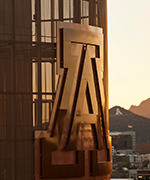
Shang Gao
- Assistant Professor, Watershed Management and Ecohydrology
- Member of the Graduate Faculty
Contact
- (520) 621-6360
- Environment and Natural Res. 2, Rm. N254
- Tucson, AZ 85719
- shanggao@arizona.edu
Bio
No activities entered.
Interests
No activities entered.
Courses
2025-26 Courses
-
Sustainable Earth
RNR 150C1 (Fall 2025)
2024-25 Courses
-
Independent Study
RNR 599 (Spring 2025) -
Watershed Management
WSM 462 (Spring 2025) -
Watershed Management
WSM 562 (Spring 2025) -
Dissertation
RNR 920 (Fall 2024) -
Hydrology for Water Resources
HWRS 573 (Fall 2024) -
Renewable Nat Resources
RNR 696A (Fall 2024)
2023-24 Courses
-
Watershed Management
WSM 462 (Spring 2024) -
Watershed Management
WSM 562 (Spring 2024)
Scholarly Contributions
Chapters
- Chen, M., Li, Z., & Gao, S. (2022). Multisensor Remote Sensing and the Multidimensional Modeling of Extreme Flood Events: A Case Study of Hurricane Harvey–Triggered Floods in Houston, Texas, USA. In Remote Sensing of Water-Related Hazards. doi:10.1002/9781119159131.ch5More infoClimate change has increased the magnitude and frequency of extreme storm events. Using Hurricane Harvey as a case study event, this study investigates the detectability of extreme rainfall by multisensor remote sensing and simulation performance of induced flooding via multidimensional modeling. A suite of radar and satellite precipitation products, including the Multi-Radar Multi-Sensor (MRMS) and Integrated Multisatellite Retrievals for GPM (IMERG), are statistically evaluated using both the gauge references and the triple collocation method. Driven by remotely sensed precipitation, multidimensional modeling is enabled by the Coupled Routing and Excess Storage (CREST) model family. Comprehensive simulations of floods induced by Hurricane Harvey are then conducted and evaluated in terms of stream flow, flood extent, and flood depth. The results show that remote sensing products estimate precipitation reasonably well and are more reliable than conventional gauge products during extreme events. Also, near-real-time precipitation products are accurate enough in extreme events like Hurricane Harvey and suitable for real-time flood early warning applications. Lastly, the multidimensional modeling enabled by CREST can well capture the pluvial and fluvial floods from extreme events. Overall, the integration of multisensor remote sensing precipitation and multidimensional modelling promises to provide accurate and timely flood warning in extreme events under a changing climate.
- Compson, Z., Monk, W., Sarremejane, R., DelVecchia, A., Burrows, R., Gao, S., Ruddell, B., Hong, Y., & Allen, D. (2022). Dryland Rivers and Streams. In Encyclopedia of Inland Waters, 2nd edition. doi:10.1016/B978-0-12-819166-8.00156-0More infoWe outline key features of dryland rivers and illustrate how they differ from temperate and tropical rivers, identify gaps in the understanding of dryland rivers, and illustrate how the functioning of these systems is expected to vary under human impacts. The main concepts we cover are (1) defining dryland rivers and distinguishing them from temperate and tropical rivers, (2) exploring the geomorphological, hydrological, and biological processes of dryland rivers, (3) illustrating how dryland rivers fit into the context of riparian forests and how the aquatic and terrestrial components of these systems interact, and (4) detailing how the functioning of dryland rivers is expected to change in the context of anthropogenic drivers, including climate change. We demonstrate key features of dryland rivers, including their high hydrological and geomorphological variation, how the biotic components of these systems are adapted to this variability, and how anthropogenic changes are likely to impact the functioning and aquatic-terrestrial linkages characteristic of these ecosystems. We identify knowledge gaps where research on dryland rivers is needed, particularly in arctic regions and areas experiencing intense human pressures driven by urbanization, deforestation, and agricultural development. We further highlight how common features of dryland rivers (e.g., high degree of seasonal variability, habitat fragmentation caused by disconnected flows) make them ideal systems for studying emerging ideas derived from metacommunity theory. Despite their clear ecological importance, recent political efforts to restrict what falls under the purview of the Clean Water Act by removing dryland rivers from these protections demonstrate ongoing threats to their health and persistence, especially in densely populated regions that rely on these systems for water provisioning and flood control. Bringing awareness to these systems and the key ecosystem services and functions they perform is critical for their conservation and protection.
Journals/Publications
- Gao, S., Li, Z., Graves, G., Mattes, H., Fathollahifard, S., Vogel, J., Neeson, T., Strevett, K., & Hong, Y. (2024). Hydro-modeling the ecosystem impact of wastewater reuse under climate change: A case study in upper Red River basin, Oklahoma. Journal of the American Water Resources Association, 60(4). doi:10.1111/1752-1688.13208More infoWater reuse, as a viable option for water supply, must be implemented to minimize the adverse impacts on stream ecosystems that previously received this wastewater effluent. In the State of Oklahoma (OK), USA, local communities have implemented wastewater reuse, and many seek to expand the reuse programs. This study presents a hydro-modeling analysis based on the Coupled Routing and Excess STorage with VECtor routing (CREST-VEC) model focusing on the potential ecosystem impacts and societal benefits of wastewater reuse under climate change in the OK portion of the Red River basin. First, a CREST-VEC model is established for the upper Red River basin and validated against observed streamflow for a 30-year historical period (1990–2020). Based on the established model, we then assess the sensitivity of ecosystem impact to various climate change scenarios and hypothetical wastewater reuse scenarios. Results show that dominant effects of climate change cause the annual time below environmental flow to increase in the next 30 years, which constrains the room to implement wastewater reuse. However, at sub-catchment scale, the analyses identify viable locations for allocating wastewater reuse while maintaining ecosystem health. The results also reveal that wastewater reuse brings about the most societal water benefits at minimal cost of ecosystem health under representative concentration pathway (RCP) 2.6 followed by RCP 4.5 and then RCP 8.5. Overall, the study demonstrates capabilities of the hydro-modeling framework in developing water management plans facing the changing climate.
- Jing, H., Li, Z., Wen, Y., Gao, S., Wang, Y., Qian, W., & Kisembe, J. (2024). Changes in Convective Precipitation Reflectivity over the CONUS Revealed by High-Resolution Radar Observations from 2015 to 2021. Atmosphere, 15(6). doi:10.3390/atmos15060627More infoThe change in extreme precipitation events in the conterminous United States (CONUS) has been of interest to the research communities in recent years for its intensification under environmental and climate change. Previous studies have not yet used sub-hourly precipitation observations to examine convective precipitation change over the CONUS. This study aims to fill the gap by examining convective precipitation, identified by radar reflectivity, in the CONUS using the state-of-the-art Multi-radar Multi-sensor data, operated at the NOAA/National Severe Storms Laboratory, with an unprecedentedly high spatial (1 km) and temporal (2 min) resolutions. These high-resolution data are expected to better capture the precipitation peak and the precipitation pattern. The results showed that in CONUS, precipitation reflectivity increased both in magnitude and the number of convective days from 2015 to 2021. For example, in 2019, 60% of areas showed an increase in the magnitude of precipitation, and the average number of convective days over CONUS has increased by 19%. Changes in precipitation also vary by season and region. This study highlights the need for continued monitoring and understanding of the evolving pattern of extreme precipitation in the CONUS, especially at sub-hourly frequency, as it exposes significant impacts on various sectors, including agriculture, infrastructure, and human health.
