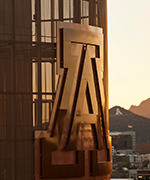
Pratik Chittaranjan Satam
- Assistant Professor, Systems and Industrial Engineering
- Member of the Graduate Faculty
- Assistant Professor, Electrical and Computer Engineering
Contact
- (520) 621-6548
- ENGINEERING, Rm. 221
- TUCSON, AZ 85721-0020
- pratiksatam@arizona.edu
Bio
No activities entered.
Interests
No activities entered.
Courses
2025-26 Courses
-
SW for Industrial Control Syst
SFWE 411 (Fall 2025) -
SW for Industrial Control Syst
SFWE 511 (Fall 2025)
2024-25 Courses
-
Dissertation
ECE 920 (Spring 2025) -
Dissertation
SIE 920 (Spring 2025) -
Research
SFWE 900 (Spring 2025) -
Software Assurance & Securit
SFWE 501 (Spring 2025) -
Software Assurance & Security
SFWE 401 (Spring 2025) -
Dissertation
ECE 920 (Fall 2024) -
Dissertation
SIE 920 (Fall 2024) -
Research
SFWE 900 (Fall 2024) -
SW for Industrial Control Syst
SFWE 411 (Fall 2024) -
SW for Industrial Control Syst
SFWE 511 (Fall 2024)
2023-24 Courses
-
Directed Research
SFWE 492 (Summer I 2024) -
Internship
SIE 493 (Summer I 2024) -
Dissertation
ECE 920 (Spring 2024) -
Research
SIE 900 (Spring 2024) -
Software Assurance & Securit
SFWE 501 (Spring 2024) -
Software Assurance & Security
SFWE 401 (Spring 2024) -
Dissertation
ECE 920 (Fall 2023) -
Dissertation
SIE 920 (Fall 2023) -
Independent Study
ECE 599 (Fall 2023) -
Research
ECE 900 (Fall 2023) -
Research
SIE 900 (Fall 2023) -
SW for Industrial Control Syst
SFWE 411 (Fall 2023) -
SW for Industrial Control Syst
SFWE 511 (Fall 2023)
2022-23 Courses
-
Directed Research
SIE 492 (Summer I 2023) -
Dissertation
ECE 920 (Spring 2023) -
Research
SIE 900 (Spring 2023) -
Software Assurance & Security
SFWE 401 (Spring 2023)
2021-22 Courses
-
Fundamentals of Cloud Security
ECE 524 (Spring 2022)
2020-21 Courses
-
Fundamentals of Cloud Security
ECE 524 (Spring 2021)
2019-20 Courses
-
Circuit Theory
ECE 320A (Fall 2019)
2018-19 Courses
-
Obj Oriented Sftwr Dsgn
ECE 373 (Fall 2018)
2017-18 Courses
-
Obj Oriented Sftwr Dsgn
ECE 373 (Fall 2017)
2016-17 Courses
-
Obj Oriented Sftwr Dsgn
ECE 373 (Fall 2016)
Scholarly Contributions
Journals/Publications
- Rahman, M. W., Abrar, M. M., Copening, H. G., Hariri, S., Shao, S., Satam, P., & Salehi, S. (2023). Quantized Transformer Language Model Implementations on Edge Devices. ICMLA.More infoLarge-scale transformer-based models like the Bidirectional Encoder Representations from Transformers (BERT) are widely used for Natural Language Processing (NLP) applications, wherein these models are initially pre-trained with a large corpus with millions of parameters and then fine-tuned for a downstream NLP task. One of the major limitations of these large-scale models is that they cannot be deployed on resource-constrained devices due to their large model size and increased inference latency. In order to overcome these limitations, such large-scale models can be converted to an optimized FlatBuffer format, tailored for deployment on resource-constrained edge devices. Herein, we evaluate the performance of such FlatBuffer transformed MobileBERT models on three different edge devices, fine-tuned for Reputation analysis of English language tweets in the RepLab 2013 dataset. In addition, this study encompassed an evaluation of the deployed models, wherein their latency, performance, and resource efficiency were meticulously assessed. Our experiment results show that, compared to the original BERT large model, the converted and quantized MobileBERT models have 160$\times$ smaller footprints for a 4.1% drop in accuracy while analyzing at least one tweet per second on edge devices. Furthermore, our study highlights the privacy-preserving aspect of TinyML systems as all data is processed locally within a serverless environment. [Journal_ref: ]
- Rodriguez, J. J., Shao, S., Szep, J., Satam, P., Ram, S., Philip, R. C., Pacheco, J., Hariri, S., & Gao, X. (2022). Selecting Post-Processing Schemes for Accurate Detection of Small Objects in Low-Resolution Wide-Area Aerial Imagery. Remote Sensing, 14(2), 255. doi:10.3390/rs14020255More infoIn low-resolution wide-area aerial imagery, object detection algorithms are categorized as feature extraction and machine learning approaches, where the former often requires a post-processing scheme to reduce false detections and the latter demands multi-stage learning followed by post-processing. In this paper, we present an approach on how to select post-processing schemes for aerial object detection. We evaluated combinations of each of ten vehicle detection algorithms with any of seven post-processing