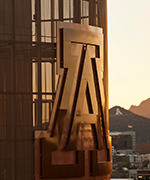
Kevin Lin
- Professor
- Professor, Statistics-GIDP
- Professor, Applied Mathematics - GIDP
- Professor, Cognitive Science - GIDP
- Professor, Neuroscience - GIDP
- Member of the Graduate Faculty
Contact
- (520) 626-6628
- Mathematics, Rm. 606
- Tucson, AZ 85721
- lin1@arizona.edu
Degrees
- Ph.D. Mathematics
- University of California at Berkeley, Berkeley, California, United States
- Random perturbations of SRB measures and numerical studies of chaotic dynamics
- B.S. Computer Science and Engineering
- Massachusetts Institute of Technology, Cambridge, Massachusetts, United States
- M.S. Electrical Engineering and Computer Science
- Massachusetts Institute of Technology, Cambridge, Massachusetts, United States
- Coordinate-Independent Computations on Differential Equations
- B.S. Mathematics
- Massachusetts Institute of Technology, Cambridge, Massachusetts, United States
Interests
Research
Dynamical systems; computation.
Teaching
Dynamical systems; applied mathematics; computing.
Courses
2025-26 Courses
-
Dissertation
APPL 920 (Fall 2025) -
Linear Algebra for Data Sci
DATA 412 (Fall 2025) -
Linear Algebra for Data Sci
MATH 412 (Fall 2025)
2024-25 Courses
-
Dissertation
APPL 920 (Spring 2025) -
Honors Thesis
MATH 498H (Spring 2025) -
Dissertation
APPL 920 (Fall 2024) -
Honors Thesis
MATH 498H (Fall 2024)
2023-24 Courses
-
Appl Stochastic Process
DATA 468 (Spring 2024) -
Appl Stochastic Process
MATH 468 (Spring 2024) -
Appl Stochastic Process
MATH 568 (Spring 2024) -
Dissertation
MATH 920 (Spring 2024) -
Dissertation
MATH 920 (Fall 2023) -
Linear Algebra for Data Sci
DATA 412 (Fall 2023) -
Linear Algebra for Data Sci
MATH 412 (Fall 2023)
2022-23 Courses
-
Appl Stochastic Process
DATA 468 (Spring 2023) -
Appl Stochastic Process
MATH 468 (Spring 2023) -
Dissertation
MATH 920 (Spring 2023) -
Honors Thesis
MATH 498H (Spring 2023) -
Advncd Tpcs in Undergrad Stats
DATA 496T (Fall 2022) -
Dissertation
MATH 920 (Fall 2022) -
Honors Thesis
MATH 498H (Fall 2022) -
Independent Study
MATH 599 (Fall 2022)
2021-22 Courses
-
Dissertation
MATH 920 (Spring 2022) -
Topics In Applied Math
MATH 577 (Spring 2022) -
Calculus II
MATH 129 (Fall 2021) -
Dissertation
MATH 920 (Fall 2021)
2020-21 Courses
-
Dissertation
MATH 920 (Spring 2021) -
Research
MATH 900 (Spring 2021) -
Thesis
MATH 910 (Spring 2021) -
Dissertation
MATH 920 (Fall 2020) -
Independent Study
MATH 599 (Fall 2020) -
Thesis
MATH 910 (Fall 2020)
2019-20 Courses
-
Appl Stochastic Process
DATA 468 (Spring 2020) -
Appl Stochastic Process
MATH 468 (Spring 2020) -
Appl Stochastic Process
MATH 568 (Spring 2020) -
Dissertation
MATH 920 (Spring 2020) -
Independent Study
MATH 599 (Spring 2020) -
Dissertation
MATH 920 (Fall 2019) -
Honors Thesis
MATH 498H (Fall 2019) -
Independent Study
MATH 599 (Fall 2019) -
Theory of Probability
MATH 464 (Fall 2019)
2018-19 Courses
-
Honors Independent Study
MATH 499H (Spring 2019) -
Research
MATH 900 (Spring 2019) -
Topics In Applied Math
MATH 577 (Spring 2019) -
Research
MATH 900 (Fall 2018)
2017-18 Courses
-
Dissertation
MATH 920 (Spring 2018) -
Dynamical Systems+Chaos
MATH 557B (Spring 2018) -
Independent Study
MATH 599 (Spring 2018) -
Journal Club
APPL 595B (Spring 2018) -
Dissertation
MATH 920 (Fall 2017) -
Dynamical Systems+Chaos
MATH 557A (Fall 2017) -
Independent Study
MATH 599 (Fall 2017) -
Intro:Stat+Biostatistics
MATH 263 (Fall 2017)
2016-17 Courses
-
Dissertation
MATH 920 (Spring 2017) -
Honors Thesis
MATH 498H (Spring 2017) -
Journal Club
APPL 595B (Spring 2017) -
Calculus II
MATH 129 (Fall 2016) -
Dissertation
MATH 920 (Fall 2016) -
Honors Thesis
MATH 498H (Fall 2016) -
Intro Ord Diff Equations
MATH 254 (Fall 2016) -
Journal Club
APPL 595B (Fall 2016)
2015-16 Courses
-
Dissertation
MATH 920 (Spring 2016) -
Dynamical Systems+Chaos
MATH 557B (Spring 2016) -
Honors Thesis
MATH 498H (Spring 2016) -
Journal Club
APPL 595B (Spring 2016)
Scholarly Contributions
Chapters
- Lin, K. K. (2013). Stimulus-Response Reliability of Biological Networks. In Nonautonomous and Random Dynamical Systems in Life Sciences. Springer, Cham. doi:10.1007/978-3-319-03080-7_4More infoIf a network of cells is repeatedly driven by the same sustained, complex signal, will it give the same response each time? A system whose response is reproducible across repeated trials is said to be reliable. Reliability is of interest in, e.g., computational neuroscience because the degree to which a neuronal network is reliable constrains its ability to encode information via precise temporal patterns of spikes. This chapter reviews a body of work aimed at discovering network conditions and dynamical mechanisms that can affect the reliability of a network. A number of results are surveyed here, including a general condition for reliability and studies of specific mechanisms for reliable and unreliable behavior in concrete models. This work relies on qualitative arguments using random dynamical systems theory, in combination with systematic numerical simulations.
