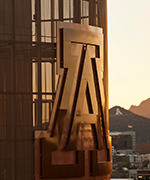
Thomas A.R. Purcell
- Assistant Professor, Chemistry and Biochemistry-Sci
- Member of the Graduate Faculty
- Assistant Professor, Applied Mathematics - GIDP
Contact
- (520) 626-6194
- Chemical Sciences Building, Rm. 214
- Tucson, AZ 85721
- purcellt@arizona.edu
Bio
No activities entered.
Interests
No activities entered.
Courses
2025-26 Courses
-
Exchange Chemical Info
CHEM 695B (Fall 2025) -
Intro To Quantum Chem
CHEM 580 (Fall 2025) -
Intro to Quantum
CHEM 480 (Fall 2025) -
Research
CHEM 900 (Fall 2025) -
Thesis
CHEM 910 (Fall 2025)
2024-25 Courses
-
Directed Research
CHEM 392 (Spring 2025) -
Exchange Chemical Info
CHEM 695B (Spring 2025) -
Research
CHEM 900 (Spring 2025) -
Intro To Quantum Chem
CHEM 580 (Fall 2024) -
Intro to Quantum
CHEM 480 (Fall 2024) -
Research
CHEM 900 (Fall 2024)
2023-24 Courses
-
Computational Chemistry
CHEM 418 (Spring 2024) -
Computational Chemistry
CHEM 518 (Spring 2024)
Scholarly Contributions
Journals/Publications
- Scheffler, M., Bauer, S., Benner, P., Bereau, T., Blum, V., Boley, M., Carbogno, C., Catlow, C. R., Dehm, G., Eibl, S., Ernstorfer, R., Fekete, Á., Foppa, L., Fratzl, P., Freysoldt, C., Gault, B., Ghiringhelli, L. M., Giri, S. K., Gladyshev, A., , Goyal, P., et al. (2024). Roadmap on Data-Centric Materials Science. IOP Science home Accessibility Help Modelling and Simulation in Materials Science and Engineering, 32, 063301. doi:10.1088/1361-651X/ad4d0dMore infoLarge collaboration paper for the BigMax Consertium.
- Shaban Tameh, M., Coropceanu, V., Purcell, T., & Brédas, J. (2024). Prediction of the Infrared Absorbance Intensities and Frequencies of Hydrocarbons: A Message Passing Neural Network Approach. Journal of Physical Chemistry A, 128(44). doi:10.1021/acs.jpca.4c06745More infoAccurately and efficiently predicting the infrared (IR) spectra of a molecule can provide insights into the structure-properties relationships of molecular species, which has led to a proliferation of machine learning tools designed for this purpose. However, earlier studies have focused primarily on obtaining normalized IR spectra, which limits their potential for a comprehensive analysis of molecular behavior in the IR range. For instance, to fully understand and predict the optical properties, such as the transparency characteristics, it is necessary to predict the molar absorptivity IR spectra instead. Here, we propose a graph-based communicative message passing neural network algorithm that can predict both the peak positions and absolute intensities corresponding to density functional theory calculated molar absorptivities in the IR domain. By modifying existing spectral loss functions, we show that our method is able to predict with DFT-accuracy level the IR molar absorptivities of a series of hydrocarbons containing up to ten carbon atoms and apply the model to a set of larger molecules. We also compare the predicted spectra with those generated by the direct message passing neural network. The results suggest that both algorithms demonstrate similar predictive capabilities for hydrocarbons, indicating that either model could be effectively used in future research on spectral prediction for such systems.
- Dean, J., Scheffler, M., Purcell, T., Barabash, S., Bhowmik, R., & Bazhirov, T. (2023). Interpretable machine learning for materials design. Journal of Materials Research, 38(20). doi:10.1557/s43578-023-01164-wMore infoFueled by the widespread adoption of machine learning and the high-throughput screening of materials, the data-centric approach to materials design has asserted itself as a robust and powerful tool for the in silico prediction of materials properties. When training models to predict material properties, researchers often face a difficult choice between a model’s interpretability and performance. We study this trade-off by leveraging four different state-of-the-art machine learning techniques: XGBoost, SISSO, Roost, and TPOT for the prediction of structural and electronic properties of perovskites and 2D materials. We then assess the future outlook of the continued integration of machine learning into materials discovery and identify the key problems that will continue to challenge researchers as the size of the literature’s datasets and complexity of models increases. Finally, we offer several possible solutions to these challenges with a focus on retaining interpretability and share our thoughts on magnifying the impact of machine learning on materials design. Graphical abstract: [Figure not available: see fulltext.].
