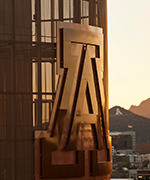
Hao Zhang
- Professor, Mathematics
- Professor, Statistics-GIDP
- Professor, Applied Mathematics - GIDP
- Member of the Graduate Faculty
- Chair, Statistics-GIDP
Contact
- (520) 621-6868
- Environment and Natural Res. 2, Rm. S323
- Tucson, AZ 85719
- haozhang@arizona.edu
Degrees
- Ph.D. Statistics
- University of Wisconsin at Madison, Madison, Wisconsin, USA
- Nonparametric Variable Selection and Model Building via Likelihood Basis Pursuit.
- B.S. Mathematics
- Peking University, Beijing, China
Work Experience
- North Carolina State University (2008 - 2011)
- North Carolina State University (2002 - 2008)
Awards
- Woman of Impact Award
- University of Arizona, Fall 2024
- Fellow member
- Mu Sigma Rho Chapter (at the University of Arizona), Fall 2021
- Renewed Editor-in-Chief Appointment for STAT
- International Statistical Institute, Winter 2020
- Galileo Circle Fellow Award
- College of Science, University of Arizona, Fall 2018
- Appointed as Editor-in-Chief for STAT
- International Statistical Institute, Fall 2017
- Medallion Lecturer
- Institute of Mathematical Statistics (IMS), Summer 2017
- Fellow
- Institute of Mathematical Statistics (IMS), Summer 2016
- American Statistical Association (ASA), Spring 2015
- Elected Member
- International Statistical Institute (ISI), Summer 2015
Interests
Teaching
Theory of Statistics, Statistical Machine Learning, Nonparametric Smoothing
Research
statistical machine learning, high dimensional data analysis, nonparametric regression and smoothing, variables selection, dimension reduction
Courses
2024-25 Courses
-
Dissertation
STAT 920 (Spring 2025) -
Honors Thesis
DATA 498H (Spring 2025) -
Intro Stat Machine Learning
DATA 474 (Spring 2025) -
Thesis
STAT 910 (Spring 2025) -
Dissertation
STAT 920 (Fall 2024) -
Honors Thesis
DATA 498H (Fall 2024) -
Research
STAT 900 (Fall 2024) -
Statistical Machine Learning
MATH 574M (Fall 2024) -
Thesis
STAT 910 (Fall 2024)
2023-24 Courses
-
Independent Study
STAT 599 (Summer I 2024) -
Thesis
STAT 910 (Summer I 2024) -
Capstone: Stats/Data Science
DATA 498A (Spring 2024) -
Directed Research
MATH 492 (Spring 2024) -
Dissertation
MATH 920 (Spring 2024) -
Independent Study
STAT 599 (Spring 2024) -
Research
STAT 900 (Spring 2024) -
Thesis
STAT 910 (Spring 2024) -
Dissertation
MATH 920 (Fall 2023) -
Independent Study
STAT 599 (Fall 2023) -
Statistical Machine Learning
MATH 574M (Fall 2023)
2022-23 Courses
-
Independent Study
STAT 599 (Summer I 2023) -
Capstone: Stats/Data Science
DATA 498A (Spring 2023) -
Dissertation
STAT 920 (Spring 2023) -
Independent Study
MATH 599 (Spring 2023) -
Dissertation
STAT 920 (Fall 2022) -
Independent Study
MATH 599 (Fall 2022) -
Statistical Machine Learning
MATH 574M (Fall 2022)
2021-22 Courses
-
Capstone: Stats/Data Science
DATA 498A (Spring 2022) -
Dissertation
STAT 920 (Spring 2022) -
Independent Study
MATH 599 (Spring 2022) -
Dissertation
STAT 920 (Fall 2021) -
Independent Study
MATH 599 (Fall 2021) -
Independent Study
STAT 599 (Fall 2021) -
Statistical Machine Learning
MATH 574M (Fall 2021) -
Thesis
STAT 910 (Fall 2021)
2020-21 Courses
-
Capstone: Stats/Data Science
DATA 498A (Spring 2021) -
Dissertation
STAT 920 (Spring 2021) -
Independent Study
MATH 599 (Spring 2021) -
Thesis
MATH 910 (Spring 2021) -
Thesis
STAT 910 (Spring 2021) -
Dissertation
MATH 920 (Fall 2020) -
Dissertation
STAT 920 (Fall 2020) -
Research
STAT 900 (Fall 2020) -
Statistical Machine Learning
MATH 574M (Fall 2020)
2019-20 Courses
-
Capstone: Stats/Data Science
DATA 498A (Spring 2020) -
Dissertation
STAT 920 (Spring 2020) -
Independent Study
MATH 499 (Spring 2020) -
Research
MATH 900 (Spring 2020) -
Research
STAT 900 (Spring 2020) -
Statistical Machine Learning
MATH 574M (Spring 2020) -
Dissertation
STAT 920 (Fall 2019) -
Independent Study
STAT 599 (Fall 2019) -
Internship
MATH 593 (Fall 2019) -
Research
MATH 900 (Fall 2019) -
Research
STAT 900 (Fall 2019)
2018-19 Courses
-
Independent Study
STAT 599 (Spring 2019) -
Research
MATH 900 (Spring 2019) -
Statistical Machine Learning
MATH 574M (Spring 2019) -
Theory of Statistics
MATH 566 (Spring 2019) -
Theory of Statistics
STAT 566 (Spring 2019) -
Independent Study
STAT 599 (Fall 2018) -
Research
MATH 900 (Fall 2018)
2017-18 Courses
-
Dissertation
STAT 920 (Spring 2018) -
Independent Study
MATH 599 (Spring 2018) -
Research
MATH 900 (Spring 2018) -
Theory of Statistics
MATH 566 (Spring 2018) -
Theory of Statistics
STAT 566 (Spring 2018) -
Dissertation
STAT 920 (Fall 2017) -
Statistical Machine Learning
MATH 574M (Fall 2017)
2016-17 Courses
-
Dissertation
MATH 920 (Spring 2017) -
Dissertation
STAT 920 (Spring 2017) -
Theory of Statistics
MATH 466 (Spring 2017) -
Theory of Statistics
MATH 566 (Spring 2017) -
Theory of Statistics
STAT 566 (Spring 2017) -
Dissertation
STAT 920 (Fall 2016) -
Statistical Machine Learning
MATH 574M (Fall 2016)
2015-16 Courses
-
Dissertation
MATH 920 (Spring 2016) -
Dissertation
STAT 920 (Spring 2016) -
Theory of Statistics
MATH 466 (Spring 2016) -
Theory of Statistics
MATH 566 (Spring 2016) -
Theory of Statistics
STAT 566 (Spring 2016)
Scholarly Contributions
Books
- Lee, T. C., Zhang, H., Levine, R. A., & Piegorsch, W. W. (2022). Computational Statistics in Data Science. Chichester: John Wiley & Sons.
Chapters
- Zhang, H. (2021). Model Selection in High-Dimensional Regression. In Computational Statistics in Data Science.
- Zhang, H. (2018). Nonparametric methods for big data analytics.. In Handbook of Big Data(pp 103-124).
- Zhang, H. (2017). Supervised learning. In Wiley StatsRef (WSR)-Statistics Reference Online.
Journals/Publications
- Cai, R., Yang, X., Ma, Y., Zhang, H., Olatosi, B., Weissman, S., Li, X., & Zhang, J. (2024). Use of machine learning approaches to predict transition of retention in care among people living with HIV in Sout Carolina: a real-world data study.. AIDS Care, 36(12), 1745-1753.
- Ebrahimi, M., Chai, Y., Zhang, H., & Chen, H. (2021). Heterogeneous Domain Adaptation with Adversarial Neural Representation Learning: Experiments on E-Commerce and Cybersecurity. IEEE TRANSACTIONS ON PATTERN ANALYSIS AND MACHINE INTELLIGENCE.
- Hao, N., Feng, Y., & Zhang, H. (2015). Model Selection for High Dimensional Quadratic Regression via Regularization. Journal of American Statistical Association.
- Hao, N., Feng, Y., & Zhang, H. (2015). Model Selection for High Dimensional Quadratic Regression via Regularization. Journal of the American Statistical Association.
- Hara, A., Lu, E., Johnstone, L., Wei, M., Sun, S., Hallmark, B., Watkins, J., Zhang, H. H., Yao, G., & Chilton, F. (2024). Identification of an allele-specific transcription factor binding interaction that may regulate PLA2G2A gene expression.. Bioinformatics and Biological Insights.
- Kong, D., Xue, K., Yao, F., & Zhang, H. (2015). Partially functional linear regression in high dimensions. Biometrika.
