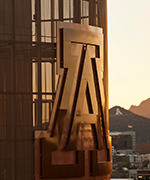
Mihai Surdeanu
- Professor, Computer Science
- Associate Professor, Cognitive Science - GIDP
- Professor, BIO5 Institute
- Member of the Graduate Faculty
Contact
- (520) 626-2706
- Gould-Simpson, Rm. 745
- Tucson, AZ 85721
- msurdeanu@arizona.edu
Awards
- Distinguished Early Career Teaching Award
- College of Science, UA, Fall 2018
Interests
No activities entered.
Courses
2025-26 Courses
-
Dissertation
CSC 920 (Fall 2025) -
Independent Study
CSC 599 (Fall 2025) -
Special Topics in Comp Science
CSC 396 (Fall 2025)
2024-25 Courses
-
AdvTpc Artificial Intelligence
CSC 696H (Spring 2025) -
Dissertation
CSC 920 (Spring 2025) -
Honors Independent Study
CSC 399H (Spring 2025) -
Honors Thesis
CSC 498H (Spring 2025) -
Independent Study
COGS 699 (Spring 2025) -
Independent Study
CSC 399 (Spring 2025) -
Research
CSC 900 (Spring 2025) -
Dissertation
CSC 920 (Fall 2024) -
Honors Directed Research
HNRS 492H (Fall 2024) -
Honors Thesis
CSC 498H (Fall 2024) -
Independent Study
CSC 699 (Fall 2024) -
Research
CSC 900 (Fall 2024) -
Special Topics in Comp Science
CSC 396 (Fall 2024)
2023-24 Courses
-
Dissertation
CSC 920 (Spring 2024) -
Honors Independent Study
CSC 499H (Spring 2024) -
Honors Thesis
CSC 498H (Spring 2024) -
Independent Study
CSC 599 (Spring 2024) -
Independent Study
CSC 699 (Spring 2024) -
Research
CSC 900 (Spring 2024) -
Text Retrieval & Web Search
CSC 483 (Spring 2024) -
Text Retrieval & Web Search
CSC 583 (Spring 2024) -
Dissertation
CSC 920 (Fall 2023) -
Honors Independent Study
CSC 499H (Fall 2023) -
Honors Thesis
CSC 498H (Fall 2023) -
Research
CSC 900 (Fall 2023)
2022-23 Courses
-
Dissertation
CSC 920 (Spring 2023) -
Honors Independent Study
CSC 399H (Spring 2023) -
Honors Thesis
CSC 498H (Spring 2023) -
Independent Study
CSC 599 (Spring 2023) -
Research
CSC 900 (Spring 2023) -
Text Retrieval & Web Search
CSC 483 (Spring 2023) -
Text Retrieval & Web Search
CSC 583 (Spring 2023) -
Dissertation
CSC 920 (Fall 2022) -
Honors Independent Study
CSC 399H (Fall 2022) -
Independent Study
CSC 599 (Fall 2022) -
Research
CSC 900 (Fall 2022) -
Thesis
CSC 910 (Fall 2022)
2021-22 Courses
-
AdvTpc Artificial Intelligence
CSC 696H (Spring 2022) -
Dissertation
CSC 920 (Spring 2022) -
Honors Thesis
CSC 498H (Spring 2022) -
Research
CSC 900 (Spring 2022) -
Thesis
CSC 910 (Spring 2022) -
Dissertation
CSC 920 (Fall 2021) -
Honors Thesis
CSC 498H (Fall 2021) -
Independent Study
CSC 599 (Fall 2021) -
Research
CSC 900 (Fall 2021) -
Text Retrieval & Web Search
CSC 483 (Fall 2021) -
Text Retrieval & Web Search
CSC 583 (Fall 2021) -
Thesis
CSC 910 (Fall 2021)
2020-21 Courses
-
Dissertation
CSC 920 (Spring 2021) -
Research
CSC 900 (Spring 2021) -
Dissertation
CSC 920 (Fall 2020) -
Honors Thesis
CSC 498H (Fall 2020) -
Independent Study
CSC 599 (Fall 2020) -
Research
CSC 900 (Fall 2020) -
Text Retrieval & Web Search
CSC 483 (Fall 2020) -
Text Retrieval & Web Search
CSC 583 (Fall 2020)
2019-20 Courses
-
Adv Tpcs:Doctoral Colloq
CSC 695C (Spring 2020) -
Dissertation
CSC 920 (Spring 2020) -
Honors Thesis
CSC 498H (Spring 2020) -
Research
CSC 900 (Spring 2020) -
Thesis
CSC 910 (Spring 2020) -
Adv Tpcs:Doctoral Colloq
CSC 695C (Fall 2019) -
Honors Thesis
CSC 498H (Fall 2019) -
Research
CSC 900 (Fall 2019) -
Thesis
CSC 910 (Fall 2019)
2018-19 Courses
-
Adv Tpcs:Doctoral Colloq
CSC 695C (Spring 2019) -
Independent Study
CSC 699 (Spring 2019) -
Research
CSC 900 (Spring 2019) -
Text Retrieval & Web Search
CSC 483 (Spring 2019) -
Text Retrieval & Web Search
CSC 583 (Spring 2019) -
Thesis
CSC 910 (Spring 2019) -
Adv Tpcs:Doctoral Colloq
CSC 695C (Fall 2018) -
Algorithms for NLP
CSC 585 (Fall 2018) -
Research
CSC 900 (Fall 2018) -
Thesis
CSC 910 (Fall 2018)
2017-18 Courses
-
Adv Tpcs:Doctoral Colloq
CSC 695C (Spring 2018) -
Dissertation
CSC 920 (Spring 2018) -
Research
CSC 900 (Spring 2018) -
Adv Tpcs:Doctoral Colloq
CSC 695C (Fall 2017) -
Dissertation
CSC 920 (Fall 2017) -
Honors Thesis
CSC 498H (Fall 2017) -
Independent Study
CSC 599 (Fall 2017) -
Independent Study
CSC 699 (Fall 2017) -
Stat Nat Lang Processing
CSC 439 (Fall 2017) -
Stat Nat Lang Processing
CSC 539 (Fall 2017) -
Stat Nat Lang Processing
LING 439 (Fall 2017) -
Stat Nat Lang Processing
LING 539 (Fall 2017)
2016-17 Courses
-
Adv Tpcs:Doctoral Colloq
CSC 695C (Spring 2017) -
Dissertation
CSC 920 (Spring 2017) -
Research
CSC 900 (Spring 2017) -
Text Retrieval & Web Search
CSC 483 (Spring 2017) -
Text Retrieval & Web Search
CSC 583 (Spring 2017) -
Adv Tpcs:Doctoral Colloq
CSC 695C (Fall 2016) -
Dissertation
CSC 920 (Fall 2016) -
Independent Study
CSC 599 (Fall 2016) -
Independent Study
CSC 699 (Fall 2016)
2015-16 Courses
-
Adv Tpcs Computat Intell
CSC 665 (Spring 2016) -
Dissertation
CSC 920 (Spring 2016) -
Independent Study
CSC 599 (Spring 2016) -
Independent Study
CSC 699 (Spring 2016) -
Research
CSC 900 (Spring 2016) -
Thesis
CSC 910 (Spring 2016)
Scholarly Contributions
Journals/Publications
- Rains, S. A., Hingle, M. D., Surdeanu, M., Bell, D., & Kobourov, S. (2018). A Test of The Risk Perception Attitude Framework as a Message Tailoring Strategy to Promote Diabetes Screening. Health Communication.
- Valenzuela-Escarcega, M. A., Babur, O., Hahn-Powel, G., Bell, D., Hicks, T., Noriega-Atala, E., Wang, X., Surdeanu, M., Demir, E., & Morrison, C. T. (2018). Large-scale Automated Machine Reading Discovers New Cancer Driving Mechanisms. Database: The Journal of Biological Databases and Curation.
- Zhou, J., Bell, D., Nusrat, S., Hingle, M. D., Surdeanu, M., & Kobourov, S. (2018). A Study of Calorie Estimation in Pictures of Food. Journal of Medical Internet Research (JMIR).More infoThis journal has a C rank in CORE.
