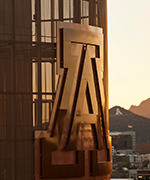
Mary M Poulton
- (520) 621-5292
- Mines And Metallurgy, Rm. 229
- Tucson, AZ 85721
- mpoulton@arizona.edu
Biography
Dr. Mary Poulton is a University Distinguished Professor with appointments in Geosciences, Mining and Geological Engineering, Law and Public Health. She was the first woman to lead an engineering department in the history of the University of Arizona, serving from July 1, 2000 to January 1, 2015. She founded and directs the interdisciplinary Lowell Institute for Mineral Resources (IMR), the largest minerals research and education center in the US. Dr. Poulton directs the Western Mine Safety and Health Training Resource Center. She created the Latin American Natural Resources Academy with US State Department support to promote student exchange in mineral resources. She is also a founding member of the Pan American Hub for Compatible Mining.
Dr. Poulton is co-founder and vice-president of NOAH, LLC, a water and energy management technology company and co-founder and principal of Custos Fratris L3C, a safety training company.
Dr. Poulton has more than 90 publications on the application of computational neural networks to pattern recognition problems in the earth sciences, including geophysics, mining, mineral and petroleum exploration, hydrology, and atmospheric science. She is the author of a book on the use of neural networks for geophysical data analysis. She has led or participated in nearly $29 million in research funding.
She has chaired the Mine Safety and Health Research Advisory Committee for NIOSH and the Board on Natural Resources for NASULGC (now APLU). Dr. Poulton was appointed to serve on three National Research Council Committees including co-authoring the 2007 report on critical minerals. She currently chairs the NRC Committee on Earth Resources and also serves on the Board on Earth Science and Resources. Dr. Poulton has given testimony before Congress on workforce issues in the mining industry and helped draft the Energy and Mineral Schools Re-Investment Act which passed the US House of Representatives in 2006.
Degrees
- Ph.D. Geological Engineering
- University of Arizona, Tucson, Arizona
Work Experience
- University of Arizona, Tucson, Arizona (2010 - Ongoing)
- Lowell Institute for Mineral Resources (2009 - Ongoing)
- University of Arizona, Tucson, Arizona (2003 - 2010)
- Mining and Geological Engineering (2000 - 2014)
- University of Arizona, Tucson, Arizona (1996 - 2003)
- University of Arizona, Tucson, Arizona (1990 - 1996)
- Pittsburgh and Midway Coal (1984)
- UA Army Corps of Engineers (1982 - 1983)
- US Army Corps of Engineers (1980 - 1981)
Awards
- Carnegie Professor of Year
- Carnegie Foundation, Summer 1999 (Award Nominee)
- Wakonse Fellow
- UA, Spring 1999
- Excellence at the Student Interface
- MGE Department, Spring 1998
- MGE Department, Spring 2011
- Who's Who
- Marquis, Fall 1997
- Strathmore, Fall 1997
- Outstanding Advisor
- Center for Off Campus Students, Spring 1994
- Ivan B. Rahn Award
- SME, Spring 2015
- Eugene Sander Faculty Fundraising Award
- UA Foundation, Fall 2012
- SME Distinguished Member
- SME, Spring 2012
- University Research Innovation Award
- University of Arizona, Spring 2011
- American Hall of Fame Medal of Merit
- Mining Foundation of the Southwest, Fall 2010
- Stephen McCann Award for Excellence in Education
- Pittsburgh Coal Mining Assocation, Fall 2010
- University Distinguished Professor
- University of Arizona, Fall 2010
- AIME Industry Educator Award
- AIME, Spring 2010
- Udall Center For Public Policy Fellow
- Udall Center for Public Policy Studies, Spring 2008
- Distinguished Lecturer
- Arizona Conference of SME, Fall 2006
- Woman of Distinction Award
- Business and Professional Women, Spring 2006
Licensure & Certification
- EIT (1987)
Interests
Research
Sustainable Resource Development, Mining Health and Safety, Environmental issues in mining
Courses
2020-21 Courses
-
Intro to International Mining
LAW 640G (Fall 2020)
2019-20 Courses
-
Intro to International Mining
LAW 640G (Fall 2019)
2018-19 Courses
-
Intro to International Mining
LAW 640G (Fall 2018)
2017-18 Courses
-
Dissertation
MNE 920 (Spring 2018) -
Int'l Mining Health Law
LAW 640E (Spring 2018) -
Intro to International Mining
LAW 640G (Fall 2017)
2016-17 Courses
-
Dissertation
MNE 920 (Spring 2017) -
Independent Study
MNE 699 (Spring 2017) -
Intro to International Mining
LAW 640G (Spring 2017) -
Dissertation
MNE 920 (Fall 2016) -
Intro to International Mining
LAW 640G (Fall 2016) -
Research
MNE 900 (Fall 2016) -
Thesis
MNE 910 (Fall 2016)
2015-16 Courses
-
Dissertation
MNE 920 (Spring 2016)
Scholarly Contributions
Journals/Publications
- Botin, J., Gatica, C., Poulton, M. M., & Tenorio Gutierrez, V. O. (2016). Profesionales Femeninas en la Minera Chilena - Female Professionals in Chilean Mining. TECNOLOGIA MINERA, 10(62), 76-80.More infoThe Chilean minerals industry faces a shortage of 27.000 new skilled professional by 2023. Enhancing the integration of women into the workforce is a possible solution. This study analyzes the state of women in the mining workforce over the past decade. Several key indicators are presented: percent in the workforce, salaries, career profiles, presence at management level, and participation in academia are studied. The study also analyzes the strategies used by government, industry, and universities to recruit and retain women for the minerals industy. The main results show that women represent 7.4% of the mining workforce in Chile and less than 1% of senior positions with a salary gap of approximately 30%. Systematically, women prefer professional programs in geology, chemistry, and environmental areas. The Chilean statistics are compared to data from the United States mining industry.