- Li, Q., Lussier, Y. A., Rachid Zaim, S., Zhang, H., Kenost, C., Berghout, J., Vitali, F., Vitali, F., Kenost, C., Berghout, J., Rachid Zaim, S., Zhang, H., Li, Q., & Lussier, Y. A. (2019). iDEG: a single-subject method utilizing local estimates of dispersion to impute differential expression between two transcriptomes. BMC Medical Genomics. doi:http://dx.doi.org/10.1101/405332
- Li, Q., Schissler, A. G., Gardeux, V., Achour, I., Kenost, C., Li, H., Zhang, H., & Lussier, Y. A. (2016). Interpreting transcriptome dynamics for precision medicine: analyzing noisy and bidirectional pathway mRNA signals in single subjects. Journal of Biomedical Informatics.
- Li, Q., Schissler, A. G., Li, H., Zhang, H., & Lussier, Y. A. (2016). N-of-1-pathways MixEnrich robustly detects personal dynamic transcriptomic changes. BMC Bioinformatics.
- Lu, Z., Hao, N., & Zhang, H. (2024). Simultaneous change-point detection and curve estimation.. Statistics and Its Interface, 17, 493-500.
- Rachid Zaim, S., Kenost, C., Berghout, J., Vitali, F., Zhang, H., & Lussier, Y. A. (2018). Evaluating single-subject study methods for personal transcriptomic interpretation to advance precision medicine. BMC Medical Genomics. doi:https://doi.org/10.1101/428581
- Sharma, Y., Chen, X., Wu, J., Zhou, J., Zhang, H., & Xin, H. (2021). Machine Learning Methods-based Modeling and Optimization of 3-D-Printed Dielectrics around Monopole Antenna.. IEEE Transactions on Antennas and Propagation.
- Sun, S., Hara, A., Johnstone, L., Hallmark, B. R., Liu, E., Watkins, J. C., Thomson, C. A., Schembre, S. M., Sergeant, S., Umans, J., Yao, G., Zhang, H., & Chilton, F. (2024). Optimal Pair Matching Combined with Machine Learning Predicts that Omega-3 Fatty Acid Supplementation Markedly Reduces the Risk of Myocardial Infarction in African Americans. Nutrients, 16(2933).More infoConflicting results from clinical trials have contributed to a lack of consensus about cardioprotective effects of omega-3 (n-3) highly unsaturated fatty acids (HUFA). Although the VITAL trial did not demonstrate an overall benefit of n-3 HUFA supplementation on composite cardiovascular disease (CVD) and cancer outcomes, it afforded an unique opportunity for a post-hoc analysis of racial/ethnic differences in the supplementation response, given the substantial enrollment of African Americans (AfAm). We employed propensity score matching to address potential covariate imbalances between AfAm and European American (EuAm) subgroups, analyzing data from 3,766 participants across both groups. Using Kaplan-Meier curves and two machine learning methodologies, we found that n-3 HUFA supplementation was significantly associated with a reduced risk of myocardial infarction (MI) exclusively in the AfAm subgroup, as evidenced by an odds ratio of 0.17 (95% CI [0.048, 0.59]). These findings indicate a potential cardioprotective benefit of n-3 supplementation in AfAm, specifically in reducing MI risks. Considering the significant association identified, further investigation through a hypothesis-driven randomized clinical trial is needed to explore the possibility of race-specific recommendations for n-3 HUFA supplementation.
- Wang, J., Wang, H., & Zhang, H. (2024). Scale-invariant optimal sampling for rare-events data and sparse model.. NeurIPS.
- Wang, Z., Fang, Y., Liu, Z., Hao, N., Zhang, H., Sun, X., Que, J., & Ding, H. (2024). Adapting Nanopore Sequencing Basecalling Models for Modification Detection via Incremental Learning and Anomaly Detection.. Nature Communications., 15, 7148.
- Xiao, W., Lu, W., & Zhang, H. (2015). Joint structure selection and estimation in the time-varying coefficient Cox model.. Statistica Sinica.
- Zaim, S., Kenost, C., Zhang, H., & Lussier, Y. (2020). Personalized beyond precision: designing unbiased gold standards to improve single-subject studies of personal genome dynamics from gene products.. Journal of Personalized Medicine.
- Zeng, L., & Zhang, H. (2023). Linear algorithms for robust and scalable nonparametric multiclass probability estimation.. Journal of Data Sciene.
- Zeng, L., & Zhang, H. (2024). Robust brain MRI image classification with SIBOW-SVM.. Computerized Medical Imaging and Graphics, 118, 102451.
- Zhang, H., Hao, N., & Niu, Y. (2017). Interaction Screening by Partial Correlation. Statistics and Its Interface.
- Wang, X., Zhu, Z., & Zhang, H. (2023). Spatial Heterogeneity Automatic Detection and Estimation. Computational Statistics and Data Analysis, 180, 107667.
- Ebrahimi, M., Chen, Y., Zhang, H., & Chen, H. (2023). Heterogeneous domain adaptation with adversarial neural representation learning: experiments on e-commerce and cybersecurity.. IEEE Transactions on Pattern Analysis and Machine Intelligence., 45 (2), 1862-1875. doi:10.1109/TPAMI.2022.3163338.
- Lo-Ciganic, W., Donohue, J., Yang, Q., Huang, J., Chang, C., Weiss, J., Guo, J., Zhang, H., Cochran, G., Gordon, A., Malone, D., Kwoh, C., Wilson, D., Kuza, C., & Gellad, W. (2022).
Developing and validating a machine-learning algorithm to predict opioid overdose among Medicaid beneficiaries in two US states: a prognostic modeling study.
. The Lancet Digital Health, 4, E455-E465. doi:https://doi.org/10.1016/S2589-7500(22)00062-0 - Sharma, Y., Chen, X., Wu, J., Zhou, Q., Zhang, H., & Hao, X. (2022). Machine learning methods-based modeling and optimization of 3-D-printed dielectrics around monopole antenna.. IEEE Transactions on Antennas and Propagation., 70(7), 4997-5006. doi:10.1109/TAP.2022.3153688.
- Li, N., & Zhang, H. (2021). Sparse Learning with Non-convex Penalty in Multi-classification. Journal of Data Science, 19, 56-74.
- Russell, S., Barton, J. K., Rodriguez, G., Zhang, H., & Alberts, D. S. (2021). Karyometry Identifies a Distinguishing Fallopian Tube Epithelium Phenotype in Subjects at High Risk for Ovarian Cancer. Analytical and Quantitative Cytopathology and Histopathology, 43(2), 44-51.
- Zaim, S., Kenost, C., Zhang, H., & Lussier, Y. (2020). Personalized beyond precision: designing unbiased gold standards to improve single-subject studies of personal genome dynamics from gene products. Journal of Personalized Medicine, 11(1), 24. doi:10.3390/jpm11010024
- Baldwin, E., Li, H., Han, J., Zhang, H., Luo, W., Liu, J., An, L., Zhou, J., Zhou, J., An, L., Luo, W., Liu, J., Han, J., Zhang, H., Baldwin, E., & Li, H. (2020). On fusion methods for knowledge discovery from multi-omics datasets. Computational and structural biotechnology journal, 18, 509–517. doi:https://doi.org/10.1016/j.csbj.2020.02.011
- Lo-Ciganic, W. H., Huang, J. L., Zhang, H. H., Weiss, J. C., Kwoh, C. K., Donohue, J. M., Gordon, A. J., Cochran, G., Malone, D. C., Kuza, C. C., & Gellad, W. F. (2020). Using machine learning to predict risk of incident opioid use disorder among fee-for-service Medicare beneficiaries: A prognostic study. PloS one, 15(7), e0235981.More infoTo develop and validate a machine-learning algorithm to improve prediction of incident OUD diagnosis among Medicare beneficiaries with ≥1 opioid prescriptions.
- Sharma, Y., Zhang, H., & Xin, H. (2020). Machine Learning Techniques for Optimizing Design of Double T-Shaped Monopole Antenna,. IEEE Transactions on Antennas and Propagation, 68, 5658-5663.
- Zaim, S., Kenost, C., Berghout, J., Chiu, W., Zhang, H., & Lussier, Y. (2020). binomialRF: interpretable combinatoric efficiency of random forests to identify biomarker interactions. BMC Bioinformatics, 21(1), 374.
- Garland, L., Guillen-Rodriguez, J., Hsu, C., Yozwiak, M., Zhang, H., & Alberts, D. (2019). Effect of Intermittent Versus Continuous Low-Dose Aspirin on Nasal Epithelium Gene Expression in Current Smokers: A Randomized, Double-Blinded Trial. Cancer Prevention Research, 12, 809-820.
- Huang, D., Lan, W., Zhang, H., & Wang, H. (2019). Least squares estimation of spatial autoregressive models for large-scale social networks. Electronic Journal of Statistics, 13(1), 1135-1165.
- Lo-Ciganic, W., Huang, J., Zhang, H., Weiss, J., Wu, Y., & Walid, G. (2019). Evaluation of Machine-Learning Algorithms for Predicting Opioid Overdose Risk Among Medicare Beneficiaries With Opioid Prescriptions.. JAMA New Open.