- Hahn-Powell, G., Valenzuela-Escarcega, M. A., & Surdeanu, M. (2017). Swanson linking revisited: Accelerating literature-based discovery across domains using a conceptual influence graph. Proceedings of ACL 2017, System Demonstrations, 103--108.More infoThis conference is ranked A* in CORE.
- Jansen, P., Sharp, R., Surdeanu, M., & Clark, P. (2017). Framing QA as Building and Ranking Intersentence Answer Justifications. Computational Linguistics.More infoThis journal is ranked A* in CORE.
- Lee, H., Surdeanu, M., & Jurafsky, D. (2017). A scaffolding approach to coreference resolution integrating statistical and rule-based models. Natural Language Engineering, 1--30.More infoThis journal is ranked A in CORE.
- Fried, D., Jansen, P., Hahn-Powell, G., Surdeanu, M., & Clark, P. (2015). Higher-order Lexical Semantic Models for Non-factoid Answer Reranking. Transactions of the Association for Computational Linguistics, 3, 197--210.
- Intxaurrondo, A., Surdeanu, M., Lopez, O., & Agirre, E. (2013). Removing noisy mentions for distant supervision. Procesamiento de Lenguaje Natural, 51, 41-48.More infoAbstract: Relation Extraction methods based on Distant Supervision rely on true tuples to retrieve noisy mentions, which are then used to train traditional supervised relation extraction methods. In this paper we analyze the sources of noise in the mentions, and explore simple methods to filter out noisy mentions. The results show that a combination of mention frequency cut-off, Pointwise Mutual Information and removal of mentions which are far from the feature centroids of relation labels is able to significantly improve the results of two relation extraction models. © 2013 Sociedad Española Para el Procesamiento del Lenguaje Natural.
- Lee, H., Chang, A., Peirsman, Y., Chambers, N., Surdeanu, M., & Jurafsky, D. (2013). Deterministic Coreference Resolution Based on Entity-Centric, Precision-Ranked Rules. Computational Linguistics, 39(4), 885-916.More infoAbstract: We propose a new deterministic approach to coreference resolution that combines the global information and precise features of modern machine-learning models with the transparency and modularity of deterministic, rule-based systems. Our sieve architecture applies a battery of deterministic coreference models one at a time from highest to lowest precision, where each model builds on the previous model's cluster output. The two stages of our sieve-based architecture, a mention detection stage that heavily favors recall, followed by coreference sieves that are precision-oriented, offer a powerful way to achieve both high precision and high recall. Further, our approach makes use of global information through an entity-centric model that encourages the sharing of features across all mentions that point to the same real-world entity. Despite its simplicity, our approach gives state-of-the-art performance on several corpora and genres, and has also been incorporated into hybrid state-of-the-art coreference systems for Chinese and Arabic. Our system thus offers a new paradigm for combining knowledge in rule-based systems that has implications throughout computational linguistics. © 2013 Association for Computational Linguistics.
- Surdeanu, M., & Jeruss, S. (2013). Identifying patent monetization entities. Proceedings of the International Conference on Artificial Intelligence and Law, 131-139.More infoAbstract: The United States has seen an explosion in patent litigation lawsuits in recent years. Recent studies indicate that a large proportion of these lawsuits, increasing from 22% in 2007 to 40% in 2011, were filed by patent monetization entities (PMEs), i.e., companies that hold patents, license patents, and file patent lawsuits, but do not sell products or provide services practicing the technologies described in their patents. We introduce a classifier that identifies which patent litigation lawsuits are initiated by PMEs. Using features extracted from the entities' litigation behavior, the patents they asserted, and their presence on the web, the proposed classifier correctly separates PMEs from operating companies with a F1 score of 85%. We believe that such a classifier will be a useful tool to policy makers and patent litigators, allowing them to gain a clearer picture of the 37, 000+ patent lawsuits filed to date and assessing newly filed cases in real time. Copyright 2013 ACM.
- Zapirain, B., Agirre, E., Marquez, L., & Surdeanu, M. (2013). Selectional preferences for semantic role classification. Computational Linguistics, 39(3), 631-663.More infoAbstract: This paper focuses on a well-known open issue in Semantic Role Classification (SRC) research: the limited influence and sparseness of lexical features. We mitigate this problem using models that integrate automatically learned selectional preferences (SP). We explore a range of models based onWordNet and distributional-similarity SPs. Furthermore, we demonstrate that the SRC task is better modeled by SP models centered on both verbs and prepositions, rather than verbs alone. Our experiments with SP-based models in isolation indicate that they outperform a lexical baseline with 20 F1 points in domain and almost 40 F1 points out of domain. Furthermore, we show that a state-of-the-art SRC system extended with features based on selectional preferences performs significantly better, both in domain (17% error reduction) and out of domain (13% error reduction). Finally, we show that in an end-to-end semantic role labeling system we obtain small but statistically significant improvements, even though our modified SRC model affects only approximately 4% of the argument candidates. Our post hoc error analysis indicates that the SP-based features help mostly in situations where syntactic information is either incorrect or insufficient to disambiguate the correct role. © 2013 Association for Computational Linguistics.
- Dominguez-Sal, D., Aguilar-Saborit, J., Surdeanu, M., & Larriba-Pey, J. L. (2012). Using evolutive summary counters for efficient cooperative caching in search engines. IEEE Transactions on Parallel and Distributed Systems, 23(4), 776-784.More infoAbstract: We propose and analyze a distributed cooperative caching strategy based on the Evolutive Summary Counters (ESC), a new data structure that stores an approximated record of the data accesses in each computing node of a search engine. The ESC capture the frequency of accesses to the elements of a data collection, and the evolution of the access patterns for each node in a network of computers. The ESC can be efficiently summarized into what we call ESC-summaries to obtain approximate statistics of the document entries accessed by each computing node. We use the ESC-summaries to introduce two algorithms that manage our distributed caching strategy, one for the distribution of the cache contents, ESC-placement, and another one for the search of documents in the distributed cache, ESC-search. While the former improves the hit rate of the system and keeps a large ratio of data accesses local, the latter reduces the network traffic by restricting the number of nodes queried to find a document. We show that our cooperative caching approach outperforms state-of-the-art models in both hit rate, throughput, and location recall for multiple scenarios, i.e., different query distributions and systems with varying degrees of complexity. © 2012 IEEE.
- Lee, H., Recasens, M., Chang, A., Surdeanu, M., & Jurafsky, D. (2012). Joint entity and event coreference resolution across documents. EMNLP-CoNLL 2012 - 2012 Joint Conference on Empirical Methods in Natural Language Processing and Computational Natural Language Learning, Proceedings of the Conference, 489-500.More infoAbstract: We introduce a novel coreference resolution system that models entities and events jointly. Our iterative method cautiously constructs clusters of entity and event mentions using linear regression to model cluster merge operations. As clusters are built, information flows between entity and event clusters through features that model semantic role dependencies. Our system handles nominal and verbal events as well as entities, and our joint formulation allows information from event coreference to help entity coreference, and vice versa. In a cross-document domain with comparable documents, joint coreference resolution performs significantly better (over 3 CoNLL F1 points) than two strong baselines that resolve entities and events separately. © 2012 Association for Computational Linguistics.
- McClosky, D., Riedel, S., Surdeanu, M., McCallum, A., & Manning, C. D. (2012). Combining joint models for biomedical event extraction.. BMC bioinformatics, 13 Suppl 11, S9.More infoPMID: 22759463;PMCID: PMC3395172;Abstract: We explore techniques for performing model combination between the UMass and Stanford biomedical event extraction systems. Both sub-components address event extraction as a structured prediction problem, and use dual decomposition (UMass) and parsing algorithms (Stanford) to find the best scoring event structure. Our primary focus is on stacking where the predictions from the Stanford system are used as features in the UMass system. For comparison, we look at simpler model combination techniques such as intersection and union which require only the outputs from each system and combine them directly. First, we find that stacking substantially improves performance while intersection and union provide no significant benefits. Second, we investigate the graph properties of event structures and their impact on the combination of our systems. Finally, we trace the origins of events proposed by the stacked model to determine the role each system plays in different components of the output. We learn that, while stacking can propose novel event structures not seen in either base model, these events have extremely low precision. Removing these novel events improves our already state-of-the-art F1 to 56.6% on the test set of Genia (Task 1). Overall, the combined system formed via stacking ("FAUST") performed well in the BioNLP 2011 shared task. The FAUST system obtained 1st place in three out of four tasks: 1st place in Genia Task 1 (56.0% F1) and Task 2 (53.9%), 2nd place in the Epigenetics and Post-translational Modifications track (35.0%), and 1st place in the Infectious Diseases track (55.6%). We present a state-of-the-art event extraction system that relies on the strengths of structured prediction and model combination through stacking. Akin to results on other tasks, stacking outperforms intersection and union and leads to very strong results. The utility of model combination hinges on complementary views of the data, and we show that our sub-systems capture different graph properties of event structures. Finally, by removing low precision novel events, we show that performance from stacking can be further improved.