schemes, where the best three schemes for each algorithm were determined using average F-score metric. The performance improvement is quantified using basic information retrieval metrics as well as the classification of events, activities and relationships (CLEAR) metrics. We also implemented a two-stage learning algorithm using a hundred-layer densely connected convolutional neural network for small object detection and evaluated its degree of improvement when combined with the various post-processing schemes. The highest average F-scores after post-processing are 0.902, 0.704 and 0.891 for the Tucson, Phoenix and online VEDAI datasets, respectively. The combined results prove that our enhanced three-stage post-processing scheme achieves a mean average precision (mAP) of 63.9% for feature extraction methods and 82.8% for the machine learning approach.
- Satam, P., & Hariri, S. (2021). WIDS: An Anomaly Based Intrusion Detection System for Wi-Fi (IEEE 802.11) Protocol. IEEE Transactions on Network and Service Management, 18(1), 1077-1091. doi:10.1109/tnsm.2020.3036138More infoOver the last few decades, the Internet has seen unprecedented growth, with over 4.57 billion active users as of July 2022, encompassing 59% of the global population. In recent years, we have seen an increase in mobile computing and the Internet of Things (IoT), allowing more users to communicate through the Internet using wireless devices. Modern Internet users use their wireless IoT devices for a wide variety of services that include cloud computing and storage, social networking, content services, online banking, shopping, to name a few. Moreover, with the omnipresence of IoT devices, wireless networks are used for services like device control, user authentication, etc. Wi-Fi is the network of choice for most of these wireless communications. Although Wi-Fi networks have improved over recent years, little has been done to secure Wi-Fi networks against attacks. In this article, we present a Wireless Intrusion Detection System (WIDS); an anomaly behavior analysis approach to detect attacks on Wi-Fi networks with high accuracy and low false alarms. In this approach, we model the normal behavior of the Wi-Fi protocol, using n-grams, and use machine learning models to classify Wi-Fi traffic flows as normal or malicious. We have extensively tested our approach on multiple datasets collected locally at the University of Arizona and AWID family of datasets. Our approach can successfully detect all attacks on Wi-Fi protocols with low false positives (0.0174) and a varying low rate of false negatives for different attacks.
- Satam, P., Hariri, S., Alshawi, A., & Almoualem, F. (2020). Effective Wireless Communication Architecture for Resisting Jamming Attacks. IEEE Access, 8, 176691-176703. doi:10.1109/access.2020.3027325More infoOver time, the use of wireless technologies has significantly increased due to bandwidth improvements, cost-effectiveness, and ease of deployment. Owing to the ease of access to the communication medium, wireless communications and technologies are inherently vulnerable to attacks. These attacks include brute force attacks such as jamming attacks and those that target the communication protocol (Wi-Fi and Bluetooth protocols). Thus, there is a need to make wireless communication resilient and secure against attacks. Existing wireless protocols and applications have attempted to address the need to improve systems security as well as privacy. They have been highly effective in addressing privacy issues, but ineffective in addressing security threats like jamming and session hijacking attacks and other types of Denial of Service Attacks. In this article, we present an “architecture for resilient wireless communications” based on the concept of Moving Target Defense. To increase the difficulty of launching successful attacks and achieve resilient operation, we changed the runtime characteristics of wireless links, such as the modulation type, network address, packet size, and channel operating frequency. The architecture reduces the overhead resulting from changing channel configurations using two communication channels, in which one is used for communication, while the other acts as a standby channel. A prototype was built using Software Defined Radio to test the performance of the architecture. Experimental evaluations showed that the approach was resilient against jamming attacks. We also present a mathematical analysis to demonstrate the difficulty of performing a successful attack against our proposed architecture.
