synthesis methods, 11(4), 535-552.More infoPublication bias threatens meta-analysis validity. It is often assessed via the funnel plot; an asymmetric plot implies small-study effects, and publication bias is one cause of the asymmetry. Egger's regression test is a widely used tool to quantitatively assess such asymmetry. It examines the association between the observed effect sizes and their sample SEs; a strong association indicates small-study effects. However, its false positive rates may be inflated if such an association intrinsically exists even if no small-study effects appear, particularly in meta-analyses of odds ratios (ORs). Various alternatives are available to address this problem. They usually replace Egger's regression predictor or response with different measures; consequently, they are powerful only in specific cases. We propose a Bayesian approach to assessing small-study effects in meta-analyses of ORs. It controls false positive rates by using latent "true" SEs, rather than sample SEs, in the Egger-type regression to avoid the intrinsic association between ORs and their SEs. Although "true" SEs are unknown in practice, they can be modeled under the Bayesian framework. We use simulated and real data to compare various methods. When ORs are away from 1, the proposed method may have high powers with controlled false positive rates, while Egger's test has seriously inflated false positive rates; nevertheless, in other situations, some other methods may be superior. In general, the proposed method may serve as an alternative to rule out potential confounding effects caused by the intrinsic association between ORs and their SEs in the assessment of small-study effects.
- Wang, Z., Lin, L., Hodges, J. S., & Chu, H. (2020). The impact of covariance priors on arm-based Bayesian network meta-analyses with binary outcomes. Statistics in medicine, 39(22), 2883-2900.More infoBayesian analyses with the arm-based (AB) network meta-analysis (NMA) model require researchers to specify a prior distribution for the covariance matrix of the treatment-specific event rates in a transformed scale, for example, the treatment-specific log-odds when a logit transformation is used. The commonly used conjugate prior for the covariance matrix, the inverse-Wishart (IW) distribution, has several limitations. For example, although the IW distribution is often described as noninformative or weakly informative, it may in fact provide strong information when some variance components are small (eg, when the standard deviation of study-specific log-odds of a treatment is smaller than 1/2), as is common in NMAs with binary outcomes. In addition, the IW prior generally leads to underestimation of correlations between treatment-specific log-odds, which are critical for borrowing strength across treatment arms to estimate treatment effects efficiently and to reduce potential bias. Alternatively, several separation strategies (ie, separate priors on variances and correlations) can be considered. To study the IW prior's impact on NMA results and compare it with separation strategies, we did simulation studies under different missing-treatment mechanisms. A separation strategy with appropriate priors for the correlation matrix and variances performs better than the IW prior, and should be recommended as the default vague prior in the AB NMA approach. Finally, we reanalyzed three case studies and illustrated the importance, when performing AB-NMA, of sensitivity analyses with different prior specifications on variances.
- Xing, A., & Lin, L. (2020). Effects of treatment classifications in network meta-analysis. Research Methods in Medicine & Health Sciences, 1(1), 12-24. doi:10.1177/2632084320932756
- Xing, A., Chu, H., & Lin, L. (2020). Fragility index of network meta-analysis with application to smoking cessation data. Journal of clinical epidemiology, 127, 29-39.More infoThe network meta-analysis (NMA) is frequently used to synthesize evidence for multiple treatment comparisons, but its complexity may affect the robustness (or fragility) of the results. The fragility index (FI) is recently proposed to assess the fragility of the results from clinical studies and from pairwise meta-analyses. We extend the FI to NMAs with binary outcomes.
- Xu, C., Li, L., Lin, L., Chu, H., Thabane, L., Zou, K., & Sun, X. (2020). Exclusion of studies with no events in both arms in meta-analysis impacted the conclusions. Journal of clinical epidemiology, 123, 91-99.More infoClassical meta-analyses routinely treated studies with no events in both arms noninformative and excluded them from analyses. This study assessed whether such studies contain information and have an influence on the conclusions of meta-analyses.
