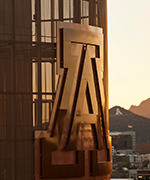
Shengjie Xu
- Assistant Professor
- Member of the Graduate Faculty
- (520) 626-2422
- UA Sierra Vista Campus, Rm. 101
- Tucson, AZ 85721
- sjxu@arizona.edu
Biography
Shengjie Xu received a Ph.D. degree in Computer Engineering from the University of Nebraska-Lincoln in 2019, an M.S. degree in Telecommunications from the University of Pittsburgh in 2014, and a B.E. degree in Information Security in 2012. He has been a tenure-track assistant professor at the University of Arizona (U of A) since August 2024, a Co-Principal Investigator (Co-PI) of the Google-Funded Arizona Cybersecurity Clinic, and an affiliate faculty at the College of Information Science. Before joining U of A, he was a tenure-track assistant professor at San Diego State University from 2022 to 2024 and at Dakota State University from 2019 to 2022.
His research interests include cybersecurity, Cyber AI, Zero Trust, Cryptography, Secure AI, and Graph Modeling. He is the author of one book and more than 40 articles. He is the lead author of the book `Cybersecurity in Intelligent Networking Systems' (John Wiley/IEEE Press, 2023). He currently serves as a Technical Editor for the IEEE Wireless Communications Magazine, an Associate Editor for the IEEE Access, an Editor for the International Journal of Sensor Networks, and a Technical Program Committee (TPC) Vice Chair for the International Symposium on Intelligent Computing and Networking (ISICN) 2025. He has received grants and contracts in research and educational projects from the U.S. National Science Foundation (NSF) and other organizations. He served as a proposal panel reviewer for the NSF.
He was a recipient of the Exemplary Editor Award of 2024 from the IEEE Wireless Communications in 2025, the Outstanding Faculty Award from the Fowler College of Business at San Diego State University in 2024, the Institution of Engineering and Technology (IET) Journals Premium Award for Best Paper in 2020, the Milton E. Mohr Graduate Fellowship Award from the College of Engineering at the University of Nebraska-Lincoln in 2017, and the Best Poster Award from the International Conference on Design of Reliable Communication Networks (DRCN) in 2015. He is a senior member of IEEE and holds multiple professional certifications in cybersecurity and computer networking.
Degrees
- Ph.D. Computer Engineering
- University of Nebraska-Lincoln, Lincoln, Nebraska, United States
- M.S. Telecommunications
- University of Pittsburgh, Pittsburgh, Pennsylvania, United States
Work Experience
- San Diego State University, San Diego, California (2022 - 2024)
- Dakota State University (2019 - 2022)
Awards
- Exemplary Editor Award
- IEEE Wireless Communications Magazine, Spring 2025
- Outstanding Faculty Award
- San Diego State University, Spring 2024
- IET Journal Premium Award for Best Paper
- IET - Institution of Engineering and Technology, Winter 2020
- Milton E. Mohr Graduate Fellowship Award
- University of Nebraska-Lincoln, Spring 2017
- Best Poster Award
- International Conference on Design of Reliable Communication Networks, Spring 2015
- Outstanding Graduate Students Scholarship
- University of Pittsburgh, Spring 2013
Licensure & Certification
- CNSS No. 4014A – Information Systems Security Officer, Committee on National Security Systems (CNSS) (2014)
- CNSS No. 4015 – System Certifiers, Committee on National Security Systems (CNSS) (2014)
- Cisco Certified Network Associate (CCNA) in Routing and Switching, Cisco (2012)
- CNSS No. 4011 – Information Systems Security Professionals, Committee on National Security Systems (CNSS) (2014)
- CNSS No. 4012 – Designated Approving Authority, Committee on National Security Systems (CNSS) (2014)
- CNSS No. 4013 – System Administrators in Information Systems Security, Committee on National Security Systems (CNSS) (2014)
- Cisco Certified Network Associate (CCNA) in Security, Cisco (2013)
Interests
Teaching
Cybersecurity, AI, Machine Learning, Computer Networks
Research
Cybersecurity, AI, Machine Learning, Zero Trust, Graph Modeling, Edge Computing, Edge AI, Cryptography
Courses
2025-26 Courses
-
Artificial Intelligence
APCV 471 (Fall 2025)
2024-25 Courses
-
Statistics in Information Age
APCV 302 (Summer I 2025) -
Capstone in Cyber Operations
CYBV 498 (Spring 2025) -
Directed Research
CYBV 692 (Spring 2025) -
Network Security Prin
NETV 371 (Spring 2025) -
Artificial Intelligence
CSCV 471 (Fall 2024) -
Capstone in Cyber Operations
CYBV 498 (Fall 2024)
Scholarly Contributions
Books
- Xu, S., Qian, Y. i., & Hu, R. Q. (2022). Cybersecurity in Intelligent Networking Systems. John Wiley & Sons.