- Satam, P., Pacheco, J., Felix-herran, L. C., & Benitez, V. H. (2020). Artificial Neural Networks Based Intrusion Detection System for Internet of Things Fog Nodes. IEEE Access, 8(1), 73907-73918. doi:10.1109/access.2020.2988055More infoThe Internet of Things (IoT) represents a mean to share resources (memory, storage computational power, data, etc.) between computers and mobile devices, as well as buildings, wearable devices, electrical grids, and automobiles, just to name few. The IoT is leading to the development of advanced information services that will require large storage and computational power, as well as real-time processing capabilities. The integration of IoT with emerging technologies such as Fog Computing can complement these requirements with pervasive and cost-effective services capable of processing large-scale geo-distributed information. In any IoT application, communication availability is essential to deliver accurate and useful information, for instance, to take actions during dangerous situations, or to manage critical infrastructures. IoT components like gateways, also called Fog Nodes, face outstanding security challenges as the attack surface grows with the number of connected devices requesting communication services. These Fog nodes can be targeted by an attacker, preventing the nodes from delivering important information to the final users or to perform accurate automated actions. This paper introduces an Anomaly Behavior Analysis Methodology based on Artificial Neural Networks, to implement an adaptive Intrusion Detection System (IDS) capable of detecting when a Fog node has been compromised, and then take the required actions to ensure communication availability. The experimental results reveal that the proposed approach has the capability for characterizing the normal behavior of Fog Nodes despite its complexity due to the adaptive scheme, and also has the capability of detecting anomalies due to any kind of sources such as misuses, cyber-attacks or system glitches, with high detection rate and low false alarms.
- Satam, P., Skaria, R., & Khalpey, Z. (2020). Opportunities and Challenges of Disruptive Innovation in Medicine Using Artificial Intelligence.. The American journal of medicine, 133(6), e215-e217. doi:10.1016/j.amjmed.2019.12.016
- Szep, J., Satam, P., Rodriguez, J. J., Ram, S., Hariri, S., & Gao, X. (2020). Spatio-Temporal Processing for Automatic Vehicle Detection in Wide-Area Aerial Video. IEEE Access, 8, 199562-199572. doi:10.1109/access.2020.3033466More infoVehicle detection in aerial videos often requires post-processing to eliminate false detections. This paper presents a spatio-temporal processing scheme to improve automatic vehicle detection performance by replacing the thresholding step of existing detection algorithms with multi-neighborhood hysteresis thresholding for foreground pixel classification. The proposed scheme also performs spatial post-processing, which includes morphological opening and closing to shape and prune the detected objects, and temporal post-processing to further reduce false detections. We evaluate the performance of the proposed spatial processing on two local aerial video datasets and one parking vehicle dataset, and the performance of the proposed spatio-temporal processing scheme on five local aerial video datasets and one public dataset. Experimental evaluation shows that the proposed schemes improve vehicle detection performance for each of the nine algorithms when evaluated on seven datasets. Overall, the use of the proposed spatio-temporal processing scheme improves average F-score to above 0.8 and achieves an average reduction of 83.8% in false positives.
- Tunc, C., Satam, P., Tunc, C., Pacheco, J., & Hariri, S. (2016). Secure and Resilient Cloud Services for Enhanced Living Environments. IEEE Cloud Computing, 3(6), 44-52. doi:10.1109/mcc.2016.129More infoIt is critical to provide enhanced living environments (ELEs) to people with special needs (such as the elderly and individuals with disabilities) that offer 24/7 continuous monitoring and control of the environment and access to care services when needed. Recently, there has been a strong interest in building ELEs using implantable and wearable sensors, and wireless sensor networks that are supported by cloud computing. However, ELE technologies and information are vulnerable to cyberattacks and exploitations that can lead to life-threatening scenarios such as incorrect medical diagnoses. This article presents a platform that offers secure and resilient services for ELEs. The main components of the platform are the ELE end nodes, secure gateway, and a secure and resilient cloud computing system. End nodes collect ELE variables and human body signals that are stored securely in the cloud using a secure gateway. The secure gateway manages communication between the end nodes and the cloud services using biocyber metrics for authentication. In addition, the cloud architecture provides the required ELE services at any time and from anywhere in a resilient manner.
