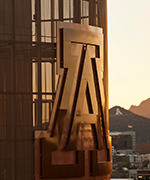
Ning Hao
- Associate Professor, Mathematics
- Associate Professor, Statistics-GIDP
- Member of the Graduate Faculty
Contact
- (520) 621-2416
- Environment and Natural Res. 2, Rm. S325
- Tucson, AZ 85719
- nhao@arizona.edu
Degrees
- Ph.D. Mathematics
- Stony Brook University, Stony Brook, New York, United States
- D-bar spark theory and Deligne cohomology
- B.S. Mathematics
- Peking University, Beijing, China
Interests
Research
High-dimensional statistical learning; Change-point detection; Bioinformatics;Geometry and topology.
Teaching
Statistics
Courses
2024-25 Courses
-
Dissertation
STAT 920 (Fall 2024) -
Independent Study
STAT 599 (Fall 2024)
2023-24 Courses
-
Dissertation
STAT 920 (Summer I 2024) -
Independent Study
MATH 599 (Spring 2024) -
Research
STAT 900 (Spring 2024) -
Statistical Computing
STAT 675 (Spring 2024) -
Thesis
STAT 910 (Spring 2024) -
Intro to Statistical Computing
DATA 375 (Fall 2023) -
Topics In Applied Math
MATH 577 (Fall 2023)
2022-23 Courses
-
Theoretical Statistics II
MATH 567B (Spring 2023) -
Dissertation
MATH 920 (Fall 2022) -
Intro to Statistical Computing
DATA 375 (Fall 2022) -
Theoretical Statistics I
MATH 567A (Fall 2022) -
Theoretical Statistics I
STAT 567A (Fall 2022)
2021-22 Courses
-
Dissertation
MATH 920 (Spring 2022) -
Intro to Statistical Computing
DATA 375 (Spring 2022) -
Dissertation
MATH 920 (Fall 2021) -
Intro to Statistical Computing
DATA 375 (Fall 2021)
2020-21 Courses
-
Dissertation
MATH 920 (Spring 2021) -
Theoretical Statistics II
MATH 567B (Spring 2021) -
Theoretical Statistics II
STAT 567B (Spring 2021) -
Calculus II
MATH 129 (Fall 2020) -
Dissertation
MATH 920 (Fall 2020) -
Theoretical Statistics I
MATH 567A (Fall 2020) -
Theoretical Statistics I
STAT 567A (Fall 2020)
2019-20 Courses
-
Independent Study
MATH 599 (Spring 2020)
2018-19 Courses
-
Calculus II
MATH 129 (Spring 2019) -
Independent Study
MATH 599 (Spring 2019) -
Intro to Statistical Computing
DATA 375 (Spring 2019) -
Independent Study
MATH 599 (Fall 2018) -
Theoretical Statistics II
MATH 567B (Fall 2018) -
Theoretical Statistics II
STAT 567B (Fall 2018)
2017-18 Courses
-
Independent Study
MATH 599 (Spring 2018) -
Theoretical Statistics I
MATH 567A (Spring 2018) -
Theoretical Statistics I
STAT 567A (Spring 2018) -
Independent Study
STAT 599 (Fall 2017) -
Intro Statistical Method
MATH 363 (Fall 2017) -
Theory of Statistics
MATH 466 (Fall 2017)
2016-17 Courses
-
Calculus II
MATH 129 (Spring 2017) -
Calculus II
MATH 129 (Fall 2016)
2015-16 Courses
-
Intro:Stat+Biostatistics
MATH 263 (Spring 2016) -
Theoretical Statistics I
MATH 567A (Spring 2016) -
Theoretical Statistics I
STAT 567A (Spring 2016)
Scholarly Contributions
Journals/Publications
- Hao, N., Niu, Y., & Xiao, H. (2023). Equivariant Variance Estimation for Multiple Change-point Model. Electronic Journal of Statistics, 17(2), 3811-3853. doi:10.1214/23-EJS2190
- Wu, R., & Hao, N. (2022). Quadratic Discriminant Analysis by Projection. Journal of Multivariate Analysis.
- Hao, N., Niu, Y., Xiao, F., & Zhang, H. (2020). A super scalable algorithm for segment detection. Statistics in Biosciences. doi:https://doi.org/10.1007/s12561-020-09278-z
- Shin, S. J., Wu, Y., & Hao, N. (2020). A Backward Procedure for Change-point Detection with Application to Copy Number Variation Detection. Canadian Journal of Statistics, 48(3), 366–385. doi:https://doi.org/10.1002/cjs.11535
- Xiao, F., Luo, X., Hao, N., Niu, Y., Xiao, X., Cai, G., Amos, C. I., & Zhang, H. (2019). An Accurate and Powerful Method for Copy Number Variation Detection. Bioinformatics, 35(17), 2891-2898. doi:https://doi.org/10.1093/bioinformatics/bty1041
- Hao, N., Feng, Y., & Zhang, H. (2018). Model Selection for High Dimensional Quadratic Regression via Regularization. Journal of the American Statistical Association, 113(522), 615-625. doi:https://doi.org/10.1080/01621459.2016.1264956
- Niu, Y., Hao, N., & Dong, B. (2018). A New Reduced-Rank Linear Discriminant Analysis Method and Its Applications. Statistica Sinica, 28, 189-202. doi:https://doi.org/10.5705/ss.202015.0387
- Niu, Y., Hao, N., & Zhang, H. (2018). Interaction Screening by Partial Correlation. Statistics and Its Interface, 11(2), 317-325. doi:http://dx.doi.org/10.4310/SII.2018.v11.n2.a9
- Hao, N., & Zhang, H. (2017). A Note on High Dimensional Regression Models with Interactions. The American Statistician, 71(4), 291-297. doi:https://doi.org/10.1080/00031305.2016.1264311
- Hao, N., & Zhang, H. (2017). Oracle P-values and Variable Screening. Electronic Journal of Statistics, 11, 3251-3271. doi:doi:10.1214/17-EJS1284
- Xiao, F., Niu, Y., Hao, N., Xu, Y., Jin, Z., & Zhang, H. (2017). modSaRa: a computationally efficient R package for CNV identification. Bioinformatics, 33(15), 2384–2385. doi:https://doi.org/10.1093/bioinformatics/btx212
- Niu, Y., Hao, N., & Zhang, H. (2016). Multiple Change-Point Detection, a Selective Overview. Statistical Science, 31(4), 611-623. doi:doi:10.1214/16-STS587
- Dong, B., & Hao, N. (2015). Semi-supervised high dimensional clustering by tight wavelet frames. SPIE Optical Engineering+ Applications.