- Surdeanu, M., Tibshirani, J., Nallapati, R., & Manning, C. D. (2012). Multi-instance multi-label learning for relation extraction. EMNLP-CoNLL 2012 - 2012 Joint Conference on Empirical Methods in Natural Language Processing and Computational Natural Language Learning, Proceedings of the Conference, 455-465.More infoAbstract: Distant supervision for relation extraction (RE) - gathering training data by aligning a database of facts with text - is an efficient approach to scale RE to thousands of different relations. However, this introduces a challenging learning scenario where the relation expressed by a pair of entities found in a sentence is unknown. For example, a sentence containing Balzac and France may express BornIn or Died, an unknown relation, or no relation at all. Because of this, traditional supervised learning, which assumes that each example is explicitly mapped to a label, is not appropriate. We propose a novel approach to multi-instance multi-label learning for RE, which jointly models all the instances of a pair of entities in text and all their labels using a graphical model with latent variables. Our model performs competitively on two difficult domains. © 2012 Association for Computational Linguistics.
- McClosky, D., Surdeanu, M., & Manning, C. D. (2011). Event extraction as dependency parsing. ACL-HLT 2011 - Proceedings of the 49th Annual Meeting of the Association for Computational Linguistics: Human Language Technologies, 1, 1626-1635.More infoAbstract: Nested event structures are a common occurrence in both open domain and domain specific extraction tasks, e.g., a "crime" event can cause a "investigation" event, which can lead to an "arrest" event. However, most current approaches address event extraction with highly local models that extract each event and argument independently. We propose a simple approach for the extraction of such structures by taking the tree of event-argument relations and using it directly as the representation in a reranking dependency parser. This provides a simple framework that captures global properties of both nested and flat event structures. We explore a rich feature space that models both the events to be parsed and context from the original supporting text. Our approach obtains competitive results in the extraction of biomedical events from the BioNLP'09 shared task with a F1 score of 53.5% in development and 48.6% in testing. © 2011 Association for Computational Linguistics.
- Surdeanu, M., Ciaramita, M., & Zaragoza, H. (2011). Learning to rank answers to non-factoid questions from web collections. Computational Linguistics, 37(2), 351-383.More infoAbstract: This work investigates the use of linguistically motivated features to improve search, in particular for ranking answers to non-factoid questions. We show that it is possible to exploit existing large collections of question-answer pairs (from online social Question Answering sites) to extract such features and train ranking models which combine them effectively.We investigate a wide range of feature types, some exploiting natural language processing such as coarse word sense disambiguation, named-entity identification, syntactic parsing, and semantic role labeling. Our experiments demonstrate that linguistic features, in combination, yield considerable improvements in accuracy. Depending on the system settings we measure relative improvements of 14% to 21% in Mean Reciprocal Rank and Precision@1, providing one of the most compelling evidence to date that complex linguistic features such as word senses and semantic roles can have a significant impact on large-scale information retrieval tasks. © 2011 Association for Computational Linguistics.
- Surdeanu, M., Nallapati, R., Gregory, G., Walker, J., & Manning, C. D. (2011). Risk analysis for intellectual property litigation. Proceedings of the International Conference on Artificial Intelligence and Law, 116-120.More infoAbstract: We introduce the problem of risk analysis for Intellectual Property (IP) lawsuits. More specifically, we focus on estimating the risk for participating parties using solely prior factors, i. e., historical and concurrent behavior of the entities involved in the case. This work represents a first step towards building a comprehensive legal risk assessment system for parties involved in litigation. This technology will allow parties to optimize their case parameters to minimize their own risk, or to settle disputes out of court and thereby ease the burden on the judicial system. In addition, it will also help U.S. courts detect and fix any inherent biases in the system. We model risk estimation as a relational classification problem using conditional random fields [6] to jointly estimate the risks of concurrent cases. We evaluate our model on data collected by the Stanford Intellectual Property Litigation Clearinghouse, which consists of over 4,200 IP lawsuits filed across 88 U.S. federal districts and ranging over 8 years, probably the largest legal data set reported in data mining research. Despite being agnostic to the merits of the case, our best model achieves a classification accuracy of 64%, 22% (relative) higher than the majority-class baseline. © 2011 Authors.
- Raghunathan, K., Lee, H., Rangarajan, S., Chambers, N., Surdeanu, M., Jurafsky, D., & Manning, C. (2010). A multi-pass sieve for coreference resolution. EMNLP 2010 - Conference on Empirical Methods in Natural Language Processing, Proceedings of the Conference, 492-501.More infoAbstract: Most coreference resolution models determine if two mentions are coreferent using a single function over a set of constraints or features. This approach can lead to incorrect decisions as lower precision features often overwhelm the smaller number of high precision ones. To overcome this problem, we propose a simple coreference architecture based on a sieve that applies tiers of deterministic coreference models one at a time from highest to lowest precision. Each tier builds on the previous tier's entity cluster output. Further, our model propagates global information by sharing attributes (e.g., gender and number) across mentions in the same cluster. This cautious sieve guarantees that stronger features are given precedence over weaker ones and that each decision is made using all of the information available at the time. The framework is highly modular: new coreference modules can be plugged in without any change to the other modules. In spite of its simplicity, our approach outperforms many state-of-the-art supervised and unsupervised models on several standard corpora. This suggests that sieve-based approaches could be applied to other NLP tasks. © 2010 Association for Computational Linguistics.
- Surdeanu, M., & Manning, C. D. (2010). Ensemble Models for dependency parsing: Cheap and good?. NAACL HLT 2010 - Human Language Technologies: The 2010 Annual Conference of the North American Chapter of the Association for Computational Linguistics, Proceedings of the Main Conference, 649-652.More infoAbstract: Previous work on dependency parsing used various kinds of combination models but a systematic analysis and comparison of these approaches is lacking. In this paper we implemented such a study for English dependency parsing and find several non-obvious facts: (a) the diversity of base parsers is more important than complex models for learning (e.g., stacking, supervised meta-classification), (b) approximate, linear-time re-parsing algorithms guarantee well-formed dependency trees without significant performance loss, and (c) the simplest scoring model for re-parsing (unweighted voting) performs essentially as well as other more complex models. This study proves that fast and accurate ensemble parsers can be built with minimal effort. © 2010 Association for Computational Linguistics.
- Zapirain, B., Agirre, E., Marquez, L., & Surdeanu, M. (2010). Improving semantic role classification with selectional preferences. NAACL HLT 2010 - Human Language Technologies: The 2010 Annual Conference of the North American Chapter of the Association for Computational Linguistics, Proceedings of the Main Conference, 373-376.More infoAbstract: This work incorporates Selectional Preferences (SP) into a Semantic Role (SR) Classification system. We learn separate selectional preferences for noun phrases and prepositional phrases and we integrate them in a state-of-the-art SR classification system both in the form of features and individual class predictors. We show that the inclusion of the refined SPs yields statistically significant improvements on both in domain and out of domain data (14.07% and 11.67% error reduction, respectively). The key factor for success is the combination of several SP methods with the original classification model using meta-classification. © 2010 Association for Computational Linguistics.