- Zhou, Y., Zhu, B., Lin, L., Kwong, J. S., & Xu, C. (2020). Protocols for meta-analysis of intervention safety seldom specified methods to deal with rare events. Journal of clinical epidemiology, 128, 109-117.More infoMeta-analyses of rare events often generate unstable results, and selective reporting of the results may mislead the health care decision. Developing a synthesis plan for rare events in protocol may help to formulate the reporting. We aim to investigate whether existing protocols specified methods to deal with rare events.
- Lin, L. (2019). Graphical augmentations to sample-size-based funnel plot in meta-analysis. Research synthesis methods, 10(3), 376-388.More infoAssessing publication bias is a critical procedure in meta-analyses for rating the synthesized overall evidence. Because statistical tests for publication bias are usually not powerful and only give P values that inform either the presence or absence of the bias, examining the asymmetry of funnel plots has been popular to investigate potentially missing studies and the direction of the bias. Most funnel plots present treatment effects against their standard errors, and the contours depicting studies' significance levels have been used in the plots to distinguish publication bias from other factors (such as heterogeneity and subgroup effects) that may cause the plots' asymmetry. However, treatment effects and their standard errors are frequently associated even if no publication bias exists (eg, both variables depend on the four data cells in a 2 × 2 table for the odds ratio), so standard-error-based funnel plots may lead to false positive conclusions when such association may not be negligible. In addition, the missingness of studies may relate to their sample sizes besides P values (which are partly determined by standard errors); studies with more samples are more likely published. Therefore, funnel plots based on sample sizes can be an alternative tool. However, the contours for standard-error-based funnel plots cannot be directly applied to sample-size-based ones. This article introduces contours for sample-size-based funnel plots of various effect sizes, which may help meta-analysts properly interpret such plots' asymmetry. We provide five examples to illustrate the use of the proposed contours.
- Lin, L. (2019). Use of Prediction Intervals in Network Meta-analysis. JAMA network open, 2(8), e199735.More infoThis cross-sectional study examines the prevalence of reporting prediction intervals in network meta-analysis articles and provides a worked example.
- Lin, L., Xing, A., Kofler, M. J., & Murad, M. H. (2019). Borrowing of strength from indirect evidence in 40 network meta-analyses. Journal of clinical epidemiology, 106, 41-49.More infoNetwork meta-analysis (NMA) is increasingly being used to synthesize direct and indirect evidence and help decision makers simultaneously compare multiple treatments. We empirically evaluate the incremental gain in precision achieved by incorporating indirect evidence in NMAs.
- Murad, M. H., Wang, Z., Chu, H., & Lin, L. (2019). When continuous outcomes are measured using different scales: guide for meta-analysis and interpretation. BMJ (Clinical research ed.), 364, k4817.More infoIt is common to measure continuous outcomes using different scales (eg, quality of life, severity of anxiety or depression), therefore these outcomes need to be standardized before pooling in a meta-analysis. Common methods of standardization include using the standardized mean difference, the odds ratio derived from continuous data, the minimally important difference, and the ratio of means. Other ways of making data more meaningful to end users include transforming standardized effects back to original scales and transforming odds ratios to absolute effects using an assumed baseline risk. For these methods to be valid, the scales or instruments being combined across studies need to have assessed the same or a similar construct
- Ren, Y., Lin, L., Lian, Q., Zou, H., & Chu, H. (2019). Real-world Performance of Meta-analysis Methods for Double-Zero-Event Studies with Dichotomous Outcomes Using the Cochrane Database of Systematic Reviews. Journal of general internal medicine, 34(6), 960-968.More infoMeta-analysis combines multiple independent studies, which can increase power and provide better estimates. However, it is unclear how best to deal with studies with zero events; such studies are also known as double-zero-event studies (DZS). Several statistical methods have been proposed, but the agreement among different approaches has not been systematically assessed using real-world published systematic reviews.