Journals/Publications
- Xiao, Z., Lin, K. K., & Young, L. (2024). Efficient models of cortical activity via local dynamic equilibria and coarse-grained interactions. Proceedings of the National Academy of Sciences. doi:doi:10.1073/pnas.2320454121
- Glickenstein, D., Karamchandani, A., Korbut, C., Bang, M., Aucoin, A., Callovini, L., Lin, K., & Haynes, P. (2023). 0023 Circadian fragmentation and stability distinguish employment status. SLEEP, 46(Supplement_1), A10-A10. doi:10.1093/sleep/zsad077.0023
- , Z. X., , K. K., & , L. Y. (2021). A data-informed mean-field approach to mapping of cortical parameter landscapes. PLoS Computat. Biol..More infoConstraining the many biological parameters that govern cortical dynamics iscomputationally and conceptually difficult because of the curse ofdimensionality. This paper addresses these challenges by proposing (1) a noveldata-informed mean-field (MF) approach to efficiently map the parameter spaceof network models; and (2) an organizing principle for studying parameter spacethat enables the extraction biologically meaningful relations from thishigh-dimensional data. We illustrate these ideas using a large-scale networkmodel of the Macaque primary visual cortex. Of the 10-20 model parameters, weidentify 7 that are especially poorly constrained, and use the MF algorithm in(1) to discover the firing rate contours in this 7D parameter cube. Defining a"biologically plausible" region to consist of parameters that exhibitspontaneous Excitatory and Inhibitory firing rates compatible with experimentalvalues, we find that this region is a slightly thickened codimension-1submanifold. An implication of this finding is that while plausible regimesdepend sensitively on parameters, they are also robust and flexible providedone compensates appropriately when parameters are varied. Our organizingprinciple for conceptualizing parameter dependence is to focus on certain 2Dparameter planes that govern lateral inhibition: Intersecting these planes withthe biologically plausible region leads to very simple geometric structureswhich, when suitably scaled, have a universal character independent of wherethe intersections are taken. In addition to elucidating the geometry of theplausible region, this invariance suggests useful approximate scalingrelations. Our study offers, for the first time, a complete characterization ofthe set of all biologically plausible parameters for a detailed cortical model,which has been out of reach due to the high dimensionality of parameter space.[Journal_ref: PLoS Computat. Biol. 17 (2021)]
- Xiao, Z., & Lin, K. (2020). Multilevel Monte Carlo for Cortical Circuit Models. Journal of Computational Neuroscience.
- Xiao, Z., Lin, K., & Fellous, J. (2020). Conjunctive reward-place coding properties of dorsal distal CA1 hippocampus cells. Biological Cybernetics, 114, 285-301. doi:10.1007/s00422-020-00830-0
- Leach, A., Morzfeld, M., Lin, K., Lin, K., Leach, A., & Morzfeld, M. (2017). Symmetrized importance samplers for stochastic differential equations. Communications in Applied Mathematics and Computational Science.
- Lin, K. (2021). Data-driven model reduction, Wiener projections, and the Mori-Zwanzig formalism. Journal of Computational Physics, 424. doi:10.1016/j.jcp.2020.109864More infoModel reduction methods aim to describe complex dynamic phenomena using only relevant dynamical variables, decreasing computational cost, and potentially highlighting key dynamical mechanisms. In the absence of special dynamical features such as scale separation or symmetries, the time evolution of these variables typically exhibits memory effects. Recent work has found a variety of data-driven model reduction methods to be effective for representing such non-Markovian dynamics, but their scope and dynamical underpinning remain incompletely understood. Here, we study data-driven model reduction from a dynamical systems perspective. For both chaotic and randomly-forced systems, we show the problem can be naturally formulated within the framework of Koopman operators and the Mori-Zwanzig projection operator formalism. We give a heuristic derivation of a NARMAX (Nonlinear Auto-Regressive Moving Average with eXogenous input) model from an underlying dynamical model. The derivation is based on a simple construction we call Wiener projection, which links Mori-Zwanzig theory to both NARMAX and to classical Wiener filtering. We apply these ideas to the Kuramoto-Sivashinsky model of spatiotemporal chaos and a viscous Burgers equation with stochastic forcing.
- Goodman, J., Lin, K. K., & Morzfeld, M. (2014). Small-noise analysis and symmetrization of implicit Monte Carlo samplers. Communications in Pure and Applied Mathematics.More infoThis paper is about a new class of sampling algorithms, called implicit samplers, introduced by Chorin and Tu. It was accepted for publication in February 2015.
- Lajoie, G., Lin, K., Thivierge, J., & Shea-Brown, E. (2016). Encoding in balanced networks: revisiting spike patterns and chaos in stimulus-driven systems. PLOS Computational Biology.