- Rachid Zaim, S., Kenost, C., Berghout, J., Vitali, F., Zhang, H., & Lussier, Y. A. (2019). Evaluating single-subject study methods for personal transcriptomic interpretation to advance precision medicine. BMC Medical Genomics, 12(Suppl 5), 96. doi:doi:10.1186/s12920-019-0513-8
- Rodriguez, G., Kauderer, J., Hunn, J., Thaete, L., Watkin, W., & Zhang, H. (2019). Phase II Trial of Chemopreventive Effects of Levonorgestrel on Ovarian and Fallopian Tube Epithelium in Women at High Risk for Ovarian Cancer: An NRG Oncology Group/GOG Study.. Cancer Prevention Research, 12(6), 401-412.
- Wang, X., Zhang, H., & Wu, Y. (2019). Multiclass Probability Estimation With Support Vector Machines. Journal of Computational and Graphical Statistics, 28, 586-595.
- Xiao, W., Zhang, H., & Lu, W. (2019). Robust Regression for Optimal Individualized Treatment Rules. Statistics in medicine, 38(11), 2059-2073.
- Hao, N., Feng, Y., & Zhang, H. (2018). Model Selection for High Dimensional Quadratic Regression via Regularization. Journal of the American Statistical Association, 113(522), 615-625. doi:https://doi.org/10.1080/01621459.2016.1264956
- Zhang, H. (2018). Discussion on "Doubly sparsity kernel learning with automatic variable selection and data extraction". Statistics and Its Interface, 11, 425-428.
- Zhang, H., Niu, Y., Hao, N., Hao, N., Niu, Y., & Zhang, H. (2018). Interaction Screening by Partial Correlation. Statistics and Its Interface, 11(2), 317-325. doi:http://dx.doi.org/10.4310/SII.2018.v11.n2.a9
- Li, Q., Schissler, G., Gardeux, V., Berghout, J., Achour, I., Kenost, C., Li, H., Zhang, H., & Luisser, Y. A. (2017). kMEn: analyzing noisy and bidirectional transcriptional pathway responses in single subjects. Journal of Biomedical Informatics, 66, 32-41.
- Lussier, Y. A., Zhang, H. H., Li, H., Berghout, J., Kenost, C., Achour, I., Gardeux, V., Schissler, A. G., & Li, Q. (2017). N-of-1-pathways MixEnrich: advancing precision medicine via single-subject analysis in discovering dynamic changes of transcriptomes. BMC medical genomics, 10(Suppl 1), 27.More infoTranscriptome analytic tools are commonly used across patient cohorts to develop drugs and predict clinical outcomes. However, as precision medicine pursues more accurate and individualized treatment decisions, these methods are not designed to address single-patient transcriptome analyses. We previously developed and validated the N-of-1-pathways framework using two methods, Wilcoxon and Mahalanobis Distance (MD), for personal transcriptome analysis derived from a pair of samples of a single patient. Although, both methods uncover concordantly dysregulated pathways, they are not designed to detect dysregulated pathways with up- and down-regulated genes (bidirectional dysregulation) that are ubiquitous in biological systems.
- Shin, S., Wu, Y., Zhang, H., & Liu, Y. (2017). Principal weighted support vector machines for sufficient dimension reduction in binary classification. Biometrika, 104(1), 67-81.
- Shin, S., Zhang, H., & Wu, Y. (2017). A nonparametric survival function estimator via censored kernel quantile regression. Statistica Sinca, 27(1), 457-478.
- Song, R., Luo, S., Zeng, D., Zhang, H., Lu, W., & Li, Z. (2017). Semiparametric single-index model for estimating optimal individualized treatment strategy. Electronic Journal of Statistics, 11(1), 364-384. doi:10.1214/17-EJS1226
- Wang, X., Fujimaki, K., Mitchell, G., Kwon, J., Croce, K., Langsdorf, C., Zhang, H., & Yao, G. (2017). Exit from quiescence displays a memory of cell growth and division. Nature Communications, 8(1), 321.
- Zhang, H., & Hao, N. (2017). A Note on High Dimensional Regression Models with Interactions. The American Statistician, 71(4), 291-297. doi:https://doi.org/10.1080/00031305.2016.1264311
- Zhang, H., & Hao, N. (2017). Oracle P-values and Variable Screening. Electronic Journal of Statistics, 11, 3251-3271. doi:doi:10.1214/17-EJS1284
- Zhang, H., Feng, Y., & Hao, N. (2017). Model Selection for High Dimensional Quadratic Regression via Regularization. Journal of the American Statistical Association.
- Ghosal, S., Turnbull, B., Zhang, H. H., & Hwang, W. Y. (2016). Sparse Penalized Forward Selection for Support Vector Classification. JOURNAL OF COMPUTATIONAL AND GRAPHICAL STATISTICS, 25(2), 493-514.
- Glazer, E. S., Zhang, H. H., Hill, K. A., Patel, C., Kha, S. T., Yozwiak, M. L., Bartels, H., Nafissi, N. N., Watkins, J. C., Alberts, D. S., & Krouse, R. S. (2016). Evaluating IPMN and pancreatic carcinoma utilizing quantitative histopathology. Cancer medicine, 5(10), 2841-2847.
- He, Q., Zhang, H. H., Avery, C. L., & Lin, D. Y. (2016). Sparse meta-analysis with high-dimensional data. Biostatistics (Oxford, England), 17(2), 205-20.
- Kong, D., Xue, K., Yao, F., & Zhang, H. H. (2016). Partially functional linear regression in high dimensions. BIOMETRIKA, 103(1), 147-159.
- Li, Q., Schissler, A. G., Gardeux, V., Berghout, J., Achour, I., Kenost, C., Li, H., Zhang, H. H., & Lussier, Y. A. (2016). kMEn: analyzing noisy and bidirectional transcriptional pathway responses in single subjects. Journal of Biomedical Informatics, 66, 32-41. doi:http://dx.doi.org/10.1016/j.jbi.2016.12.009
- Xiao, W., Lu, W., & Zhang, H. H. (2016). JOINT STRUCTURE SELECTION AND ESTIMATION IN THE TIME-VARYING COEFFICIENT COX MODEL. Statistica Sinica, 26(2), 547-567.
- Zhang, H. H. (2016). Comments on: Probability Enhanced Effective Dimension Reduction for Classifying Sparse Functional Data. Test (Madrid, Spain), 25(1), 47-51.
- Cheng, G., Zhang, H., & Shang, Z. (2015). Sparse and efficient estimation for partial spline models with increasing dimension. Annals of the Institute of Statistical Mathematics, 67, 93-127.
- Geng, Y., Lu, W., & Zhang, H. (2015). On optimal treatment regimes selection for mean survival time.. Statistics in Medicine, 34, 1169-1184.
- Li, H., Pouladi, N., Achour, I., Gardeux, V., Li, J., Li, Q., Zhang, H. H., Martinez, F., Garcia, J. G., & Lussier, Y. A. (2015). eQTL networks unveil enriched mRNA master integrators downstream of complex disease-associated SNPs.. Journal of Biomedical Informatics.
- Avery, M., Wu, Y., Zhang, H., & Zhang, J. (2014). RKHS-based functional nonlinear regres- sion for sparse and irregular longitudinal data.. Canadian Journal of Statistics, 42, 204-216.
- Caner, M., & Zhang, H. (2014). Adaptive elastic net for generalized methods of moments.. Journal of Business & Economic Statistics, 32, 30-47.
- Hao, N., & Zhang, H. (2014). Interaction screening for ultra-high dimensional data.. Journal of American Statistical Association, 109, 1285-1301.
- Ma, C., Zhang, H., & Wang, X. (2014). Machine learning for big data analytics in plants.. Trends in Plant Science, 19, 798-808.
- Shin, S., Wu, Y., & Zhang, H. (2014). Two-dimensional solution surface for weighted support vector machines.. Journal of Computational and Graphical Statistics, 23, 383-402.
- Shin, S., Wu, Y., Zhang, H., & Liu, Y. (2014). Probability-enhanced sufficient dimension reduction for binary classification.. Biometrics, 70, 546-555.
- Zhu, H., Yao, F., & Zhang, H. (2014). Structured functional additive regression in reproducing kernel Hilbert spaces.. Journal of the Royal Statistical Society, Series B, 76, 581-603.
- Cheng, G., Zhang, H. H., & Shang, Z. (2013). Sparse and efficient estimation for partial spline models with increasing dimension. Annals of the Institute of Statistical Mathematics, 1-35.More infoAbstract: We consider model selection and estimation for partial spline models and propose a new regularization method in the context of smoothing splines. The regularization method has a simple yet elegant form, consisting of roughness penalty on the nonparametric component and shrinkage penalty on the parametric components, which can achieve function smoothing and sparse estimation simultaneously. We establish the convergence rate and oracle properties of the estimator under weak regularity conditions. Remarkably, the estimated parametric components are sparse and efficient, and the nonparametric component can be estimated with the optimal rate. The procedure also has attractive computational properties. Using the representer theory of smoothing splines, we reformulate the objective function as a LASSO-type problem, enabling us to use the LARS algorithm to compute the solution path. We then extend the procedure to situations when the number of predictors increases with the sample size and investigate its asymptotic properties in that context. Finite-sample performance is illustrated by simulations. © 2013 The Institute of Statistical Mathematics, Tokyo.