- Satam, P., Hariri, S., Alipour, H., & Al-nashif, Y. B. (2015). Wireless Anomaly Detection Based on IEEE 802.11 Behavior Analysis. IEEE Transactions on Information Forensics and Security, 10(10), 2158-2170. doi:10.1109/tifs.2015.2433898More infoWireless communication networks are pervading every aspect of our lives due to their fast, easy, and inexpensive deployment. They are becoming ubiquitous and have been widely used to transfer critical information, such as banking accounts, credit cards, e-mails, and social network credentials. The more pervasive the wireless technology is going to be, the more important its security issue will be. Whereas the current security protocols for wireless networks have addressed the privacy and confidentiality issues, there are unaddressed vulnerabilities threatening their availability and integrity (e.g., denial of service, session hijacking, and MAC address spoofing attacks). In this paper, we describe an anomaly based intrusion detection system for the IEEE 802.11 wireless networks based on behavioral analysis to detect deviations from normal behaviors that are triggered by wireless network attacks. Our anomaly behavior analysis of the 802.11 protocols is based on monitoring the n-consecutive transitions of the protocol state machine. We apply sequential machine learning techniques to model the n-transition patterns in the protocol and characterize the probabilities of these transitions being normal. We have implemented several experiments to evaluate our system performance. By cross validating the system over two different wireless channels, we have achieved a low false alarm rate (
Proceedings Publications
- Tunc, C., Hariri, S. A., Ditzler, G., AI-Awady, K., Satam, S., Satam, P. C., & Shao, S. (2021, 10). Multi-Layer Mapping of Cyberspace for Intrusion Detection. In ACS/IEEE International Conference on Computer Systems and Applications.
- Satam, P., Satam, S., Hariri, S., & Alshawi, A. (2020). Anomaly Behavior Analysis of IoT Protocols. In Book Chapter, 295-330.
- Satam, S., Satam, P., & Hariri, S. (2020). Multi-level Bluetooth Intrusion Detection System. In 2020 IEEE/ACS 17th International Conference on Computer Systems and Applications (AICCSA).More infoLarge scale deployment of IoT devices has made Bluetooth Protocol (IEEE 802.15.1) the wireless protocol of choice for close-range communications. Devices such as keyboards, smartwatches, headphones, computer mouse, and various wearable connecting devices use Bluetooth network for communication. Moreover, Bluetooth networks are widely used in medical devices like heart monitors, blood glucose monitors, asthma inhalers, and pulse oximeters. Also, Bluetooth has replaced cables for wire-free equipment in a surgical environment. In hospitals, devices communicate with one another, sharing sensitive and critical information over Bluetooth scatter-networks. Thus, it is imperative to secure the Bluetooth networks against attacks like Man in the Middle attack (MITM), eavesdropping attacks, and Denial of Service (DoS) attacks. This paper presents a Multi-Level Bluetooth Intrusion Detection System (ML-BIDS) to detect malicious attacks against Bluetooth devices. In the ML-IDS framework, we perform continuous device identification and authorization in Bluetooth networks following the zero-trust principle [ref]. The ML-BIDS framework includes an anomaly-based intrusion detection system (ABIDS) to detect attacks on the Bluetooth protocol. The ABIDS tracks the normal behavior of the Bluetooth protocol by comparing it with the Bluetooth protocol state machine. Bluetooth frame flows consisting of Bluetooth frames received over 10 seconds are split into n-grams to track the current state of the protocol in the state machine. We evaluated the performance of several machine learning algorithms like C4.5, Adaboost, SVM, Naive Bayes, Jrip, and Bagging to classify normal Bluetooth protocol flows from abnormal Bluetooth protocol flows. The ABIDS detects attacks on Bluetooth protocols with a precision of up to 99.6% and recall up to 99.6%. The ML-BIDS framework also performs whitelisting of the devices on the Bluetooth network to prevent unauthorized devices from connecting to the network. ML-BIDS uses a combination of the Bluetooth Address, mac address, and IP address to uniquely identify a Bluetooth device connecting to the network, and hence ensuring only authorized devices can connect to the Bluetooth network.