- Maier, R. M., Diaz-Barriga, F., Field, J. A., Hopkins, J. C., Klein, B., & Poulton, M. M. (2014). Socially responsible mining: the relationship between mining and poverty, human health and the environment. Reviews on Environmental Health, 29, 83-89.
- King, A., Poate, J., Poulton, M. M., Duclos, S., Espinal, L., Traversa, E., Mao, S. S., & Baraton, M. (2013). Materials Research Society Symposium Proceedings: Preface. Materials Research Society Symposium Proceedings, 1492, ix.
- Poulton, M. M., & Tenorio Gutierrez, V. O. (2013). De Nanoescala a Terabytes (parte 2) - From Nanoscale to Terabytes (part 2). TECNOLOGIA MINERA, 7(42), 62-64.More infoThe Lowell Institute of Mineral Resources (IMR) was created for covering the need for interdisciplinary research in mineral resources and to provide continuous education for professionals. Research projects range from fundamental geology to ethics and sustainable development.
- Poulton, M. M., Jagers, S. C., Linde, S., Zyl, D. V., Danielson, L. J., & Matti, S. (2013). State of the world's nonfuel mineral resources: Supply, demand, and socio-institutional fundamentals. Annual Review of Environment and Resources, 38, 345-371.More infoAbstract: Current material supply-demand imbalances are driven by situational rather than physical scarcities, resulting in a growing interest among government, civil society, and industry groups to consider not only the availability of mineral resources, but also the sustainability implications of their production. This, in turn, places increasing pressure on mining companies to broaden their concerns when planning new mining projects, covering its "social license to operate" by incorporating strategies for limiting negative socioenvironmental impacts alongside calculations of the project's economic viability as well as balancing a large number of potential stakeholders. Accordingly, understanding also the sociopolitical context of mineral development is crucial for the creation of sustainable practices within the mining industry. By applying a sustainable development framework, this article outlines the complex web of challenges associated with sustainable mineral extraction, ranging from technological and economic development to political and institutional concerns on how to govern and manage scarce resources in a globalized world. © 2013 by Annual Reviews. All rights reserved.
- Poulton, M. M., Poulton, M. M., Tenorio Gutierrez, V. O., Tenorio Gutierrez, V. O., Poulton, M. M., & Tenorio Gutierrez, V. O. (2013). De Nanoescala a Terabytes (parte 1) - From Nanoscale to Terabytes (part 1). TECNOLOGIA MINERA, 7(41), 134-136.More infoIntegrating interdisciplinary research in mining technology.
- Poulton, M., Szidarovszky, F., Coppola, E. A., Long, J., Hall, A. D., & Poulton, M. M. (0). A hybrid artificial neural network-numerical model for ground water problems. Ground water, 45(5).More infoNumerical models constitute the most advanced physical-based methods for modeling complex ground water systems. Spatial and/or temporal variability of aquifer parameters, boundary conditions, and initial conditions (for transient simulations) can be assigned across the numerical model domain. While this constitutes a powerful modeling advantage, it also presents the formidable challenge of overcoming parameter uncertainty, which, to date, has not been satisfactorily resolved, inevitably producing model prediction errors. In previous research, artificial neural networks (ANNs), developed with more accessible field data, have achieved excellent predictive accuracy over discrete stress periods at site-specific field locations in complex ground water systems. In an effort to combine the relative advantages of numerical models and ANNs, a new modeling paradigm is presented. The ANN models generate accurate predictions for a limited number of field locations. Appending them to a numerical model produces an overdetermined system of equations, which can be solved using a variety of mathematical techniques, potentially yielding more accurate numerical predictions. Mathematical theory and a simple two-dimensional example are presented to overview relevant mathematical and modeling issues. Two of the three methods for solving the overdetermined system achieved an overall improvement in numerical model accuracy for various levels of synthetic ANN errors using relatively few constrained head values (i.e., cells), which, while demonstrating promise, requires further research. This hybrid approach is not limited to ANN technology; it can be used with other approaches for improving numerical model predictions, such as regression or support vector machines (SVMs).