- Li, Z., Tsoodle, T., Chen, M., Gao, S., Zhang, J., Wen, Y., Yang, T., King, F., & Hong, Y. (2024). Future Heavy Rainfall and Flood Risks for Native Americans under Climate and Demographic Changes: A Case Study in Oklahoma. Weather, Climate, and Society, 16(1). doi:10.1175/WCAS-D-23-0005.1More infoClimate change has posed inequitable risks to different communities. Among communities of color in the United States, Native Americans stand out because 1) they desire resources to sustain resilient nations and 2) they have developed nature-based solutions through experiences with local climate-related challenges, which can provide deep insight for the whole society. Projection of climate risks for Native Americans is essential to assess future risks and support their climateready nations, yet there has been lack of useable information. In this study, we projected three climate hazards__heavy rainfall, 2-yr floods, and flash floods__for tribal nations in Oklahoma. To break down into tribal jurisdictions, we utilize a coupled regional climate model at 4 km and flash-flood forecast model at 1 km. A hazard–exposure–vulnerability risk framework is applied to integrate both climate and demographic changes in a high-emissions scenario. It is found that 1) Indigenous people are the most vulnerable community in Oklahoma; 2) heavy rainfall and 2-yr floods have marked increases in risks at 501.1% and 632.6%, respectively, while flash floods have a moderate increase (296.4%); 3) Native Americans bear 68.0%, 64.3%, and 64.0% higher risks in heavy rainfall, 2-yr flooding, and flash flooding, respectively, than the general population in Oklahoma; 4) in comparing climate and demographic changes, it is seen that population growth leads to greater climate hazard risks than does climate change; and 5) emerging tribal nations are projected to have 10 times as much population, resulting in great exposures to climate extremes. This study can raise awareness of the impact of climate changes and draw attention to address climate injustice issues for minoritized communities.
- Malish, M., Gao, S., Allen, D., & Neeson, T. (2024). Impacts of stream drying depend on stream network size and location of drying. Ecological Applications, 34(6). doi:10.1002/eap.3015More infoStream drying is increasing globally, with widespread impacts on stream ecosystems. Here, we investigated how the impacts of drying on stream ecosystem connectivity might depend on stream network size and the location of drying within the stream network. Using 11 stream networks from across the United States, we simulated drying scenarios in which we varied the location and spatial extent of drying. We found that the rate of connectivity loss varied with stream network size, such that larger stream networks lost connectivity more rapidly than smaller stream networks. We also found that the rate of connectivity loss varied with the location of drying. When drying occurred in the mainstem, even small amounts of drying resulted in rapid losses in ecosystem connectivity. When drying occurred in headwater reaches, small amounts of drying had little impact on connectivity. Beyond a certain threshold, however, connectivity declined rapidly with further increases in drying. Given the increasing stream drying worldwide, our findings underscore the need for managers to be particularly vigilant about fragmentation when managing at large spatial scales and when stream drying occurs in mainstem reaches.
- Zhang, L., Gao, S., & Yang, T. (2024). Adapting subseasonal-to-seasonal (S2S) precipitation forecast at watersheds for hydrologic ensemble streamflow forecasting with a machine learning-based post-processing approach. Journal of Hydrology, 631. doi:10.1016/j.jhydrol.2024.130643More infoAccurate and reliable precipitation predictions made by dynamical forecast models could provide crucial information for human socioeconomic activities by enabling hydrologic forecasts at the Subseasonal-to-Seasonal (S2S) timescale. To utilize available S2S precipitation predictions for hydrologic forecasts, post-processing techniques have been applied to adapt the raw S2S precipitation to local watersheds. However, conventional statistical-based post-processing techniques are more focused on correcting the forecast bias, but rather limited in improving the predictive skill of available S2S precipitation forecasts. In this study, we combine the Random Forest classifiers (RF) with the Bias Correction and Spatial Disaggregation (BCSD) to adapt the 10-member ensemble precipitation forecast from the NASA Goddard Earth Observing System model version 5 (GEOS5) at 4 watersheds located in the NCEI South climate region of the United States. The adapted S2S precipitation is further applied for streamflow forecast by forcing a classical lumped hydrologic model. The performance of S2S precipitation as well as the corresponding streamflow predictions are benchmarked with the randomly resampled precipitation and the corresponding Ensemble Streamflow Prediction (ESP) framework-generated streamflow predictions. Evaluation statistics of Kling-Gupta Efficiency (KGE), Continuous Ranked Probability Skill Score (CRPSS), Reliability, Resolution, and Sharpness are employed to evaluate the predictive skill of precipitation and streamflow both deterministically and probabilistically. Our results indicate that dynamical S2S precipitation after forecast adaptation leads to consistently higher deterministic skill over ESP at all forecast lead times and across study watersheds. However, at longer forecast lead times beyond 10–15 days, S2S precipitation with a limited ensemble size does not present higher probabilistic skill than ESP. Our results shows that the joint application of RF and BCSD improves the predictive skill of the raw S2S precipitation at study watersheds in contrast to BCSD. Further, the added predictive skill of S2S precipitation brought by RF propagates into streamflow predictions, predominantly at longer forecast lead times exceeding 10 days. Overall, our results highlight the potential success of future work to apply other data-driven approaches to adapt the raw precipitation to local watersheds for more accurate and reliable streamflow forecasts at the S2S timescale.
- "Li, Z., Gao, S., Chen, M., Zhang, J., Gourley, J. J., Wen, Y., Yang, T., & Hong, Y. (2023). "Introducing flashiness‐intensity‐duration‐frequency (F‐IDF): A new metric to quantify flash flood intensity". "Geophys. Res. Lett.", 50(23).
- Chen, M., Li, Z., Vergara, H., Gourley, J., Xue, M., Hong, Y., Hu, X., Mayol Novoa, H., Martin, E., McPherson, R., Gao, S., Vitaliano Perez, A., & Yanqui Morales, I. (2023). Conus-wide model calibration and validation for CRESTv3.0 – An improved Coupled Routing and Excess STorage distributed hydrological model. Journal of Hydrology, 626. doi:10.1016/j.jhydrol.2023.130333More infoThe Coupled Routing and Excess Storage (CREST) is a flood-centric distributed hydrological model that has been widely used by researchers, educators, and decision-makers around the world since 2011. With growing public concern about the impact of climate change on water security, hydrological models such as CREST need to continue improving to provide more accurate simulations, more output products, and reduced application difficulties for users. In this study, an improved version of the CREST model (version 3.0) was proposed to consider the groundwater component of the hydrological cycle. A CONUS-wide CREST model calibration was conducted to provide usable model parameters for CREST users worldwide. The calibration improved the overall NSCE score from −0.97 to 0.11 over 3206 gauge points in CONUS. The validation results indicated that the CREST model performed well in the eastern CONUS but not in the Rocky Mountains and the Mountain West. The newly added groundwater module reduced the overestimation and the steepness of the falling limb during flood events in the eastern CONUS but drastically reduced the simulated water quantities in the mountainous regions.