- Shi, L., & Lin, L. (2019). The trim-and-fill method for publication bias: practical guidelines and recommendations based on a large database of meta-analyses. Medicine, 98(23), e15987.More infoPublication bias is a type of systematic error when synthesizing evidence that cannot represent the underlying truth. Clinical studies with favorable results are more likely published and thus exaggerate the synthesized evidence in meta-analyses. The trim-and-fill method is a popular tool to detect and adjust for publication bias. Simulation studies have been performed to assess this method, but they may not fully represent realistic settings about publication bias. Based on real-world meta-analyses, this article provides practical guidelines and recommendations for using the trim-and-fill method. We used a worked illustrative example to demonstrate the idea of the trim-and-fill method, and we reviewed three estimators (R0, L0, and Q0) for imputing missing studies. A resampling method was proposed to calculate P values for all 3 estimators. We also summarized available meta-analysis software programs for implementing the trim-and-fill method. Moreover, we applied the method to 29,932 meta-analyses from the Cochrane Database of Systematic Reviews, and empirically evaluated its overall performance. We carefully explored potential issues occurred in our analysis. The estimators L0 and Q0 detected at least one missing study in more meta-analyses than R0, while Q0 often imputed more missing studies than L0. After adding imputed missing studies, the significance of heterogeneity and overall effect sizes changed in many meta-analyses. All estimators generally converged fast. However, L0 and Q0 failed to converge in a few meta-analyses that contained studies with identical effect sizes. Also, P values produced by different estimators could yield different conclusions of publication bias significance. Outliers and the pre-specified direction of missing studies could have influential impact on the trim-and-fill results. Meta-analysts are recommended to perform the trim-and-fill method with great caution when using meta-analysis software programs. Some default settings (e.g., the choice of estimators and the direction of missing studies) in the programs may not be optimal for a certain meta-analysis; they should be determined on a case-by-case basis. Sensitivity analyses are encouraged to examine effects of different estimators and outlying studies. Also, the trim-and-fill estimator should be routinely reported in meta-analyses, because the results depend highly on it.
- Kalyanam, K., McAteer, J., Marek, J., Hodges, J., & Lin, L. (2018). Cross channel effects of search engine advertising on brick & mortar retail sales: Meta analysis of large scale field experiments on Google.com. Quantitative Marketing and Economics, 16, 1-42. doi:10.1007/s11129-017-9188-7
- Lin, L. (2018). Bias caused by sampling error in meta-analysis with small sample sizes. PloS one, 13(9), e0204056.More infoMeta-analyses frequently include studies with small sample sizes. Researchers usually fail to account for sampling error in the reported within-study variances; they model the observed study-specific effect sizes with the within-study variances and treat these sample variances as if they were the true variances. However, this sampling error may be influential when sample sizes are small. This article illustrates that the sampling error may lead to substantial bias in meta-analysis results.
- Lin, L. (2018). Quantifying and presenting overall evidence in network meta-analysis. Statistics in medicine, 37(28), 4114-4125.More infoNetwork meta-analysis (NMA) has become an increasingly used tool to compare multiple treatments simultaneously by synthesizing direct and indirect evidence in clinical research. However, many existing studies did not properly report the evidence of treatment comparisons and show the comparison structure to audience. In addition, nearly all treatment networks presented only direct evidence, not overall evidence that can reflect the benefit of performing NMAs. This article classifies treatment networks into three types under different assumptions; they include networks with each treatment comparison's edge width proportional to the corresponding number of studies, sample size, and precision. In addition, three new measures (ie, the effective number of studies, the effective sample size, and the effective precision) are proposed to preliminarily quantify overall evidence gained in NMAs. They permit audience to intuitively evaluate the benefit of performing NMAs, compared with pairwise meta-analyses based on only direct evidence. We use four case studies, including one illustrative example, to demonstrate their derivations and interpretations. Treatment networks may look fairly differently when different measures are used to present the evidence. The proposed measures provide clear information about overall evidence of all treatment comparisons, and they also imply the additional number of studies, sample size, and precision obtained from indirect evidence. Some comparisons may benefit little from NMAs. Researchers are encouraged to present overall evidence of all treatment comparisons, so that audience can preliminarily evaluate the quality of NMAs.