- Sharma, D. B., Bondell, H. D., & Zhang, H. H. (2013). Consistent group identification and variable selection in regression with correlated predictors. Journal of Computational and Graphical Statistics, 22(2), 319-340.More infoAbstract: Statistical procedures for variable selection have become integral elements in any analysis. Successful procedures are characterized by high predictive accuracy, yielding interpretable models while retaining computational efficiency. Penalized methods that perform coefficient shrinkage have been shown to be successful in many cases. Models with correlated predictors are particularly challenging to tackle. We propose a penalization procedure that performs variable selection while clustering groups of predictors automatically. The oracle properties of this procedure, including consistency in group identification, are also studied. The proposed method compares favorably with existing selection approaches in both prediction accuracy and model discovery, while retaining its computational efficiency. Supplementary materials are available online. © 2013 American Statistical Association, Institute of Mathematical Statistics, and Interface Foundation of North America.
- Turnbull, B., Ghosal, S., & Zhang, H. H. (2013). Iterative selection using orthogonal regression techniques. Statistical Analysis and Data Mining, 6(6), 557-564.More infoAbstract: High dimensional data are nowadays encountered in various branches of science. Variable selection techniques play a key role in analyzing high dimensional data. Generally two approaches for variable selection in the high dimensional data setting are considered-forward selection methods and penalization methods. In the former, variables are introduced in the model one at a time depending on their ability to explain variation and the procedure is terminated at some stage following some stopping rule. In penalization techniques such as the least absolute selection and shrinkage operator (LASSO), as optimization procedure is carried out with an added carefully chosen penalty function, so that the solutions have a sparse structure. Recently, the idea of penalized forward selection has been introduced. The motivation comes from the fact that the penalization techniques like the LASSO give rise to closed form expressions when used in one dimension, just like the least squares estimator. Hence one can repeat such a procedure in a forward selection setting until it converges. The resulting procedure selects sparser models than comparable methods without compromising on predictive power. However, when the regressor is high dimensional, it is typical that many predictors are highly correlated. We show that in such situations, it is possible to improve stability and computational efficiency of the procedure further by introducing an orthogonalization step. At each selection step, variables potentially available to be selected in the model are screened on the basis of their correlation with variables already in the model, thus preventing unnecessary duplication. The new strategy, called the Selection Technique in Orthogonalized Regression Models (STORM), turns out to be extremely successful in reducing the model dimension further and also leads to improved predicting power. We also consider an aggressive version of the STORM, where a potential predictor will be permanently removed from further consideration if its regression coefficient is estimated as zero at any stage. We shall carry out a detailed simulation study to compare the newly proposed method with existing ones and analyze a real dataset. © 2013 Wiley Periodicals, Inc., A Wiley Company.
- Wenbin, L. u., Zhang, H. H., & Zeng, D. (2013). Variable selection for optimal treatment decision. Statistical Methods in Medical Research, 22(5), 493-504.More infoPMID: 22116341;PMCID: PMC3303960;Abstract: In decision-making on optimal treatment strategies, it is of great importance to identify variables that are involved in the decision rule, i.e. those interacting with the treatment. Effective variable selection helps to improve the prediction accuracy and enhance the interpretability of the decision rule. We propose a new penalized regression framework which can simultaneously estimate the optimal treatment strategy and identify important variables. The advantages of the new approach include: (i) it does not require the estimation of the baseline mean function of the response, which greatly improves the robustness of the estimator; (ii) the convenient loss-based framework makes it easier to adopt shrinkage methods for variable selection, which greatly facilitates implementation and statistical inferences for the estimator. The new procedure can be easily implemented by existing state-of-art software packages like LARS. Theoretical properties of the new estimator are studied. Its empirical performance is evaluated using simulation studies and further illustrated with an application to an AIDS clinical trial. © The Authors 2011 Reprints and permissions: sagepub.co.uk/journalsPermissions.nav.
- Ahn, M., Zhang, H. H., & Wenbin, L. u. (2012). Moment-based method for random effects selection in linear mixed models. Statistica Sinica, 22(4), 1539-1562.More infoAbstract: The selection of random effects in linear mixed models is an important yet challenging problem in practice. We propose a robust and unified framework for automatically selecting random effects and estimating covariance components in linear mixed models. A moment-based loss function is first constructed for estimating the covariance matrix of random effects. Two types of shrinkage penalties, a hard thresholding operator and a new sandwich-type soft-thresholding penalty, are then imposed for sparse estimation and random effects selection. Compared with existing approaches, the new procedure does not require any distributional assumption on the random effects and error terms. We establish the asymptotic properties of the resulting estimator in terms of its consistency in both random effects selection and variance component estimation. Optimization strategies are suggested to tackle the computational challenges involved in estimating the sparse variance-covariance matrix. Furthermore, we extend the procedure to incorporate the selection of fixed effects as well. Numerical results show the promising performance of the new approach in selecting both random and fixed effects, and consequently, improving the efficiency of estimating model parameters. Finally, we apply the approach to a data set from the Amsterdam Growth and Health study.
- Cai, N., Wenbin, L. u., & Zhang, H. H. (2012). Time-Varying Latent Effect Model for Longitudinal Data with Informative Observation Times. Biometrics, 68(4), 1093-1102.More infoPMID: 23025338;PMCID: PMC3543780;Abstract: In analysis of longitudinal data, it is not uncommon that observation times of repeated measurements are subject-specific and correlated with underlying longitudinal outcomes. Taking account of the dependence between observation times and longitudinal outcomes is critical under these situations to assure the validity of statistical inference. In this article, we propose a flexible joint model for longitudinal data analysis in the presence of informative observation times. In particular, the new procedure considers the shared random-effect model and assumes a time-varying coefficient for the latent variable, allowing a flexible way of modeling longitudinal outcomes while adjusting their association with observation times. Estimating equations are developed for parameter estimation. We show that the resulting estimators are consistent and asymptotically normal, with variance-covariance matrix that has a closed form and can be consistently estimated by the usual plug-in method. One additional advantage of the procedure is that it provides a unified framework to test whether the effect of the latent variable is zero, constant, or time-varying. Simulation studies show that the proposed approach is appropriate for practical use. An application to a bladder cancer data is also given to illustrate the methodology. © 2012, The International Biometric Society.
- Yuan, S., Zhang, H. H., & Davidian, M. (2012). Variable selection for covariate-adjusted semiparametric inference in randomized clinical trials. Statistics in Medicine, 31(29), 3789-3804.More infoPMID: 22733628;PMCID: PMC3855673;Abstract: Extensive baseline covariate information is routinely collected on participants in randomized clinical trials, and it is well recognized that a proper covariate-adjusted analysis can improve the efficiency of inference on the treatment effect. However, such covariate adjustment has engendered considerable controversy, as post hoc selection of covariates may involve subjectivity and may lead to biased inference, whereas prior specification of the adjustment may exclude important variables from consideration. Accordingly, how to select covariates objectively to gain maximal efficiency is of broad interest. We propose and study the use of modern variable selection methods for this purpose in the context of a semiparametric framework, under which variable selection in modeling the relationship between outcome and covariates is separated from estimation of the treatment effect, circumventing the potential for selection bias associated with standard analysis of covariance methods. We demonstrate that such objective variable selection techniques combined with this framework can identify key variables and lead to unbiased and efficient inference on the treatment effect. A critical issue in finite samples is validity of estimators of uncertainty, such as standard errors and confidence intervals for the treatment effect. We propose an approach to estimation of sampling variation of estimated treatment effect and show its superior performance relative to that of existing methods. © 2012 John Wiley & Sons, Ltd.
- Liu, Y., Zhang, H. H., & Yichao, W. u. (2011). Hard or soft classification? large-margin unified machines. Journal of the American Statistical Association, 106(493), 166-177.More infoAbstract: Margin-based classifiers have been popular in both machine learning and statistics for classification problems. Among numerous classifiers, some are hard classifiers while some are soft ones. Soft classifiers explicitly estimate the class conditional probabilities and then perform classification based on estimated probabilities. In contrast, hard classifiers directly target the classification decision boundary without producing the probability estimation. These two types of classifiers are based on different philosophies and each has its own merits. In this article, we propose a novel family of large-margin classifiers, namely large-margin unified machines (LUMs), which covers a broad range of margin-based classifiers including both hard and soft ones. By offering a natural bridge from soft to hard classification, the LUM provides a unified algorithm to fit various classifiers and hence a convenient platform to compare hard and soft classification. Both theoretical consistency and numerical performance of LUMs are explored. Our numerical study sheds some light on the choice between hard and soft classifiers in various classification problems. © 2011 American Statistical Association.