- Filippova, K., Surdeanu, M., Ciaramita, M., & Zaragoza, H. (2009). Company-oriented extractive summarization of financial news. EACL 2009 - 12th Conference of the European Chapter of the Association for Computational Linguistics, Proceedings, 246-254.More infoAbstract: The paper presents a multi-document summarization system which builds company-specific summaries from a collection of financial news such that the extracted sentences contain novel and relevant information about the corresponding organization. The user's familiarity with the company's profile is assumed. The goal of such summaries is to provide information useful for the short-term trading of the corresponding company, i.e., to facilitate the inference from news to stock price movement in the next day. We introduce a novel query (i.e., company name) expansion method and a simple unsupervized algorithm for sentence ranking. The system shows promising results in comparison with a competitive baseline. © 2009 Association for Computational Linguistics.
- Hajǐ, J., Ciaramita, M., Johansson, R., Kawahara, D., Martí, M. A., Màrquez, L., Meyers, A., Nivre, J., Padó, S., Šťpánek, J., Strǎák, P., Surdeanu, M., Xue, N., & Zhang, Y. (2009). The CoNLL-2009 shared task: Syntactic and semantic dependencies in multiple languages. CoNLL- 2009: Shared Task - Proceedings of the Thirteenth Conference on Computational Natural Language Learning, CoNLL: Shared Task, 1-18.More infoAbstract: For the 11th straight year, the Conference on Computational Natural Language Learning has been accompanied by a shared task whose purpose is to promote natural language processing applications and evaluate them in a standard setting. In 2009, the shared task was dedicated to the joint parsing of syntactic and semantic dependencies in multiple languages. This shared task combines the shared tasks of the previous five years under a unique dependency-based formalism similar to the 2008 task. In this paper, we define the shared task, describe how the data sets were created and show their quantitative properties, report the results and summarize the approaches of the participating systems. © 2009 Association for Computational Linguistics.
- Ciaramita, M., Attardi, G., Dell'Orletta, F., & Surdeanu, M. (2008). DeSRL: A linear-time semantic role labeling system. CoNLL 2008 - Proceedings of the Twelfth Conference on Computational Natural Language Learning, 258-262.More infoAbstract: This paper describes the DeSRL system, a joined effort of Yahoo! Research Barcelona and Università di Pisa for the CoNLL-2008 Shared Task (Surdeanu et al., 2008). The system is characterized by an efficient pipeline of linear complexity components, each carrying out a different sub-task. Classifier errors and ambiguities are addressed with several strategies: revision models, voting, and reranking. The system participated in the closed challenge ranking third in the complete problem evaluation with the following scores: 82.06 labeled macro F1 for the overall task, 86.6 labeled attachment for syntactic dependencies, and 77.5 labeled F1 for semantic dependencies. © 2008.
- Comas, P. R., Turmo, J., & Surdeanu, M. (2008). Robust question answering for speech transcripts using minimal syntactic analysis. Lecture Notes in Computer Science (including subseries Lecture Notes in Artificial Intelligence and Lecture Notes in Bioinformatics), 5152 LNCS, 424-432.More infoAbstract: This paper describes the participation of the Technical University of Catalonia in the CLEF 2007 Question Answering on Speech Transcripts track. For the processing of manual transcripts we have deployed a robust factual Question Answering that uses minimal syntactic information. For the handling of automatic transcripts we combine the QA system with a novel Passage Retrieval and Answer Extraction engine, which is based on a sequence alignment algorithm that searches for "sounds like" sequences in the document collection. We have also enriched the NERC with phonetic features to facilitate the recognition of named entities even when they are incorrectly transcribed. © 2008 Springer-Verlag Berlin Heidelberg.
- Dominguez-Sal, D., Aguilar-Saborit, J., Surdeanu, M., & Larriba-Pey, J. L. (2008). Cache-aware load balancing for question answering. International Conference on Information and Knowledge Management, Proceedings, 1271-1280.More infoAbstract: The need for high performance and throughput Question Answering (QA) systems demands for their migration to distributed environments. However, even in such cases it is necessary to provide the distributed system with cooper- ative caches and load balancing facilities in order to achieve the desired goals. Until now, the literature on QA has notconsidered such a complex system as a whole. Currently, the load balancer regulates the assignment of tasks based only on the CPU and I/O loads without considering the status of the system cache. This paper investigates the load balancing problem propos- ing two novel algorithms that take into account the dis- tributed cache status, in addition to the CPU and I/O load in each processing node. We have implemented, and tested the proposed algorithms in a fully fledged distributed QA system. The two algorithms show that the choice of using the status of the cache was determinant in achieving good performance, and high throughput for QA systems. Copyright 2008 ACM.
- Surdeanu, M., Ciaramita, M., & Zaragoza, H. (2008). Learning to rank answers on large online QA collections. ACL-08: HLT - 46th Annual Meeting of the Association for Computational Linguistics: Human Language Technologies, Proceedings of the Conference, 719-727.More infoAbstract: This work describes an answer ranking engine for non-factoid questions built using a large online community-generated question-answer collection (Yahoo! Answers). We show how such collections may be used to effectively set up large supervised learning experiments. Furthermore we investigate a wide range of feature types, some exploiting NLP processors, and demonstrate that using them in combination leads to considerable improvements in accuracy. © 2008 Association for Computational Linguistics.
- Surdeanu, M., Johansson, R., Meyers, A., Màrquez, L., & Nivre, J. (2008). The CoNLL-2008 shared task on joint parsing of syntactic and semantic dependencies. CoNLL 2008 - Proceedings of the Twelfth Conference on Computational Natural Language Learning, 159-177.More infoAbstract: The Conference on Computational Natural Language Learning is accompanied every year by a shared task whose purpose is to promote natural language processing applications and evaluate them in a standard setting. In 2008 the shared task was dedicated to the joint parsing of syntactic and semantic dependencies. This shared task not only unifies the shared tasks of the previous four years under a unique dependency-based formalism, but also extends them significantly: this year's syntactic dependencies include more information such as named-entity boundaries; the semantic dependencies model roles of both verbal and nominal predicates. In this paper, we define the shared task and describe how the data sets were created. Furthermore, we report and analyze the results and describe the approaches of the participating systems. © 2008.
- Surdeanu, M., Morante, R., & Màrquez, L. (2008). Analysis of joint inference strategies for the semantic role labeling of Spanish and Catalan. Lecture Notes in Computer Science (including subseries Lecture Notes in Artificial Intelligence and Lecture Notes in Bioinformatics), 4919 LNCS, 206-218.More infoAbstract: This paper analyzes two joint inference approaches for semantic role labeling: re-ranking of candidate semantic frames generated by one local model and combination of two distinct models at argument-level using meta learning. We perform an empirical analysis on two recently released corpora of annotated semantic roles in Spanish and Catalan. This work yields several novel conclusions: (a) the proposed joint inference strategies yield good results even under adverse conditions: small training corpora, only two individual models available for combination, minimal output available from the individual models; (b) stacking of the two joint inference approaches is successful, which indicates that the two inference models provide complementary benefits. Our results are currently the best for the identification of semantic role for Spanish and Catalan. © 2008 Springer-Verlag Berlin Heidelberg.
- Dominguez-Sal, D., Larriba-Pey, J. L., & Surdeanu, M. (2007). A multi-layer collaborative cache for question answering. Lecture Notes in Computer Science (including subseries Lecture Notes in Artificial Intelligence and Lecture Notes in Bioinformatics), 4641 LNCS, 295-306.More infoAbstract: This paper is the first analysis of caching architectures for Question Answering (QA). We introduce the novel concept of multi-layer collaborative caches, where: (a) each resource intensive QA component is allocated a distinct segment of the cache, and (b) the overall cache is transparently spread across all nodes of the distributed system. We empirically analyze the proposed architecture using a real-world QA system installed on a cluster of 16 nodes. Our analysis indicates that multi-layer collaborative caches induce an almost two fold reduction in QA execution time compared to a QA system with local cache. © Springer-Verlag Berlin Heidelberg 2007.