- Ding, M., Lin, P., Gao, S., Wang, J., Zeng, Z., Zheng, K., Zhou, X., Yamazaki, D., Gao, Y., & Liu, Y. (2023). Reversal of the levee effect towards sustainable floodplain management. Nature Sustainability, 6(12). doi:10.1038/s41893-023-01202-9More infoLevees constrain roaring floodwater but are blamed for reducing people’s perception of flood risks and promoting floodplain human settlements unprepared for high-consequence flood events. Yet the interplay between levee construction and floodplain development remains poorly quantified, obscuring an objective assessment of human–water relations. Here, to quantitatively assess how floodplain urban expansion is linked to levee construction, we develop a multiscale composite analysis framework leveraging a national levee database and decades of annual land-cover maps. We find that in the contiguous United States, levee construction is associated with a 62% acceleration in floodplain urban expansion, outpacing that of the county (29%), highlighting a clear change in risk perception after levees are built. Regions historically lacking strong momentum for population growth while experiencing frequent floods tend to rely more strongly on levees and we suggest these areas to develop a more diversified portfolio to cope with floods. Temporally, the positive levee effect is found to have weakened and then reversed since the late 1970s, reflecting the role of legislative regulations to suppress floodplain urban expansion. Our quantitative framework sheds light on how structural and non-structural measures jointly influence floodplain urban growth patterns. It also provides a viable framework to objectively assess the floodplain management strategies currently in place, which may provide useful guidance for managing flood risks towards sustainable development goals.
- Malish, M., Gao, S., Kopp, D., Hong, Y., Allen, D., & Neeson, T. (2023). Small increases in stream drying can dramatically reduce ecosystem connectivity. Ecosphere, 14(3). doi:10.1002/ecs2.4450More infoHabitat fragmentation drives biodiversity loss in rivers around the world. Although the effects of anthropogenic barriers on river connectivity are well known, there has been little research on the ways in which stream drying may alter connections among habitats and resources. Given that stream drying is increasing in many regions, there is a pressing need to understand the effects of drying on habitat fragmentation. Here, we quantify spatiotemporal drying patterns under current and future climate scenarios in the Upper Blue River Basin, Oklahoma. We used a hydrologic model to simulate daily streamflow for nine climate scenarios. For each scenario, we calculated metrics of streamflow temporal continuity (dry days, dry periods, and dry period duration) and spatial connectivity (wetted length, number of dry stream fragments, length of dry stream fragments, and dendritic connectivity index) from simulated daily streamflow. We found that stream drying is likely to increase in all future climate scenarios and that increases in stream drying reduce connectivity. However, the effects of stream drying on connectivity were highly nonlinear. Specifically, we observed a threshold around which a small increase in stream drying led to a rapid drop in connectivity. We also found that the greatest increases in stream drying were not associated with the highest emission scenarios, underscoring the complex linkages among climate, water availability, and connectivity. Given that connectivity is essential to ecosystem structure and function, we discuss water management strategies informed by impacts of stream drying.
- Zhang, J., Chen, M., Gao, S., Li, Z., Fekete, B., Miara, A., Hong, Y., & Vörösmarty, C. (2023). Examining impacts of policy, technology, and climate extremes on thermoelectric power production and river thermal pollution in the Midwest and Northeast of the United States. Frontiers in Environmental Science, 11. doi:10.3389/fenvs.2023.1212211More infoAs one of the components comprising food-energy-water systems (FEWS), the energy sector, especially electricity production, is intimately connected to water. Climate extremes-related impacts on water resources will directly affect the interdependence of water, food, and energy. A better understanding of the extent of climate impacts on energy sector and the options to improve water-energy security are needed for planning an overall resilient FEWS. Therefore, we are motivated to examine the climate stress on the thermoelectric power supply using the Water Balance Model coupled with Thermoelectric Power & Thermal Pollution Model (WBM-TP2M), which can simulate water-energy linkages at the power plant, river reach, and regional scales. Using the Midwest (MW) and Northeast (NE) regions as our study area, we design a group of single- and multi-factor experiments both for historical climate period (1980–2019) and a case where we create a series of intensified extremes (2010–2019). The results show that power generation over the two regions features a gradually increasing trend in the past four decades, while, in contrast, thermal pollution has been decreasing steadily since 2005. Heat waves created temporary dips in the generation of electricity and peaks of heavily thermal-polluted stream length. The experiments reveal the significant role of cooling towers in reducing thermal pollution without losing much capacity to generate power, one important measure of resilience against climate extremes. Constraints placed on effluent temperature from the Clean Water Act lead to interrupted operations, which reduces (up to 20%) power generation, increases sensitivity to climatic extremes, but only show a small reduction in thermal pollution. Coal, as a fuel source, is subject to low thermal efficiency and high-water demand, which results in clearly decreased power generation. Overall, our findings suggest that replacing a less thermal-efficient fuel mix and environmentally destructive once-through cooling can move the energy sector towards several beneficial outcomes. Chief among these is a more efficient power production system that uses less water and does so while fostering clean, less carbon-intense technologies (e.g., combined gas cycle turbines, cooling towers, renewable energy), thus linking positive outcomes that simultaneously and positively impact aquatic ecosystems, regional airsheds and human health.
- Zhang, J., Gao, S., & Fang, Z. (2023). Investigation of Infiltration Loss in North Central Texas by Retrieving Initial Abstraction and Constant Loss from Observed Rainfall and Runoff Events. Journal of Hydrologic Engineering, 28(5). doi:10.1061/JHYEFF.HEENG-5883More infoAccurate modeling of infiltration losses is vital for runoff estimation and thus the development of flood design/protection criteria and water management schemes, etc. In design flood practices, the initial abstraction and constant loss (IACL) method has been widely applied due to its simplicity. However, due to a lack of physical equivalent properties, the IACL method is often subject to issues in parametrization and has large dependency on calibration efforts for storm events. Despite the wide range/variability of IACL values, a single set of IA and CL values is normally adopted for specific flood frequency, which may introduce uncertainty and bias in resulting peak streamflow. In this study, we identified a total of 2,036 rainfall-runoff events for 18 watersheds in North Central Texas to estimate the total losses with their IA and CL components based on time-series of mean areal precipitation (MAP) and streamflow data. Threshold behavior is found for all studied subbasins between the summation of gross rainfall and antecedent soil moisture versus runoff depth: below the threshold, runoff depth is minimal; whereas above it, runoff is largely linearly correlated with the summation of rainfall and antecedent soil moisture. This finding provides a convenient way to estimate/predict total loss or runoff depth given MAP and antecedent soil moisture. In addition, this study shows that the IA and CL values can be approximated by the gamma and Weibull distributions, respectively. The fitted distributions of IA and CL values can be applied in a Monte Carlo simulation framework to stochastically simulate numerous rainfall-runoff events for a flood frequency analysis.
- Zhang, L., Yang, T., Gao, S., Hong, Y., Zhang, Q., Wen, X., & Cheng, C. (2023). Improving Subseasonal-to-Seasonal forecasts in predicting the occurrence of extreme precipitation events over the contiguous U.S. using machine learning models. Atmospheric Research, 281. doi:10.1016/j.atmosres.2022.106502More infoThe precipitation forecasts at the Subseasonal-to-Seasonal (S2S) scale are valuable information to assist water resources planning and decision-making at an extended range. But the raw S2S precipitation forecasts are rather limited regarding their predictive skills, which hinders further hydrological applications. Many previous studies were carried out to validate the S2S precipitation forecasts from the perspective of the ensemble “mean”, while existing study, which evaluates and improves the ability of S2S forecasts in predicting extreme precipitation events with their ensemble spreads, is rarely reported. This study aims to improve the S2S forecasts in predicting the occurrence of weekly extreme precipitation events above 99% over the contiguous United States (CONUS). The Random Forest Classifiers (RF) were employed and additional forecasts variables (i.e., surface air temperature, geopotential heights at 500 hPa and 850 hPa) were included to post-process the raw S2S forecasts from the NASA's Goddard Earth Observation System model version five (GEOS5). Different RF training inputs and RF hyperparameter sensitivity analysis are examined. We found that (1) using S2S precipitation forecast as the only inputs to RF, the forecast quality is improved significantly only at week 1; (2) When additional forecasts variables are included in RF training, the forecast skill tend to improve slightly at longer lead times after weeks 2; (3) The tunning of the maximum tree depth of RFs combine with the inclusion of additional forecasts variables as inputs to RF can improve the forecasts skill at all lead times over CONUS. In short, this study demonstrated the effectiveness of the application of RF as well as the effectiveness of additional forecast variables in improving the S2S extreme precipitation forecasts, which could be potentially useful for flood and river ensemble forecasting. Multiple statistical metrics, including the ensemble probability of detections (EPOD), ensemble false alarm ratios (EFAR), ensemble critical success index (ECSI), Brier Sill Score (BSS), and Area Under the Receiver Operating Characteristics Curves (AUROC) are employed for a comprehensive evaluation of the predictive performances of S2S extreme precipitation forecasts under different experiment scenarios.
