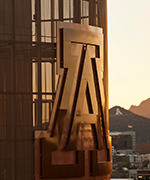
Chicheng Zhang
- Assistant Professor, Computer Science
- Member of the Graduate Faculty
Contact
- (520) 621-4622
- Gould-Simpson, Rm. 720
- Tucson, AZ 85721
- chichengz@arizona.edu
Bio
No activities entered.
Interests
No activities entered.
Courses
2024-25 Courses
-
Dissertation
CSC 920 (Spring 2025) -
Dissertation
STAT 920 (Spring 2025) -
Honors Thesis
CSC 498H (Spring 2025) -
Independent Study
CSC 699 (Spring 2025) -
Principles of Data Science
CSC 380 (Spring 2025) -
AdvTpc Artificial Intelligence
CSC 696H (Fall 2024) -
Dissertation
CSC 920 (Fall 2024) -
Dissertation
STAT 920 (Fall 2024) -
Honors Thesis
CSC 498H (Fall 2024) -
Research
CSC 900 (Fall 2024)
2023-24 Courses
-
Dissertation
STAT 920 (Summer I 2024) -
Dissertation
CSC 920 (Spring 2024) -
Independent Study
CSC 599 (Spring 2024) -
Principles of Machine Learning
CSC 480 (Spring 2024) -
Principles of Machine Learning
CSC 580 (Spring 2024) -
Research
CSC 900 (Spring 2024) -
Thesis
STAT 910 (Spring 2024) -
AdvTpc Artificial Intelligence
CSC 696H (Fall 2023) -
Independent Study
CSC 399 (Fall 2023) -
Independent Study
STAT 599 (Fall 2023) -
Research
CSC 900 (Fall 2023)
2022-23 Courses
-
Principles of Data Science
CSC 380 (Spring 2023) -
Research
CSC 900 (Spring 2023) -
Principles of Machine Learning
CSC 580 (Fall 2022) -
Research
CSC 900 (Fall 2022)
2021-22 Courses
-
Independent Study
STAT 599 (Spring 2022) -
Machine Learning Theory
CSC 588 (Spring 2022) -
Research
CSC 900 (Spring 2022) -
AdvTpc Artificial Intelligence
CSC 696H (Fall 2021) -
Research
CSC 900 (Fall 2021)
2020-21 Courses
-
Machine Learning Theory
CSC 588 (Spring 2021) -
Research
CSC 900 (Spring 2021) -
Research
CSC 900 (Fall 2020)
2019-20 Courses
-
Adv Tpcs:Doctoral Colloq
CSC 695C (Spring 2020) -
Adv Tpcs Computat Intell
CSC 665 (Fall 2019) -
Adv Tpcs:Doctoral Colloq
CSC 695C (Fall 2019) -
Directed Research
CSC 492 (Fall 2019)
Scholarly Contributions
Journals/Publications
- Zhang, C. (2020). Contextual Bandits with Continuous Actions: Smoothing, Zooming, and Adapting. Journal of Machine Learning Research.More infoThis is a journal version of our COLT 2019 paper. JMLR is a CORE A* journal.
- Zhang, C. (2019). Bandit Multiclass Linear Classification: Efficient Algorithms for the Separable Case. In Proceedings of the 36th International Conference on Machine Learning, volume 97 of Proceedings of Machine Learning Research, pages 624–633. PMLR, 2019..More infoTop-tier ML conference
- Zhang, C. (2019). Warm-starting Contextual Bandits: Robustly Combining Supervised and Bandit Feedback. In Proceedings of the 36th International Conference on Machine Learning, volume 97 of Proceedings of Machine Learning Research, pages 7335–7344. PMLR, 2019..More infoTop tier conference in ML
- Zhang, C. (2017). Efficient Online Bandit Multiclass Learning with $\tilde{O}(\sqrt{T})$ Regret. Proceedings of the 34th International Conference on Machine Learning-Volume 70, pages 488–497. JMLR. org, 2017..More infoTop-tier conference in ML
- Zhang, C. (2017). Revisiting Perceptron: Efficient and Label-Optimal Learning of Halfspaces. Advances in Neural Information Processing Systems, pages 1056–1066, 2017..More infoTop-tier conference in ML
- Zhang, C. (2018). Efficient active learning of sparse halfspaces. In Conference On Learning Theory, pages 1856–1880, 2018..More infoTop-tier conference in ML
- Zhang, C. (2015). Search Improves Label for Active Learning. In Advances in Neural Information Processing Systems, pages 3342–3350, 2016.More infoTop-tier conference in ML
- Zhang, C. (2016). The Extended Littlestone's Dimension for Learning with Mistakes and Abstentions. In Conference on Learning Theory, pages 1584– 1616, 2016..More infoTop-tier conference in ML
- Zhang, C. (2015). Active Learning from Weak and Strong Labelers. Advances in Neural Information Processing Systems, pages 703–711, 2015..More infoTop-tier ML conference
- Zhang, C. (2015). Spectral Learning of Large Structured HMMs for Comparative Epigenomics. Advances in Neural Information Processing Systems, pages 469–477, 2015..More infoTop-tier ML conference
- Zhang, C. (2014). Beyond Disagreement-based Agnostic Active Learning. Advances in Neural Information Processing Systems, pages 442–450, 2014..More infoTop-tier ML conference
Proceedings Publications
- Shen, J., & Zhang, C. (2021, March). Attribute-Efficient Learning of Halfspaces with Malicious Noise: Near-Optimal Label Complexity and Noise Tolerance. In International Conference on Algorithmic Learning Theory (ALT) 2021.More infoALT is a CORE2020 B conference.This paper is concerned with computationally efficient learning ofhomogeneous sparse halfspaces in $\mathbb{R}^d$ under noise. Though recentworks have established attribute-efficient learning algorithms under varioustypes of label noise (e.g. bounded noise), it remains an open question when andhow $s$-sparse halfspaces can be efficiently learned under the challengingmalicious noise model, where an adversary may corrupt both the unlabeledexamples and the labels. We answer this question in the affirmative bydesigning a computationally efficient active