- Poveda, J., Surdeanu, M., & Turmo, J. (2007). A comparison of statistical and rule-induction learners for automatic tagging of time expressions in English. Proceedings of the International Workshop on Temporal Representation and Reasoning, 141-149.More infoAbstract: Proper recognition and handling of temporal information contained in a text is key to understanding the flow of events depicted in the text and their accompanying circumstances. Consequently, time expression recognition and representation of the time information they convey in a suitable normalized form is an important task relevant to several problems in Natural Language Processing. In particular, such an analysis is largely significant for Information Extraction (IE), Question Answering (QA) and Automatic Summarization (AS). The most common approach to time expression recognition in the past has been the use of handmade extraction rules (grammars), which also served as the basis for normalization. Our aim is to explore the possibilities afforded by applying machine learning techniques to the recognition of time expressions. We focus on recognizing the appearances of time expressions in text (not normalization) and transform the problem into one of chunking, where the aim is to correctly assign Begin, Inside or Outside (BIO) tags to tokens. In this paper, we explain the knowledge representation used and compare the results obtained in our experiments with two different methods, one statistical (support vector machines) and one of rule induction (FOIL). Our empirical analysis shows that SVMs are superior. © 2007 IEEE.
- Surdeanu, M., Marquez, L., Carreras, X., & Comas, P. R. (2007). Combination strategies for semantic role labeling. Journal of Artificial Intelligence Research, 29, 105-151.More infoAbstract: This paper introduces and analyzes a battery of inference models for the problem of semantic role labeling: one based on constraint satisfaction, and several strategies that model the inference as a meta-learning problem using discriminative classifiers. These classifiers are developed with a rich set of novel features that encode proposition and sentence-level information. To our knowledge, this is the first work that: (a) performs a thorough analysis of learning-based inference models for semantic role labeling, and (b) compares several inference strategies in this context. We evaluate the proposed inference strategies in the framework of the CoNLL-2005 shared task using only automatically-generated syntactic information. The extensive experimental evaluation and analysis indicates that all the proposed inference strategies are successful -they all outperform the current best results reported in the CoNLL-2005 evaluation exercise- but each of the proposed approaches has its advantages and disadvantages. Several important traits of a state-of-the-art SRL combination strategy emerge from this analysis: (i) individual models should be combined at the granularity of candidate arguments rather than at the granularity of complete solutions; (ii) the best combination strategy uses an inference model based in learning; and (iii) the learning-based inference benefits from max-margin classifiers and global feedback. ©2007 AI Access Foundation. All rights reserved.
- Carreras, X., Surdeanu, M., & Màrquez, L. (2006). Projective dependency parsing with perceptron. Proceedings of the Tenth Conference on Computational Natural Language Learning, CoNLL-X.More infoAbstract: We describe an online learning dependency parser for the CoNLL-X Shared Task, based on the bottom-up projective algorithm of Eisner (2000). We experiment with a large feature set that models: the tokens involved in dependencies and their immediate context, the surfacetext distance between tokens, and the syntactic context dominated by each dependency. In experiments, the treatment of multilingual information was totally blind.
- Surdeanu, M., Dominguez-Sal, D., & Comas, P. R. (2006). Design and performance analysis of a factoid question answering system for spontaneous speech transcriptions. INTERSPEECH 2006 and 9th International Conference on Spoken Language Processing, INTERSPEECH 2006 - ICSLP, 3, 1165-1168.More infoAbstract: This paper introduces a QA designed from scratch to handle speech transcriptions. The system's strength is achieved by analyzing the speech transcriptions with a mix of IR-oriented methodologies and a small number of robust NLP components. We evaluate the system on transcriptions of spontaneous speech from several 1-hour-long seminars and presentations and show that the system obtains encouraging performance.
- Ferrés, D., Kanaan, S., Ageno, A., González, E., Rodríguez, H., Surdeanu, M., & Turmo, J. (2005). The TALP-QA system for Spanish at CLEF 2004: Structural and hierarchical relaxing of semantic constraints. Lecture Notes in Computer Science, 3491, 557-568.More infoAbstract: This paper describes TALP-QA, a multilingual open-domain Question Answering (QA) system that processes both factoid and definition questions. The system is described and evaluated in the context of our participation in the CLEF 2004 Spanish Monolingual QA task. Our approach to factoid questions is to build a semantic representation of the questions and the sentences in the passages retrieved for each question. A set of Semantic Constraints (SC) are extracted for each question. An answer extraction algorithm extracts and ranks sentences that satisfy the SCs of the question. If matches are not possible the algorithm relaxes the SCs structurally (removing constraints) and/or hierarchically (abstracting the constraints using a taxonomy). Answers to definition questions are generated by selecting the text fragment with more density of those terms more frequently related to the question's target (the Named Entity (NE) that appears in the question) throughout the corpus. © Springer-Verlag Berlin Heidelberg 2005.
- Ferrés, D., Kanaan, S., Dominguez-Sal, D., González, E., Ageno, A., Fuentes, M., Rodríguez, H., Surdeanu, M., & Turmo, J. (2005). TALP-UPC at TREC 2005: Experiments using a voting scheme among three heterogeneous QA systems. NIST Special Publication.More infoAbstract: This paper describes the experiments of the TALPUPC group for factoid and 'other' (definitional) questions at TREC 2005 Main Question Answering (QA) task. Our current approach for factoid questions is based on a voting scheme among three QA systems: TALP-QA (our previous QA system), Sibyl (a new QA system developed at DAMA-UPC and TALP-UPC), and Aranea (a web-based data-driven approach). For defitional questions, we used two different systems: the TALP-QA Definitional system and LCSUM (a Summarization-based system). Our results for factoid questions indicate that the voting strategy improves the accuracy from 7.5% to 17.1%. While these numbers are low (due to technical problems in the Answer Extraction phase of TALPQA system) they indicate that voting is a succesful approach for performance boosting of QA systems. The answer to definitional questions is produced by selecting phrases using set of patterns associated with definitions. Its results are 17.2% of F-score in the best configuration of TALP-QA Definitional system.
- Màrquez, L., Surdeanu, M., Comas, P., & Turmo, J. (2005). A robust combination strategy for semantic role labeling. HLT/EMNLP 2005 - Human Language Technology Conference and Conference on Empirical Methods in Natural Language Processing, Proceedings of the Conference, 644-651.More infoAbstract: This paper focuses on semantic role labeling using automatically-generated syntactic information. A simple and robust strategy for system combination is presented, which allows to partially recover from input parsing errors and to significantly boost results of individual systems. This combination scheme is also very flexible since the individual systems are not required to provide any information other than their solution. Extensive experimental evaluation in the CoNLL- 2005 shared task framework supports our previous claims. The proposed architecture outperforms the best results reported in that evaluation exercise. © 2005 Association for Computational Linguistics.
- Surdeanu, M., & Turmo, J. (2005). Semantic role labeling using complete syntactic analysis. CoNLL 2005 - Proceedings of the Ninth Conference on Computational Natural Language Learning, 221-224.More infoAbstract: In this paper we introduce a semantic role labeling system constructed on top of the full syntactic analysis of text. The labeling problem is modeled using a rich set of lexical, syntactic, and semantic attributes and learned using one-versus-all AdaBoost classifiers. Our results indicate that even a simple approach that assumes that each semantic argument maps into exactly one syntactic phrase obtains encouraging performance, surpassing the best system that uses partial syntax by almost 6%. © 2005 Association for Computational Linguistics.
- Surdeanu, M., Turmo, J., & Ageno, A. (2005). A hybrid unsupervised approach for document clustering. Proceedings of the ACM SIGKDD International Conference on Knowledge Discovery and Data Mining, 685-690.More infoAbstract: We propose a hybrid, unsupervised document clustering approach that combines a hierarchical clustering algorithm with Expectation Maximization. We developed several heuristics to automatically select a subset of the clusters generated by the first algorithm as the initial points of the second one. Furthermore, our initialization algorithm generates not only an initial model for the iterative refinement algorithm but also an estimate of the model dimension, thus eliminating another important element of human supervision. We have evaluated the proposed system on five real-world document collections. The results show that our approach generates clustering solutions of higher quality than both its individual components. Copyright 2005 ACM.
- Surdeanu, M., Turmo, J., & Comelles, E. (2005). Named entity recognition from spontaneous open-domain speech. 9th European Conference on Speech Communication and Technology, 3433-3436.More infoAbstract: This paper presents an analysis of named entity recognition and classification in spontaneous speech transcripts. We annotated a significant fraction of the Switchboard corpus with six named entity classes and investigated a battery of machine learning models that include lexical, syntactic, and semantic attributes. The best recognition and classification model obtains promising results, approaching within 5% a system evaluated on clean textual data.