- Lin, L. (2018). Re: Incidence and Risk Factors for Prediabetes and Diabetes Mellitus Among HIV-infected Adults on Antiretroviral Therapy: A Systematic Review and Meta-analysis. Epidemiology (Cambridge, Mass.), 29(6), e58.
- Lin, L., & Chu, H. (2018). Bayesian multivariate meta-analysis of multiple factors. Research synthesis methods, 9(2), 261-272.More infoIn medical sciences, a disease condition is typically associated with multiple risk and protective factors. Although many studies report results of multiple factors, nearly all meta-analyses separately synthesize the association between each factor and the disease condition of interest. The collected studies usually report different subsets of factors, and the results from separate analyses on multiple factors may not be comparable because each analysis may use different subpopulation. This may impact on selecting most important factors to design a multifactor intervention program. This article proposes a new concept, multivariate meta-analysis of multiple factors (MVMA-MF), to synthesize all available factors simultaneously. By borrowing information across factors, MVMA-MF can improve statistical efficiency and reduce biases compared with separate analyses when factors were missing not at random. As within-study correlations between factors are commonly unavailable from published articles, we use a Bayesian hybrid model to perform MVMA-MF, which effectively accounts for both within- and between-study correlations. The performance of MVMA-MF and the conventional methods are compared using simulations and an application to a pterygium dataset consisting of 29 studies on 8 risk factors.
- Lin, L., & Chu, H. (2018). Quantifying publication bias in meta-analysis. Biometrics, 74(3), 785-794.More infoPublication bias is a serious problem in systematic reviews and meta-analyses, which can affect the validity and generalization of conclusions. Currently, approaches to dealing with publication bias can be distinguished into two classes: selection models and funnel-plot-based methods. Selection models use weight functions to adjust the overall effect size estimate and are usually employed as sensitivity analyses to assess the potential impact of publication bias. Funnel-plot-based methods include visual examination of a funnel plot, regression and rank tests, and the nonparametric trim and fill method. Although these approaches have been widely used in applications, measures for quantifying publication bias are seldom studied in the literature. Such measures can be used as a characteristic of a meta-analysis; also, they permit comparisons of publication biases between different meta-analyses. Egger's regression intercept may be considered as a candidate measure, but it lacks an intuitive interpretation. This article introduces a new measure, the skewness of the standardized deviates, to quantify publication bias. This measure describes the asymmetry of the collected studies' distribution. In addition, a new test for publication bias is derived based on the skewness. Large sample properties of the new measure are studied, and its performance is illustrated using simulations and three case studies.
- Lin, L., & Chu, H. (2018). Rejoinder to "quantifying publication bias in meta-analysis". Biometrics, 74(3), 801-802.
- Lin, L., Chu, H., Murad, M. H., Hong, C., Qu, Z., Cole, S. R., & Chen, Y. (2018). Empirical Comparison of Publication Bias Tests in Meta-Analysis. Journal of general internal medicine, 33(8), 1260-1267.More infoDecision makers rely on meta-analytic estimates to trade off benefits and harms. Publication bias impairs the validity and generalizability of such estimates. The performance of various statistical tests for publication bias has been largely compared using simulation studies and has not been systematically evaluated in empirical data.