- Lu, F., Lin, K., & Chorin, A. (2016). "Comparison of continuous and discrete-time data-based modeling for hypoelliptic systems. Communications in Applied Mathematics and Computational Science, 11, 187--216. doi:10.2140/camcos.2016.11.187
- Lu, F., Lin, K., & Chorin, A. (2017). Data-based stochastic model reduction for the Kuramoto-Sivashinsky equation. Physica D.
- Rogers, B., Lin, K. K., & Hooker, G. (2015). Control Theory and Experimental Design in Diffusion Processes. SIAM/ASA Journal on Uncertainty Quantification, 3(1), 234-264. doi:10.1137/140962280More infoThis paper considers the problem of designing time-dependent real-time control policies for controllable nonlinear diffusion processes, with the goal of obtaining maximally informative observations about parameters of interest. More precisely, we maximize the expected Fisher information for the parameter obtained over the duration of the experiment, conditional on observations made up to that time. We propose to accomplish this with a two-step strategy: when the full state vector of the diffusion process is observable continuously, we formulate this as an optimal control problem and apply numerical techniques from stochastic optimal control to solve it. When observations are incomplete, infrequent, or noisy, we propose using standard filtering techniques to first estimate the state of the system and then apply the optimal control policy using the posterior expectation of the state. We assess the effectiveness of these methods in three situations: a paradigmatic bistable model from statistical physics, a m...
- Barrat, A., Fernandez, B., Lin, K. K., & Young, L. (2013). Modeling temporal networks using random itineraries. Physical Review Letters, 110(15).More infoAbstract: We propose a procedure to generate dynamical networks with bursty, possibly repetitive and correlated temporal behaviors. Regarding any weighted directed graph as being composed of the accumulation of paths between its nodes, our construction uses random walks of variable length to produce time-extended structures with adjustable features. The procedure is first described in a general framework. It is then illustrated in a case study inspired by a transportation system for which the resulting synthetic network is shown to accurately mimic the empirical phenomenology. © 2013 American Physical Society.
- Hooker, G., Lin, K. K., & Rogers, B. (2014). Control theory and experimental design in diffusion processes. SIAM Journal on Uncertainty Quantification.More infoThis paper is about using stochastic optimal control methods to design adaptive experimental protocols so as to improve the information yield of dynamic experiments (such as those done in neuroscience and in micro-organism population biology). It was accepted for publication in January 2015.
- Lajoie, G., Lin, K. K., & Shea-Brown, E. (2013). Chaos and reliability in balanced spiking networks with temporal drive. Physical Review E - Statistical, Nonlinear, and Soft Matter Physics, 87(5).More infoAbstract: Biological information processing is often carried out by complex networks of interconnected dynamical units. A basic question about such networks is that of reliability: If the same signal is presented many times with the network in different initial states, will the system entrain to the signal in a repeatable way? Reliability is of particular interest in neuroscience, where large, complex networks of excitatory and inhibitory cells are ubiquitous. These networks are known to autonomously produce strongly chaotic dynamics - an obvious threat to reliability. Here, we show that such chaos persists in the presence of weak and strong stimuli, but that even in the presence of chaos, intermittent periods of highly reliable spiking often coexist with unreliable activity. We elucidate the local dynamical mechanisms involved in this intermittent reliability, and investigate the relationship between this phenomenon and certain time-dependent attractors arising from the dynamics. A conclusion is that chaotic dynamics do not have to be an obstacle to precise spike responses, a fact with implications for signal coding in large networks. © 2013 American Physical Society.
- Lin, K. K., Wedgwood, K. C., Coombes, S., & Young, L. (2013). Limitations of perturbative techniques in the analysis of rhythms and oscillations. Journal of Mathematical Biology, 66(1-2), 139-161.More infoPMID: 22290314;Abstract: Perturbation theory is an important tool in the analysis of oscillators and their response to external stimuli. It is predicated on the assumption that the perturbations in question are "sufficiently weak", an assumption that is not always valid when perturbative methods are applied. In this paper, we identify a number of concrete dynamical scenarios in which a standard perturbative technique, based on the infinitesimal phase response curve (PRC), is shown to give different predictions than the full model. Shear-induced chaos, i. e., chaotic behavior that results from the amplification of small perturbations by underlying shear, is missed entirely by the PRC. We show also that the presence of "sticky" phase-space structures tend to cause perturbative techniques to overestimate the frequencies and regularity of the oscillations. The phenomena we describe can all be observed in a simple 2D neuron model, which we choose for illustration as the PRC is widely used in mathematical neuroscience. © 2012 The Author(s).
- Lyttle, D., Lyttle, D., Lin, K. K., Lin, K. K., Gereke, B., Gereke, B., Fellous, J. M., & Fellous, J. M. (2013). Spatial scale and place field stability in a grid-to-place cell model of the dorsoventral axis of the hippocampus.. Hippocampus, 23(8), 729-44. doi:10.1002/hipo.22132More infoThe rodent hippocampus and entorhinal cortex contain spatially modulated cells that serve as the basis for spatial coding. Both medial entorhinal grid cells and hippocampal place cells have been shown to encode spatial information across multiple spatial scales that increase along the dorsoventral axis of these structures. Place cells near the dorsal pole possess small, stable, and spatially selective firing fields, while ventral cells have larger, less stable, and less spatially selective firing fields. One possible explanation for these dorsoventral changes in place field properties is that they arise as a result of similar dorsoventral differences in the properties of the grid cell inputs to place cells. Here, we test the alternative hypothesis that dorsoventral place field differences are due to higher amounts of nonspatial inputs to ventral hippocampal cells. We use a computational model of the entorhinal-hippocampal network to assess the relative contributions of grid scale and nonspatial inputs in determining place field size and stability. In addition, we assess the consequences of grid node firing rate heterogeneity on place field stability. Our results suggest that dorsoventral differences in place cell properties can be better explained by changes in the amount of nonspatial inputs, rather than by changes in the scale of grid cell inputs, and that grid node heterogeneity may have important functional consequences. The observed gradient in field size may reflect a shift from processing primarily spatial information in the dorsal hippocampus to processing more nonspatial, contextual, and emotional information near the ventral hippocampus.