- Storlie, C. B., Bondell, H. D., Reich, B. J., & Zhang, H. H. (2011). Surface estimation, variable selection, and the nonparametric oracle property. Statistica Sinica, 21(2), 679-705.More infoAbstract: Variable selection for multivariate nonparametric regression is an important, yet challenging, problem due, in part, to the infinite dimensionality of the function space. An ideal selection procedure would be automatic, stable, easy to use, and have desirable asymptotic properties. In particular, we define a selection procedure to be nonparametric oracle (np-oracle) if it consistently selects the correct subset of predictors and, at the same time, estimates the smooth surface at the optimal nonparametric rate, as the sample size goes to infinity. In this paper, we propose a model selection procedure for nonparametric models, and explore the conditions under which the new method enjoys the aforementioned properties. Developed in the framework of smoothing spline ANOVA, our estimator is obtained via solving a regularization problem with a novel adaptive penalty on the sum of functional component norms. Theoretical properties of the new estimator are established. Additionally, numerous simulations and examples suggest that the new approach substantially outperforms other existing methods in the finite sample setting.
- Zhang, H. H., Cheng, G., & Liu, Y. (2011). Linear or nonlinear? automatic structure discovery for partially linear models. Journal of the American Statistical Association, 106(495), 1099-1112.More infoAbstract: Partially linear models provide a useful class of tools for modeling complex data by naturally incorporating a combination of linear and nonlinear effects within one framework. One key question in partially linear models is the choice of model structure, that is, how to decide which covariates are linear and which are nonlinear. This is a fundamental, yet largely unsolved problem for partially linear models. In practice, one often assumes that the model structure is given or known and then makes estimation and inference based on that structure. Alternatively, there are two methods in common use for tackling the problem: hypotheses testing and visual screening based on the marginal fits. Both methods are quite useful in practice but have their drawbacks. First, it is difficult to construct a powerful procedure for testing multiple hypotheses of linear against nonlinear fits. Second, the screening procedure based on the scatterplots of individual covariate fits may provide an educated guess on the regression function form, but the procedure is ad hoc and lacks theoretical justifications. In this article, we propose a new approach to structure selection for partially linear models, called the LAND (Linear And Nonlinear Discoverer). The procedure is developed in an elegant mathematical framework and possesses desired theoretical and computational properties. Under certain regularity conditions, we show that the LAND estimator is able to identify the underlying true model structure correctly and at the same time estimate the multivariate regression function consistently. The convergence rate of the new estimator is established as well. We further propose an iterative algorithm to implement the procedure and illustrate its performance by simulated and real examples. Supplementary materials for this article are available online. © 2011 American Statistical Association.
- Qiao, X., Zhang, H. H., Liu, Y., Todd, M. J., & Marron, J. S. (2010). Weighted distance weighted discrimination and its asymptotic properties. Journal of the American Statistical Association, 105(489), 401-414.More infoAbstract: While Distance Weighted Discrimination (DWD) is an appealing approach to classification in high dimensions, it was designed for balanced datasets. In the case of unequal costs, biased sampling, or unbalanced data, there are major improvements available, using appropriately weighted versions of DWD (wDWD). A major contribution of this paper is the development of optimal weighting schemes for various nonstandard classification problems. In addition, we discuss several alternative criteria and propose an adaptive weighting scheme (awDWD) and demonstrate its advantages over nonadaptive weighting schemes under some situations. The second major contribution is a theoretical study of weighted DWD. Both high-dimensional low sample-size asymptotics and Fisher consistency of DWD are studied. The performance of weighted DWD is evaluated using simulated examples and two real data examples. The theoretical results are also confirmed by simulations. © 2010 American Statistical Association.
- Shows, J. H., Wenbin, L. u., & Zhang, H. H. (2010). Sparse estimation and inference for censored median regression. Journal of Statistical Planning and Inference, 140(7), 1903-1917.More infoAbstract: Censored median regression has proved useful for analyzing survival data in complicated situations, say, when the variance is heteroscedastic or the data contain outliers. In this paper, we study the sparse estimation for censored median regression models, which is an important problem for high dimensional survival data analysis. In particular, a new procedure is proposed to minimize an inverse-censoring-probability weighted least absolute deviation loss subject to the adaptive LASSO penalty and result in a sparse and robust median estimator. We show that, with a proper choice of the tuning parameter, the procedure can identify the underlying sparse model consistently and has desired large-sample properties including root-n consistency and the asymptotic normality. The procedure also enjoys great advantages in computation, since its entire solution path can be obtained efficiently. Furthermore, we propose a resampling method to estimate the variance of the estimator. The performance of the procedure is illustrated by extensive simulations and two real data applications including one microarray gene expression survival data. © 2010 Elsevier B.V. All rights reserved.
- Wenbin, L. u., & Zhang, H. H. (2010). On estimation of partially linear transformation models. Journal of the American Statistical Association, 105(490), 683-691.More infoAbstract: We study a general class of partially linear transformation models, which extend linear transformation models by incorporating nonlinear covariate effects in survival data analysis. A new martingale-based estimating equation approach, consisting of both global and kernelweighted local estimation equations, is developed for estimating the parametric and nonparametric covariate effects in a unified manner. We show that with a proper choice of the kernel bandwidth parameter, one can obtain the consistent and asymptotically normal parameter estimates for the linear effects. Asymptotic properties of the estimated nonlinear effects are established as well.We further suggest a simple resampling method to estimate the asymptotic variance of the linear estimates and show its effectiveness. To facilitate the implementation of the new procedure, an iterative algorithm is developed. Numerical examples are given to illustrate the finite-sample performance of the procedure. Supplementary materials are available online. © 2010 American Statistical Association.
- Xiao, N. i., Zhang, D., & Zhang, H. H. (2010). Variable selection for semiparametric mixed models in longitudinal studies. Biometrics, 66(1), 79-88.More infoPMID: 19397585;PMCID: PMC2875374;Abstract: We propose a double-penalized likelihood approach for simultaneous model selection and estimation in semiparametric mixed models for longitudinal data. Two types of penalties are jointly imposed on the ordinary log-likelihood: the roughness penalty on the nonparametric baseline function and a nonconcave shrinkage penalty on linear coefficients to achieve model sparsity. Compared to existing estimation equation based approaches, our procedure provides valid inference for data with missing at random, and will be more efficient if the specified model is correct. Another advantage of the new procedure is its easy computation for both regression components and variance parameters. We show that the double-penalized problem can be conveniently reformulated into a linear mixed model framework, so that existing software can be directly used to implement our method. For the purpose of model inference, we derive both frequentist and Bayesian variance estimation for estimated parametric and nonparametric components. Simulation is used to evaluate and compare the performance of our method to the existing ones. We then apply the new method to a real data set from a lactation study. © 2009, The International Biometric Society.
- Yichao, W. u., Zhang, H. H., & Liu, Y. (2010). Robust model-free multiclass probability estimation. Journal of the American Statistical Association, 105(489), 424-436.More infoAbstract: Classical statistical approaches for multiclass probability estimation are typically based on regression techniques such as multiple logistic regression, or density estimation approaches such as linear discriminant analysis (LDA) and quadratic discriminant analysis (QDA). These methods often make certain assumptions on the form of probability functions or on the underlying distributions of subclasses. In this article, we develop a model-free procedure to estimate multiclass probabilities based on large-margin classifiers. In particular, the new estimation scheme is employed by solving a series of weighted large-margin classifiers and then systematically extracting the probability information from these multiple classification rules. A main advantage of the proposed probability estimation technique is that it does not impose any strong parametric assumption on the underlying distribution and can be applied for a wide range of large-margin classification methods. A general computational algorithm is developed for class probability estimation. Furthermore, we establish asymptotic consistency of the probability estimates. Both simulated and real data examples are presented to illustrate competitive performance of the new approach and compare it with several other existing methods. © 2010 American Statistical Association.
- Zhang, H. (2010). Maximum Penalized Likelihood Estimation: Volume II: Regression by EGGERMONT, P. P. and LARICCA, V. N.. Biometrics, 66(2), 662-.More infoPMID: 20579046;
- Zhang, H. H., Wenbin, L. u., & Wang, H. (2010). On sparse estimation for semiparametric linear transformation models. Journal of Multivariate Analysis, 101(7), 1594-1606.More infoAbstract: Semiparametric linear transformation models have received much attention due to their high flexibility in modeling survival data. A useful estimating equation procedure was recently proposed by Chen et al. (2002) [21] for linear transformation models to jointly estimate parametric and nonparametric terms. They showed that this procedure can yield a consistent and robust estimator. However, the problem of variable selection for linear transformation models has been less studied, partially because a convenient loss function is not readily available under this context. In this paper, we propose a simple yet powerful approach to achieve both sparse and consistent estimation for linear transformation models. The main idea is to derive a profiled score from the estimating equation of Chen et al. [21], construct a loss function based on the profile scored and its variance, and then minimize the loss subject to some shrinkage penalty. Under regularity conditions, we have shown that the resulting estimator is consistent for both model estimation and variable selection. Furthermore, the estimated parametric terms are asymptotically normal and can achieve a higher efficiency than that yielded from the estimation equations. For computation, we suggest a one-step approximation algorithm which can take advantage of the LARS and build the entire solution path efficiently. Performance of the new procedure is illustrated through numerous simulations and real examples including one microarray data. © 2010 Elsevier Inc.