- Ma, X., Lin, L., Qu, Z., Zhu, M., & Chu, H. (2018). Performance of Between-study Heterogeneity Measures in the Cochrane Library. Epidemiology (Cambridge, Mass.), 29(6), 821-824.More infoThe growth in comparative effectiveness research and evidence-based medicine has increased attention to systematic reviews and meta-analyses. Meta-analysis synthesizes and contrasts evidence from multiple independent studies to improve statistical efficiency and reduce bias. Assessing heterogeneity is critical for performing a meta-analysis and interpreting results. As a widely used heterogeneity measure, the I statistic quantifies the proportion of total variation across studies that is caused by real differences in effect size. The presence of outlying studies can seriously exaggerate the I statistic. Two alternative heterogeneity measures, the (Equation is included in full-text article.)and (Equation is included in full-text article.)have been recently proposed to reduce the impact of outlying studies. To evaluate these measures' performance empirically, we applied them to 20,599 meta-analyses in the Cochrane Library. We found that the (Equation is included in full-text article.)and (Equation is included in full-text article.)have strong agreement with the I, while they are more robust than the I when outlying studies appear.
- Murad, M. H., Chu, H., Lin, L., & Wang, Z. (2018). The effect of publication bias magnitude and direction on the certainty in evidence. BMJ evidence-based medicine, 23(3), 84-86.More infoPublication bias occurs when studies with statistically significant results have increased likelihood of being published. Publication bias is commonly associated with inflated treatment effect which lowers the certainty of decision makers about the evidence. In this guide we propose that systematic reviewers and decision makers consider the direction and magnitude of publication bias, as opposed to just the binary determination of the presence of this bias, before lowering their certainty in the evidence. Direction of bias may not always exaggerate the treatment effect. The presence of bias with a trivial magnitude may not affect the decision at hand. Various statistical approaches are available to determine the direction and magnitude of publication bias.
- Wu, Y., Lin, L., Shen, Y., & Wu, H. (2018). Comparison between PD-1/PD-L1 inhibitors (nivolumab, pembrolizumab, and atezolizumab) in pretreated NSCLC patients: Evidence from a Bayesian network model. International journal of cancer, 143(11), 3038-3040.
- Lin, L., Chu, H., & Hodges, J. S. (2017). Alternative measures of between-study heterogeneity in meta-analysis: Reducing the impact of outlying studies. Biometrics, 73(1), 156-166.More infoMeta-analysis has become a widely used tool to combine results from independent studies. The collected studies are homogeneous if they share a common underlying true effect size; otherwise, they are heterogeneous. A fixed-effect model is customarily used when the studies are deemed homogeneous, while a random-effects model is used for heterogeneous studies. Assessing heterogeneity in meta-analysis is critical for model selection and decision making. Ideally, if heterogeneity is present, it should permeate the entire collection of studies, instead of being limited to a small number of outlying studies. Outliers can have great impact on conventional measures of heterogeneity and the conclusions of a meta-analysis. However, no widely accepted guidelines exist for handling outliers. This article proposes several new heterogeneity measures. In the presence of outliers, the proposed measures are less affected than the conventional ones. The performance of the proposed and conventional heterogeneity measures are compared theoretically, by studying their asymptotic properties, and empirically, using simulations and case studies.
- Lin, L., Zhang, J., Hodges, J. S., & Chu, H. (2017). Performing Arm-Based Network Meta-Analysis in R with the pcnetmeta Package. Journal of statistical software, 80.More infoNetwork meta-analysis is a powerful approach for synthesizing direct and indirect evidence about multiple treatment comparisons from a collection of independent studies. At present, the most widely used method in network meta-analysis is contrast-based, in which a baseline treatment needs to be specified in each study, and the analysis focuses on modeling relative treatment effects (typically log odds ratios). However, population-averaged treatment-specific parameters, such as absolute risks, cannot be estimated by this method without an external data source or a separate model for a reference treatment. Recently, an arm-based network meta-analysis method has been proposed, and the R package provides user-friendly functions for its implementation. This package estimates both absolute and relative effects, and can handle binary, continuous, and count outcomes.
