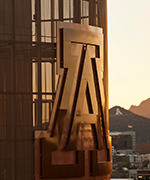
Kwang-Sung Jun
- Assistant Professor, Computer Science
- Member of the Graduate Faculty
- Assistant Professor, Statistics-GIDP
- Assistant Professor, Applied Mathematics - GIDP
Contact
- (520) 621-4632
- Gould-Simpson, Rm. 746
- Tucson, AZ 85721
- kjun@arizona.edu
Degrees
- Ph.D. Computer Science
- University of Wisconsin-Madison, Madison, Wisconsin, United States
- Some Machine Learning Methods from Sequential Input
- M.S. Computer Science
- University of Wisconsin-Madison, Madison, Wisconsin, United States
- B.S. Computer Science
- Soongsil University, Seoul, Korea, Republic of
Awards
- Top reviewer for AISTATS'22
- International Conference on Artificial Intelligence and Statistics (AISTATS), Spring 2022
- NeurIPS 2021 Outstanding Reviewer Award
- Neural Information Processing Systems, Fall 2021
Interests
Research
interactive machine learning, multi-armed bandits, online learning, active learning
Teaching
Machine learning, theory of machine learning, online learning (optimization), multi-armed bandits.
Courses
2024-25 Courses
-
Dissertation
CSC 920 (Spring 2025) -
Independent Study
CSC 599 (Spring 2025) -
Independent Study
CSC 699 (Spring 2025) -
Machine Learning Theory
CSC 588 (Spring 2025) -
Research
CSC 900 (Spring 2025) -
Dissertation
CSC 920 (Fall 2024) -
Independent Study
CSC 599 (Fall 2024) -
Principles of Machine Learning
CSC 480 (Fall 2024) -
Principles of Machine Learning
CSC 580 (Fall 2024) -
Research
CSC 900 (Fall 2024)
2023-24 Courses
-
Dissertation
CSC 920 (Spring 2024) -
Machine Learning Theory
CSC 588 (Spring 2024) -
Research
CSC 900 (Spring 2024) -
AdvTpc Artificial Intelligence
CSC 696H (Fall 2023) -
Research
CSC 900 (Fall 2023)
2022-23 Courses
-
Machine Learning Theory
CSC 588 (Spring 2023) -
Research
CSC 900 (Spring 2023) -
Principles of Data Science
CSC 380 (Fall 2022) -
Research
CSC 900 (Fall 2022)
2021-22 Courses
-
Principles of Data Science
CSC 380 (Spring 2022) -
Research
CSC 900 (Spring 2022) -
Principles of Machine Learning
CSC 580 (Fall 2021) -
Research
CSC 900 (Fall 2021)
2020-21 Courses
-
Research
CSC 900 (Spring 2021) -
Independent Study
CSC 599 (Fall 2020) -
Principles of Machine Learning
CSC 580 (Fall 2020)
2019-20 Courses
-
Adv Tpcs Computat Intell
CSC 665 (Spring 2020) -
Independent Study
CSC 599 (Fall 2019)
Scholarly Contributions
Journals/Publications
- Orabona, F., & Jun, K. (2021). Tight Concentrations and Confidence Sequences from the Regret of Universal Portfolio. arXiv preprint arXiv:2110.14099.
Proceedings Publications
- Bian, J., & Jun, K. (2022). Maillard Sampling: Boltzmann Exploration Done Optimally. In Proceedings of the International Conference on Artificial Intelligence and Statistics (AISTATS).More infoCORE A
- Faury, L., Abeille, M., Jun, K., & Calauz`{e}nes, C. (2022). Jointly Efficient and Optimal Algorithms for Logistic Bandits. In Proceedings of the International Conference on Artificial Intelligence and Statistics (AISTATS).More infocore A
- Gales, S. B., Sethuraman, S., & Jun, K. (2022). Norm-Agnostic Linear Bandits. In Proceedings of the International Conference on Artificial Intelligence and Statistics (AISTATS).More infocore A
- Kim, Y., Yang, I., & Jun, K. (2022). Improved regret analysis for variance-adaptive linear bandits and horizon-free linear mixture mdps. In submitted to Proceedings of the International Conference on Machine Learning (ICML).
- Mason, B., Jun, K., & Jain, L. (2022). An Experimental Design Approach for Regret Minimization in Logistic Bandits. In AAAI Conference on Artificial Intelligence (AAAI).More infocore A*
- Jang, K., Jun, K., Yun, S. Y., & Kang, W. (2021). Improved Regret Bounds of Bilinear Bandits using Action Space Dimension Analysis. In Proceedings of the International Conference on Machine Learning (ICML).More infocore A*
- Jun, K., Jain, L., Mason, B., & Nassif, H. (2021). Improved Confidence Bounds for the Linear Logistic Model and Applications to Linear Bandits. In Proceedings of the International Conference on Machine Learning (ICML).More infocore A*
- Park, H., Shin, S., Jun, K., & Ok, J. (2021). Transfer Learning in Bandits with Latent Continuity. In IEEE International Symposium on Information Theory (ISIT).More infoCORE B
- Jun, K., Zhang, C., Zhang, C., & Jun, K. (2020, December). Crush Optimism with Pessimism: Structured Bandits Beyond Asymptotic Optimality.. In Neural Information Processing Systems.More infoNeurIPS is a top-tier conference (A* in CORE).
- Jun, K., & Orabona, F. (2019, Dec). Parameter-Free Locally Differentially Private Stochastic Subgradient Descent. In NeurIPS Workshop on Privacy in Machine Learning (PriML).
- Jun, K., & Orabona, F. (2019, Jun). Parameter-Free Online Convex Optimization with Sub-Exponential Noise. In Proceedings of the Conference on Learning Theory, 99.More infoCOLT is a top-tier conference (A* in CORE).
- Jun, K., Cutkosky, A., & Orabona, F. (2019, Dec). Kernel Truncated Randomized Ridge Regression: Optimal Rates and Low Noise Acceleration. In Advances in Neural Information Processing Systems.More infoNeurIPS is a top-tier conference (A* in CORE).
- Jun, K., Willett, R., Wright, S., & Nowak, R. (2019, Jun). Bilinear Bandits with Low-rank Structure. In Proceedings of the 36th International Conference on Machine Learning, 97.More infoICML is a top-tier conference (A* in CORE).
Others
- Jun, K., & Zhang, C. (2020, July). Crush Optimism with Pessimism: Structured Bandits Beyond Asymptotic Optimality.. ICML Workshop on Theoretical Foundations of Reinforcement Learning.More infoThis was a preliminary work that was presented and published in ICML Workshop on Theoretical Foundations of Reinforcement Learning.