- Wedgwood, K. C., Lin, K. K., Thul, R., & Coombes, S. (2013). Phase-amplitude descriptions of neural oscillator models. Journal of Mathematical Neuroscience, 3(1), 1-22.More infoPMID: 23347723;PMCID: PMC3582465;Abstract: Phase oscillators are a common starting point for the reduced description of many single neuron models that exhibit a strongly attracting limit cycle. The framework for analysing such models in response to weak perturbations is now particularly well advanced, and has allowed for the development of a theory of weakly connected neural networks. However, the strong-attraction assumption may well not be the natural one for many neural oscillator models. For example, the popular conductance based Morris-Lecar model is known to respond to periodic pulsatile stimulation in a chaotic fashion that cannot be adequately described with a phase reduction. In this paper, we generalise the phase description that allows one to track the evolution of distance from the cycle as well as phase on cycle. We use a classical technique from the theory of ordinary differential equations that makes use of a moving coordinate system to analyse periodic orbits. The subsequent phase-amplitude description is shown to be very well suited to understanding the response of the oscillator to external stimuli (which are not necessarily weak). We consider a number of examples of neural oscillator models, ranging from planar through to high dimensional models, to illustrate the effectiveness of this approach in providing an improvement over the standard phase-reduction technique. As an explicit application of this phase-amplitude framework, we consider in some detail the response of a generic planar model where the strong-attraction assumption does not hold, and examine the response of the system to periodic pulsatile forcing. In addition, we explore how the presence of dynamical shear can lead to a chaotic response. © 2013 K.C.A.
- Weitzenfeld, A., Lyttle, D., Lin, K. K., & Fellous, J. M. (2013). The influence of multiple firing events on the formation and stability of activity patterns in continuous attractor networks. BMC Neuroscience, 14(1). doi:10.1186/1471-2202-14-s1-p241More infoContinuous attractor networks have been proposed to explain a variety of phenomena, including working memory and rodent entorhinal grid cells [1,2]. Typically, such networks consist of spatially-structured lattices of neurons in one or two dimensions with long-range inhibition and short-range excitation, which causes the network activity to spontaneously self-organize into one or more "bumps" of persistent activity. In two-dimensions, the activity patterns often take the form of triangular lattices, which bear a striking resemblance to the spatial patterns observed in recordings of entorhinal grid cells [3]. Rate-based neural field models of attractor networks predict the emergence of stable, stationary patterns [2,4], whereas simulations of integrate and fire neurons suggest a more complicated dynamical picture. In particular, synaptic timescales appear to play a crucial role [5,6] in that fast synapses tend to lead to transient, local synchronous activity, thus destabilizing activity patterns. Recent work on a model of V1 suggests that such "multiple firing events" (MFEs) may be a generic, emergent feature of spiking networks [7]. In this work, we investigate whether and how MFEs may affect pattern stability in continuous attractor networks. While the stability of spiking neuron-based attractor networks have been studied previously [5,6], the models studied to date contain neither seperate excitatory and inhibitory populations nor a mixture of synaptic timescales (as would be expected in more realistic settings), and the effects of these features on pattern stability are not known. To investigate these questions, we have implemented continuous attractor networks in both one and two dimensions using spiking, integrate-and-fire neurons with conductance-based synapses and seperate excitatory and inhibitory populations. Through simulations, we systematically assess the effect of MFEs on the formation and stability of spatiotemporal activity patterns ("bumps" and "grids"). We also investigate the effects of multiple synaptic timescales, noise, and network heterogeneity on the stability of these patterns. An overarching goal of this work is to obtain insights into how real neural systems might maintain stable persistent activity states, such as those needed for accurately integrating sensorimotor information. Furthermore, as 2-D attractor networks have been incorporated into a number of recent grid cell models [2,8], and the stability of grid cell activity may have a significant effect on place fields [9], understanding the stability of the grid patterns formed by these networks is highly relevant to studies of the rat spatial navigation system.
- Lyttle, D., Lin, K. K., & Fellous, J. M. (2012). Coding, stability, and non-spatial inputs in a modular grid-to-place cell model. BMC Neuroscience, 13(1). doi:10.1186/1471-2202-13-s1-p141More infoGrid cells in the medial entorhinal cortex (mEC) and place cells in the hippocampus are paradigms for population coding of spatial information [1]. Both the spatiallyperiodic firing fields of grid cells and the spatially localized firing fields of place cells show systematic increases in spatial scale along the dorso-ventral axes of the mEC and hippocampus, respectively [2,3], which has led to speculation that place field size is determined simply by the spatial scale of a place cell’s grid cell inputs. However, in addition to receiving spatially-modulated entorhinal inputs, place cells receive contextual, non-spatial inputs from sources such as the amygdala and hypothalamus [4], which may be important in determining place cell firing properties. These non-spatial inputs are particularly prominent toward the ventral pole of the hippocampus [4], and thus could also play a role in producing dorso-ventral place cell differences. In order to understand the relative contributions of grid cells and non-spatial inputs in determining place field size and stability, we propose a computational model of the hippocampal-entorhinal network that includes a modular organization of grid cell inputs arranged in order of increasing spatial scale, as is seen experimentally in the mEC. Our underlying place cell model is a firing-rate based model inspired by previous work [5], in which place fields are formed via competition between place cells. We also introduce a dorsoventral gradient in the amount of non-spatial input to place cells, with ventral cells receiving more input from non-spatial sources. Finally, we introduce heterogeneity into the firing rates of grid vertices within individual grid fields. This heterogeneity is observed in experimental recordings [6] but has received relatively little attention in experimental or theoretical work, despite its potential impact on place field stability. Our main findings suggest that: 1.) For a wide range of parameters, the relative amounts of spatial and non-spatial inputs to place cells plays a more important role in determining place field size and stability than the spatial scale of grid cell inputs. This implies that the dorso-ventral gradient in place field size may reflect a dorso-ventral gradient in non-spatial inputs, rather than grid scale, and is agreement with prior suggestions of a functional distinction between the dorsal and ventral regions of the hippocampus [7]. 2.) In our model, place fields are sensitive to changes in the firing rates of the grid vertices of individual grid cells, emphasizing the potential implications of this grid field heterogeneity for place field formation and stability.