- Knoop, F., Purcell, T., Scheffler, M., & Carbogno, C. (2023). Anharmonicity in Thermal Insulators: An Analysis from First Principles. Physical Review Letters, 130(23). doi:10.1103/PhysRevLett.130.236301More infoThe anharmonicity of atomic motion limits the thermal conductivity in crystalline solids. However, a microscopic understanding of the mechanisms active in strong thermal insulators is lacking. In this Letter, we classify 465 experimentally known materials with respect to their anharmonicity and perform fully anharmonic ab initio Green-Kubo calculations for 58 of them, finding 28 thermal insulators with κ
- Purcell, T., Scheffler, M., & Ghiringhelli, L. (2023). Recent advances in the SISSO method and their implementation in the SISSO++ code. Journal of Chemical Physics, 159(11). doi:10.1063/5.0156620More infoAccurate and explainable artificial-intelligence (AI) models are promising tools for accelerating the discovery of new materials. Recently, symbolic regression has become an increasingly popular tool for explainable AI because it yields models that are relatively simple analytical descriptions of target properties. Due to its deterministic nature, the sure-independence screening and sparsifying operator (SISSO) method is a particularly promising approach for this application. Here, we describe the new advancements of the SISSO algorithm, as implemented into SISSO++, a C++ code with Python bindings. We introduce a new representation of the mathematical expressions found by SISSO. This is a first step toward introducing “grammar” rules into the feature creation step. Importantly, by introducing a controlled nonlinear optimization to the feature creation step, we expand the range of possible descriptors found by the methodology. Finally, we introduce refinements to the solver algorithms for both regression and classification, which drastically increase the reliability and efficiency of SISSO. For all these improvements to the basic SISSO algorithm, we not only illustrate their potential impact but also fully detail how they operate both mathematically and computationally.
- Purcell, T., Scheffler, M., Ghiringhelli, L., & Carbogno, C. (2023). Accelerating materials-space exploration for thermal insulators by mapping materials properties via artificial intelligence. npj Computational Materials, 9(1). doi:10.1038/s41524-023-01063-yMore infoReliable artificial-intelligence models have the potential to accelerate the discovery of materials with optimal properties for various applications, including superconductivity, catalysis, and thermoelectricity. Advancements in this field are often hindered by the scarcity and quality of available data and the significant effort required to acquire new data. For such applications, reliable surrogate models that help guide materials space exploration using easily accessible materials properties are urgently needed. Here, we present a general, data-driven framework that provides quantitative predictions as well as qualitative rules for steering data creation for all datasets via a combination of symbolic regression and sensitivity analysis. We demonstrate the power of the framework by generating an accurate analytic model for the lattice thermal conductivity using only 75 experimentally measured values. By extracting the most influential material properties from this model, we are then able to hierarchically screen 732 materials and find 80 ultra-insulating materials.
- Foppa, L., Purcell, T., Levchenko, S., Scheffler, M., & Ghringhelli, L. (2022). Hierarchical Symbolic Regression for Identifying Key Physical Parameters Correlated with Bulk Properties of Perovskites. Physical Review Letters, 129(5). doi:10.1103/PhysRevLett.129.055301More infoSymbolic regression identifies nonlinear, analytical expressions relating materials properties and key physical parameters. However, the pool of expressions grows rapidly with complexity, compromising its efficiency. We tackle this challenge hierarchically: identified expressions are used as inputs for further obtaining more complex expressions. Crucially, this framework can transfer knowledge among properties, as demonstrated using the sure-independence-screening-and-sparsifying-operator approach to identify expressions for lattice constant and cohesive energy, which are then used to model the bulk modulus of ABO3 perovskites.