- Poulton, M. M. (2012). Analysis of the mining engineering faculty pipeline. 2012 SME Annual Meeting and Exhibit 2012, SME 2012, Meeting Preprints, 427-432.More infoAbstract: Universities are not immune from the large number of retirements facing the mining industry. Department heads anticipate that we will need to replace all of the senior mining engineering faculty in the U.S. by 2020. Long term studies of Ph.D. completion times and success rates indicate that in Engineering disciplines in the U.S., the ten year completion rate is 65% at best and the four year completion rate is less than 8%. We do not have accurate data on completion rates and times for mining or metallurgical engineering Ph.D. students in the U.S. but students self-report their expected completion times are around 4 years. National data on doctoral students indicate that younger, full time students with sufficient financial support have a higher probability of success in completing their degrees in a timely manner. Students in Eastern U.S. mining engineering programs are on average 32 years old, 85% are full time, and the gap between support and cost of attendance is $5,000 per year. Students in Western U.S. programs are on average 38 years old, 78% are full time, and the financial gap is $8,483. Further, it appears that domestic graduate students are not very geographically mobile. The current demand for new faculty is higher in Western programs but the longer term retirements are higher in Eastern programs. While all Ph.D. students who responded to the 2010 survey indicated an interest in a faculty position at some point in their careers, very few were applying for the open positions. Over the past 35 years for which data could be obtained, the maximum number of Ph.D. students enrolled in mining engineering in the U.S. is 115 with a maximum of 27 degrees granted in one year. On average 89 students are enrolled in doctoral programs in mining engineering in the U.S. with average degree production of 15 Ph.D.s per year. Copyright © 2012 by SME.
- Hinnell, A. C., Lazarovitch, N., Furman, A., Poulton, M., & Warrick, A. W. (2010). Neuro-Drip: Estimation of subsurface wetting patterns for drip irrigation using neural networks. Irrigation Science, 28(6), 535-544.More infoAbstract: Design of efficient drip irrigation systems requires information about the subsurface water distribution of added water during and after infiltration. Further, this information should be readily accessible to design engineers and practitioners. Neuro-Drip combines an artificial neural network (ANN) with a statistical description of the spatio-temporal distribution of the added water from a single drip emitter to provide easily accessible, rapid illustrations of the spatial and temporal subsurface wetting patterns. In this approach, the ANN is an approximator of a flow system. The ANN is trained using close to 1,000 numerical simulations of infiltration. Moment analysis is used to encapsulate the spatial distribution of water content. In practice, the user provides soil hydraulic properties and discharge rate; the ANN is then used to estimate the depth to the center of mass of the added water, and the vertical and radial spreading around the center of mass; finally, this statistical description of the added water is used to visualize the fate of the added water during and after the infiltration event. © 2010 Springer-Verlag.
- Snyder, D. D., Prouty, M., George, D. C., King, T., Poulton, M., & Szidarovszky, A. (2010). Uxo discrimination at camp san luis obispo with the metalmapper. Proceedings of the Symposium on the Application of Geophyics to Engineering and Environmental Problems, SAGEEP, 2, 1054-1064.More infoAbstract: The MetalMapper is an electromagnetic metal detector that is being commercialized by Geometrics, Inc with support from the Environmental Security Technology Certification Program (ESTCP). Together with several other systems, the MetalMapper participated in an ESTCP-sponsored classification study at the former Camp San Luis Obispo (SLO) located a few miles northwest of San Luis Obispo, CA. Operating independently, the MetalMapper conducted two surveys: a dynamic mapping survey for target detection; a precision (Cued ID) static measurement over detected targets for use in discrimination. The SLO study involved a number of different organizations deploying both commercially available EM and magnetic sensors and advanced EM systems such as the MetalMapper. Following the completion of the field work by these organizations, nearly 2000 targets identified from the detection surveys were then dug. Using a common set of targets, demonstrators prepared a dig list prioritized according to the probability that a particular target was a target of interest (TOI). The dig lists were scored by the Institute for Defense Analyses (IDA). The primary objective of this paper is to describe MetalMapper system, the data processing, and the methodology employed for assembling the prioritized dig list. We also present the scoring results for the MetalMapper as provided to us by the IDA.
