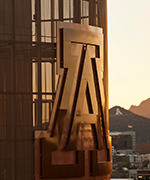
Rozhin Yasaei
- Assistant Professor
- Member of the Graduate Faculty
- Assistant Professor, Emergency Medicine
- Assistant Professor, Electrical and Computer Engineering
Contact
- (520) 626-2422
- UA Sierra Vista Campus, Rm. 101
- Tucson, AZ 85721
- yasaei@arizona.edu
Degrees
- Ph.D. Computer Engineering
- University of California Irvine, Irvine, California, United States
- Graph Neural Network for Integrated Circuits and Cyber-Physical Systems Security
- M.S. Computer Engineering
- University of California Irvine, Irvine, California, United States
- Golden Chip-Free Hardware Trojan Detection Through Side-Channel Analysis Using Machine Learning
- B.S. Electrical Engineering
- Sharif University of Technology, Tehran, Iran, Islamic Republic of
- Design and Implementation of a Smart Glove for Music Classification Based on Human Hand Movements
Awards
- CSM Fellowship Award
- Center for Semiconductor (CSM), Fall 2024 (Award Nominee)
- DSU CAE Workshop Travel Support
- Dakota State University CAE Faculty Professional Development Workshop, Summer 2024
Interests
No activities entered.
Courses
2025-26 Courses
-
Cyber-Physical Systems
CYBV 525 (Fall 2025) -
Intro Security Programming I
CYBV 310 (Fall 2025)
2024-25 Courses
-
Intro Security Programming I
CYBV 310 (Spring 2025) -
Intro Security Programming I
CYBV 310 (Fall 2024)
2023-24 Courses
-
Intro Security Programming I
CYBV 310 (Summer I 2024) -
Intro Security Programming I
CYBV 310 (Spring 2024) -
Intro Security Programming II
CYBV 311 (Spring 2024) -
Intro Security Programming I
CYBV 310 (Fall 2023) -
Intro Security Programming II
CYBV 311 (Fall 2023)
Scholarly Contributions
Chapters
- Olabanjo, O., Seurin, P., Wiggins, J., Pratt, L., Rana, L., Yasaei, R., & Renard, G. (2025). Chapter 9 - Natural Language Processing for Earth resource management: a case of H2 Golden Retriever research. In Data Analytics and Artificial Intelligence for Earth Resource Management(pp 157-183). Elsevier.
Journals/Publications
- Yasaei, R., Chen, L., Yu, S., & Faruque, M. (2024). Hardware Trojan Detection using Graph Neural Networks. IEEE Transactions on Computer-Aided Design of Integrated Circuits and Systems. doi:10.1109/TCAD.2022.3178355More infoThe globalization of the Integrated Circuit (IC) supply chain has moved most of the design, fabrication, and testing process from a single trusted entity to various untrusted third party entities around the world. The risk of using untrusted third-Party Intellectual Property (3PIP) is the possibility for adversaries to insert malicious modifications known as Hardware Trojans (HTs). These HTs can compromise the integrity, deteriorate the performance, and deny the functionality of the intended design. Various HT detection methods have been proposed in the literature; however, many fall short due to their reliance on a golden reference circuit, a limited detection scope, the need for manual code review, or the inability to scale with large modern designs. We propose a novel golden reference-free HT detection method for both Register Transfer Level (RTL) and gate-level netlists by leveraging Graph Neural Networks (GNNs) to learn the behavior of the circuit through a Data Flow Graph (DFG) representation of the hardware design. We evaluate our model on a custom dataset by expanding the Trusthub HT benchmarks trusthub1. The results demonstrate that our approach detects unknown HTs with 97% recall (true positive rate) very fast in 21.1ms for RTL and 84% recall in 13.42s for Gate-Level Netlist.
Proceedings Publications
- Yasaei, R., Moghaddas, Y., & Al, F. (2024). IoT-GRAF: IoT Graph Learning-Based Anomaly and Intrusion Detection Through Multi-Modal Data Fusion. In 2024 Design, Automation & Test in Europe Conference & Exhibition (DATE).
- Yasaei, R., Zargari, A., Al Faruque, M., & Kurdahi, F. (2024). Unraveling Sensor Correlations in Multi-Sensor Wearable Devices for Smart Anomaly Detection. In 2024 IEEE 17th Dallas Circuits and Systems Conference (DCAS).More infoIn health monitoring and activity tracking technologies, wearable or implantable sensors have become indispensable, linking various human body regions to collect vital health data. Despite their potential, ensuring the security and reliability of these devices presents significant challenges, primarily due to the complexity of real-world scenarios that these systems encounter. Current approaches often rely on anomaly detection models that process historical sensor data to identify issues. However, these models tend to falter when faced with unexpected conditions or 'corner cases,' lacking the ability to generalize across the diverse situations encountered in everyday use. This limitation is particularly critical in wearable devices, where unexpected incidents are of paramount importance and cannot be overlooked. Addressing this gap, our research investigates multi-sensor wearable systems to understand the context of system operations and their characteristics. We introduce a context-aware approach that leverages the unique physics of the human body to identify the intricate relationships between sensors. By extracting sensor relations and patterns, our approach aims to enhance the detection of security and reliability issues, offering an advancement over traditional methods.