Chapters
- Xu, S., Qian, Y. i., & Hu, R. Q. (2023). Adversarial Examples: Challenges and Solutions. In Cybersecurity in Intelligent Networking Systems.
- Xu, S., Qian, Y. i., & Hu, R. Q. (2023). Cyber Threats and Gateway Defense. In Cybersecurity in Intelligent Networking Systems.
- Xu, S., Qian, Y. i., & Hu, R. Q. (2023). Cybersecurity in the Era of Artificial Intelligence. In Cybersecurity in Intelligent Networking Systems.
- Xu, S., Qian, Y. i., & Hu, R. Q. (2023). Edge Computing and Secure Edge Intelligence. In Cybersecurity in Intelligent Networking Systems.
- Xu, S., Qian, Y. i., & Hu, R. Q. (2023). Efficient Pre-processing Scheme for Anomaly Detection. In Cybersecurity in Intelligent Networking Systems.
- Xu, S., Qian, Y. i., & Hu, R. Q. (2023). Privacy preservation in the era of big data. In Cybersecurity in Intelligent Networking Systems.
- Xu, S., Xu, S., Qian, Y. i., Qian, Y. i., Hu, R. Q., & Hu, R. Q. (2023). Robust Intrusion Detection. In Cybersecurity in Intelligent Networking Systems.
Journals/Publications
- McCully, G. A., Hastings, J. D., & Xu, S. (2024). Impact of Data Snooping on Deep Learning Models for Locating Vulnerabilities in Lifted Code. arXiv preprint arXiv:2412.02048.
- McCully, G. A., Hastings, J. D., Xu, S., & Fortier, A. (2024). Bi-Directional Transformers vs. word2vec: Discovering Vulnerabilities in Lifted Compiled Code. arXiv preprint arXiv:2405.20611.
- McCully, G. A., Hastings, J. D., Xu, S., & Fortier, A. (2024). Comparing unidirectional, bidirectional, and word2vec models for discovering vulnerabilities in compiled lifted code. arXiv preprint arXiv:2409.17513.
- Gyawali, S., Xu, S., Qian, Y., & Hu, R. (2021). Challenges and Solutions for Cellular Based V2X Communications. IEEE Communications Surveys and Tutorials, 23(1). doi:10.1109/COMST.2020.3029723More infoA wide variety of works have been conducted in vehicle-to-everything (V2X) communications to enable a variety of applications for road safety, traffic efficiency and passenger infotainment. Although dedicated short-range communications (DSRC) based V2X is already in the deployment phase, cellular based V2X is gaining more interest in academia and industry most recently. This article surveys the existing work and challenges on LTE and 5G to support efficient V2X communications. First, we present the motivations for cellular based V2X communications. Second, we summarize the LTE V2X architecture and operating scenarios being considered. Third, we discuss the challenges in existing LTE for supporting V2X communications such as physical layer structure, synchronization, multimedia broadcast multicast services (MBMS), resource allocation, security and survey the recent solutions to these challenges. We further discuss the challenges and possible solutions for 5G based vehicular communications. Finally, we discuss the open research issues and possible research directions in cellular based vehicular communications.
- Gyawali, S., Xu, S., Qian, Y. i., & Hu, R. Q. (2020). Challenges and solutions for cellular based V2X communications. IEEE Communications Surveys & Tutorials, 23(1), 222--255.
- Xu, S., Qian, Y. i., & Hu, R. Q. (2020). Edge intelligence assisted gateway defense in cyber security. IEEE Network, 34(4), 14--19.