- Lazarovitch, N., Poulton, M., Furman, A., & Warrick, A. W. (2009). Water distribution under trickle irrigation predicted using artificial neural networks. Journal of Engineering Mathematics, 64(2), 207-218.More infoAbstract: An artificial neural network (ANN) technology is presented as an alternative to physical-based modeling of subsurface water distribution from trickle emitters. Three options are explored to prepare input-output functional relations from a database created using a numerical model (HYDRUS-2D). From the database the feasibility and advantages of the three alternative options are evaluated: water-content at defined coordinates, moment analysis describing the shape of the plume, and coordinates of individual water-content contours. The best option is determined in a way by the application objectives, but results suggest that prediction using moment analyses is probably the most versatile and robust and gives an adequate picture of the subsurface distribution. Of the other two options, the direct determination of the individual water contours was subjectively judged to be more successful than predicting the water content at given coordinates, at least in terms of describing the subsurface distribution. The results can be used to estimate subsurface water distribution for essentially any soil properties, initial conditions or flow rates for trickle sources. © Springer Science+Business Media B.V. 2009.
- Szidarovszky, A., Poulton, M., & MacInnes, S. (2008). Identification of unexploded ordnance from clutter using neural networks. SEG Technical Program Expanded Abstracts, 27(1), 2912-2916.More infoAbstract: The largest costs associated with subsurface Unexploded Ordnance (UXO) remediation are associated with removing non-UXO debris. Discrimination between UXO and non-UXO is important for both cost and safety reasons. A neural network was developed to distinguish between UXO and non-UXO clutter using Time Domain Electromagnetic Method (TEM) data. There are two stages for the learning process of neural network: training and testing. A synthetic dataset was created using actual acquisition configurations, with varying amounts of random noise. This dataset included 934 UXO targets representing 7 different UXO types, and 789 clutter objects based on four templates with varying size and random asymmetry. The results show 97% accuracy for correctly classifying clutter, and 97% accuracy for correctly classifying UXO.
- Szidarovszky, A., Poulton, M., & Macinnes, S. C. (2008). Identification of unexploded ordnance from clutter using neural neworks. Environmental and Engineering Geophysical Society - 21st Symposium on the Application of Geophysics to Engineering and Environmental Problems 2008, 1, 525-535.More infoAbstract: The largest costs associated with subsurface Unexploded Ordnance (UXO) remediation are associated with removing non-UXO. Discrimination between UXO and non-UXO is important for both cost and safety reasons. A neural network was developed to distinguish between UXO and non-UXO clutter using TEM data. There are two stages for the learning process of neural network, training and validation. A synthetic dataset was created using actual acquisition configurations, with varying amounts of random noise. This dataset included 934 UXO targets representing 7 different UXO types, and 789 clutter objects based on four templates with varying size and random asymmetry. The results show 97% accuracy for correctly classifying clutter, and 97% accuracy for correctly classifying UXO. The level of success for classification is based on the classification Receiver Operating Characteristic (ROC) curves. The ROC curve represents the relationship between UXO classified correctly (Hit rate) versus clutter miss classified (False alarm).
- Coppola Jr., E. A., Szidarovszky, F., Davis, D., Spayd, S., Poulton, M. M., & Roman, E. (2007). Multiobjective analysis of a public wellfield using artificial neural networks. Ground Water, 45(1), 53-61.More infoPMID: 17257339;Abstract: As competition for increasingly scarce ground water resources grows, many decision makers may come to rely upon rigorous multiobjective techniques to help identify appropriate and defensible policies, particularly when disparate stakeholder groups are involved. In this study, decision analysis was conducted on a public water supply wellfield to balance water supply needs with well vulnerability to contamination from a nearby ground water contaminant plume. With few alternative water sources, decision makers must balance the conflicting objectives of maximizing water supply volume from noncontaminated wells while minimizing their vulnerability to contamination from the plume. Artificial neural networks (ANNs) were developed with simulation data from a numerical ground water flow model developed for the study area. The ANN-derived state transition equations were embedded into a multiobjective optimization model, from which the Pareto frontier or trade-off curve between water supply and wellfield vulnerability was identified. Relative preference values and power factors were assigned to the three stakeholders, namely the company whose waste contaminated the aquifer, the community supplied by the wells, and the water utility company that owns and operates the wells. A compromise pumping policy that effectively balances the two conflicting objectives in accordance with the preferences of the three stakeholder groups was then identified using various distance-based methods. © 2006 National Ground Water Association.