- Satam, P., Hariri, S., Hess, S., Hariri, S., & Ditzler, G. (2018). Malicious HTML File Prediction: A Detection and Classification Perspective with Noisy Data. In 2018 IEEE/ACS 15th International Conference on Computer Systems and Applications (AICCSA).More infoCybersecurity plays a critical role in protecting sensitive information and the structural integrity of networked systems. As networked systems continue to expand in numbers as well as in complexity, so does the threat of malicious activity and the necessity for advanced cybersecurity solutions. Furthermore, both the quantity and quality of available data on malicious content as well as the fact that malicious activity continuously evolves makes automated protection systems for this type of environment particularly challenging. Not only is the data quality a concern, but the volume of the data can be quite small for some of the classes. This creates a class imbalance in the data used to train a classifier; however, many classifiers are not well equipped to deal with class imbalance. One such example is detecting malicious HMTL files from static features. Unfortunately, collecting malicious HMTL files is extremely difficult and can be quite noisy from HTML files being mislabeled. This paper evaluates a specific application that is afflicted by these modern cybersecurity challenges: detection of malicious HTML files. Previous work presented a general framework for malicious HTML file classification that we modify in this work to use a χ2 feature selection technique and synthetic minority oversampling technique (SMOTE). We experiment with different classifiers (i.e., AdaBoost, Gentle-Boost, RobustBoost, RusBoost, and Random Forest) and a pure detection model (i.e., Isolation Forest). We benchmark the different classifiers using SMOTE on a real dataset that contains a limited number of malicious files (40) with respect to the normal files (7,263). It was found that the modified framework performed better than the previous framework's results. However, additional evidence was found to imply that algorithms which train on both the normal and malicious samples are likely overtraining to the malicious distribution. We demonstrate the likely overtraining by determining that a subset of the malicious files, while suspicious, did not come from a malicious source.
- Satam, P., Satam, S., & Hariri, S. (2018). Bluetooth Intrusion Detection System (BIDS). In 2018 IEEE/ACS 15th International Conference on Computer Systems and Applications (AICCSA).More infoWith the rapid deployment of IOT devices, Bluetooth networks, which form Personal Area Networks(PAN), have become the wireless network of choice for small range/indoor communications networks. Bluetooth is widely used to deliver audio streams (e.g.: Bluetooth headphones, Music systems in cars), connecting peripherals devices to more powerful devices (e.g.: keyboards to computers), connecting wearable technology like smart watches, heart monitors and fitness trackers. It's imperative that Bluetooth networks (like other wireless networks) are secure against cyberattacks such as Man In The Middle Attacks(MITM), Denial of Service attacks(DoS), etc. Moreover, Bluetooth is used heavily in mobile devices/ sensors, and consequently they become sensitive to battery utilization attacks; this type of attacks requires the Bluetooth devices to be secure against different battery draining attacks. As a part of this paper we present an anomaly-based intrusion detection system for Bluetooth networks; Bluetooth IDS (BIDS). The BIDS use an n-gram based approach to characterize the normal behavior of the Bluetooth protocol. Smoothing techniques like Jelinek-Mercer smoothing was used to improve the machine learning algorithm used for detecting abnormal Bluetooth operations. Machine learning algorithms like C4.5, AdaBoostMl, SVM, Naive Bayes, RIPPER, Bagging were used to build the behavior models for the Bluetooth protocol. The developed models had high accuracy with precision up to 99.6% and recall up to 99.6%.
- Satam, P. (2017). Anomaly Based Wi-Fi Intrusion Detection System. In 2017 IEEE 2nd International Workshops on Foundations and Applications of Self* Systems (FAS*W), 377-378.More infoThe omnipresence of mobile devices and the great need to remain connected has brought to the forefront, the ever-growing need for wireless networks. This unprecedented growth of wireless networks and their use has resulted in an era where, the security of wireless networks has become a necessity. Currently the security methods to protect the Wi-Fi are based on the use of cryptography techniques to protect the data. But these methods fail to address the issue of availability of the service (against DOS), or Integrity (against Mac address spoofing).