- Liu, H., Tang, Y., & Zhang, H. H. (2009). A new chi-square approximation to the distribution of non-negative definite quadratic forms in non-central normal variables. Computational Statistics and Data Analysis, 53(4), 853-856.More infoAbstract: This note proposes a new chi-square approximation to the distribution of non-negative definite quadratic forms in non-central normal variables. The unknown parameters are determined by the first four cumulants of the quadratic forms. The proposed method is compared with Pearson's three-moment central χ2 approximation approach, by means of numerical examples. Our method yields a better approximation to the distribution of the non-central quadratic forms than Pearson's method, particularly in the upper tail of the quadratic form, the tail most often needed in practical work. © 2008 Elsevier B.V. All rights reserved.
- Xiao, N. i., Zhang, H. H., & Zhang, D. (2009). Automatic model selection for partially linear models. Journal of Multivariate Analysis, 100(9), 2100-2111.More infoAbstract: We propose and study a unified procedure for variable selection in partially linear models. A new type of double-penalized least squares is formulated, using the smoothing spline to estimate the nonparametric part and applying a shrinkage penalty on parametric components to achieve model parsimony. Theoretically we show that, with proper choices of the smoothing and regularization parameters, the proposed procedure can be as efficient as the oracle estimator [J. Fan, R. Li, Variable selection via nonconcave penalized likelihood and its oracle properties, Journal of American Statistical Association 96 (2001) 1348-1360]. We also study the asymptotic properties of the estimator when the number of parametric effects diverges with the sample size. Frequentist and Bayesian estimates of the covariance and confidence intervals are derived for the estimators. One great advantage of this procedure is its linear mixed model (LMM) representation, which greatly facilitates its implementation by using standard statistical software. Furthermore, the LMM framework enables one to treat the smoothing parameter as a variance component and hence conveniently estimate it together with other regression coefficients. Extensive numerical studies are conducted to demonstrate the effective performance of the proposed procedure. © 2009 Elsevier Inc. All rights reserved.
- Zou, H., & Zhang, H. H. (2009). On the adaptive elastic-net with a diverging number of parameters. Annals of Statistics, 37(4), 1733-1751.More infoAbstract: We consider the problem of model selection and estimation in situations where the number of parameters diverges with the sample size. When the dimension is high, an ideal method should have the oracle property [J. Amer. Statist. Assoc. 96 (2001) 1348-1360] and [Ann. Statist. 32 (2004) 928-961] which ensures the optimal large sample performance. Furthermore, the highdimensionality often induces the collinearity problem, which should be properly handled by the ideal method. Many existing variable selection methods fail to achieve both goals simultaneously. In this paper, we propose the adaptive elastic-net that combines the strengths of the quadratic regularization and the adaptively weighted lasso shrinkage. Under weak regularity conditions, we establish the oracle property of the adaptive elastic-net. We show by simulations that the adaptive elastic-net deals with the collinearity problem better than the other oracle-like methods, thus enjoying much improved finite sample performance. © Institute of Mathematical Statistics, 2009.
- Liu, Y., Zhang, H. H., Park, C., & Ahn, J. (2007). Support vector machines with adaptive Lq penalty. Computational Statistics and Data Analysis, 51(12), 6380-6394.More infoAbstract: The standard support vector machine (SVM) minimizes the hinge loss function subject to the L2 penalty or the roughness penalty. Recently, the L1 SVM was suggested for variable selection by producing sparse solutions [Bradley, P., Mangasarian, O., 1998. Feature selection via concave minimization and support vector machines. In: Shavlik, J. (Ed.), ICML'98. Morgan Kaufmann, Los Altos, CA; Zhu, J., Hastie, T., Rosset, S., Tibshirani, R., 2003. 1-norm support vector machines. Neural Inform. Process. Systems 16]. These learning methods are non-adaptive since their penalty forms are pre-determined before looking at data, and they often perform well only in a certain type of situation. For instance, the L2 SVM generally works well except when there are too many noise inputs, while the L1 SVM is more preferred in the presence of many noise variables. In this article we propose and explore an adaptive learning procedure called the Lq SVM, where the best q > 0 is automatically chosen by data. Both two- and multi-class classification problems are considered. We show that the new adaptive approach combines the benefit of a class of non-adaptive procedures and gives the best performance of this class across a variety of situations. Moreover, we observe that the proposed Lq penalty is more robust to noise variables than the L1 and L2 penalties. An iterative algorithm is suggested to solve the Lq SVM efficiently. Simulations and real data applications support the effectiveness of the proposed procedure. © 2007 Elsevier B.V. All rights reserved.
- Wenbin, L. u., & Zhang, H. H. (2007). Variable selection for proportional odds model. Statistics in Medicine, 26(20), 3771-3781.More infoPMID: 17266170;Abstract: In this paper we study the problem of variable selection for the proportional odds model, which is a useful alternative to the proportional hazards model and might be appropriate when the proportional hazards assumption is not satisfied. We propose to fit the proportional odds model by maximizing the marginal likelihood subject to a shrinkage-type penalty, which encourages sparse solutions and hence facilitates the process of variable selection. Two types of shrinkage penalties are considered: the LASSO and the adaptive-LASSO (ALASSO) penalty. In the ALASSO penalty, different weights are imposed on different coefficients such that important variables are more protectively retained in the final model while unimportant ones are more likely to be shrunk to zeros. We further provide an efficient computation algorithm to implement the proposed methods, and demonstrate their performance through simulation studies and an application to real data. Numerical results indicate that both methods can produce accurate and interpretable models, and the ALASSO tends to work better than the usual LASSO. Copyright © 2007 John Wiley & Sons, Ltd.
- Zhang, H. H., & Wenbin, L. u. (2007). Adaptive Lasso for Cox's proportional hazards model. Biometrika, 94(3), 691-703.More infoAbstract: We investigate the variable selection problem for Coxs proportional hazards model, and propose a unified model selection and estimation procedure with desired theoretical properties and computational convenience. The new method is based on a penalized log partial likelihood with the adaptively weighted L 1 penalty on regression coefficients, providing what we call the adaptive Lasso estimator. The method incorporates different penalties for different coefficients: unimportant variables receive larger penalties than important ones, so that important variables tend to be retained in the selection process, whereas unimportant variables are more likely to be dropped. Theoretical properties, such as consistency and rate of convergence of the estimator, are studied. We also show that, with proper choice of regularization parameters, the proposed estimator has the oracle properties. The convex optimization nature of the method leads to an efficient algorithm. Both simulated and real examples show that the method performs competitively. © 2007 Biometrika Trust.
- Leng, C., & Zhang, H. H. (2006). Model selection in nonparametric hazard regression. Journal of Nonparametric Statistics, 18(7-8), 417-429.More infoAbstract: We propose a novel model selection method for a nonparametric extension of the Cox proportional hazard model, in the framework of smoothing splines ANOVA models. The method automates the model building and model selection processes simultaneously by penalizing the reproducing kernel Hilbert space norms. On the basis of a reformulation of the penalized partial likelihood, we propose an efficient algorithm to compute the estimate. The solution demonstrates great flexibility and easy interpretability in modeling relative risk functions for censored data. Adaptive choice of the smoothing parameter is discussed. Both simulations and a real example suggest that our proposal is a useful tool for multivariate function estimation and model selection in survival analysis.
- Lin, Y., & Zhang, H. H. (2006). Component selection and smoothing in multivariate nonparametric regression. Annals of Statistics, 34(5), 2272-2297.More infoAbstract: We propose a new method for model selection and model fitting in multivariate nonparametric regression models, in the framework of smoothing spline ANOVA. The "COSSO" is a method of regularization with the penalty functional being the sum of component norms, instead of the squared norm employed in the traditional smoothing spline method. The COSSO provides a unified framework for several recent proposals for model selection in linear models and smoothing spline ANOVA models. Theoretical properties, such as the existence and the rate of convergence of the COSSO estimator, are studied. In the special case of a tensor product design with periodic functions, a detailed analysis reveals that the COSSO does model selection by applying a novel soft thresholding type operation to the function components. We give an equivalent formulation of the COSSO estimator which leads naturally to an iterative algorithm. We compare the COSSO with MARS, a popular method that builds functional ANOVA models, in simulations and real examples. The COSSO method can be extended to classification problems and we compare its performance with those of a number of machine learning algorithms on real datasets. The COSSO gives very competitive performance in these studies. © Institute of Mathematical Statistics, 2006.