- Lin, L., Chu, H., & Hodges, J. S. (2016). Sensitivity to Excluding Treatments in Network Meta-analysis. Epidemiology (Cambridge, Mass.), 27(4), 562-9.More infoNetwork meta-analysis of randomized controlled trials is increasingly used to combine both direct evidence comparing treatments within trials and indirect evidence comparing treatments across different trials. When the outcome is binary, the commonly used contrast-based network meta-analysis methods focus on relative treatment effects such as odds ratios comparing two treatments. As shown in a recent report, when using contrast-based network meta-analysis, the impact of excluding a treatment in the network can be substantial, suggesting a methodological limitation. In addition, relative treatment effects are sometimes not sufficient for patients to make decisions. For example, it can be challenging for patients to trade off efficacy and safety for two drugs if they only know the relative effects, not the absolute effects. A recently proposed arm-based network meta-analysis, based on a missing-data framework, provides an alternative approach. It focuses on estimating population-averaged treatment-specific absolute effects. This article examines the influence of treatment exclusion empirically using 14 published network meta-analyses, for both arm- and contrast-based approaches. The difference between these two approaches is substantial, and it is almost entirely due to single-arm trials. When a treatment is removed from a contrast-based network meta-analysis, it is necessary to exclude other treatments in two-arm studies that investigated the excluded treatment; such exclusions are not necessary in arm-based network meta-analysis, leading to substantial gain in performance.
- Xu, G., Lin, L., Wei, P., & Pan, W. (2016). An adaptive two-sample test for high-dimensional means. Biometrika, 103(3), 609-624.More infoSeveral two-sample tests for high-dimensional data have been proposed recently, but they are powerful only against certain limited alternative hypotheses. In practice, since the true alternative hypothesis is unknown, it is unclear how to choose a powerful test. We propose an adaptive test that maintains high power across a wide range of situations, and study its asymptotic properties. Its finite sample performance is compared with existing tests. We apply it and other tests to detect possible associations between bipolar disease and a large number of single nucleotide polymorphisms on each chromosome based on a genome-wide association study dataset. Numerical studies demonstrate the superior performance and high power of the proposed test across a wide spectrum of applications.
Presentations
- Lin, L. (2023). A Bayesian model for combining standardized mean differences and odds ratios in the same meta-analysis. 2023 Western North American Region (WNAR) Annual Meeting. Anchorage, AK: International Biometric Society.
- Lin, L. (2023). A Bayesian model for combining standardized mean differences and odds ratios in the same meta-analysis. The 64th World Statistics Congress. Ottawa, Canada: International Statistical Institute.
- Lin, L. (2023). Alternative tests and measures for between-study inconsistency in meta-analysis. The 16th International Conference of the European Research Consortium for Informatics and Mathematics (ERCIM) Working Group on Computational and Methodological Statistics (CMStatistics 2023). Berlin, Germany.
- Lin, L. (2023). Bayesian model for combining standardized mean differences and odds ratios in the same meta-analysis. 2023 Joint Statistical Meetings (JSM). Toronto, Canada: American Statistical Association.
- Lin, L. (2023). Refined methods for trial sequential analyses in living systematic reviews. 2023 IMS International Conference on Statistics and Data Science (ICSDS). Lisbon, Portugal.
- Lin, L. (2023, January). A Bayesian model for combining standardized mean differences and odds ratios in the same meta-analysis. 2023 International Conference on Health Policy Statistics (ICHPS). Scottsdale, AZ.
- Lin, L. (2022, February). Innovative statistical methods for assessing publication bias. Seminar, Department of Epidemiology and Biostatistics, Mel and Enid Zuckerman College of Public Health, University of Arizona. Virtual.
- Lin, L. (2022, September). Assessing research replicability in multiple studies. Seminar, Statistics & Data Science Colloquium, Graduate Interdisciplinary Program. Tucson, AZ.
- Lin, L. (2021, April). Evidence inconsistency degrees of freedom in Bayesian network meta-analysis. 2021 Duke Industry Statistics Symposium (DISS2021). Virtual.