- Chen, M., Huang, Y., Li, Z., Larico, A., Xue, M., Hong, Y., Hu, X., Novoa, H., Martin, E., McPherson, R., Zhang, J., Gao, S., Wen, Y., Perez, A., & Morales, I. (2022). Cross-Examining Precipitation Products by Rain Gauge, Remote Sensing, and WRF Simulations over a South American Region across the Pacific Coast and Andes. Atmosphere, 13(10). doi:10.3390/atmos13101666More infoPrecipitation estimate is important for earth science studies and applications, and it is one of the most difficult meteorological quantities to estimate accurately. For regions such as Peru, reliable gridded precipitation products are lacking due to complex terrains and large portions of remote lands that limit the accuracy of satellite precipitation estimation and in situ measurement density. This study evaluates and cross-examines two high-resolution satellite-based precipitation products, a global rain-gauge interpolated precipitation product, and a Weather Research and Forecast (WRF) model that simulated precipitation for a ten-year period from 2010 to 2019 in the Peruvian Andes region across the Pacific coast, Andes, and in the Amazon. The precipitation estimates examined in this study are the Integrated Multi-SatellitE Retrievals for GPM (IMERG), Multi-Source Weighted-Ensemble Precipitation (MSWEP), Global Precipitation Climatology Center product (GPCC), and a 3 km grid spacing WRF-based regional climate model (RCM) simulation. The evaluation and cross-examination were performed at sub-daily (6 h), daily, and monthly time scales, and at various spatial resolutions. The results show that the WRF simulation performs as well as, if not better than, GPM IMERG in the low precipitation and dry regions but becomes inaccurate in wet regions. GPM IMERG is more suitable for higher precipitation and wet regions, and MSWEP shows a systematic overestimation over the study area. It is therefore important to choose the most suitable precipitation product based on research needs and climate condition of the study for the challenging Peruvian Andes region.
- Chen, M., Li, Z., Gao, S., Xue, M., Gourley, J., Kolar, R., & Hong, Y. (2022). A flood predictability study for Hurricane Harvey with the CREST-iMAP model using high-resolution quantitative precipitation forecasts and U-Net deep learning precipitation nowcasts. Journal of Hydrology, 612. doi:10.1016/j.jhydrol.2022.128168More infoA flood is one of the most hazardous natural disasters, and it commonly causes fatalities and socioeconomic damages. The advances of modeling techniques and observation data in flood prediction have found success in field operations. This paper presents a comprehensive flood prediction of Hurricane Harvey in 1-hour lead-time that is not limited to 1D streamflow forecast but also 2D flood extent and 3D inundation depth. It uses high-resolution quantitative precipitation forecasts (QPFs, from operational Rapid Refresh-RAP, and High Resolution Rapid Refresh-HRRR models) and deep learning nowcasts (AI nowcasts). The results show that the QPFs have a well-known displacement issue and the AI nowcast cannot predict the precipitation intensity, and an attempt to combine the two methods (AI hybrid) failed to improve the overall accuracy. However, the 2D flood extent predictions with the HRRR and AI hybrid forcings can provide information indicating the future flooded area with about 50% accuracy (hit rate) and stream flow prediction showed that the HRRR QPF can provide relatively accurate flood peak prediction (-8%). In contrast, the AI nowcast reveals minimal displacement errors but underpredicts precipitation intensity. The deep learning method also indicates that the binary tests with low threshold, which are commonly employed in the deep learning field, neglect the importance of precipitation intensity errors for extreme event studies.
- Li, Z., Chen, M., Gao, S., Wen, Y., Gourley, J., Yang, T., Kolar, R., & Hong, Y. (2022). Can re-infiltration process be ignored for flood inundation mapping and prediction during extreme storms? A case study in Texas Gulf Coast region. Environmental Modelling and Software, 155. doi:10.1016/j.envsoft.2022.105450More infoCoupled Hydrologic & Hydraulic (H&H) models have been widely applied for flood simulations, yet the modern H&H models suffer from one-way and weak coupling and particularly disregarded run-on infiltration, which could compromise the model accuracy. In this study, we assess the H&H model performance with and without re-infiltration process in extreme flooding events. Results highlight that the re-infiltration process should not be disregarded even in extreme flood simulations. Saturated hydraulic conductivity and antecedent soil moisture are found to be the prime contributors to such differences. For the Hurricane Harvey event, the model performance is verified against stream gauges and high water marks, from which the re-infiltration scheme increases the Nash Sutcliffe Efficiency score by 140% on average and reduces maximum depth differences by 17%. Meanwhile, the recent update of the CREST-iMAP model Version 1.1, which incorporates two-way coupling and re-infiltration scheme, is released for public access.
- Li, Z., Gao, S., Chen, M., Gourley, J., & Hong, Y. (2022). Spatiotemporal Characteristics of US Floods: Current Status and Forecast Under a Future Warmer Climate. Earth's Future, 10(10). doi:10.1029/2022EF002700More infoFloods in the US exhibit strong spatiotemporal variability, mainly controlled by precipitation types and catchment attributes. In a future warmer climate, it remains largely unclear how such features change, relative to the current climate. Global Climate Models at coarse resolution cannot resolve convective-scale storms which is one of the major weather systems causing devastating floods in the US. Alternatively, coupled, high-resolution and continental-scale climate and flood simulations can advance our understanding. In this study, we couple a 4-km convection-permitting climate simulation model with a 1-km hydrologic model to simulate the spatiotemporal variability of rainfall and flooding over the contiguous US. In particular, changes in rainfall and flood frequency, spatial scale, and seasonality are explored in major climate divisions. We found: (a) an overall increase in flood frequency (+101.7%) and spatial extent (+44.9%), mainly attributed to more extreme rainfall and variability in the future; (b) weakening rainfall and flood seasonality, resulting in more random and unpredictable events throughout the year; (c) earlier flooding season onsets in the West and snow-dominated regions while delayed onsets in the East driven by drier antecedent soil moisture; (d) correlation between extreme rainfall and flood onsets is becoming stronger in the West yet weaker in the East in the future. Findings in this study can potentially serve as a basis for future flood exposure and risk assessments, as well as more scientific understanding of changing flood-generating mechanisms across the CONUS.
- Li, Z., Gao, S., Chen, M., Gourley, J., Liu, C., Prein, A., & Hong, Y. (2022). The conterminous United States are projected to become more prone to flash floods in a high-end emissions scenario. Communications Earth and Environment, 3(1). doi:10.1038/s43247-022-00409-6More infoFlash floods are largely driven by high rainfall rates in convective storms that are projected to increase in frequency and intensity in a warmer climate in the future. However, quantifying the changes in future flood flashiness is challenging due to the lack of high-resolution climate simulations. Here we use outputs from a continental convective-permitting numerical weather model at 4-km and hourly resolution and force a numerical hydrologic model at a continental scale to depict such change. As results indicate, US floods are becoming 7.9% flashier by the end of the century assuming a high-emissions scenario. The Southwest (+10.5%) has the greatest increase in flashiness among historical flash flood hot spots, and the central US (+8.6%) is emerging as a new flash flood hot spot. Additionally, future flash flood-prone frontiers are advancing northwards. This study calls on implementing climate-resilient mitigation measures for emerging flash flood hot spots.