- Tang, Y., & Zhang, H. H. (2006). Multiclass proximal support vector machines. Journal of Computational and Graphical Statistics, 15(2), 339-355.More infoAbstract: This article proposes the multiclass proximal support vector machine (MPSVM) classifier, which extends the binary PSVM to the multiclass case. Unlike the one-versus-rest approach that constructs the decision rule based on multiple binary classification tasks, the proposed method considers all classes simultaneously and has better theoretical properties and empirical performance. We formulate the MPSVM as a regularization problem in the reproducing kernel Hilbert space and show that it implements the Bayes rule for classification. In addition, the MPSVM can handle equal and unequal misclassification costs in a unified framework. We suggest an efficient algorithm to implement the MPSVM by solving a system of linear equations. This algorithm requires much less computational effort than solving the standard SVM, which often requires quadratic programming and can be slow for large problems. We also provide an alternative and more robust algorithm for ill-posed problems. The effectiveness of the MPSVM is demonstrated by both simulation studies and applications to cancer classifications using microarray data. ©2006 American Statistical Association, Institute of Mathematical Statistics, and Interface Foundation of North America.
- Zhang, H. H. (2006). Variable selection for support vector machines via smoothing spline anova. Statistica Sinica, 16(2), 659-674.More infoAbstract: It is well-known that the support vector machine paradigm is equivalent to solving a regularization problem in a reproducing kernel Hubert space. The squared norm penalty in the standard support vector machine controls the smoothness of the classification function. We propose, under the framework of smoothing spline ANOVA models, a new type of regularization to conduct simultaneous classification and variable selection in the SVM. The penalty functional used is the sum of functional component norms, which automatically applies soft-thresholding operations to functional components, hence yields sparse solutions. We suggest an efficient algorithm to solve the proposed optimization problem by iteratively solving quadratic and linear programming problems. Numerical studies, on both simulated data and real datasets, show that the modified support vector machine gives very competitive performances compared to other popular classification algorithms, in terms of both classification accuracy and variable selection.
- Zhang, H. H., & Lin, Y. (2006). Component selection and smoothing for nonparametric regression in exponential families. Statistica Sinica, 16(3), 1021-1041.More infoAbstract: We propose a new penalized likelihood method for model selection and nonparametric regression in exponential families. In the framework of smoothing spline ANOVA, our method employs a regularization with the penalty functional being the sum of the reproducing kernel Hilbert space norms of functional components in the ANOVA decomposition. It generalizes the LASSO in the linear regression to the nonparametric context, and conducts component selection and smoothing simultaneously. Continuous and categorical variables are treated in a unified fashion. We discuss the connection of the method to the traditional smoothing spline penalized likelihood estimation. We show that an equivalent formulation of the method leads naturally to an iterative algorithm. Simulations and examples are used to demonstrate the performances of the method.
- Zhang, H. H., Ahn, J., Lin, X., & Park, C. (2006). Gene selection using support vector machines with non-convex penalty. Bioinformatics, 22(1), 88-95.More infoPMID: 16249260;Abstract: Motivation: With the development of DNA microarray technology, scientists can now measure the expression levels of thousands of genes simultaneously in one single experiment. One current difficulty in interpreting microarray data comes from their innate nature of 'high-dimensional low sample size'. Therefore, robust and accurate gene selection methods are required to identify differentially expressed group of genes across different samples, e.g. between cancerous and normal cells. Successful gene selection will help to classify different cancer types, lead to a better understanding of genetic signatures in cancers and improve treatment strategies. Although gene selection and cancer classification are two closely related problems, most existing approaches handle them separately by selecting genes prior to classification. We provide a unified procedure for simultaneous gene selection and cancer classification, achieving high accuracy in both aspects. Results: In this paper we develop a novel type of regularization in support vector machines (SVMs) to identify important genes for cancer classification. A special nonconvex penalty, called the smoothly clipped absolute deviation penalty, is imposed on the hinge loss function in the SVM. By systematically thresholding small estimates to zeros, the new procedure eliminates redundant genes automatically and yields a compact and accurate classifier. A successive quadratic algorithm is proposed to convert the non-differentiable and non-convex optimization problem into easily solved linear equation systems. The method is applied to two real datasets and has produced very promising results. © The Author 2005. Published by Oxford University Press. All rights reserved.
- Ferris, M. C., Voelker, M. M., & Zhang, H. H. (2004). Model building with likelihood basis pursuit. Optimization Methods and Software, 19(5 SPEC. ISS.), 577-594.More infoAbstract: We consider a non-parametric penalized likelihood approach for model building called likelihood basis pursuit (LBP) that determines the probabilities of binary outcomes given explanatory vectors while automatically selecting important features. The LBP model involves parameters that balance the competing goals of maximizing the log-likelihood and minimizing the penalized basis pursuit terms. These parameters are selected to minimize a proxy of misclassification error, namely, the randomized, generalized approximate cross validation (ranGACV) function. The ranGACV function is not easily represented in compact form; its functional values can only be obtained by solving two instances of the LBP model, which may be computationally expensive. A grid search is typically used to find appropriate parameters, requiring the solutions to hundreds or thousands of instances of the LBP model. Since only parameters (data) are changed between solves, the resulting problem is a nonlinear slice model in the parameter space. We show how slice-modeling techniques significantly improve the efficiency of individual solves and thus speed-up the grid search. In addition, we consider using derivative-free optimization algorithms for parameter selection, replacing the grid search. We show how, by seeding the derivative-free algorithms with a coarse grid search, these algorithms can find better solutions with fewer function evaluations. Our interest in this area comes directly from the seminal work that Olvi and his collaborators have carried out designing and applying optimization techniques to problems in machine learning and data mining.
- Zhang, H. H., Wahba, G., Lin, Y., Voelker, M., Ferris, M., Klein, R., & Klein, B. (2004). Variable selection and model building via likelihood basis pursuit. Journal of the American Statistical Association, 99(467), 659-672.More infoAbstract: This article presents a nonparametric penalized likelihood approach for variable selection and model building, called likelihood basis pursuit (LBP). In the setting of a tensor product reproducing kernel Hilbert space, we decompose the log-likelihood into the sum of different functional components such as main effects and interactions, with each component represented by appropriate basis functions. Basis functions are chosen to be compatible with variable selection and model building in the context of a smoothing spline ANOVA model. Basis pursuit is applied to obtain the optimal decomposition in terms of having the smallest l 1 norm on the coefficients. We use the functional L 1 norm to measure the importance of each component and determine the "threshold" value by a sequential Monte Carlo bootstrap test algorithm. As a generalized LASSO-type method, LBP produces shrinkage estimates for the coefficients, which greatly facilitates the variable selection process and provides highly interpretable multivariate functional estimates at the same time. To choose the regularization parameters appearing in the LBP models, generalized approximate cross-validation (GACV) is derived as a tuning criterion. To make GACV widely applicable to large datasets, its randomized version is proposed as well. A technique "slice modeling" is used to solve the optimization problem and makes the computation more efficient. LBP has great potential for a wide range of research and application areas such as medical studies, and in this article we apply it to two large ongoing epidemiologic studies, the Wisconsin Epidemiologic Study of Diabetic Retinopathy (WESDR) and the Beaver Dam Eye Study (BDES).
- Lin, Y., Wahba, G., Zhang, H., & Lee, Y. (2002). Statistical properties and adaptive tuning of support vector machines. Machine Learning, 48(1-3), 115-136.More infoAbstract: In this paper we consider the statistical aspects of support vector machines (SVMs) in the classification context, and describe an approach to adaptively tuning the smoothing parameter(s) in the SVMs. The relation between the Bayes rule of classification and the SVMs is discussed, shedding light on why the SVMs work well. This relation also reveals that the misclassification rate of the SVMs is closely related to the generalized comparative Kullback-Leibler distance (GCKL) proposed in Wahba (1999, Scholkopf, Burges, & Smola (Eds.), Advances in Kernel Methods-Support Vector Learning, Cambridge, MA: MIT Press). The adaptive tuning is based on the generalized approximate cross validation (GACV), which is an easily computable proxy of the GCKL. The results are generalized to the unbalanced case where the fraction of members of the classes in the training set is different than that in the general population, and the costs of misclassification for the two kinds of errors are different. The main results in this paper have been obtained in several places elsewhere. Here we take the opportunity to organize them in one place and note how they fit together and reinforce one another. Mostly the work of the authors is reviewed.
Proceedings Publications
- Li, Q., Zaim, S., Aberasturi, D., Berghout, J., Li, H., Vitali, F., Kenost, C., Zhang, H., & Lussier, Y. A. (2019, Nov). Interpretation of ‘Omics dynamics in a single subject using local estimates of dispersion between two transcriptomes. In AMIA Annual Symposium.
- Berger, M., Nagesh, A., Josh, L., Surdeanu, M., & Zhang, H. (2018, Oct 31-Nov4). Visual supervision in bootstrapped information extraction. In Conference on Empirical Methods in Natural Language Processing (EMNLP).