- Szidarovszky, F., Coppola Jr., E. A., Long, J., Hall, A. D., & Poulton, M. M. (2007). A hybrid artificial neural network-numerical model for ground water problems. Ground Water, 45(5), 590-600.More infoPMID: 17760585;Abstract: Numerical models constitute the most advanced physical-based methods for modeling complex ground water systems. Spatial and/or temporal variability of aquifer parameters, boundary conditions, and initial conditions (for transient simulations) can be assigned across the numerical model domain. While this constitutes a powerful modeling advantage, it also presents the formidable challenge of overcoming parameter uncertainty, which, to date, has not been satisfactorily resolved, inevitably producing model prediction errors. In previous research, artificial neural networks (ANNs), developed with more accessible field data, have achieved excellent predictive accuracy over discrete stress periods at site-specific field locations in complex ground water systems. In an effort to combine the relative advantages of numerical models and ANNs, a new modeling paradigm is presented. The ANN models generate accurate predictions for a limited number of field locations. Appending them to a numerical model produces an overdetermined system of equations, which can be solved using a variety of mathematical techniques, potentially yielding more accurate numerical predictions. Mathematical theory and a simple two-dimensional example are presented to overview relevant mathematical and modeling issues. Two of the three methods for solving the overdetermined system achieved an overall improvement in numerical model accuracy for various levels of synthetic ANN errors using relatively few constrained head values (i.e., cells), which, while demonstrating promise, requires further research. This hybrid approach is not limited to ANN technology; it can be used with other approaches for improving numerical model predictions, such as regression or support vector machines (SVMs). © 2007 National Ground Water Association.
- Coppola Jr., E. A., McLane, C. F., Poulton, M. M., Szidarovszky, F., & Magelky, R. D. (2005). Predicting conductance due to upconing using neural networks. Ground Water, 43(6), 827-836.More infoPMID: 16324004;Abstract: Artificial neural networks (ANNs) were developed to accurately predict highly time-variable specific conductance values in an unconfined coastal aquifer. Conductance values in the fresh water lens aquifer change in response to vertical displacements of the brackish zone and fresh water-salt water interface, which are caused by variable pumping and climate conditions. Unlike physical-based models, which require hydrologic parameter inputs, such as horizontal and vertical hydraulic conductivities, porosity, and fluid densities, ANNs can "learn" system behavior from easily measurable variables. In this study, the ANN input predictor variables were initial conductance, total precipitation, mean daily temperature, and total pumping extraction. The ANNs were used to predict salinity (specific conductance) at a single monitoring well located near a high-capacity municipal-supply well over time periods ranging from 30 d to several years. Model accuracy was compared against both measured/ interpolated values and predictions were made with linear regression, and in general, excellent prediction accuracy was achieved. For example, although the average percent change of conductance over 90-d periods was 39%, the absolute mean prediction error achieved with the ANN was only 1.1%. The ANNs were also used to conduct a sensitivity analysis that quantified the importance of each of the four predictor variables on final conductance values, providing valuable insights into the dynamics of the system. The results demonstrate that the ANN technology can serve as a powerful and accurate prediction and management tool, minimizing degradation of ground water quality to the extent possible by identifying appropriate pumping policies under variable and/or changing climate conditions. Copyright © 2005 National Ground Water Association.
- Coppola Jr., E. A., Rana, A. J., Poulton, M. M., Szidarovszky, F., & Uhl, V. W. (2005). A neural network model for predicting aquifer water level elevations. Ground Water, 43(2), 231-241.More infoPMID: 15819944;Abstract: Artificial neural networks (ANNs) were developed for accurately predicting potentiometric surface elevations (monitoring well water level elevations) in a semiconfined glacial sand and gravel aquifer under variable state, pumping extraction, and climate conditions. ANNs "learn" the system behavior of interest by processing representative data patterns through a mathematical structure analogous to the human brain. In this study, the ANNs used the initial water level measurements, production well extractions, and climate conditions to predict the final water level elevations 30 d into the future at two monitoring wells. A sensitivity analysis was conducted with the ANNs that quantified the importance of the various input predictor variables on final water level elevations. Unlike traditional physical-based models, ANNs do not require explicit characterization of the physical system and related physical data. Accordingly, ANN predictions were made on the basis of more easily quantifiable, measured variables, rather than physical model input parameters and conditions. This study demonstrates that ANNs can provide both excellent prediction capability and valuable sensitivity analyses, which can result in more appropriate ground water management strategies. Copyright © 2005 National Ground Water Association.