- Li, Z., Gao, S., Chen, M., Gourley, J., Mizukami, N., & Hong, Y. (2022). CREST-VEC: A framework towards more accurate and realistic flood simulation across scales. Geoscientific Model Development, 15(15). doi:10.5194/gmd-15-6181-2022More infoLarge-scale (i.e., continental and global) hydrologic simulation is an appealing yet challenging topic for the hydrologic community. First and foremost, model efficiency and scalability (flexibility in resolution and discretization) have to be prioritized. Then, sufficient model accuracy and precision are required to provide useful information for water resource applications. Towards this goal, we craft two objectives for improving US current operational hydrological models: (1) vectorized routing and (2) improved hydrological processes. This study presents a hydrologic modeling framework, CREST-VEC, that combines a gridded water balance model and a newly developed vector-based routing scheme. First, in contrast to a conventional fully gridded model, this framework can significantly reduce the computational cost of river routing by at least 10 times, based on experiments at regional (0.07 vs. 0.002 s per step) and continental scales (0.35 vs. 7.2 s per step). This provides adequate time efficiency for generating operational ensemble streamflow forecasts and even probabilistic estimates across scales. Second, the performance using the new vector-based routing is improved, with the median-aggregated NSE (Nash-Sutcliffe efficiency) score increasing from -0.06 to 0.18 over the CONUS (contiguous US). Third, with the lake module incorporated, the NSE score is further improved by 56.2 % and the systematic bias is reduced by 17 %. Lastly, over 20 % of the false alarms on 2-year floods in the US can be mitigated with the lake module enabled, at the expense of only missing 2.3 % more events. This study demonstrated the advantages of the proposed hydrological modeling framework, which could provide a solid basis for continental- and global-scale water modeling at fine resolution. Furthermore, the use of ensemble forecasts can be incorporated into this framework; and thus, optimized streamflow prediction with quantified uncertainty information can be achieved in an operational fashion for stakeholders and decision-makers.
- Li, Z., Tang, G., Kirstetter, P., Gao, S., Li, J., Wen, Y., & Hong, Y. (2022). Evaluation of GPM IMERG and its constellations in extreme events over the conterminous united states. Journal of Hydrology, 606. doi:10.1016/j.jhydrol.2021.127357More infoImproved quantification of extreme precipitation rates using observations has far-reaching implications for environmental sciences, especially for hydrometeorological studies. Yet, uncertainties still remain in satellite precipitation estimates, especially for a merged product. This study evaluates the performance of the Integrated Multi-satellite Retrievals for GPM (IMERG) in extreme events over the conterminous US. Three approaches are followed to define and evaluate extreme events: (1) a percentile-based analysis, (2) an event-based analysis using the National Weather Service storm database, and (3) a frequency-based analysis using intensity–durationfrequency (IDF) curves. The IMERG Early Run (ER), Late Run (LR), and Final Run (FR) products and their original passive microwave and infrared (IR) sensors are intercompared against the National Centers for Environmental Predictions Stage IV ground-based radar precipitation data from 2015 to 2019. In particular, we break down the performance in three types of events (rain, snow, and hail). The results reveal that: (1) three types of extreme definitions converge toward an overall agreement - the degrees of underestimation of high-end extreme precipitation rates increases with data latency (FR > LR > ER) and FR delivers overall best performance; (2) passive microwave (PMW) estimates generally exhibits better detectability and quantification of extreme precipitation than IR estimates, especially in heavy rains; (3) Amongst PMW sensors, MHS (SAPHIR)-based estimates show the best (worst) extreme detection with CSI (Critical Success Index) equaling 0.15 (0.10) while AMSR and SSMIS outperform others for quantifying extreme rates. Lastly, different sensors (e.g., imagers and sounders in PMW and IR) deliver variable performance regarding different precipitation types. These findings reveal that IMERG is not a homogeneous precipitation product when it comes to estimating precipitation extremes. There are rooms for improvement to enhance homogeneity across precipitation estimates used in IMERG.
- Chen, M., Li, Z., Gao, S., Luo, X., Wing, O., Shen, X., Gourley, J., Kolar, R., & Hong, Y. (2021). A comprehensive flood inundation mapping for Hurricane Harvey using an integrated hydrological and hydraulic model. Journal of Hydrometeorology, 22(7). doi:10.1175/JHM-D-20-0218.1More infoBecause climate change will increase the frequency and intensity of precipitation extremes and coastal flooding, there is a clear need for an integrated hydrology and hydraulic system that has the ability to model the hydrologic conditions over a long period and the flow dynamic representations of when and where the extreme hydrometeorological events occur. This system coupling provides comprehensive information (flood wave, inundation extents, and depths) about coastal flood events for emergency management and risk minimization. This study provides an integrated hydrologic and hydraulic coupled modeling system that is based on the Coupled Routing and Excessive Storage (CREST) model and the Australia National University-Geophysics Australia (ANUGA) model to simulate flood. Forced by the near-real-time Multi-Radar Multi-Sensor (MRMS) quantitative precipitation estimates, this integrated modeling system was applied during the 2017 Hurricane Harvey event to simulate the streamflow, the flood extent, and the inundation depth. The results were compared with postevent high-water-mark survey data and its interpolated flood extent by the U.S. Geological Survey and the Federal Emergency Management Agency flood insurance claims, as well as a satellite-based flood map, the National Water Model (NWM), and the Fathom (LISFLOOD-FP) model simulated flood map. The proposed hydrologic and hydraulic model simulation indicated that it could capture 87% of all flood insurance claims within the study area, and the overall error of water depth was 0.91 m, which is comparable to the mainstream operational flood models (NWM and Fathom). SIGNIFICANCE STATEMENT: We wanted to provide a tool for local emergency response officials to visualize the possible flood conditions in their region, because the heavy rainfall flood is likely becoming more frequent and more intense, possibly as a result of climate change. The simulated flood information includes the water channel flowrate, twodimensional flood extent, and the inundation depth throughout the flooded area. The results proved that, with less data requirement, the proposed tool can provide the comprehensive flood information, with accuracy of flood extent simulation comparable to mainstream flood models and acceptable inundation-depth simulation. We will keep working to expand the capability of the framework from the real-time simulation to the 1-h lead-time prediction.