- Xu, S., Qian, Y., & Hu, R. (2020). Data-Driven Edge Intelligence for Robust Network Anomaly Detection. IEEE Transactions on Network Science and Engineering, 7(3). doi:10.1109/TNSE.2019.2936466More infoThe advancement of networking platforms for assured online services requires robust and effective network intelligence systems against anomalous events and malicious threats. With the rapid development of modern communication technologies, artificial intelligence, and the revolution of computing devices, cloud computing empowered network intelligence will inevitably become a core platform for various smart applications. While cloud computing provides strong and powerful computation, storage, and networking services to detect and defend cyber threats, edge computing on the other hand will deliver more benefits in specific yet potential critical areas. In this paper, we present a study on the data-driven edge intelligence for robust network anomaly detection. We first highlight the main motivations for edge intelligence, and then propose an intelligence system empowered by edge computing for network anomaly detection. We further propose a scheme on the data-driven robust network anomaly detection. In the proposed scheme, four phases are designed to incorporate with data-driven approaches to train a learning model which is able to detect and identify a network anomaly in a robust way. In the performance evaluations with data experiments, we demonstrate that the proposed scheme achieves the robustness of trained model and the efficiency on the detection of specific anomalies.
- Xu, S., Qian, Y. i., & Hu, R. Q. (2019). Data-driven edge intelligence for robust network anomaly detection. IEEE Transactions on Network Science and Engineering, 7(3), 1481--1492.
- Xu, S., Qian, Y. i., & Hu, R. Q. (2019). Data-driven network intelligence for anomaly detection. IEEE Network, 33(3), 88--95.
- Xu, S., & Qian, Y. i. (2018). Machine Learning Enabled Data Preprocessing in Cyber Security Applications. IEEE ComSoc MMTC Communications - Frontiers, 13(6), 15--18.
- Xu, S., Qian, Y. i., & Qingyang, H. R. (2018). Reliable and resilient access network design for advanced metering infrastructures in smart grid. IET Smart Grid, 1(1), 24--30.
- Xu, S., Qian, Y. i., & Hu, R. Q. (2015). On reliability of smart grid neighborhood area networks. IEEE Access, 3, 2352--2365.
Proceedings Publications
- Hardgrove, I., & Xu, S. (2025). Towards Intelligent Fuzzing: Leveraging Large Language Models for Improved Software Security. In International Symposium on Intelligent Computing and Networking (ISICN).
- McCully, G., Hastings, J., & Xu, S. (2025). Impact of Data Snooping on Deep Learning Models for Locating Vulnerabilities in Lifted Code. In International Symposium on Intelligent Computing and Networking (ISICN).
- McCully, G., Hastings, J., Xu, S., & Fortier, A. (2025). Comparing Unidirectional, Bidirectional, and Word2vec Models for Discovering Vulnerabilities in Compiled Lifted Code. In 39th Anuual AAAI Conference on Artificial Intelligence 2025 - Artificial Intelligence for Cyber Security (AICS) Workshop.
- McCully, G., Hastings, J., Xu, S., & Fortier, A. (2025). Comparing Unidirectional, Bidirectional, and Word2vec Models for Discovering Vulnerabilities in Compiled Lifted Code. In International Symposium on Digital Forensics and Security (ISDFS).
- Xu, S., & Qian, Y. i. (2025). Zero Trust Architecture for Securing Edge AI and Federated Learning in Edge Computing. In IEEE INFOCOM 2025-IEEE Conference on Computer Communications Workshops (INFOCOM WKSHPS).
- Fehrman, B., Akowuah, F., & Xu, S. (2024). Adversarial Attacks in Problem Space for VBA Code Samples. In International Symposium on Intelligent Computing and Networking.
- Ikei, B., Thiry, H., & Xu, S. (2024). Towards Robust IoT Security: A Blockchain Design with Attribute-Based Encryption. In International Symposium on Intelligent Computing and Networking.
- Liu, J., Vyas, P., & Xu, S. (2024). Real-Time Fake News Detection on the X (Twitter): An Online Machine Learning Approach. In Americas' Conference on Information Systems (AMCIS).