- Coppola Jr., E., Poulton, M., Charles, E., Dustman, J., & Szidarovszky, F. (2003). Application of artificial neural networks to complex groundwater management problems. Natural Resources Research, 12(4), 303-320.More infoAbstract: As water quantity and quality problems become increasingly severe, accurate prediction and effective management of scarcer water resources will become critical. In this paper, the successful application of artificial neural network (ANN) technology is described for three types of groundwater prediction and management problems. In the first example, an ANN was trained with simulation data from a physically based numerical model to predict head (groundwater elevation) at locations of interest under variable pumping and climate conditions. The ANN achieved a high degree of predictive accuracy, and its derived state-transition equations were embedded into a multiobjective optimization formulation and solved to generate a tradeoff curve depicting water supply in relation to contamination risk. In the second and third examples, ANNs were developed with real-world hydrologic and climate data for different hydrogeologic environments. For the second problem, an ANN was developed using data collected for a 5-year, 8-month period to predict heads in a multilayered surficial and limestone aquifer system under variable pumping, state, and climate conditions. Using weekly stress periods, the ANN substantially outperformed a well-calibrated numerical flow model for the 71-day validation period, and provided insights into the effects of climate and pumping on water levels. For the third problem, an ANN was developed with data collected automatically over a 6-week period to predict hourly heads in 11 high-capacity public supply wells tapping a semiconfined bedrock aquifer and subject to large well-interference effects. Using hourly stress periods, the ANN accurately predicted heads for 24-hour periods in all public supply wells. These test cases demonstrate that the ANN technology can solve a variety of complex groundwater management problems and overcome many of the problems and limitations associated with traditional physically based flow models. © 2003.
- Coppola Jr., E., Szidarovszky, F., Poulton, M., & Charles, E. (2003). Artificial neural network approach for predicting transient water levels in a multilayered groundwater system under variable state, pumping, and climate conditions. Journal of Hydrologic Engineering, 8(6), 348-360.More infoAbstract: The feasibility of training an artificial neural network (ANN) for accurately predicting transient water levels in a complex multilayered ground-water system under variable state, pumping, and climate conditions is demonstrated. Using real-world data, an ANN was developed for a public supply wellfield and ground-water monitoring network located near Tampa Bay, Florida. The ANN was trained to predict transient water levels at 12 monitoring well locations screened in different aquifers in response to changing pumping and climate conditions. The trained ANN was then validated with ten sequential seven-day periods, and the results were compared against both measured and numerically simulated ground-water levels. The absolute mean error between the ANN predicted and the measured water levels is 0.16 m, compared to the 0.85 m absolute mean error achieved with the calibrated numerical model at the same locations over the same time period. The ANN also more closely reproduced the dynamic water level responses to pumping and climate conditions. The practical implication is that if ANN technology can achieve superior ground-water level predictions, it can be used to improve management strategies for a wide range of ground-water problems, from water quantity to water quality issues. It can also serve as a powerful sensitivity analysis tool for quantifying interrelationships between different variables, fostering a better understanding of the hydrogeologic system, and improving future modeling endeavors. And while physical-based numerical modeling retains some advantages over the ANN technology, both approaches may be used in a complementary fashion to achieve sound decision-making for complicated groundwater management problems.
- Poulton, M. M. (2002). Neural networks as an intelligence amplification tool: A review of applications. Geophysics, 67(3), 979-993.More infoAbstract: The sophisticated algorithms we use to process, analyze, and interpret geophysical data automate tasks we used to do by hand, transform data into domains where patterns are more obvious, and allow us to calculate quantities where we used to rely on intuition or back-of-envelope estimates. But, the crux of the exploration problem is still interpretation-associating abstract patterns with geologic formations of economic value. Artificial neural networks are able to couple the speed and efficiency of the computer with the pattern recognition and association capabilities of the brain to aid the exploration process. The key concept to understand in the application of neural network technology is that they should not be used as an artificial intelligence tool to replace a human interpreter; rather, neural networks are an intelligence amplification toolkit that allows the interpreter to focus on the important information. More than 102 neural network papers have been published by SEG since 1988, and more than 550 neural network papers pertaining to any aspect of geophysics were published in that same time period. Neural network applications in exploration geophysics can generally be divided into two eras. The focus through 1994 was largely on learning what neural networks could do with different data sets, and how to prepare data for them and analyze the results. Networks were usually trained with synthetic data and then tested with field data. The second era, from 1995 to the present, has focused on some specific application areas such as reservoir characterization. The current emphasis is to integrate neural networks within a comprehensive interpretation scheme instead of as a stand-alone application. Neural network technology has helped us turn data into information by allowing us to find nontrivial correlations between geophysical data and petrophysical properties; relate detailed changes in wavelet morphology to small-scale changes in lithology; and find features in the wavelets that allow us to locate first breaks, track horizons, identify gas chimneys, or trace faults; and attenuate multiples. As the science and engineering of data acquisition progresses, neural networks will play an increasingly vital role in helping us find relevant information in the vast streams of data under the constraints of lower costs, less time, and fewer people.