- Gao, S., Chen, M., Li, Z., Cook, S., Allen, D., Neeson, T., Yang, T., Yami, T., & Hong, Y. (2021). Mapping dynamic non-perennial stream networks using high-resolution distributed hydrologic simulation: A case study in the upper blue river basin. Journal of Hydrology, 600. doi:10.1016/j.jhydrol.2021.126522More infoMore than half of all streams globally are non-perennial, and thus dynamic due to their expanding and retracting nature. Field mapping in conjunction with observational data from gauges and/or in-situ loggers is a typical approach for studying non-perennial stream dynamics, but these approaches underrepresent their spatiotemporal variability. High-resolution distributed hydrological modeling promises to bridge this gap, thanks to advances in model physics, remote sensing, and computational power. As the first step towards this goal, we investigate the capability of distributed hydrologic modeling to capture stream dynamics in Upper Blue River Basin, OK. Coupled Routing and Excess STorage (CREST), a distributed hydrological model, is used to simulate spatiotemporally varied streamflow at 10-meter spatial resolution and daily time steps. USGS stream gauge data and in-situ state logger data are used to calibrate and validate the simulation at the watershed outlet and small headwater tributaries, respectively. Dynamic Surface Water Estimate (DSWE), a LANDSAT product is also compared with simulated water presence in high-order streams. Results show that the CREST model can capture low-moderate streamflow values at the watershed outlet with a log-NSE value over 0.7 in the validation period, while underestimating high flow values due to the daily time step. Also, the calibrated model can accurately estimate wet/dry status as monitored by in-situ state loggers in nine headwater catchments. The dynamic stream networks are mapped over 2510 stream segments using the CREST simulation. Non-perennial streams are the most dynamic in small headwater tributaries (contributing area
- Gao, S., Li, Z., Chen, M., Allen, D., Neeson, T., & Hong, Y. (2021). Monitoring drought through the lens of landsat: Drying of rivers during the California droughts. Remote Sensing, 13(17). doi:10.3390/rs13173423More infoWater scarcity during severe droughts has profound hydrological and ecological impacts on rivers. However, the drying dynamics of river surface extent during droughts remains largely understudied. Satellite remote sensing enables surveys and analyses of rivers at fine spatial resolution by providing an alternative to in-situ observations. This study investigates the seasonal drying dynamics of river extent in California where severe droughts have been occurring more frequently in recent decades. Our methods combine the use of Landsat-based Global Surface Water (GSW) and global river bankful width databases. As an indirect comparison, we examine the monthly fractional river extent (FrcSA) in 2071 river reaches and its correlation with streamflow at co-located USGS gauges. We place the extreme 2012–2015 drought into a broader context of multi-decadal river extent history and illustrate the extraordinary change between during-and post-drought periods. In addition to river extent dynamics, we perform statistical analyses to relate FrcSA with the hydroclimatic variables obtained from the National Land Data Assimilation System (NLDAS) model simulation. Results show that Landsat provides consistent observation over 90% of area in rivers from March to October and is suitable for monitoring seasonal river drying in California. FrcSA reaches fair (>0.5) correlation with streamflow except for dry and mountainous areas. During the 2012–2015 drought, 332 river reaches experienced their lowest annual mean FrcSA in the 34 years of Landsat history. At a monthly scale, FrcSA is better correlated with soil water in more humid areas. At a yearly scale, summer mean FrcSA is increasingly sensitive to winter precipitation in a drier climate; and the elasticity is also reduced with deeper ground water table. Overall, our study demonstrates the detectability of Landsat on the river surface extent in an arid region with complex terrain. River extent in catchments of deficient water storage is likely subject to higher percent drop in a future climate with longer, more frequent droughts.
- Gao, S., Li, Z., Chen, M., Lin, P., Hong, Z., Allen, D., Neeson, T., & Hong, Y. (2021). Spatiotemporal variability of global river extent and the natural driving factors revealed by decades of Landsat observations, GRACE gravimetry observations, and land surface model simulations. Remote Sensing of Environment, 267. doi:10.1016/j.rse.2021.112725More infoRivers are among the most dynamic components in Earth's terrestrial water cycle and provide critical ecosystem services. Yet, the spatiotemporal variability of river surface extents remains largely unquantified at the global scale. Satellite remote sensing provides a promising alternative to in-situ observations, which can enable a more comprehensive survey and systematic analysis of global rivers at fine spatial resolutions. The study examines the spatiotemporal variability of river surface extent globally and its natural driving factors, by combining the use of Landsat-based Global Surface Water (GSW) and Global River Widths from Landsat (GRWL) databases. In addition to examining the long-term mean river surface extent in various climate zones, we perform statistical analyses to correlate monthly times series of fractional river extent with the terrestrial water storage (TWS) components obtained from the Gravity Recovery and Climate Experiment (GRACE) satellite observation and the Global Land Data Assimilation System (GLDAS) model simulations. Results show that the spatiotemporal variability of water presence in rivers can be explained well via differentiating climate zones. The analysis also shows that 52.7% of the global maximum river extent is covered by water less than half of time. Changes of fractional river extent are found to be highly correlated with groundwater storage in low- and mid-latitudes, whereas snow melting dominates the river dynamics in high latitudes. By examining the extremes of fractional river extent, we found that the abrupt changes of fractional river extent are well linked to precipitation anomalies in the equatorial, arid, and warm temperate areas. This study offers an innovative perspective to study spatiotemporal dynamics of rivers by combining optical remote sensing (Landsat), gravimetry observations (GRACE), and land surface simulations; and it highlights the significant role of low-flow-generating processes (snow melting, infiltration, and recharge-discharge) in controlling river dynamics in certain regions, which warrants future investigation.
- Gao, S., Zhang, J., Li, D., Jiang, H., & Fang, Z. (2021). Evaluation of Multiradar Multisensor and Stage IV Quantitative Precipitation Estimates during Hurricane Harvey. Natural Hazards Review, 22(1). doi:10.1061/(ASCE)NH.1527-6996.0000435More infoRadar-based quantitative precipitation estimate (QPE) serves as input for flood forecasting, and its importance gets magnified during catastrophic storms, e.g., Hurricane Harvey in 2017. The record-breaking rainfall from Hurricane Harvey covered vast spatial extents and lasted for a 5-day period, providing a unique chance for evaluating radar errors, especially their spatiotemporal dependence. Using the rainfall data of Hurricane Harvey, the authors utilize a new method for sampling ground-based rainfall measurements over radar pixels (i.e., spatial reference rainfall) based on subpixel rainfall variability. The new method aims to enlarge the sample size and allow for compressively evaluating the QPE. Two hourly QPE products, the Next Generation Weather Radar (NEXRAD) Stage IV and Multiradar Multisensor (MRMS), are chosen for the evaluation due to their roles in major flood forecasting activities; and a dense rain gauge network covering the whole of Harris County, Texas, provides the spatial rainfall reference in this analysis. Comparative analyses are conducted based on Hurricane Harvey and other two flood-inducing storms occurring in 2015 and 2016 over Harris County. The results imply that the Stage IV and MRMS overestimate and underestimate, respectively, the total rainfall by a small factor, while both QPEs tend to overestimate very light precipitation. In addition, the study suggests that the spatial correlation of radar error from both QPEs be described as powered exponential functions of interpixel distance. This study also includes hydrologic simulations for an urban watershed, demonstrating the importance of both the accuracy and spatial resolution of QPE in representing the mean areal precipitation (MAP) over catchments. The insight gained from this study provides guidance for further improving the QPE performance, and the new sampling approach for spatial reference rainfall can be applied to comprehensively evaluate long-term radar rainfall data.