- Lin, L. (2021, August). Predictive treatment ranking in Bayesian network meta-analysis. 2021 Joint Statistical Meetings (JSM). Virtual.
- Lin, L. (2021, June). Evaluation of various estimators for standardized mean difference in meta-analysis. 2021 International Biometric Society Western North American Region (WNAR) Annual Meeting. Virtual.
- Lin, L. (2021, September). A Bayesian model for combining standardized mean differences and odds ratios in the same meta-analysis. 2021 International Chinese Statistical Association (ICSA) Applied Statistics Symposium. Virtual.
- Lin, L. (2020, December). Predictive treatment ranking in Bayesian network meta-analysis. 2020 International Chinese Statistical Association (ICSA) Applied Statistics Symposium. Virtual.
- Lin, L. (2020, May). Hybrid test for publication bias in meta-analysis. The 2020 Meeting of the International Society for Data Science and Analytics (ISDSA). Virtual.
- Lin, L. (2020, November). Treatment ranking in Bayesian network meta-analysis and predictions. Seminar, Division of Biostatistics, Department of Preventive Medicine, University of Tennessee Health Science Center. Virtual.
- Lin, L. (2019, April). Robust statistical methods and software for meta-analysis with outlying studies. Webinar, Agency for Healthcare Research and Quality (AHRQ) Evidence-Based Practice Centers (EPC). Virtual.
- Lin, L. (2019, August). Powerful methods for assessing publication bias. The 62nd International Statistical Institute (ISI) World Statistics Congress. Kuala Lumpur, Malaysia.
- Lin, L. (2019, December). Hybrid test for publication bias in meta-analysis. The 12th International Conference of the European Research Consortium for Informatics and Mathematics (ERCIM) Working Group on Computational and Methodological Statistics (CMStatistics 2019). London, UK.
- Lin, L. (2019, July). Borrowing of strength from indirect evidence in network meta-analyses. 2019 International Chinese Statistical Association (ICSA) China Conference. Tianjin, China.
- Lin, L. (2019, July). Innovative methods for assessing publication bias in meta-analysis. 2019 Joint Statistical Meetings (JSM). Denver, CO.
- Lin, L. (2019, June). Innovative methods for assessing publication bias. Seminar, School of Statistics, Renmin University of China. Beijing, China.
- Lin, L. (2019, June). On the efficiency of network meta-analysis. 2019 International Chinese Statistical Association (ICSA) Applied Statistics Symposium. Raleigh, NC.
- Lin, L. (2019, June). On the efficiency of network meta-analysis. The 3rd International Conference on Econometrics and Statistics (EcoSta 2019). Taichung, Taiwan.
- Lin, L. (2019, May). Borrowing of strength from indirect evidence in network meta-analyses. Society for Clinical Trials 40th Annual Meeting. New Orleans, LA.
- Lin, L. (2019, November). Magnitude of publication bias: an empirical study of nearly 30,000 meta-analyses. American Public Health Association (APHA) 2019 Annual Meeting and Expo. Philadelphia, PA.
- Lin, L. (2019, October). Methods for multivariate meta-analysis and quantifying reproducibility. Mini-Symposium on Research Synthesis Methods. Minneapolis, MN: Division of Biostatistics, University of Minnesota.
- Lin, L. (2019, September). Predictive treatment ranking in Bayesian network meta-analysis. Seminar, Department of Statistics, Florida State University. Tallahassee, FL.
- Lin, L. (2018, April). Efficiency of network meta-analysis. Synthesis Research Group Meeting, Florida State University College of Education. Tallahassee, FL.
- Lin, L. (2018, March). Quantifying and presenting overall evidence in network meta-analysis. 2018 International Biometric Society Eastern North American Region (ENAR) Spring Meeting. Atlanta, GA.