- Rucker, D. F., Ferré, T. P., & Poulton, M. (2002). A back propagation neural network for identifying first-break times on cross borehole ground penetrating radar traces. Proceedings of SPIE - The International Society for Optical Engineering, 4758, 630-634.More infoAbstract: Manually picking the first arrival of energy in a series of cross borehole GPR ray traces can be time consuming and subjective, especially when large data sets need to be processed. One possible remedy is the application of a back propagating neural network. Neural network applications have been used previously in seismic studies to pick the arrival of the P and S waves (Dai and MacBeth, 1997; McCormack et al. 1993; Murat et al. 1992). One particular method, which applied a moving window over the trace, is used here with slight modification. Noisy time-amplitude records were first normalized to range from -1 and 1. These data were then filtered such that values between -1 and a negative threshold were set to -1, values between 1 and a positive threshold were set to 1 and all other values were set to zero. The filtered wave was fed through a neural network that searched for a pattern related to a first arrival. Several filtering parameters were tested, including the size of the moving window, the values of the positive and negative thresholds, and neural network parameters pertaining to training and testing. With minimal training, the neural network performed very well compared to hand picking of arrival times on large data sets.
- Zhang, L., Poulton, M. M., & Wang, T. (2002). Borehole electrical resistivity modeling using neural networks. Geophysics, 67(6), 1790-1797.More infoAbstract: A neural network approach has been applied to model downhole resistivity tools, i.e., to generate a synthetic tool response for a given earth resistivity model. The microlaterolog (MLL), shallow dual laterolog (DLLs), and deep dual laterolog (DLLd) tools are modeled using neural networks to demonstrate this approach. Efforts have been made to select various neural network parameters, including the type of neural network, the length of input data for training, the number of hidden nodes, and the number of training samples. A modular neural network (MNN) has been selected because it can facilitate the training and prediction of tool responses in formations with large resistivity variations. The input data for training are taken to be the model formation resistivity values sampled over a depth window. The window length is chosen based on the tool lengths. Three different window lengths are used for experiments: 6.1, 9.1. and 30.5 m. We found the longer window lengths generally have higher modeling accuracy for the three different types of logging tools. The number of hidden nodes needed to yield satisfactory training and prediction data varies from 8 to 25, depending on the type of tool and the window length. Up to 30 000 training samples have been collected to train the MNN. Our modeling examples show that the trained MNN can achieve about 90% accuracy for the MLL log response and about 83% accuracy for the DLLs and DLLd responses. The modeling errors can be described roughly with a Gaussian distribution.
- Poulton, M. M. (2001). Chapter 1 A brief history. Handbook of Geophysical Exploration: Seismic Exploration, 30, 3-18.
- Poulton, M. M. (2001). Chapter 13 Non-seismic applications. Handbook of Geophysical Exploration: Seismic Exploration, 30, 219-233.
- Poulton, M. M. (2001). Chapter 2 Biological versus computational neural networks. Handbook of Geophysical Exploration: Seismic Exploration, 30, 19-25.
- Poulton, M. M. (2001). Chapter 3 Multi-layer perceptrons and back-propagation learning. Handbook of Geophysical Exploration: Seismic Exploration, 30, 27-53.
- Poulton, M. M. (2001). Chapter 4 Design of training and testing sets. Handbook of Geophysical Exploration: Seismic Exploration, 30, 55-65.
- Poulton, M. M. (2001). Chapter 5 Alternative architectures and learning rules. Handbook of Geophysical Exploration: Seismic Exploration, 30, 67-88.
- Poulton, M. M. (2001). Chapter 6 Software and other resources. Handbook of Geophysical Exploration: Seismic Exploration, 30, 89-98.
- Poulton, M. M. (2001). Preface. Handbook of Geophysical Exploration: Seismic Exploration, 30, xi-xii.
- Poulton, M. M., & Powell, K. S. (2001). Chapter 8 Rock mass and reservoir characterization. Handbook of Geophysical Exploration: Seismic Exploration, 30, 119-127.
- Buffenmyer, V., Poulton, M., & Johnson, R. (2000). Identification of seismic crew noise in marine surveys by neural networks. Leading Edge (Tulsa, OK), 19(4), 370, 372, 374, 376.More infoAbstract: A multi-layer feed-forward architecture with back-propagation learning was used to determine seismic crew noise. Testing was performed on the entire shot record using a sliding window. Two types of visual displays became important to the analysis of the network's results for testing upon shot records. The results indicate that a neural network can be successfully trained to identify seismic crew noise in marine surveys.
- Poulton, M. M., & Birken, R. A. (1998). Estimating one-dimensional models from frequency-domain electromagnetic data using modular neural networks. IEEE Transactions on Geoscience and Remote Sensing, 36(2), 547-555.More infoAbstract: An artificial neural network interpretation system is being used to interpret data from a frequency-domain electromagnetic (EM) geophysical system in near real time. The interpretation system integrates 45 separate networks in a data visualization shell. The networks produce interpretations at three different transmitter-receiver (Tx-Rx) separations for half-space and layered-earth interpretations. Modular neural networks (MNN's) were found to be the only paradigm that could successfully perform the layered-earth interpretations. An MNN with 16 inputs, five local experts, each with seven hidden processing elements, and three outputs was trained on 4795 patterns for 200 epochs. For two-layer models with a resistivity contrast greater than 2:1, resistivity estimates had greater than 96% accuracy for the first-layer resistivity, greater than 98% for the second-layer resistivity, and greater than 96% for the thickness of the first layer. If the contrast is less than 2:1, the resistivity accuracies are unaffected but thickness estimates for layers less than 2 m are unreliable. A Tx-Rx separation of 16 m with maximum depth of penetration of 8 m was assumed for the example cited.