- Hao, N., Dong, B., & Fan, J. (2015). Sparsifying the Fisher Linear Discriminant by Rotation. Journal of the Royal Statistical Society: Series B.
- Hao, N., & Zhang, H. H. (2014). Interaction Screening for Ultra-High Dimensional Data. Journal of the American Statistical Association, 109(507), 1285-1301.More infoIn ultra-high dimensional data analysis, it is extremely challenging to identify important interaction effects, and a top concern in practice is computational feasibility. For a data set with n observations and p predictors, the augmented design matrix including all linear and order-2 terms is of size n × (p (2) + 3p)/2. When p is large, say more than tens of hundreds, the number of interactions is enormous and beyond the capacity of standard machines and software tools for storage and analysis. In theory, the interaction selection consistency is hard to achieve in high dimensional settings. Interaction effects have heavier tails and more complex covariance structures than main effects in a random design, making theoretical analysis difficult. In this article, we propose to tackle these issues by forward-selection based procedures called iFOR, which identify interaction effects in a greedy forward fashion while maintaining the natural hierarchical model structure. Two algorithms, iFORT and iFORM, are studied. Computationally, the iFOR procedures are designed to be simple and fast to implement. No complex optimization tools are needed, since only OLS-type calculations are involved; the iFOR algorithms avoid storing and manipulating the whole augmented matrix, so the memory and CPU requirement is minimal; the computational complexity is linear in p for sparse models, hence feasible for p ≫ n. Theoretically, we prove that they possess sure screening property for ultra-high dimensional settings. Numerical examples are used to demonstrate their finite sample performance.
- Hao, N., Niu, Y. S., & Zhang, H. (2013). Multiple Change-Point Detection via a Screening and Ranking Algorithm. Statistica Sinica, 23(4), 1553-1572.More infoLet Y 1, …, Yn be a sequence whose underlying mean is a step function with an unknown number of the steps and unknown change points. The detection of the change points, namely the positions where the mean changes, is an important problem in such fields as engineering, economics, climatology and bioscience. This problem has attracted a lot of attention in statistics, and a variety of solutions have been proposed and implemented. However, there is scant literature on the theoretical properties of those algorithms. Here, we investigate a recently developed algorithm called the Screening and Ranking Algorithm (SaRa). We characterize the theoretical properties of SaRa and show its superiority over other commonly used algorithms. In particular, we develop a false discovery rate approach to the multiple change-point problem and show a strong sure coverage property for the SaRa.
- Fan, J., Guo, S., & Hao, N. (2012). Variance estimation using refitted cross-validation in ultrahigh dimensional regression. Journal of the Royal Statistical Society. Series B: Statistical Methodology, 74(1), 37-65.More infoAbstract: Variance estimation is a fundamental problem in statistical modelling. In ultrahigh dimensional linear regression where the dimensionality is much larger than the sample size, traditional variance estimation techniques are not applicable. Recent advances in variable selection in ultrahigh dimensional linear regression make this problem accessible. One of the major problems in ultrahigh dimensional regression is the high spurious correlation between the unobserved realized noise and some of the predictors. As a result, the realized noises are actually predicted when extra irrelevant variables are selected, leading to a serious underestimate of the level of noise. We propose a two-stage refitted procedure via a data splitting technique, called refitted cross-validation, to attenuate the influence of irrelevant variables with high spurious correlations. Our asymptotic results show that the resulting procedure performs as well as the oracle estimator, which knows in advance the mean regression function. The simulation studies lend further support to our theoretical claims. The naive two-stage estimator and the plug-in one-stage estimators using the lasso and smoothly clipped absolute deviation are also studied and compared. Their performances can be improved by the refitted cross-validation method proposed. © 2011 Royal Statistical Society.
Creative Productions
- Hao, N., Niu, Y., Xiao, F., & Zhang, H. (2018. R Package: SSSS. https://publichealth.yale.edu/c2s2/software/SSSS/.
- Shin, S. J., Wu, Y., & Hao, N. (2018. R Package: bwd. https://cran.r-project.org/web/packages/bwd/index.html.
- Xiao, F., Luo, X., Hao, N., Niu, Y., Xiao, X., Cai, G., Amos, C. I., & Zhang, H. (2018. R Package: modSaRa2. https://publichealth.yale.edu/c2s2/software/modSaRa2/.