- Moldovan, D., & Surdeanu, M. (2003). On the role of information retrieval and information extraction in question answering systems. Lecture Notes in Artificial Intelligence (Subseries of Lecture Notes in Computer Science), 2700, 129-147.More infoAbstract: Question Answering, the process of extracting answers to natural language questions is profoundly different from Information Retrieval (IR) or Information Extraction (IE). IR systems allow us to locate relevant documents that relate to a query, but do not specify exactly where the answers are. In IR, the documents of interest are fetched by matching query keywords to the index of the document collection. By contrast, IE systems extrat the information of interest provided the domain of extraction is well defined. In IE systems, the information of interest is in trie form of slot fillers of some predefined templates. The QA technology takes both IR and IE a step further, and provides specific and brief answers to open domain questions formulated naturally. This paper presents the major modules used to build IR, IE and QA systems and Shows similarities, differences and possible trade-offs between the three technologies. © Springer-Verlag Berlin Heidelberg 2003.
- Moldovan, D., Paşca, M., Harabagiu, S., & Surdeanu, M. (2003). Performance issues and error analysis in an open-domain question answering system. ACM Transactions on Information Systems, 21(2), 133-154.More infoAbstract: This paper presents an in-depth analysis of a state-of-the-art Question Answering system. Several scenarios are examined: (1) the performance of each module in a serial baseline system, (2) the impact of feedbacks and the insertion of a logic prover, and (3) the impact of various retrieval strategies and lexical resources. The main conclusion is that the overall performance depends on the depth of natural language processing resources and the tools used for answer finding.
- Surdeanu, M., & Moldovan, D. (2002). Design and performance analysis of a distributed Java Virtual Machine. IEEE Transactions on Parallel and Distributed Systems, 13(6), 611-627.More infoAbstract: This paper introduces DISK, a distributed Java Virtual Machine for networks of heterogenous workstations. Several research issues are addressed. A novelty of the system is its object-based, multiple-writer memory consistency protocol (OMW). The correctness of the protocol and its Java compliance is demonstrated by comparing the nonoperational definitions of Release Consistency, the consistency model implemented by OMW, with the Java Virtual Machine memory consistency model (JVMC), as defined in the Java Virtual Machine Specification. An analytical performance model was developed to study and compare the design trade-offs between OMW and the lazy invalidate Release Consistency (LI) protocols as a function of the number of processors, network characteristics, and application types. The DISK system has been implemented and running on a network of 16 Pentium III computers interconnected by a 100Mbps Ethernet network. Experiments performed with two applications: parallel matrix multiplication and traveling salesman problem confirm the analytical model.
- Surdeanu, M., Moldovan, D. I., & Harabagiu, S. M. (2002). Performance analysis of a distributed question/answering system. IEEE Transactions on Parallel and Distributed Systems, 13(6), 579-596.More infoAbstract: The problem of question/answering (Q/A) is to find answers to open-domain questions by searching large collections of documents. Unlike information retrieval systems very common today in the form of Internet search engines, Q/A systems do not retrieve documents, but instead provide short, relevant answers located in small fragments of text. This enhanced functionality comes with a price: Q/A systems are significantly slower and require more hardware resources than information retrieval systems. This paper proposes a distributed Q/A architecture that enhances the system throughput through the exploitation of interquestion parallelism and dynamic load balancing and reduces the individual question response time through the exploitation of intraquestion parallelism. Inter and intraquestion parallelism are both exploited using several scheduling points: one before the Q/A task is started and two embedded in the Q/A task. An analytical performance model is introduced. The model analyzes both the interquestion parallelism overhead generated by the migration of questions and the intraquestion parallelism overhead generated by the partitioning of the Q/A task. The analytical model indicates that both question migration and partitioning are required for a high-performance system: Intraquestion parallelism leads to significant speedup of individual questions, but it is practical up to about 90 processors, depending on the system parameters. The exploitation of intertask parallelism provides a scalable way to improve the system throughput. The distributed Q/A system has been implemented on a network of 16 Pentium III computers. The experimental results indicate that, at high system load, the dynamic load balancing strategy proposed in this paper outperforms two other traditional approaches. At low system load, the distributed Q/A system reduces question response times through task partitioning, with factors close to the ones indicated by the analytical model.
- Surdeanu, M. (2000). Distributed Java virtual machine for message passing architectures. Proceedings - International Conference on Distributed Computing Systems, 128-135.More infoAbstract: This paper introduces a distributed shared memory Java Virtual Machine architecture. This project is targeted for any distributed message-passing architecture, and specifically for networks of workstations. The whole system is implemented in user space which offers portability and flexibility. The memory consistency is provided by one of four protocols implementing Release Consistency. The novelty of the consistency protocols presented is that access faults are avoided by replicating objects ahead-of-time where necessary. The relative performance of these protocols is evaluated for three benchmark applications. Our experimental results indicate that, in the majority of cases, update protocols outperform invalidate protocols.
Proceedings Publications
- Liang, Z., Bethard, S., & Surdeanu, M. (2021, jun). Explainable Multi-hop Verbal Reasoning Through Internal Monologue. In Proceedings of the 2021 Conference of the North American Chapter of the Association for Computational Linguistics: Human Language Technologies.More infoNAACL is an A conference in CORE.
- Liang, Z., Zhao, Y., & Surdeanu, M. (2021, Spring). Using the Hammer Only on Nails: A Hybrid Method for Evidence Retrieval for Question Answering. In Proceedings of the 43rd European Conference on Information Retrieval.More infoECIR is an A conference in CORE.
- Mithun, M. P., Suntwal, S., & Surdeanu, M. (2021). Data and Model Distillation as a Solution for Domain-transferable Fact Verification. In Proceedings of the 2021 Conference of the North American Chapter of the Association for Computational Linguistics: Human Language Technologies.More infoNAACL is an A conference in CORE.
- Mithun, M. P., Suntwal, S., & Surdeanu, M. (2021). Students Who Study Together Learn Better: On the Importance of Collective Knowledge Distillation for Domain Transfer in Fact Verification. In Proceedings of the 2021 Conference on Empirical Methods in Natural Language Processing.More infoEMNLP is an A conference in CORE.
- Tang, Z., & Surdeanu, M. (2021). Interpretability Rules: Jointly Bootstrapping a Neural Relation Extractor with an Explanation Decoder. In Proceedings of the 2021 Conference of the North American Chapter of the Association for Computational Linguistics: Human Language Technologies: TrustNLP Workshop.More infoThis workshop is associated with NAACL, which is an A conference in CORE.
- Van, H., Tang, Z., & Surdeanu, M. (2021, nov). How May I Help You? Using Neural Text Simplification to Improve Downstream NLP Tasks. In Findings of the Association for Computational Linguistics: EMNLP 2021.More infoEMNLP is an A conference in CORE. "Findings of EMNLP" is an extended proceedings for this conference (which grew considerably in the last few years). However, the acceptance rate for Findings was lower than the main proceedings (12% vs 20+%).
- Van, H., Yadav, V., & Surdeanu, M. (2021). Cheap and good? simple and effective data augmentation for low resource machine reading. In Proceedings of the 44th International ACM SIGIR Conference on Research and Development in Information Retrieval.More infoSIGIR is an A* conference in CORE.
- Yadav, V., Bethard, S., & Surdeanu, M. (2021, jun). If You Want to Go Far Go Together: Unsupervised Joint Candidate Evidence Retrieval for Multi-hop Question Answering. In Proceedings of the 2021 Conference of the North American Chapter of the Association for Computational Linguistics: Human Language Technologies.
- Liang, Z., & Surdeanu, M. (2020, Fall). Do Transformers Dream of Inference, or Can Pretrained Generative Models Learn Implicit Inferential Rules?. In Proceedings of the 2020 Conference on Empirical Methods in Natural Language Processing (EMNLP), Workshop on Insights from Negative Results in NLP.More infoThis is a workshop associated with an A conference in CORE.
- Tang, Z., Hahn-Powell, G., & Surdeanu, M. (2020, jul). Exploring Interpretability in Event Extraction: Multitask Learning of a Neural Event Classifier and an Explanation Decoder. In Proceedings of the 58th Annual Meeting of the Association for Computational Linguistics: Student Research Workshop.More infoThis is a student research workshop associated with an A* conference in CORE.