learning algorithm withnear-optimal label complexity of $\tilde{O}\big({s \log^4 \frac d \epsilon}\big)$ and noise tolerance $\eta = \Omega(\epsilon)$, where $\epsilon \in (0,1)$ is the target error rate, under the assumption that the distribution over(uncorrupted) unlabeled examples is isotropic log-concave. Our algorithm can bestraightforwardly tailored to the passive learning setting, and we show thatthe sample complexity is $\tilde{O}\big({\frac 1 \epsilon s^2 \log^5 d} \big)$which also enjoys the attribute efficiency. Our main techniques includeattribute-efficient paradigms for instance reweighting and for empirical riskminimization, and a new analysis of uniform concentration for unbounded data --all of them crucially take the structure of the underlying halfspace intoaccount.[Journal_ref: ]
- Zhang, C. (2021). Active Online Learning with Hidden Shifting Domains. In International Conference on Artificial Intelligence and Statistics (AISTATS) 2021.More infoOnline machine learning systems need to adapt to domain shifts. Meanwhile,acquiring label at every timestep is expensive. We propose a surprisinglysimple algorithm that adaptively balances its regret and its number of labelqueries in settings where the data streams are from a mixture of hiddendomains. For online linear regression with oblivious adversaries, we provide atight tradeoff that depends on the durations and dimensionalities of the hiddendomains. Our algorithm can adaptively deal with interleaving spans of inputsfrom different domains. We also generalize our results to non-linear regressionfor hypothesis classes with bounded eluder dimension and adaptive adversaries.Experiments on synthetic and realistic datasets demonstrate that our algorithmachieves lower regret than uniform queries and greedy queries with equallabeling budget.[Journal_ref: ]
- Zhang, C. (2021). Improved Algorithms for Efficient Active Learning Halfspaces with Massart and Tsybakov noise. In Proceedings of Conference on Learning Theory (COLT) 2021.More infoWe give a computationally-efficient PAC active learning algorithm for$d$-dimensional homogeneous halfspaces that can tolerate Massart noise (Massartand N\'ed\'elec, 2006) and Tsybakov noise (Tsybakov, 2004). Specialized to the$\eta$-Massart noise setting, our algorithm achieves aninformation-theoretically near-optimal label complexity of $\tilde{O}\left(\frac{d}{(1-2\eta)^2} \mathrm{polylog}(\frac1\epsilon) \right)$ under a widerange of unlabeled data distributions (specifically, the family of "structureddistributions" defined in Diakonikolas et al. (2020)). Under the morechallenging Tsybakov noise condition, we identify two subfamilies of noiseconditions, under which our efficient algorithm provides label complexityguarantees strictly lower than passive learning algorithms.[Journal_ref: ]
- Zhang, C. (2021). Multitask Bandit Learning Through Heterogeneous Feedback Aggregation. In International Conference on Artificial Intelligence and Statistics (AISTATS) 2021, 1531-1539.More infoIn many real-world applications, multiple agents seek to learn how to performhighly related yet slightly different tasks in an online bandit learningprotocol. We formulate this problem as the $\epsilon$-multi-player multi-armedbandit problem, in which a set of players concurrently interact with a set ofarms, and for each arm, the reward distributions for all players are similarbut not necessarily identical. We develop an upper confidence bound-basedalgorithm, RobustAgg$(\epsilon)$, that adaptively aggregates rewards collectedby different players. In the setting where an upper bound on the pairwisesimilarities of reward distributions between players is known, we achieveinstance-dependent regret guarantees that depend on the amenability ofinformation sharing across players. We complement these upper bounds withnearly matching lower bounds. In the setting where pairwise similarities areunknown, we provide a lower bound, as well as an algorithm that trades offminimax regret guarantees for adaptivity to unknown similarity structure.[Journal_ref: In International Conference on Artificial Intelligence and Statistics (pp. 1531-1539). PMLR (2021, March)]
- Zhang, C., & Wang, Z. (2021). Provably Efficient Multi-Task Reinforcement Learning with Model Transfer. In Proceeding of Neural Information Processing Systems (NeurIPS) 2021.More infoWe study multi-task reinforcement learning (RL) in tabular episodic Markovdecision processes (MDPs). We formulate a heterogeneous multi-player RLproblem, in which a group of players concurrently face similar but notnecessarily identical MDPs, with a goal of improving their collectiveperformance through inter-player information sharing. We design and analyze analgorithm based on the idea of model transfer, and provide gap-dependent andgap-independent upper and lower bounds that characterize the intrinsiccomplexity of the problem.[Journal_ref: ]
- Jun, K., & Zhang, C. (2020, December). Crush Optimism with Pessimism: Structured Bandits Beyond Asymptotic Optimality.. In Neural Information Processing Systems.More infoNeurIPS is a top-tier conference (A* in CORE).