- Lin, L. (2018, May). Sampling error in meta-analysis with small sample sizes. 2018 International Indian Statistical Association (IISA) International Conference on Statistics. Gainesville, FL.
- Lin, L. (2017, February). On evidence cycles in network meta-analysis. Seminar, Department of Biostatistics, Brown University. Providence, RI.
- Lin, L. (2017, February). On evidence cycles in network meta-analysis. Seminar, Department of Epidemiology and Biostatistics, Memorial Sloan Kettering Cancer Center. New York, NY.
- Lin, L. (2017, January). On evidence cycles in network meta-analysis. Seminar, Department of Biostatistics and Computational Biology, University of Rochester Medical Center. Rochester, NY.
- Lin, L. (2017, January). On evidence cycles in network meta-analysis. Seminar, Department of Statistics, Florida State University. Tallahassee, FL.
- Lin, L. (2017, July). Quantifying publication bias in meta-analysis. 2017 Joint Statistical Meetings (JSM). Baltimore, MD.
- Lin, L. (2017, June). On evidence cycles in network meta-analysis. 2017 International Chinese Statistical Association (ICSA) Applied Statistics Symposium. Chicago, IL.
- Lin, L. (2017, March). Quantifying publication bias in meta-analysis. 2017 International Biometric Society Eastern North American Region (ENAR) Spring Meeting. Washington, DC.
- Lin, L. (2017, November). Assessing publication bias in meta-analysis. Seminar, Department of Statistics, University of Florida. Gainesville, FL.
- Lin, L. (2017, October). Assessing publication bias in meta-analysis. Seminar, Department of Statistics, University of South Carolina. Columbia, SC.
- Lin, L. (2016, August). Network meta-analysis of multiple factors. 2016 Joint Statistical Meetings (JSM). Chicago, IL.
- Lin, L. (2016, February). Alternative measures of between-study heterogeneity in meta-analysis: reducing the impact of outlying studies. Division of Biostatistics Student Seminar, University of Minnesota. Minneapolis, MN.
- Lin, L. (2016, March). Alternative measures of between-study heterogeneity in meta-analysis: reducing the impact of outlying studies. 2016 International Biometric Society Eastern North American Region (ENAR) Spring Meeting. Austin, TX.
- Lin, L. (2015, May). Sensitivity to excluding treatments in network meta-analysis. Society for Clinical Trials 36th Annual Meeting. Arlington, VA.
Poster Presentations
- Lin, L. (2018, September). The magnitude of publication bias: an empirical study of nearly 30,000 meta-analyses. First-Year Assistant Professor Workshop. Tallahassee, FL: Florida State University Council on Research and Creativity.
- Lin, L. (2017, April). Quantifying publication bias in meta-analysis. University of Minnesota School of Public Health Research Day. Minneapolis, MN.
- Lin, L. (2017, April). Statistical methods for meta-analysis. Doctoral Research Showcase. Minneapolis, MN.
- Lin, L. (2017, May). Quantifying publication bias in meta-analysis. The 40th Annual Midwest Biopharmaceutical Statistics Workshop. Muncie, IN.
- Lin, L. (2016, April). Network meta-analysis of multiple factors. University of Minnesota School of Public Health Research Day. Minneapolis, MN.
- Lin, L. (2016, November). Quantifying publication bias in meta-analysis. Council of International Graduate Students Fall 2016 International Graduate Research Showcase. Minneapolis, MN.
- Lin, L. (2016, October). Quantifying publication bias in meta-analysis. The 2nd Annual Twin Cities American Statistical Association Fall Research Meeting. Mounds View, MN.
- Lin, L. (2015, October). Alternative measures of between-study heterogeneity in meta-analysis: reducing the impact of outlying studies. The 1st Annual Twin Cities American Statistical Association Fall Research Meeting. Rochester, MN.
Others
- Madhivanan, P., & Lin, L. (2022, November). Introduction to Systematic Review and Meta-Analysis. One-day workshop.