- Kim, T., Yang, T., Gao, S., Zhang, L., Ding, Z., Wen, X., Gourley, J., & Hong, Y. (2021). Can artificial intelligence and data-driven machine learning models match or even replace process-driven hydrologic models for streamflow simulation?: A case study of four watersheds with different hydro-climatic regions across the CONUS. Journal of Hydrology, 598. doi:10.1016/j.jhydrol.2021.126423More infoWith recent developments in computational techniques, Data-driven Machine Learning Models (DMLs) have shown great potential in simulating streamflow and capturing the rainfall-runoff relationship in given watersheds, which are traditionally fulfilled by Process-based Hydrologic Models (PHMs). There are debates on whether the DMLs can outperform and possibly replace the classical PHMs for streamflow simulation and river forecasting, but no clear conclusions have been made. This study aims to investigate whether the newer DMLs have any potential in further improving the simulation accuracy of classical PHMs, and vice versa. To do this, we compared a few popular PHMs and DMLs over four watersheds across the Continental US (CONUS) that are associated with different input, climate, and regional conditions. A total of five hydrologic models were chosen, including (1) two classical lumped models, i.e., the Sacramento Soil Moisture Accounting (SAC-SMA) and Xinanjiang (XAJ); (2) one modern distributed model, termed Coupled Routing and Excess Storage (CREST); (3) and two DMLs including an Artificial Neural Networks (ANN) and a deep learning model, termed Long Short Term Memory (LSTM). Our results demonstrated that the DMLs still significantly biased when using the baseline input scenario with the PHMs. However, the DMLs fed with delayed input scenarios had great potential and can reach high simulation accuracy. The DMLs, especially the ANN, outperformed other employed models under the rainfall-runoff relationship in which rainfall dominantly drives. The DMLs also showed better performance in the high-flow regime, while the PHMs had a better performance for the low-flow regime, implying both PHMs and DMLs have their own merits and are worthy of joint development. In general, our study indicated a great potential of using DMLs to simulate streamflow, but further studies are still needed to verify the transferability and scalability of DMLs in large-scale experiments, such as the Distributed Model Intercomparison Projects 1&2 conducted by National Weather Services but to compare modern DMLs and PHMs.
- Li, Z., Chen, M., Gao, S., Luo, X., Gourley, J., Kirstetter, P., Yang, T., Kolar, R., McGovern, A., Wen, Y., Rao, B., Yami, T., & Hong, Y. (2021). CREST-iMAP v1.0: A fully coupled hydrologic-hydraulic modeling framework dedicated to flood inundation mapping and prediction. Environmental Modelling and Software, 141. doi:10.1016/j.envsoft.2021.105051More infoUnder the impacts of hydrologic extremes, there is a growing need for integrated frameworks for flood inundation mapping. In this study, we introduce a framework: the Coupled Routing and Excess Storage for inundation MApping and Prediction (CREST-iMAP). It utilizes a highly parallelized and fully integrated hydrologic-hydraulic model and aims to improve flood prediction. To highlight the advantages, a synthetic rainfall event and the 500-year Hurricane Harvey event were investigated using the CREST-iMAP, compared with a hydrologic model, a hydraulic model, and a simplified hydrologic-hydraulic model. The results indicate the CREST-iMAP achieves good performances in both hydrologic simulation and floodplain inundation mapping. Moreover, the antecedent soil moisture is the most sensitive parameter to model accuracy, followed by the land surface characteristics; the infiltration process to a flood forecasting model is significant. Overall, the CREST-iMAP delivers accurate and timely flood information, making it one of the latest developments of an integrated hydrologic-hydraulic expert system.
- Li, Z., Li, Z., Chen, M., Chen, M., Gao, S., Gao, S., Gourley, J., Gourley, J., Yang, T., Yang, T., Shen, X., Shen, X., Kolar, R., Kolar, R., Hong, Y., & Hong, Y. (2021). A multi-source 120-year US flood database with a unified common format and public access. Earth System Science Data, 13(8). doi:10.5194/essd-13-3755-2021More infoDespite several flood databases available in the United States, there is a benefit to combine and reconcile these diverse data sources into a comprehensive flood database with a unified common format and easy public access in order to facilitate flood-related research and applications. Typically, floods are reported by specialists or media according to their socioeconomic impacts. Recently, data-driven analysis can reconstruct flood events based on in situ and/or remote-sensing data. Lately, with the increasing engagement of citizen scientists, there is the potential to enhance flood reporting in near-real time. The central objective of this study is to integrate information from seven popular multi-sourced flood databases into a comprehensive flood database in the United States, made readily available to the public in a common data format. Natural language processing, geocoding, and harmonizing processing steps are undertaken to facilitate such development. In total, there are 698ĝ€¯507 flood records in the United States from 1900 to the present, which highlights the longest and most comprehensive recording of flooding across the country. The database features event locations, durations, date/times, socioeconomic impacts (e.g., fatalities and economic damages), and geographic information (e.g., elevation, slope, contributing area, and land cover types retrieved from ancillary data for given flood locations). Finally, this study utilizes the flood database to analyze flood seasonality within major basins and socioeconomic impacts over time. It is anticipated that thus far the most comprehensive yet unified database can support a variety of flood-related research, such as a validation resource for hydrologic or hydraulic simulations, hydroclimatic studies concerning spatiotemporal patterns of floods, and flood susceptibility analysis for vulnerable geophysical locations. The dataset is publicly available with the following DOI: 10.5281/zenodo.4547036 (Li, 2020).
- Li, Z., Tang, G., Hong, Z., Chen, M., Gao, S., Kirstetter, P., Gourley, J., Wen, Y., Yami, T., Nabih, S., & Hong, Y. (2021). Two-decades of GPM IMERG early and final run products intercomparison: Similarity and difference in climatology, rates, and extremes. Journal of Hydrology, 594. doi:10.1016/j.jhydrol.2021.125975More infoPrecipitation is an essential climate and forcing variable for modeling the global water cycle. Particularly, the recent Integrated Multi-satellite Retrievals for GPM (IMERG) product retrospectively provides an unprecedented two decades of high-resolution satellite precipitation estimates. The primary goal of this study is to examine the similarities and differences between the two latest and also arguably the most popular, GPM IMERG Early and Final Run (ER and FR) products across the globe. The results reveal that: (1) ER systematically estimates 12.0% higher annual rainfall than FR, particularly over land (16.7%); (2) ER and FR show significant differences in instantaneous rates (Root Mean Squared Difference: RMSD = 2.38 mm h−1 and normalized RMSD: RMSD_norm = 1.09), especially in Africa (RMSD = 2.40 mm h−1) and hot, arid regions (RMSD_norm = 1.11), but less so in Europe (RMSD = 2.16 mm h−1) and cold areas (RMSD_norm = 0.87); and (3) ER measures 33.0% higher extreme rainfall rates than FR over the globe. The exploration of their similarities and differences provides a first-order global assessment of various hydrological utilities: FR is designed to be more suitable for retrospective hydroclimatology and water resource applications, while the earliest available ER product, though not bias-corrected by rain gauges, still shows potential utility for operational modeling of rainfall-triggered hazards. The findings of this study can provide an assessment to product developers and broader data users and practitioners to address the inherent issues in hardware limitations, retrieval algorithms, and uncertainty quantification for research and applications.
- Chen, M., Nabih, S., Brauer, N., Gao, S., Gourley, J., Hong, Z., Kolar, R., & Hong, Y. (2020). Can remote sensing technologies capture the extreme precipitation event and its cascading hydrological response? A case study of hurricane harvey using ef5 modeling framework. Remote Sensing, 12(3). doi:10.3390/rs12030445More infoA new generation of precipitation measurement products has emerged, and their performances have gained much attention from the scientific community, such as the Multi-Radar Multi-Sensor system (MRMS) from the National Severe Storm Laboratory (NSSL) and the Global Precipitation Measurement Mission (GPM) from the National Aeronautics and Space Administration (NASA). This study statistically evaluated the MRMS and GPM products and investigated their cascading hydrological response in August of 2017, when Hurricane Harvey brought historical and record-breaking precipitation to the Gulf Coast (>1500 mm), causing 107 fatalities along with about USD 125 billion worth of damage. Rain-gauge observations from Harris County Flood Control District (HCFCD) and stream-gauge measurements by the United States Geological Survey (USGS) were used as ground truths to evaluate MRMS, GPM and National Centers for Environmental Prediction (NCEP) gauge-only data by using statistical metrics and hydrological simulations using the Ensemble Framework for Flash Flooding Forecast (EF5) model. The results indicate that remote sensing technologies can accurately detect and estimate the unprecedented precipitation event with their near-real-time products, and all precipitation products produced good hydrological simulations, where the Nash-Sutcliff model efficiency coefficients (NSCE) were close to 0.9 for both the MRMS and GPM products. With the timeliness and seamless coverage of MRMS and GPM, the study also demonstrated the capability and efficiency of the EF5 framework for flash flood modeling over the United States and potentially additional international domains.