- Lin, K. K., & Young, L. (2010). Dynamics of periodically kicked oscillators. Journal of Fixed Point Theory and Applications, 7(2), 291-312.More infoAbstract: We review some recent results surrounding a general mechanism for producing chaotic behavior in periodically kicked oscillators. The key geometric ideas are illustrated via a simple linear shear model. © 2010 Springer Basel AG.
- Lin, K. K., & Young, L. (2010). Nonequilibrium steady states for certain Hamiltonian models. Journal of Statistical Physics, 139(4), 630-657.More infoAbstract: We report the results of a numerical study of nonequilibrium steady states for a class of Hamiltonian models. In these models of coupled matter-energy transport, particles exchange energy through collisions with pinned-down rotating disks. In Commun. Math. Phys. 262, 237-267, 2006, Eckmann and Young studied 1D chains and showed that certain simple formulas give excellent approximations of energy and particle density profiles. Keeping the basic mode of interaction in Commun. Math. Phys. 262, 237-267, 2006, we extend their prediction scheme to a number of new settings: 2D systems on different lattices, driven by a variety of boundary (heat bath) conditions including the use of thermostats. Particle-conserving models of the same type are shown to behave similarly. The second half of this paper examines memory and finite-size effects, which appear to impact only minimally the profiles of the models tested in Commun. Math. Phys. 262, 237-267, 2006. We demonstrate that these effects can be significant or insignificant depending on the local geometry. Dynamical mechanisms are proposed, and in the case of directional bias in particle trajectories due to memory, correction schemes are derived and shown to give accurate predictions. © 2010 Springer Science+Business Media, LLC.
- Young, L. S., Lin, K. K., & Balint, P. (2010). Ergodicity and Energy Distributions for Some Boundary Driven Integrable Hamiltonian Chains. Communications in Mathematical Physics, 294(1), 199-228. doi:10.1007/s00220-009-0918-xMore infoWe consider systems of moving particles in 1-dimensional space interacting through energy storage sites. The ends of the systems are coupled to heat baths, and resulting steady states are studied. When the two heat baths are equal, an explicit formula for the (unique) equilibrium distribution is given. The bulk of the paper concerns nonequilibrium steady states, i.e., when the chain is coupled to two unequal heat baths. Rigorous results including ergodicity are proved. Numerical studies are carried out for two types of bath distributions. For chains driven by exponential baths, our main finding is that the system does not approach local thermodynamic equilibrium as system size tends to infinity. For bath distributions that are sharply peaked Gaussians, in spite of the near-integrable dynamics, transport properties are found to be more normal than expected.
- Balint, P., Lin, K. K., & Young, L. (2009). Ergodicity and energy distributions for some boundary driven integrable Hamiltonian chains. Communications in Mathematical Physics, 294(1), 199-228.More infoAbstract: We consider systems of moving particles in 1-dimensional space interacting through energy storage sites. The ends of the systems are coupled to heat baths, and resulting steady states are studied. When the two heat baths are equal, an explicit formula for the (unique) equilibrium distribution is given. The bulk of the paper concerns nonequilibrium steady states, i.e., when the chain is coupled to two unequal heat baths. Rigorous results including ergodicity are proved. Numerical studies are carried out for two types of bath distributions. For chains driven by exponential baths, our main finding is that the system does not approach local thermodynamic equilibrium as system size tends to infinity. For bath distributions that are sharply peaked Gaussians, in spite of the near-integrable dynamics, transport properties are found to be more normal than expected. © 2009 Springer-Verlag.
- Goodman, J. B., & Lin, K. K. (2009). Coupling control variates for Markov chain Monte Carlo. Journal of Computational Physics, 228(19), 7127-7136.More infoAbstract: We show that Markov couplings can be used to improve the accuracy of Markov chain Monte Carlo calculations in some situations where the steady-state probability distribution is not explicitly known. The technique generalizes the notion of control variates from classical Monte Carlo integration. We illustrate it using two models of nonequilibrium transport. © 2009 Elsevier Inc. All rights reserved.
- Lin, K. K., Shea-Brown, E., & Young, L. (2009). Reliability of coupled oscillators. Journal of Nonlinear Science, 19(5), 497-545.More infoAbstract: We study the reliability of phase oscillator networks in response to fluctuating inputs. Reliability means that an input elicits essentially identical responses upon repeated presentations, regardless of the network's initial condition. Single oscillators are well known to be reliable. We show in this paper that unreliable behavior can occur in a network as small as a coupled oscillator pair in which the signal is received by the first oscillator and relayed to the second with feedback. A geometric explanation based on shear-induced chaos at the onset of phase-locking is proposed. We treat larger networks as decomposed into modules connected by acyclic graphs, and give a mathematical analysis of the acyclic parts. Moreover, for networks in this class, we show how the source of unreliability can be localized, and address questions concerning downstream propagation of unreliability once it is produced. © 2009 Springer Science+Business Media, LLC.