- Poulton, M. M., & Birken, R. A. (1998). Estimating onedimensional models from frequencydomain electromagnetic data using modular neural networks. IEEE Transactions on Geoscience and Remote Sensing, 36(2), 547555-.More infoAbstract: An artificial neural network interpretation system is being used to interpret data from a frequencydomain electromagnetic (EM) geophysical system in near real time. The interpretation system integrates 45 separate networks in a data visualization shell. The networks produce interpretations at three different transmitterreceiver (TxRx) separations for halfspace and layeredearth interpretations. Modular neural networks (MNN's) were found to be the only paradigm that could successfully perform the layeredearth interpretations. An MNN with 16 inputs, five local experts, each with seven hidden processing elements, and three outputs was trained on 4795 patterns for 200 epochs. For twolayer models with a resistivity contrast greater than 2:1, resistivity estimates had greater than 96% accuracy for the firstlayer resistivity, greater than 98% for the secondlayer resistivity, and greater than 96% for the thickness of the first layer. If the contrast is less than 2:1, the resistivity accuracies are unaffected but thickness estimates for layers less than 2 m are unreliable. A TxRx separation of 16 m with maximum depth of penetration of 8 m was assumed for the example cited. © 1998 IEEE.
- Ashley, D., & Poulton, M. (1993). Geophysical target identification in environmental investigations. Intelligent Engineering Systems Through Artificial Neural Networks, 3, 903-908.More infoAbstract: A system of fully-connected, feedforward neural networks is being developed for environmental engineering applications. The networks will be used in conjunction with a high-frequency electromagnetic induction imaging system with a loop-loop transmitter and receiver, and a frequency range of 1 KHz-1 MHz. Input to the neural network system will be the elliptical polarization of the received magnetic field in three directions for 11 frequencies in the same frequency range. The goal is to produce a plausible interpretation of the data in real time so that the geophysical survey parameters can be adjusted. The system will be used in environmental investigations, from hazardous waste site investigations to the detection of voids in the subsurface.
- Kulatilake, P. H., Wathugala, D. N., Poulton, M., & Stephansson, O. (1990). Analysis of structural homogeneity of rock masses. Engineering Geology, 29(3), 195-211.More infoAbstract: The salient features and capabilities of the statistical tests suggested by Miller (1983) and Mahtab and Yegulalp (1984) to investigate structural homogeneity in rock masses are reviewed. The difficulty in making a decision about statistical homogeneity only through the results of any one of these two tests or through equal-area polar plots, is shown through a case study. New interpretations are suggested for these two tests to use them in assigning relative ranks for the strength of homogeneity of different regions in rock masses. An example is given to illustrate how one can use Miller's method with new interpretations along with equal-area plots in making decisions about statistical homogeneity in rock masses. The example also shows an attempt to incorporate results of Mahtab and Yegulalp's test with new interpretations to the decision-making process. © 1990.
- Poulton, M. M., Mojtabai, N., & Farmer, I. W. (1990). Scale invariant behaviour of massive and fragmented rock. International Journal of Rock Mechanics and Mining Sciences and, 27(3), 219-221.
Presentations
- Poulton, M. M. (2015, December). Successful Change Management for University Mineral Resources Programs. Business Process Improvement in Mining Conference. Tucson, AZ: BPI-Mining and SME.More infoKeynote speech
- Poulton, M. M. (2015, December). The New Face of Mining: The Culture Change for Compatible Mining. Arizona Conference of SME. Tucson, AZ: SME.More infoKeynote speech
- Poulton, M. M. (2015, February). How many anthropologists does it take to build a mine?. SME Annual Meeting. Denver: SME.
- Poulton, M. M. (2015, February). The New Face of Mining – Industry-University Research Partnerships. SME Annual meeting. Denver: SME.
- Poulton, M. M. (2015, July). Satisfying the Material World: The Challenges of the 21st Century. ASU Singulair Project. Phoenix, AZ: ASU.More infoInvited talk
- Poulton, M. M. (2015, October). Resourcing the Material World: Balancing Material and Soceital Demands. International Society of Exposure Science. Las Vegas: ISES.More infoKeynote Address
- Poulton, M. M., & Barton, M. D. (2015, november). AZ 101: Mineral Resources. tutorial on mining for staffs of city, county and mayoral officials. Tucson, AZ: UA State Relations.