- Satam, P., Ki, J., Hariri, S., & Almoualem, F. (2017). SDR-Based Resilient Wireless Communications. In 2017 International Conference on Cloud and Autonomic Computing (ICCAC), 114-119.More infoAs the use of wireless technologies increases significantly due to ease of deployment, cost-effectiveness and the increase in bandwidth, there is a critical need to make the wireless communications secure, and resilient to attacks or faults (malicious or natural). Wireless communications are inherently prone to cyberattacks due to the open access to the medium. While current wireless protocols have addressed the privacy issues, they have failed to provide effective solutions against denial of service attacks, session hijacking and jamming attacks.In this paper, we present a resilient wireless communication architecture based on Moving Target Defense, and Software Defined Radios (SDRs). The approach achieves its resilient operations by randomly changing the runtime characteristics of the wireless communications channels between different wireless nodes to make it extremely difficult to succeed in launching attacks. The runtime characteristics that can be changed include packet size, network address, modulation type, and the operating frequency of the channel. In addition, the lifespan for each configuration will be random. To reduce the overhead in switching between two consecutive configurations, we use two radio channels that are selected at random from a finite set of potential channels, one will be designated as an active channel while the second acts as a standby channel. This will harden the wireless communications attacks because the attackers have no clue on what channels are currently being used to exploit existing vulnerability and launch an attack. The experimental results and evaluation show that our approach can tolerate a wide range of attacks (Jamming, DOS and session attacks) against wireless networks.
- Satam, P., Pacheco, J., Horani, M., & Hariri, S. (2017). Autoinfotainment Security Development Framework (ASDF) for Smart Cars. In 2017 International Conference on Cloud and Autonomic Computing (ICCAC), 153-159.More infoThe Autoinfotainment system will not only provide information systems and entertainment to car components, but it will also connect to the Internet and a wide range of multimedia and mobile devices. However, with the introduction of many smart devices and a variety of wireless communications through Wi-Fi, Bluetooth, DSRC, and cellular, we are experiencing major challenges to secure and protect vehicular advanced information and entertainment services due to the significant increase of the attack surface, complexity, heterogeneity and number of interconnected resources. In this paper, we present an Auto Security Development Framework (ASDF) to build trustworthy and highly secure auto information and entertainment services. The ASDF enables developers to consider security issues at all the auto car communications layers and integrate security algorithms with the functions and services offered in each layer rather than considering security in an ad-hoc and after thought manner. We also show how this framework can be used to develop anomaly behavior analysis algorithm to detect wireless attacks against the QUALCOMM DragonBoard Autoinfotainment system.
- Tunc, C., Satam, P., Shao, S., Tunc, C., & Hariri, S. (2017). Real-Time IRC Threat Detection Framework. In 2017 IEEE 2nd International Workshops on Foundations and Applications of Self* Systems (FAS*W), 318-323.More infoMost of the social media platforms generate a massive amount of raw data that is slow-paced. On the other hand, Internet Relay Chat (IRC) protocol, which has been extensively used by hacker community to discuss and share their knowledge, facilitates fast-paced and real-time text communications. Previous studies of malicious IRC behavior analysis were mostly either offline or batch processing. This results in a long response time for data collection, pre-processing, and threat detection. However, since the threats can use the latest vulnerabilities to exploit systems (e.g. zero-day attack) and which can spread fast using IRC channels. Current IRC channel monitoring techniques cannot provide the required fast detection and alerting. In this paper, we present an alternative approach to overcome this limitation by providing real-time and autonomic threat detection in IRC channels. We demonstrate the capabilities of our approach using as an example the shadow brokers' leak exploit (the exploit leveraged by WannaCry ransomware attack) that was captured and detected by our framework.
- Satam, P., Kelly, D., & Hariri, S. (2016). Anomaly behavior analysis of website vulnerability and security. In 2016 IEEE/ACS 13th International Conference of Computer Systems and Applications (AICCSA), 1-7.More infoThe world wide web has grown exponentially over the previous decade in terms of its size that is currently over a billion sties, as well as the number of users. In fact, web usage has become pervasive to touch all aspects of our life, economy and education. These rapid advances have also significantly increase the vulnerabilities of websites that are being hacked on a daily basis. According to White Hat security's “2015 Website Security Statistics Report” more than 86% of all websites have one or more critical vulnerability and the likelihood of information leakage is 56%. With no effective website security measures in place, one can expect the website security to be even more critical. The main research goal of this paper is to overcome this challenge by presenting an online anomaly behavior analysis of websites (e.g., HTML files) to detect any malicious codes or pages that have been injected by web attacks. Our anomaly analysis approach utilizes feature selection, data mining, data analytics and statistical techniques to identify accurately the webpage contents that have been compromised or can be exploited by attacks such as phishing attacks, cross site scripting attacks, html injection attacks, malware insertion attacks, just to name a few. We have validated our approach on more than 10,000 files and showed that our approach can detect malicious HTML files with a true positive rate of 99% and a false positive rate of 0.8% for abnormal files.