- Li, Z., Chen, M., Gao, S., Hong, Z., Tang, G., Wen, Y., Gourley, J., & Hong, Y. (2020). Cross-examination of similarity, difference and deficiency of gauge, radar and satellite precipitation measuring uncertainties for extreme events using conventional metrics and multiplicative triple collocation. Remote Sensing, 12(8). doi:10.3390/RS12081258More infoQuantifying uncertainties of precipitation estimation, especially in extreme events, could benefit early warning of water-related hazards like flash floods and landslides. Rain gauges, weather radars, and satellites are three mainstream data sources used in measuring precipitation but have their own inherent advantages and deficiencies. With a focus on extremes, the overarching goal of this study is to cross-examine the similarities and differences of three state-of-the-art independent products (Muti-Radar Muti-Sensor Quantitative Precipitation Estimates, MRMS; National Center for Environmental Prediction gridded gauge-only hourly precipitation product, NCEP; Integrated Multi-satellitE Retrievals for GPM, IMERG), with both traditional metrics and the Multiplicative Triple Collection (MTC) method during Hurricane Harvey and multiple Tropical Cyclones. The results reveal that: (a) the consistency of cross-examination results against traditional metrics approves the applicability of MTC in extreme events; (b) the consistency of cross-events of MTC evaluation results also suggests its robustness across individual storms; (c) all products demonstrate their capacity of capturing the spatial and temporal variability of the storm structures while also magnifying respective inherent deficiencies; (d) NCEP and IMERG likely underestimate while MRMS overestimates the storm total accumulation, especially for the 500-year return Hurricane Harvey; (e) both NCEP and IMERG underestimate extreme rainrates (>= 90 mm/h) likely due to device insensitivity or saturation while MRMS maintains robust across the rainrate range; (g) all three show inherent deficiencies in capturing the storm core of Harvey possibly due to device malfunctions with the NCEP gauges, relative low spatiotemporal resolution of IMERG, and the unusual "hot" MRMS radar signals. Given the unknown ground reference assumption of MTC, this study suggests that MRMS has the best overall performance. The similarities, differences, advantages, and deficiencies revealed in this study could guide the users for emergency response and motivate the community not only to improve the respective sensor/algorithm but also innovate multidata merging methods for one best possible product, specifically suitable for extreme storm events.
- Zhang, J., Lin, P., Gao, S., & Fang, Z. (2020). Understanding the re-infiltration process to simulating streamflow in North Central Texas using the WRF-hydro modeling system. Journal of Hydrology, 587. doi:10.1016/j.jhydrol.2020.124902More infoWRF-Hydro (Weather Research and Forecasting model-Hydrological modeling system), as the core engine of the United States National Water Model (NWM), has now been used in many hydrometeorological applications throughout the world. One important feature that WRF-Hydro introduced is to allow infiltration excess (“ponded water”) for subsequent lateral re-distribution and soil re-infiltration, which is a major enhancement in terms of physical realism. Nonetheless, how well WRF-Hydro models re-infiltration is largely unknown, because this process is difficult to be directly measured. To gain an in-depth understanding of re-infiltration process under different hydrometeorological/geographical conditions with model parameter settings, we start conducting a series of idealized numerical experiments using 18 watersheds in North Central Texas as a testbed. Next, the model is automatically calibrated to best quantify re-infiltration amounts during two major storms (2010 Tropical Storm Hermine and 2015 May Event), which is accomplished by coupling the dynamically dimensioned search (DDS) algorithm with WRF-Hydro to achieve optimal calibration efficiency. The results show that re-infiltration has quite substantial impacts on streamflow simulation in WRF-Hydro, especially for areas with flat terrains and soils with high clay content. Among all examined factors, precipitation, saturated hydraulic conductivity (Ksat) and runoff partition parameter (REFKDT) are found to impose relatively higher impacts on both re-infiltration ratio and runoff coefficient. It is also found that the runoff coefficient and the re-infiltration ratio are positively correlated based on results from both hypothetical and real events, indicating re-infiltration effects can become more pronounced as flood potential increases. These findings collectively show the significance of representing the re-infiltration process in flood forecasting. Models that do not incorporate this process may be over-calibrated to compensate errors originated from the missing process.
- Fang, Z., Shultz, M., Wienhold, K., Zhang, J., & Gao, S. (2019). Case study: Comparative analysis of hydrologic simulations with areal-averaging of moving rainfall. Hydrology, 6(1). doi:10.3390/hydrology6010010More infoThe goal of this investigation is to compare the hydrologic simulations caused by the areal-averaging of dynamic moving rainfall. Two types of synthetic rainfall are developed: spatially varied rainfall (SVR) is the typical input to a distributed model while temporally varied rainfall (TVR) emulates SVR but is spread uniformly over the entire watershed as in the case of a lumped model. This study demonstrates a direct comparison of peak discharge and peak timing generated by synthetic moving storms over idealized rectangular basins and a real watershed. It is found that the difference between the hydrologic responses from SVR and TVR reflects the impact from the areal-averaging of rainfall; the areal-averaging of rainfall for the movement from upstream to downstream over a lumped model can result in underestimated and delayed peak values in comparison to those from a distributed model; the flood peaks from SVR and TVR are found similar when the storm moves from downstream to upstream. The findings of the study suggest that extra cautions are needed for practitioners when evaluating simulated results from distributed and lumped modeling approaches even using the same rainfall information.
- Gao, S., & Fang, Z. (2019). Investigating hydrologic responses to spatio-temporal characteristics of storms using a Dynamic Moving Storm generator. Hydrological Processes, 33(21). doi:10.1002/hyp.13524More infoA synthetic storm generator—Dynamic Moving Storm (DMS)—is developed in this study to represent spatio-temporal variabilities of rainfall and storm movement in synthetic storms. Using an urban watershed as the testbed, the authors investigate the hydrologic responses to the DMS parameters and their interactions. In order to reveal the complex nature of rainfall–run-off processes, previously simplified assumptions are relaxed in this study regarding (a) temporal variability of rainfall intensity and (b) time-invariant flow velocity in channel routing. The results of this study demonstrate the significant contribution of storm moving velocity to the variation of peak discharge based on a global sensitivity analysis. Furthermore, a pairwise sensitivity analysis is conducted to elucidate not only the patterns in individual contributions from parameters to hydrologic responses but also their interactions with storm moving velocity. The intricacies of peak discharges resulting from sensitivity analyses are then dissected into independent hydrologic metrics, that is, run-off volume and standard deviation of run-off timings, for deeper insights. It is confirmed that peak discharge is increased when storms travel downstream along the main channel at the speed that corresponds to a temporal superposition of run-off. Spatial concentration of catchment rainfall is found to be a critical linkage through which characteristics of moving storms affect peak discharges. In addition, altering peak timing of rainfall intensity in conjunction with storm movement results in varied storm core locations in the channel network, which further changes the flow attenuation effects from channel routing. For future directions, the DMS generator will be embedded in a stochastic modelling framework and applied in rainfall/flow frequency analysis.