- McCully, G., Hastings, J., Xu, S., & Fortier, A. (2024). Bi-Directional Transformers vs. word2vec: Discovering Vulnerabilities in Lifted Compiled Code. In 2024 IEEE Cyber Awareness and Research Symposium (CARS).
- Nelson, T., O’Brien, A., Noteboom, C., & Xu, S. (2024). WCFG: A Weighted Control Flow Graph Dataset Design for Malware Classification. In International Symposium on Intelligent Computing and Networking.
- Ward, B., Gonzalez, D., Alt, J., Fang, D., & Xu, S. (2024). Optimizing Weighted Ensemble ML IDS Using Parallelization and Batch Learning in Healthcare Networks. In 2024 IEEE International Conference on E-health Networking, Application & Services (HealthCom).
- Zhang, K., Xu, S., & Shin, B. (2023). Towards Adaptive Zero Trust Model for Secure AI. In 2023 IEEE Conference on Communications and Network Security (CNS).
- Ahmed, T., & Xu, S. (2022). Shellcoding: Hunting for Kernel32 Base Address. In IEEE INFOCOM 2022-IEEE Conference on Computer Communications Workshops (INFOCOM WKSHPS).
- Bradley, M., & Xu, S. (2021). A Metric for Machine Learning Vulnerability to Adversarial Examples. In IEEE International Conference on Computer Communications (INFOCOM).
- Burr, J., & Xu, S. (2021). Improving Adversarial Attacks Against Executable Raw Byte Classifiers. In IEEE International Conference on Computer Communications (INFOCOM).
- Chaudhary, S., O’Brien, A., & Xu, S. (2020). Automated post-breach penetration testing through reinforcement learning. In 2020 IEEE Conference on Communications and Network Security (CNS).
- Fang, D., Xu, S., & Sharif, H. (2019). Security analysis of wireless train control systems. In 2019 IEEE Globecom Workshops (GC Wkshps).
- Xu, S., Fang, D., & Sharif, H. (2019). Efficient network anomaly detection for edge gateway defense in 5G. In 2019 IEEE Globecom Workshops (GC Wkshps).
- Xu, S., Qian, Y. i., & Hu, R. Q. (2019). A semi-supervised learning approach for network anomaly detection in fog computing. In ICC 2019-2019 IEEE International Conference on Communications (ICC).
- Gyawali, S., Xu, S., Ye, F., Hu, R. Q., & Qian, Y. i. (2018). A D2D based clustering scheme for public safety communications. In 2018 IEEE 87th Vehicular Technology Conference (VTC Spring).
- Xu, S., & Ye, F. (2018). A predicate encryption based anomaly detection scheme for e-Health communications network. In 2018 IEEE International Conference on Communications (ICC).
- Xu, S., Qian, Y. i., & Hu, R. Q. (2018). Privacy-preserving data preprocessing for fog computing in 5G network security. In 2018 IEEE Global Communications Conference (GLOBECOM).
- Xu, S., Qian, Y. i., & Hu, R. Q. (2017). A data-driven preprocessing scheme on anomaly detection in big data applications. In 2017 IEEE Conference on Computer Communications Workshops (INFOCOM WKSHPS).
- Xu, S., Qian, Y. i., & Hu, R. Q. (2017). A study on communication network reliability for advanced metering infrastructure in smart grid. In 2017 IEEE 15th Intl Conf on Dependable, Autonomic and Secure Computing, 15th Intl Conf on Pervasive Intelligence and Computing, 3rd Intl Conf on Big Data Intelligence and Computing and Cyber Science and Technology Congress (DASC/PiCom/DataCom/CyberSciTech).
- Xu, S., Qian, Y. i., & Hu, R. Q. (2016). A secure data learning scheme in big data applications. In 2016 25th International Conference on Computer Communication and Networks (ICCCN).
- Xu, S., & Qian, Y. i. (2015). Quantitative study of reliable communication infrastructure in smart grid NAN. In 2015 11th International Conference on the Design of Reliable Communication Networks (DRCN).
- Xu, S., Lei, X., Li, J., & Shi, H. (2011). USB Storage Device Surveillance System in Intranet. In Proceedings of the 2011 International Conference on Information Security and Intelligence Control.
Others
- Zurawski, J. (2021, November). South Dakota Region Scientific Deep Dive. osti.gov.