- Vacareanu, R., Barbosa, G., Valenzuela-Escarcega, M. A., & Surdeanu, M. (2020, Summer). Parsing as Tagging. In Proceedings of the 12th International Conference on Language Resources and Evaluation (LREC).More infoLREC is a C conference in CORE.
- Vacareanu, R., Valenzuela-Escarcega, M. A., Sharp, R., & Surdeanu, M. (2020, Fall). An Unsupervised Method for Learning Representations of Multi-word Expressions for Semantic Classification. In The 28th International Conference on Computational Linguistics in Barcelona (COLING 2020).More infoCOLING is an A conference in CORE.
- Yadav, V., Bethard, S., & Surdeanu, M. (2020, Summer). Having Your Cake and Eating it Too: Training Neural Retrieval for Language Inference without Losing Lexical Match. In Proceedings of the 43rd International ACM SIGIR Conference on Research and Development in Information Retrieval.More infoSIGIR is an A* conference in CORE.
- Yadav, V., Bethard, S., & Surdeanu, M. (2020, jul). Unsupervised Alignment-based Iterative Evidence Retrieval for Multi-hop Question Answering. In Proceedings of the 58th Annual Meeting of the Association for Computational Linguistics.More infoACL is an A* conference in CORE.
- Zupon, A., Rafique, F., & Surdeanu, M. (2020, Fall). An Analysis of Capsule Networks for Part of Speech Tagging in High- and Low-resource Scenarios. In Proceedings of the 2020 Conference on Empirical Methods in Natural Language Processing (EMNLP), Workshop on Insights from Negative Results in NLP.More infoThis is a workshop associated with an A conference in CORE.
- "Nagesh, A., & Surdeanu, M. (2019). "An Exploration of Three Lightly-supervised Representation Learning Approaches for Named Entity Classification". In "Proceedings of the 27th International Conference on Computational Linguistics".
- Barbosa, G. C., Wong, Z., Hahn-Powell, G., Bell, D., Sharp, R., Valenzuela-Escarcega, M. A., & Surdeanu, M. (2019, Summer). Enabling Search and Collaborative Assembly of Causal Interactions Extracted from Multilingual and Multi-domain Free Text. In Proceedings of the Conference of the North American Chapter of the Association for Computational Linguistics - Human Language Technologies (NAACL HLT): Software Demonstrations.More infoThis conference is ranked A in CORE.This paper won the best system demonstration paper.
- Luo, F., Nagesh, A., Sharp, R., & Surdeanu, M. (2019). Semi-Supervised Teacher-Student Architecture for Relation Extraction. In Proceedings of the 3rd Workshop on Structured Prediction for Natural Language Processing.
- Narayan, P. L., Nagesh, A., & Surdeanu, M. (2019). Exploration of Noise Strategies in Semi-supervised Named Entity Classification. In Proceedings of the Eighth Joint Conference on Lexical and Computational Semantics (* SEM 2019).
- Noriega-Atala, E., Liang, Z., Bachman, J. A., Morrison, C. T., & Surdeanu, M. (2019). Understanding the Polarity of Events in the Biomedical Literature: Deep Learning vs. Linguistically-informed Methods. In Proceedings of the Workshop on Extracting Structured Knowledge from Scientific Publications.
- Sharp, R., Pyarelal, A., Gyori, B., Alcock, K., Laparra, E., Valenzuela-Escarcega, M. A., Nagesh, A., Yadav, V., Bachman, J., Tang, Z., Lent, H., Luo, F., Paul, M., Bethard, S., Barnard, K., Morrison, C., & Surdeanu, M. (2019, 6). Eidos, INDRA, \& Delphi: From Free Text to Executable Causal Models. In Proceedings of the 2019 Conference of the North American Chapter of the Association for Computational Linguistics (Demonstrations).More infoThis conference is ranked A in CORE.
- Suntwal, S., Paul, M., Sharp, R., & Surdeanu, M. (2019, November). On the Importance of Delexicalization for Fact Verification. In Proceedings of the 2019 Conference on Empirical Methods in Natural Language Processing and the 9th International Joint Conference on Natural Language Processing, (Short Papers).More infoThis conference is ranked A in CORE.
- Van, H., Musa, A., Chen, H., Surdeanu, M., & Kobourov, S. (2019). What does the language of foods say about us?. In Tenth International Workshop on Health Text Mining and Information Analysis (LOUHI).
- Yadav, V., Bethard, S., & Surdeanu, M. (2019, 6). Alignment over Heterogeneous Embeddings for Question Answering. In Proceedings of the 2019 Conference of the North American Chapter of the Association for Computational Linguistics: Human Language Technologies, Volume 1 (Long and Short Papers).More infoThis conference is ranked A in CORE.
- Yadav, V., Bethard, S., & Surdeanu, M. (2019, November). Quick and (not so) Dirty: Unsupervised Selection of Justification Sentences for Multi-hop Question Answering. In Proceedings of the 2019 Conference on Empirical Methods in Natural Language Processing and the 9th International Joint Conference on Natural Language Processing, (Long Papers).More infoThis conference is ranked A in CORE.
- Yadav, V., Laparra, E., Wang, T., Surdeanu, M., & Bethard, S. (2019, 6). University of Arizona at SemEval-2019 Task 12: Deep-Affix Named Entity Recognition of Geolocation Entities. In Proceedings of the 13th International Workshop on Semantic Evaluation.
- Zupon, A., Alexeeva, M., Valenzuela-Escarcega, M. A., Nagesh, A., & Surdeanu, M. (2019). Lightly Supervised Representation Learning with Global Interpretability. In Proceedings of the 3rd Workshop on Structured Prediction for Natural Language Processing.
- Berger, M., Nagesh, A., Levine, J. A., Surdeanu, M., & Zhang, H. H. (2018, Fall). Visual Supervision in Bootstrapped Information Extraction. In Proceedings of the Conference on Empirical Methods in Natural Language Processing (EMNLP).More infoThis conference has an A rank in CORE.
- Ebrahimi, M., Surdeanu, M., Samtani, S., & Chen, H. (2018, Fall). Detecting Cyber Threats in Non-English Dark Net Markets: A Cross-Lingual Transfer Learning Approach. In Proceedings of the IEEE Intelligence and Security Informatics Conference (ISI).More infoThis conference has a C rank in CORE.
- Forbes, A. G., Lee, K., Hahn-Powell, G., Valenzuela-Escarcega, M. A., & Surdeanu, M. (2018, May). Text Annotation Graphs: Annotating Complex Natural Language Phenomena. In Proceedings of the Eleventh International Conference on Language Resources and Evaluation (LREC'18).More infoThis conference has a C rank in CORE.
- Gniady, C., Surdeanu, M., & Gaska, B. (2018, 12). MLStar: Machine Learning in Energy Profile Estimation of Android Apps. In MobiQuitous.More infoThis is ranked in category Systems on CSRankingsThis conference is ranked A in CORE
- Kwon, H., Trivedi, H., Jansen, P., Surdeanu, M., & Balasubramanian, N. (2018, Spring). Controlling Information Aggregation for Complex Question Answering. In Proceedings of the 40th European Conference on Information Retrieval (ECIR).More infoThis conference has an A rank in CORE.
- Luo, F., Valenzuela-Escarcega, M. A., Hahn-Powell, G., & Surdeanu, M. (2018, June). Scientific Discovery as Link Prediction in Influence and Citation Graphs. In TextGraphs: 12th Workshop on Graph-Based Natural Language Processing.More infoThis workshop is associated with a conference ranked A by CORE.
- Nagesh, A., & Surdeanu, M. (2018, June). Keep your bearings: Lightly-supervised Information Extraction with Ladder Networks that avoids Semantic Drift. In NAACL HLT 2018, The 16th Annual Conference of the North American Chapter of the Association for Computational Linguistics: Human Language Technologies, New Orleans, Louisiana, USA, Jun 1 - June 6, 2018.More infoThis conference has an A rank in CORE.
- Sharp, R., Paul, M., Nagesh, A., Bell, D., & Surdeanu, M. (2018, may). Grounding Gradable Adjectives through Crowdsourcing. In Proceedings of the Eleventh International Conference on Language Resources and Evaluation (LREC 2018).More infoThis conference has a C rank in CORE.