- Satam, P. (2015). Cross Layer Anomaly Based Intrusion Detection System. In 2015 IEEE International Conference on Self-Adaptive and Self-Organizing Systems Workshops, 157-161.More infoSince the start of the 21st century, computer networks have been through an exponential growth in terms of the network capacity, the number of the users and the type of tasks that are performed over the network. With the resent boom of mobile devices (e.g., Tablet computers, smart phones, smart devices, and wearable computing), the number of network users is bound to increase exponentially. But, most of the communications protocols, that span over the 7 layers of the OSI model, were designed in the late 1980's or 90's. Although most of these protocols have had subsequent updates over time, most of these protocols still remain largely unsecure and open to attacks. Hence it is critically important to secure these protocols across the 7 layers of the OSI model. As a part of my PhD research, I am working on a cross layer anomaly behavior detection system for various protocols. This system will be comprised of intrusion detection systems (IDS) for each of the protocols that are present in each layer. The behavior analysis of each protocol will be carried out in two phases. In the first phase (training), the features that accurately characterize the normal operations of the protocol are identified using data mining and statistical techniques and then use them to build a runtime model of protocol normal operations. In addition, some known attacks against the studied protocol are also studied to develop a partial attack model for the protocol. The anomaly behavior analysis modules of each layer are then fused to generate a highly accurate detection system with low false alarms. In the second phase, the cross-layer anomaly based IDS is used to detect attacks against any communication protocols. We have already developed anomaly behavior modules for TCP, UDP, IP, DNS and Wi-Fi protocols. Our experimental results show that our approach can detect attacks accurately and with very low false alarms.
- Satam, P., Hariri, S., Alipour, H., & Al-nashif, Y. (2015). DNS-IDS: Securing DNS in the Cloud Era. In 2015 International Conference on Cloud and Autonomic Computing, 296-301.More infoRecently, there has been a rapid growth in cloud computing due to their ability to offer computing and storage on demand, its elasticity, and significant reduction in operational costs. However, cloud security is a grand obstacle for full deployment and utilization of cloud services. In this paper, we address the security of the DNS protocol that is widely used to translate the cloud domain names to correct IP addresses. The DNS protocol is prone to attacks like cache poisoning attacks and DNS hijacking attacks that can lead to compromising user's cloud accounts and stored information. We present an anomaly based Intrusion Detection System (IDS) for the DNS protocol (DNS-IDS) that models the normal operations of the DNS protocol and accurately detects any abnormal behavior or exploitation of the protocol. The DNS-IDS system operates in two phases, the training phase and the operational phase. In the training phase, we model the normal behavior of the DNS protocol as a finite state machine and we derive the normal temporal statistics of how normal DNS traffic transition within that state machine and store them in a database. To bound the normal event space, we also apply few known DNS attacks (e.g. Cache poisoning) and store the temporal statistics of the abnormal DNS traffic transition in a separate database. Then we develop an anomaly metric for the DNS protocol that is a function of the temporal statistics for both the normal and abnormal transitions of the DNS by applying classification algorithms like the Bagging algorithm. During the operational phase, the anomaly metric is used to detect DNS attacks (both known and novel attacks). We have evaluated our approach against a wide range of DNS attacks (DNS hijacking, Kaminsky attack, amplification attack, Birthday attack, DNS Rebinding attack). Our results show attack detection rate of 97% with very low false positive alarm rate (0.01397%), and round 3% false negatives.