- Lin, K. K., Shea-Brown, E., & Young, L. (2009). Reliability of layered neural oscillator networks. Communications in Mathematical Sciences, 7(1), 239-247.More infoAbstract: We study the reliability of large networks of pulse-coupled oscillators in response to fluctuating stimuli. Reliability means that a stimulus elicits essentially identical responses upon repeated presentations. We view the problem on two scales: neuronal reliability, which concerns the repeatability of spike times of individual oscillators embedded within a network, and pooled-response reliability, which addresses the repeatability of the total output from the network. We find that individual embedded oscillators can be reliable or unreliable depending on network conditions, whereas pooled responses of sufficiently large networks are mostly reliable. © 2009 International Press.
- Lin, K. K., Shea-Brown, E., & Young, L. (2009). Spike-time reliability of layered neural oscillator networks. Journal of Computational Neuroscience, 27(1), 135-160.More infoPMID: 19156509;Abstract: We study the reliability of layered networks of coupled 'type I' neural oscillators in response to fluctuating input signals. Reliability means that a signal elicits essentially identical responses upon repeated presentations, regardless of the network's initial condition. We study reliability on two distinct scales: neuronal reliability, which concerns the repeatability of spike times of individual neurons embedded within a network, and pooled-response reliability, which concerns the repeatability of total synaptic outputs from a subpopulation of the neurons in a network. We find that neuronal reliability depends strongly both on the overall architecture of a network, such as whether it is arranged into one or two layers, and on the strengths of the synaptic connections. Specifically, for the type of single-neuron dynamics and coupling considered, single-layer networks are found to be very reliable, while two-layer networks lose their reliability with the introduction of even a small amount of feedback. As expected, pooled responses for large enough populations become more reliable, even when individual neurons are not. We also study the effects of noise on reliability, and find that noise that affects all neurons similarly has much greater impact on reliability than noise that affects each neuron differently. Qualitative explanations are proposed for the phenomena observed. © Springer Science+Business Media, LLC 2009.
- Lin, K. K., & Young, L. (2008). Shear-induced chaos. Nonlinearity, 21(5), 899-922.More infoAbstract: Guided by a geometric understanding developed in earlier works of Wang and Young, we carry out numerical studies of shear-induced chaos in several parallel but different situations. The settings considered include periodic kicking of limit cycles, random kicks at Poisson times and continuous-time driving by white noise. The forcing of a quasi-periodic model describing two coupled oscillators is also investigated. In all cases, positive Lyapunov exponents are found in suitable parameter ranges when the forcing is suitably directed. © 2008 IOP Publishing Ltd and London Mathematical Society.
- Lin, K. K., & Young, L. (2007). Correlations in nonequilibrium steady states of random halves models. Journal of Statistical Physics, 128(3), 607-639.More infoAbstract: We present the results of a detailed study of energy correlations at steady state for a 1-D model of coupled energy and matter transport. Our aim is to discover-via theoretical arguments, conjectures, and numerical simulations-how spatial covariances scale with system size, their relations to local thermodynamic quantities, and the randomizing effects of heat baths. Among our findings are that short-range covariances respond quadratically to local temperature gradients, and long-range covariances decay linearly with macroscopic distance. These findings are consistent with exact results for the simple exclusion and KMP models. © 2007 Springer Science+Business Media, LLC.
- Lin, K. K. (2006). Entrainment and chaos in a pulse-driven hodgkin-huxley oscillator. SIAM Journal on Applied Dynamical Systems, 5(2), 179-204.More infoAbstract: The Hodgkin-Huxley model describes action potential generation in certain types of neurons and is a standard model for conductance-based, excitable cells. Following the early work of Winfree and Best, this paper explores the response of a spontaneously spiking Hodgkin-Huxley neuron model to a periodic pulsatile drive. The response as a function of drive period and amplitude is systematically characterized. A wide range of qualitatively distinct responses are found, including entrainment to the input pulse train and persistent chaos. These observations are consistent with a theory of kicked oscillators developed by Q. Wang and L.-S. Young. In addition to general features predicted by Wang-Young theory, it is found that most combinations of drive period and amplitude lead to entrainment instead of chaos. This preference for entrainment over chaos is explained by the structure of the Hodgkin-Huxley phase-resetting curve. © 2006 Society for Industrial and Applied Mathematics.
- Lin, K. K. (2005). Convergence of invariant densities in the small-noise limit. Nonlinearity, 18(2), 659-683.More infoAbstract: Let ρ0 be an invariant probability density of a deterministic dynamical system f and ρε the invariant probability density of a random perturbation of f by additive noise of amplitude ε. Suppose ρ0 is stochastically stable in the sense that ρε → 0 as ε → 0. Through a systematic numerical study of concrete examples, I show that: The rate of convergence of ρε to 7rho;ε0 as ε → 0 is frequently governed by power laws: ∥ρε - ρ0∥1 ∼ ε γ for some γ > 0. When the deterministic system / exhibits exponential decay of correlations, a simple heuristic can correctly predict the exponent γ based on the structure of ρ0. The heuristic fails for systems with some 'intermittency', i.e. systems which do not exhibit exponential decay of correlations. For these examples, the convergence of ρε to ρ0 as ε → 0 continues to be governed by power laws but the heuristic provides only an upper bound on the power law exponent γ. Furthermore, this numerical study requires the computation of ∥ρε - ρ0∥ 1 for 1.5-2.5 decades of ε and provides an opportunity to discuss and compare standard numerical methods for computing invariant probability densities in some depth. © 2005 IOP Publishing Ltd and London Mathematical Society.