- Yadav, V., Sharp, R., & Surdeanu, M. (2018, Spring). Sanity Check: A Strong Alignment and Information Retrieval Baseline for Question Answering. In The 41st International ACM SIGIR Conference on Research & Development in Information Retrieval.More infoThis conference has an A* rank in CORE.
- Noriega-Atala, E., Valenzuela-Escarcega, M. A., Morrison, C. T., & Surdeanu, M. (2017, NA). Focused Reading: Reinforcement Learning for What Documents to Read. In Proceedings of the Interactive Machine Learning and Semantic Information Retrieval Workshop at ICML, 2017.More infoThis is a workshop at a conference ranked A* in CORE.
- Noriega-Atala, E., Valenzuela-Escarcega, M. A., Morrison, C., & Surdeanu, M. (2017, June). Learning what to read: Focused machine reading. In Proceedings of the 2017 Conference on Empirical Methods in Natural Language Processing.More infoThis conference is ranked A in CORE.
- Sharp, R., Surdeanu, M., Jansen, P., Valenzuela-Escarcega, M. A., Clark, P., & Hammond, M. (2017, June). Tell Me Why: Using Question Answering as Distant Supervision for Answer Justification. In Proceedings of the 21st Conference on Computational Natural Language Learning (CoNLL 2017).More infoThis conference is ranked A in CORE
- Valenzuela-Escarcega, M. A., Babur, O., Hahn-Powell, G., Bell, D., Hicks, T., Noriega-Atala, E., Wang, X., Surdeanu, M., Demir, E., & Morrison, C. T. (2017, NA). Large-scale automated reading with Reach discovers new cancer driving mechanisms. In Proceedings of the Sixth BioCreative Challenge Evaluation Workshop.More infoThis workshop is not listed in CORE or CSRankings
- Bell, D., Freed, D., Huangfu, L., Surdeanu, M., & Kobourov, S. G. (2016, May). Towards Using Social Media to Identify Individuals at Risk for Preventable Chronic Illness. In 10th International Conference on Language Resources and Evaluation (LREC).
- Hahn-Powell, G., Bell, D., Valenzuela-Escarcega, M. A., & Surdeanu, M. (2016, Spring). This before That: Causal Precedence in the Biomedical Domain. In Proceedings of the 2016 Workshop on Biomedical Natural Language Processing (BioNLP 2016).More infoThis is a workshop; but it is a competitive top-tier venue for bio NLP work.
- Jansen, P., Balasubramanian, N., Surdeanu, M., & Clark, P. (2016, Fall). What's in an Explanation? Characterizing Knowledge and Inference Requirements for Elementary Science Exams. In Proceedings of the 26th International Conference on Computational Linguistics (COLING).More infoTop-tier NLP conference
- Sharp, R., Surdeanu, M., Jansen, P., Clark, P., & Hammond, M. (2016, Fall). Creating Causal Embeddings for Question Answering with Minimal Supervision. In Proceedings of the Conference on Empirical Methods in Natural Language Processing (EMNLP).More infoTop-tier NLP conference
- Surdeanu, M., Bell, D., Fried, D., Huangfu, L., & Kobourov, S. (2016, Spring). Towards Using Social Media to Identify Individuals at Risk for Preventable Chronic Illness. In Language Resources and Evaluation Conference.More infoSecond-tier NLP conference
- Surdeanu, M., Bell, D., Valenzuela, M., & Hahn-Powell, G. (2016, Spring). An Investigation of Coreference Phenomena in the Biomedical Domain. In Language Resources and Evaluation Conference.More infoSecond-tier NLP conference
- Surdeanu, M., Valenzuela, M., & Hahn-Powell, G. (2016, Spring). Odin’s Runes: A Rule Language for Information Extraction. In Language Resources and Evaluation Conference.More infoSecond-tier NLP conference
- Valenzuela-Escarcega, M. A., Hahn-Powell, G., Bell, D., & Surdeanu, M. (2016, Spring). SnapToGrid: From Statistical to Interpretable Models for Biomedical Information Extraction. In Proceedings of the 2016 Workshop on Biomedical Natural Language Processing (BioNLP 2016).More infoThis is a workshop; but it is a competitive top-tier venue for bio NLP work.
- Intxaurrondo, A., Agirre, E., Lacalle, O. L., & Surdeanu, M. (2015, Summer). Diamonds in the Rough: Event Extraction from Imperfect Microblog Data. In Proceedings of the Conference of the North American Chapter of the Association for Computational Linguistics - Human Language Technologies (NAACL HLT).
- Sharp, R., Jansen, P., Surdeanu, M., & Clark, P. (2015, Summer). Spinning Straw into Gold: Using Free Text to Train Monolingual Alignment Models for Non-factoid Question Answering. In Proceedings of the Conference of the North American Chapter of the Association for Computational Linguistics - Human Language Technologies (NAACL HLT).
- Surdeanu, M., Hicks, T., & Valenzuela-Esc\'{a}rcega, M. A. (2015, Summer). Two Practical Rhetorical Structure Theory Parsers. In Proceedings of the Conference of the North American Chapter of the Association for Computational Linguistics - Human Language Technologies (NAACL HLT): Software Demonstrations.
- Valenzuela-Esc\'{a}rcega, M. A., Hahn-Powell, G., Hicks, T., & Surdeanu, M. (2015, Summer). A Domain-independent Rule-based Framework for Event Extraction. In Proceedings of the 53rd Annual Meeting of the Association for Computational Linguistics and the 7th International Joint Conference on Natural Language Processing of the Assian Federation of Natural Language Processing: Software Demonstrations (ACL-IJCNLP).
- Fried, D., Surdeanu, M., Kobourov, S., Hingle, M., & Bell, D. (2014, Fall). Analyzing the Language of Food on Social Media. In Proceedings of the 2014 IEEE International Conference on Big Data.
- Jansen, P., Surdeanu, M., & Clark, P. (2014, Summer). Discourse Complements Lexical Semantics for Non-factoid Answer Reranking. In Proceedings of the 52nd Annual Meeting of the Association for Computational Linguistics (ACL).
- Lee, H., MacCartney, B., Surdeanu, M., & Jurafsky, D. (2014, Summer). On the Importance of Text Analysis for Stock Price Prediction. In Proceedings of the 9th edition of the Language Resources and Evaluation Conference (LREC).
- Manning, C. D., Surdeanu, M., Bauer, J., Finkel, J., Bethard, S. J., & McClosky, D. (2014, Summer). The Stanford CoreNLP Natural Language Processing Toolkit. In Proceedings of the 52nd Annual Meeting of the Association for Computational Linguistics (ACL).
- Reschke, K., Jankowiak, M., Surdeanu, M., Manning, C. D., & Jurafsky, D. (2014, Summer). Event Extraction Using Distant Supervision. In Proceedings of the 9th edition of the Language Resources and Evaluation Conference (LREC).
- Tran, A., Surdeanu, M., & Cohen, P. (2014, Fall). Extracting Latent Attributes from Video Scenes Using Text as Background Knowledge. In Proceedings of the Third Joint Conference on Lexical and Computational Semantics (*SEM).
- Surdeanu, M. (2013, May/Spring). Overview of the TAC2013 Knowledge Base Population Evaluation: English Slot Filling and Temporal Slot Filling. In Proceedings of the TAC-KBP 2013 Workshop.
- Surdeanu, M., & Heng, J. (2013). Overview of the English Slot Filling Track at the TAC2014 Knowledge Base Population Evaluation. In Proceedings of the TAC-KBP 2014 Workshop.
- Surdeanu, M., Dawson, C., Del Pero, L., Morrison, C., Hahn-Powell, G., Chapman, Z., & Barnard, K. (2013, June/Summer). Bayesian modeling of scenes and captions.. In 2013 Conference of the North American Chapter of the Association for Computational Linguistics: Human Language Technologies (NAACL-HLT 2013), Workshop on Vision and Language (WVL).
- Surdeanu, M., Forbes, A., Carrington, J., & Jansen, P. (2013, Fall). Transmitting Narrative: An Interactive Shift-Summarization Tool for Improving Nurse Communication. In 3rd IEEE Workshop on Interactive Visual Text Analytics.