- Zhang, C. (2020, April). Deep Batch Active Learning by Diverse, Uncertain Gradient Lower Bounds. In International Conference on Learning Representations, 2020..More infoTop tier ML conference
- Zhang, C. (2020, December). Efficient Contextual Bandits with Continuous Actions. In Advances in Neural Information Processing Systems (NeurIPS 2020).More infoNeurIPS is a CORE A* conference.We create a computationally tractable algorithm for contextual bandits withcontinuous actions having unknown structure. Our reduction-style algorithmcomposes with most supervised learning representations. We prove that it worksin a general sense and verify the new functionality with large-scaleexperiments.[Journal_ref: ]
- Zhang, C. (2020, December). Efficient active learning of sparse halfspaces with arbitrary bounded noise. In Advances in Neural Information Processing Systems (NeurIPS 2020).More infoNeurIPS is a CORE A* conference. Our work is accepted for oral presentation, which is top 5% of the accepted papers.We study active learning of homogeneous $s$-sparse halfspaces in$\mathbb{R}^d$ under label noise. Even in the presence of mild label noise thisis a challenging problem and only recently have label complexity bounds of theform $\tilde{\mathcal{O}} (s \cdot \mathrm{polylog}(d, \frac{1}{\epsilon}) )$been established in \cite{zhang2018efficient} for computationally efficientalgorithms under the broad class of isotropic log-concave distributions. Incontrast, under high levels of label noise, the label complexity boundsachieved by computationally efficient algorithms are much worse. When the labelnoise satisfies the {\em Massart} condition \cite{massart2006risk}, i.e., eachlabel is flipped with probability at most $\eta$ for a parameter $\eta \in\big[0, \frac12\big)$, state-of-the-art result \cite{awasthi2016learning}provides a computationally efficient active learning algorithm under isotropiclog-concave distributions with label complexity$\tilde{\mathcal{O}}(s^{\mathrm{poly}({1/(1-2\eta)})} \mathrm{poly}(\ln d,\frac{1}{\epsilon}) )$, which is label-efficient only when the noise rate$\eta$ is a constant. In this work, we substantially improve on it by designinga polynomial time algorithm for active learning of $s$-sparse halfspaces underbounded noise and isotropic log-concave distributions, with a label complexityof $\tilde{\mathcal{O}}\Big(\frac{s}{(1-2\eta)^4} \mathrm{polylog} (d, \frac 1\epsilon) \Big)$. This is the first efficient algorithm with label complexitypolynomial in $\frac{1}{1-2\eta}$ in this setting, which is label-efficienteven for $\eta$ arbitrarily close to $\frac12$. Our guarantees also immediatelytranslate to new state-of-the-art label complexity results for full-dimensionalactive and passive halfspace learning under arbitrary bounded noise andisotropic log-concave distributions.[Journal_ref: ]
- Zhang, C. (2019, June). Contextual Bandits with Continuous Actions: Smoothing, Zooming, and Adapting. In In Proceedings of the Thirty-Second Conference on Learning Theory, volume 99 of Proceedings of Machine Learning Research, pages 2025–2027. PMLR, 2019..More infoTop-tier conference in ML
Presentations
- Zhang, C. (2021). Efficient active learning of halfspaces: noise tolerance and exploiting sparsity. Arizona State University Machine Learning Day.More infoa research talk given in the workshop.
Others
- Zhang, C., & Jun, K. (2020, July). Crush Optimism with Pessimism: Structured Bandits Beyond Asymptotic Optimality.. ICML Workshop on Theoretical Foundations of Reinforcement Learning.More infoThis was a preliminary work that was presented and published in ICML Workshop on Theoretical Foundations of Reinforcement Learning.