- Guillopé, L., Lin, K. K., & Zworski, M. (2004). The Selberg Zeta Function for Convex Co-Compact Schottky Groups. Communications in Mathematical Physics, 245(1), 149-176.More infoAbstract: We give a new upper bound on the Selberg zeta function for a convex co-compact Schottky group acting on the hyperbolic space ℍn+1 : in strips parallel to the imaginary axis the zeta function is bounded by exp(C|s|δ) where δ is the dimension of the limit set of the group. This bound is more precise than the optimal global bound exp(C|s|n+1), and it gives new bounds on the number of resonances (scattering poles) of Γ\Hn+1. The proof of this result is based on the application of holomorphic L2techniques to the study of the determinants of the Ruelle transfer operators and on the quasi-self-similarity of limit sets. We also study this problem numerically and provide evidence that the bound may be optimal. Our motivation comes from molecular dynamics and we consider γ\Hn+1 as the simplest model of quantum chaotic scattering.
- Lin, K. K. (2002). Numerical study of quantum resonances in chaotic scattering. Journal of Computational Physics, 176(2), 295-329.More infoAbstract: This paper presents numerical evidence that in quantum systems with chaotic classical dynamics, the number of scattering resonances near an energy E scales like h-DKE+1/2 as h → 0. Here, KE denotes the subset of the energy surface {H = E} which stays bounded for all time under the flow generated by the classical Hamiltonian H and D(KE) denotes its fractal dimension. Since the number of bound states in a quantum system with n degrees of freedom scales like h-n, this suggests that the quantity (D(KE) + 1)/2 represents the effective number of degrees of freedom in chaotic scattering problems. The calculations were performed using a recursive refinement technique for estimating the dimension of fractal repellors in classical Hamiltonian scattering, in conjunction with tools from modern quantum chemistry and numerical linear algebra. © 2002 Elsevier Science (USA).
- Lin, K. K., & Zworski, M. (2002). Quantum resonances in chaoti scattering. Chemical Physics Letters, 355(1-2), 201-205.More infoAbstract: This Letter summarizes numerical results from [J. Comp. Phys. (to appear)] which show that in quantum systems with chaotic classical dynamics, the number of scattering resonances near an energy E scales like h -(D(KE)+1)/2. Here, K E denotes the subset of the classical energy surface H = E which stays bounded for all time under the flow of H and D(K E) denotes its fractal dimension. Since the number of bound states in a quantum system with n degrees of freedom scales like h -n, this suggests that the quantity (D(K E) + 1)/2 represents the effective number of degrees of freedom in chaotic scattering problems. © 2002 Published by Elsevier Science B.V.
- Lin, K. K. (1998). Coordinate-Independent Computations on Differential Equations. MIT Artificial Intelligence Laboratory Technical Memo.More infoThis project investigates the computational representation of differentiable manifolds, with the primary goal of solving partial differential equations using multiple coordinate systems on general n-dimensional spaces. In the process, this abstraction is used to perform accurate integrations of ordinary differential equations using multiple coordinate systems. In the case of linear partial differential equations, however, unexpected difficulties arise even with the simplest equations. This report is a revised version of a thesis submitted to the Department of Electrical Engineering and Computer Science in Septembr 1997 in partial fulfillment of the requirements for the degree of Master of Engineering. This report describes research done at the Artificial Intelligence Laboratory of the Massachusetts Institute of Technology. Support for this research is provided in part by the Advanced Research Projects Agency of the Department of Defense under Office of Naval Research contracts N0001496-1-1228 and N00014-92-J-4097.
Proceedings Publications
- Lin, K. K., & Greene, P. (2022). A comparison of probe geometries for neuronal localization. In 2022 IEEE EMBS Conference.
- Young, L. S., Shea-brown, E., & Lin, K. K. (2013). Spike-time reliability of layered neural oscillator networks. In AIP Conference Proceedings, 1510, 207-209.More infoIf a network of neurons is repeatedly driven by the same fluctuating signal, will it give the same response each time? If so, the network is said to be reliable. Reliability is of interest in computational neuroscience because the degree to which a network is reliable constrains its ability to encode information in precise temporal patterns of spikes. This note outlines how the question of reliability may be fruitfully formulated and studied within the framework of random dynamical systems theory. A specific network architecture, that of a single-layer network, is examined. For the type of single-neuron dynamics and coupling considered here, single-layer networks are found to be very reliable. A qualitative explanation is proposed for this phenomenon.
Presentations
- Lin, K., & Lu, F. (2024, April). Data-driven stochastic non-Markovian models of multiscale dynamical systems. SIAM Conference on Uncertainty Quantification. Trieste, Italy.
- Lin, K., & Lu, F. (2024, September). Mori-Zwanzig formalism, Wiener projections, and random dynamics. Model Reduction and Surrogate Modeling. San Diego, CA.
- Xiao, Z., Lin, K., & Young, L. (2024, January). Coarse-grained models of cortical circuits. Dynamics Days. Davis, CA.
- Xiao, Z., Lin, K., & Young, L. (2024, June). Coarse-grained models of cortical circuits via local dynamic equilibria. SIAM Conference on Life Sciences. Portland, OR.
- Lin, K. (2022, April). Data-based model reduction and Mori-Zwanzig formalism for random dynamical systems. SIAM Conference on Uncertainty Quantification. Atlanta, Georgia.