- Tunc, C., Satam, P., Tunc, C., Hariri, S., Blasch, E., & Almoualem, F. (2015). DDDAS-Based Resilient Cyber Battle Management Services (D-RCBMS). In IEEE 22nd International Conference on High Performance Computing Workshops (HiPCW), 65-65.More infoThe integration of data from numerous, disparate sources of a cyber battle space makes the transformation of those data into actionable information extremely complex. Applying dynamic analysis, agile synthesis, and predictive modeling techniques to this problem space using human interaction would be impractical. An example of this type of situation is a hostile area where lives are at risk. By adopting the Dynamic Data-Driven Applications System (DDDAS) paradigm, we can efficiently address cyber battle management challenges through the following capabilities: (a) a simulation infrastructure that encompasses realistically complex scenarios, (b) information integration and informatics capacity to both ingest the massive data sets needed to capture large-scale cyber battle management complexity to process the result in a timely manner in order to support decision making processes, and (c) resilient computations and communications services to enable cyber battle management system to tolerate any type of attacks against command and control operations. In this paper, we will show how the DDDAS paradigm can be used to develop a DDDAS-based Resilient Cyber Battle Management Services (D-RCBMS) that would generate immediate transformative opportunities in development of network centric warfare management capabilities and utilization. The disruptive concept of cyber battle space comes from the potential to treat a weapon as well as all other resources (logical or physical) involved in a cyber battle space as nodes on the network. Within a network-centric environment such as the Global Information Grid (GIG), consuming the data streaming in from hundreds or thousands of data sources (e.g., sensors, observations, etc.) and creating actionable information is a desired capability. However, achieving this vision presents significant computing and computational challenges that require updates to current practices, paradigms and infrastructures in network-centric warfare, which are based on static, pre-orchestrated and centrally mediated models and implementations. The hallmark of D-RCBMS framework is the ability to dynamically couple and autonomically configure, in real-time, complex and customized interaction between physical models, environmental sensors and effectors, and embedded protocols. The D-RCBMS framework is based on a service-oriented cyberinfrastructure for pervasive access to, and coordinated sharing of geographically distributed hardware, software, and information resources, as well as interactions between computations, simulations, information/data, and experts distributed across the global information grid (GIG).
- Tunc, C., Satam, P., Tunc, C., Montero, F. D., Hariri, S., & Fargo, F. (2015). CLaaS: Cybersecurity Lab as a Service -- Design, Analysis, and Evaluation. In 2015 International Conference on Cloud and Autonomic Computing, 224-227.More infoThe explosive growth of IT infrastructures, cloud systems, and Internet of Things (IoT) have resulted in complex systems that are extremely difficult to secure and protect against cyberattacks that are growing exponentially in the complexity and also in the number. Overcoming the cybersecurity challenges require cybersecurity environments supporting the development of innovative cybersecurity algorithms and evaluation of the experiments. In this paper, we present the design, analysis, and evaluation of the Cybersecurity Lab as a Service (CLaaS) which offers virtual cybersecurity experiments as a cloud service that can be accessed from anywhere and from any device (desktop, laptop, tablet, smart mobile device, etc.) with Internet connectivity. We exploit cloud computing systems and virtualization technologies to provide isolated and virtual cybersecurity experiments for vulnerability exploitation, launching cyberattacks, how cyber resources and services can be hardened, etc. We also present our performance evaluation and effectiveness of CLaaS experiments used by students.
- Tunc, C., Satam, P., Tunc, C., Montero, F. D., Hariri, S., Fargo, F., & Al-nashif, Y. (2015). Teaching and Training Cybersecurity as a Cloud Service. In 2015 International Conference on Cloud and Autonomic Computing, 302-308.More infoThe explosive growth of IT infrastructures, cloud systems, and Internet of Things (IoT) have resulted in complex systems that are extremely difficult to secure and protect against cyberattacks which are growing exponentially in complexity and in number. Overcoming the cybersecurity challenges is even more complicated due to the lack of training and widely available cybersecurity environments to experiment with and evaluate new cybersecurity methods. The goal of our research is to address these challenges by exploiting cloud services. In this paper, we present the design, analysis, and evaluation of a cloud service that we refer to as Cybersecurity Lab as a Service (CLaaS) which offers virtual cybersecurity experiments that can be accessed from anywhere and from any device (desktop, laptop, tablet, smart mobile device, etc.) with Internet connectivity. In CLaaS, we exploit cloud computing systems and virtualization technologies to provide virtual cybersecurity experiments and hands-on experiences on how vulnerabilities are exploited to launch cyberattacks, how they can be removed, and how cyber resources and services can be hardened or better protected. We also present our experimental results and evaluation of CLaaS virtual cybersecurity experiments that have been used by graduate students taking our cybersecurity class as well as by high school students participating in GenCyber camps.