- Lin, K. (2022, July). Data-based model reduction and Mori-Zwanzig formalism for random dynamical systems. World Congress in Computational Mechanics. Yokohama, Japan (virtual).
- Lin, K. (2022, September). A coarse-graining approach to mapping cortical parameter space. 3rd Symposium on Machine Learning and Dynamical Systems. Fields Institute, Toronto, Canada.
- Miller, L., Glickenstein, D. A., & Lin, K. (2022). Applied Mathematics and Statistics for Data-Driven Discovery. SIAM Conference on Applied Mathematics Education.
- Lin, K. (2021, April). Couplings-based sensitivity estimates for stochastic dynamics. University of Delaware Stochastic Processes and Applications Seminar. virtual.
- Lin, K. (2021, April). Data-based approach to non-Markovian model reduction for chaotic & stochastic dynamical systems. Department of Computational Mathematics, Science and Engineering Colloquium. virtual.More infoInvited talk
- Lin, K. (2021, August). Data-based approach to non-Markovian model reduction for chaotic & stochastic dynamical systems. DynamicsDays Europe.More infoOrganizer of a session
- Lin, K. (2021, March). Couplings-based sensitivity estimates for stochastic dynamics. SIAM Computational Science & Engineering. virtual.
- Lin, K. (2021, September). Mori-Zwanzig formalism and data-based non-Markovian model reduction for chaotic & stochastic dynamical systems. MATH+ CECAM Discussion Meeting on Generalized Langevin Equations. Berlin, Germany (hybrid; I attended virtually).
- Lin, K. (2020, July). Multilevel Monte Carlo Methods for Spiking Neuronal Networks. International Conference on Mathematical Neuroscience.
- Lin, K. (2020, September). Data driven model reduction and the Koopman-Mori-Zwanzig formalism. Symposium on Machine Learning and Dynamical Systems. Online.
- Lin, K. (2019, Fall). Couplings-based sensitivity estimates for stochastic dynamics. AMS Western Sectional Meeting. UC Riverside: American Mathematical Society.
- Lin, K. (2019, February). Data-driven modeling of chaotic dynamics: a model reduction perspective. Symposium on Machine Learning and Dynamical Systems. London, UK: Imperial College London.
- Lin, K. (2019, July). Data-driven modeling of chaotic dynamics: a model reduction perspective. SciCADE Conference. Innsbruck, Austria: International Conference on Scientific Computation and Differential Equations.
- Lin, K. (2019, May). Data-driven modeling and model reduction for chaotic dynamical systems. UC Santa Cruz Applied Math Seminar. Santa Cruz, CA: UC Santa Cruz.
- Lin, K. (2019, May). International Conference on Scientific Computation and Differential Equations. SIAM Conference on Applications of Dynamical Systems. Snowbird, UT: Society for Industrial and Applied Mathematics.
- Lin, K. (2019, September). Data-driven model reduction for chaotic dynamical systems. University of Houston Mathematics Colloquium. Houston, TX: Department of Mathematics, University of Houston.
- Lin, K. (2019, Spring). Mori-Zwanzig formalism and discrete-time modeling of chaotic dynamics. SIAM Computational Science & Engineering. Spokane, WA: Society for Industrial and Applied Mathematics.
- Lin, K. (2017, April). Control Theory and Experimental Design in Diffusion Processes. ASU Mathematical Biology Seminar. Arizona State.
- Lin, K. (2017, April). Implicit sampling in the small-noise limit. UC Santa Cruz Applied Math Seminar. UC Santa Cruz.
- Lin, K. (2017, August). Discrete-time approach to stochastic parameterization of chaotic dynamics. PIMS Workshop on Nonlinear Stochastic Dynamics. University of Alberta.
- Lin, K. (2017, March). Empirical approaches to the Mori-Zwanzig formalism. SIAM Computational Science and Engineering Conference. Atlanta, GA: SIAM.
- Lin, K. (2017, May). Data-driven modeling and the Mori-Zwanzig formalism. SIAM Conference on Applications of Dynamical Systems. Snowbird, UT: SIAM.More infoThis talk was part of a minisymposium co-organized by myself and John Harlim (Penn State).
- Lin, K. (2017, May). Discrete-time approach to stochastic parameterization and model reduction. University of Chicago Scientific & Statistical Computing Seminar. University of Chicago.
- Lin, K. (2017, November). Implicit sampling in the small-noise limit. Georgia Tech Applied and Computational Mathematics Seminar. Georgia Tech.
- Lin, K. (2017, November). Mori-Zwanzig formalism and discrete-time stochastic parameterization of chaotic dynamics. West Coast Reduced-Order Modeling Workshop. Lawrence Berkeley National Lab.
- Lin, K. (2016, February). Implicit samplers in the small-noise limit. Mathematics Colloquium, Tulane University.
- Lin, K. (2016, June). Implicit samplers in the small-noise limit. Isaac Newton Institute (UK) Seminar. Cambridge University, UK.
- Lin, K. (2016, June). Implicit samplers in the small-noise limit. Statistical Mechanics Seminar, University of Warwick. University of Warwick (UK).
Poster Presentations
- Lin, K., Fellous, J., & Greene, P. (2017, 11). Spike sorting via source localization. Society for Neuroscience. Washington DC.
- Leach, A., Lin, K., & Morzfeld, M. (2016, December). Symmetrized importance samplers for uncertainty quantification and data assimilation. American Geophysical Union: General Assembly.
- Lin, K. (2016, December). Discrete-time data-driven modeling. American Geophysical Union Fall Meeting. San Francisco, CA.