Presentations
- Li, Q., Lussier, Y. A., Schissler, A. G., Zhang, H. H., Gardeux, V., Li, H., Achour, I., Berghout, J., Kenost, C., Kenost, C., Achour, I., Berghout, J., Gardeux, V., Li, H., Schissler, A. G., Zhang, H. H., Li, Q., & Lussier, Y. A. (2016, Oct.). N-of-1-pathways MixEnrich: advancing precision medicine via single-subject analysis in discovering dynamic changes of transcriptomes. Translational Bioinformatics Conference 2016. Jeju Island, Korea.
- Zhang, H. (2024, July).
binomialRF: Scalable Feature Selection and Interaction Screening via Random Forests.
. , 2024 Joint Conference of Statistics and Data Science (JCSDS).. Kunming, China.: ICSA, IMS. - Zhang, H. (2024, July). Scalable Algorithms for Multiclass Probability Estimation in High Dimensional Data.. 2024 IMS- China.. Yinchuan, China.: Institute of Mathematical Statistics (IMS).
- Zhang, H. (2024, June). binomialRF: Scalable Feature Selection and Interaction Screening via Random Forest. WNAR 2024. Fort Colins, Colorado.
- Zhang, H. (2024, June/Summer). binomialRF: Scalable Feature Selection and Interaction Screening via Random Forest. 2024 ICSA- China. Wuhan, China.: International Chinese Statistician Association (ICSA).
- Zhang, H. (2024, November/Winter). Scalable Algorithms for Multiclass Probability Estimation in High Dimensional Data.. Department of Applied Math & Statistics, Stony Brook University.. zoom: Stony Brook University.
- Zhang, H. (2023, Feb). Oracle P-value and Variable Screening Health Data.. 2023 National Big Data Health SCience (NBDHS).. University of South Carolina..
- Zhang, H. (2022). Scalable Model-free Estimation for Multiclass Probabilities.. 'Department of Statistics, Kansas State University..
- Zhang, H. (2022). Scalable Model-free Estimation for Multiclass Probabilities.. Department of Statistics, University of Iowa..
- Zhang, H. (2022, August). Spatial Heterogeneity Automatic Detection and Estimation (SHADE).. Joint Statistical Meetings (JSM). Washington, DC..
- Zhang, H. (2022, December). Flexible and Interpretable Learning for High-Dimensional Health Data.. International Indian Statistical Association Annual Conference (Keynote Speech). Bengaluru, India.: International Indian Statistical Association.
- Zhang, H. (2022, Feb). Flexible and Interpretable Learning for High-dimensional Health Data.. National Big Data Health SCience (NBDHS), Keynote Talk.. University of South Carolina..
- Zhang, H. (2022, June). Grace Wahba's Contribution to Statistical Machine Learning and Optimization.. 'IMS2022 Annual Meeting.. London, UK. (online).
- Zhang, H. (2022, June). Oracle P-value and Variable Screening.. 5th International Conference on Econometrics and Statistics (EcoSta 2022).. Kyoto, Japan..
- Zhang, H. (2021). Oracle P-value and Variable Screening. UC-Irvine Statistical SeminarUC Irvine.
- Zhang, H. (2021, April). Scalable Model-free Estimation for Multiclass Probabilities. Department Colloquium. University of Georgia.
- Zhang, H. (2021, Dec). Scalable Model-free Estimation for Multiclass Probabilities.. Department of Statistics, San Diego State University..
- Zhang, H. (2021, Feb). Transdisciplinary Collaborations for Building Theoretical Foundations of Data Science. ASA Webinar. online: American Statistical Association.
- Zhang, H. (2021, Nov). Breaking Curse of Dimensionality in Nonparametrics. Duke Statistical Science Seminar. online: Duke University.
- Zhang, H. (2020, August). Build A Data Science Team. Joint Statistical Meetings (JSM) 2020. virtual.
- Zhang, H. (2020, December). Breaking Curse of Dimensionality in Nonparametrics. Department of Economics, Eller College of Management, University of Arizona,. virtual: Eller College of Management, University of Arizona.
- Zhang, H. (2020, Jan). Oracle P-value and Variable Screening. CIMAT/UA-TRIPODS Workshop on Data Science. CIMAT, Guanajuato, Mexico.
- Zhang, H. (2020, June). Oracle P-value and Variable Screening. Workshop on Big Data and Statistical Sciences. Shanxi University of Finance and Economics, Taiyuan, China (virtual).
- Zhang, H. (2020, October). Build A Data Science Team. 2020 Academic Data Science Alliance (ADSA) Leadership Summit & Annual Meetings.
- Zhang, H. (2020, October). Sparse and Smooth Function Estimation in Reproducing Kernel Hilbert Spaces. Theoretical and Applied Data Science Seminar at TRIPODS, Iowa State University. virtual: Iowa State University.
- Zhang, H. (2019, April). Scalable Methods and Algorithms for Interaction Selection. Machine Learning Day, Arizona State University. ASU West Campus.
- Zhang, H. (2019, April). UA-TRIPODS Program. College of Science Dean’s Board of Advisors Meeting. University of Arizona.
- Zhang, H. (2019, August). Discussions: Highlights of the STAT Journal. ISI World Congress of Statistics. Kuala Lumpur, Malaysia: International Statistical Institute.
- Zhang, H. (2019, July). Breaking Curse of Dimensionality in Nonparametrics. Joint Statistical Meetings (JSM). Denver, CO: American Statistical Association, Institute of Mathematical Statistics.
- Zhang, H. (2019, July). Scalable Model-free Estimation for Multiclass Probabilities. ICSA China Conference. Nankai University, China: International Chinese Statistician Association.
- Zhang, H. (2019, July). Scalable Model-free Estimation for Multiclass Probabilities. Statistics Workshop. Department of Statistics, Jilin University, China.
- Zhang, H. (2019, June). Scalable Model-free Estimation for Multiclass Probabilities. Symposium on Data Science and Statistics. Seattle, WA: American Statistical Association.
- Zhang, H. (2019, May). Scalable Model-free Estimation for Multiclass Probabilities. Rao Prize Conference. Penn State University: Penn State University.
- Zhang, H. (2018, July 28-August 2). Scalable model-free estimation for multiclass probabilities. The 2018 Joint Statistical Meetings. Vancouver: American Statistical Association.
- Zhang, H. (2018, June 14-17). Partially function linear regression in high dimensions. The ICSA 2018 Applied Statistics Symposium,.
- Zhang, H. (2018, June). Scalable model-free estimation for multiclass probabilities.. Conference on Statistical Learning and Data Science/Nonparametric Statistics. Columbia University.
- Zhang, H. (2018, May). Interaction Selection: Its Past, Present, and Future. Conference on Predictive Inference and Its Applications. Iowa State University.
- Zhang, H. (2018, Nov). Scalable Methods and Algorithms for Interaction Selection. Department of Statistics Seminar. Georgia Institute of Technology.
- Zhang, H. (2018, Nov). Scalable Methods and Algorithms for Interaction Selection. Department of Statistics Seminar. University of California at Santa Barbara.
- Zhang, H. (2018, Oct 17-18). Scalable model-free estimation for multiclass probabilities'. Department of Biostatistics Seminar. Columbia University.
- Zhang, H. (2018, Oct 19-20). Scalable Methods and Algorithms for Interaction Selection. Department of Statistics, Statistics Seminar. Purdue University.
- Zhang, H. (2018, Sep 8-10). Oracle P-value and variable screening. Workshop on Higher-order Asymptotics and Post-Selection Inference. Washington University.
- Zhang, H. (2017, Dec). Interaction selection: Its past , present, and future. RIT Data Science Research Group Seminar.
- Zhang, H. (2017, Dec). Scalable methods and algorithms for interaction selection. The 2017 International Conference on Data Science. Shanghai, China: School of Data Science, Fudan University.
- Zhang, H. (2017, Dec). Scalable methods and algorithms for interaction selection. Workshop for big data and statistical sciences. Taiyuan, Shanxi, China: Shanxi University of Finance and Ecnomics.
- Zhang, H. (2017, July). Scalable methods and algorithms for interaction selection. Workshop SINW01L: Scalable statistics inference. Cambridge, UK.: Issac Newton Institute for Mathematical Sciences, Cambridge University..
- Zhang, H. (2017, June). Hierarchy-preserving regularization solution paths for identifying interactions in high dimensional data. The Third International Workshop on Statistical Genetics and Genomics. Taiyuan, Shanxi, China: Shanxi Medical University.
- Zhang, H. (2017, June). Structured functional additive regression in RKHS. The 1st International Conference on Econometrics and Statistics. Hong Kong: Hongkong University of Science and Technology.
- Zhang, H. (2017, Nov). Hierarchy-preserving regularization solution paths for identifying interactions in high dimensional data. Statitsics Colloquium.
- Zhang, H. (2016, Feb.). Conquering Cancer.. Seminar Course for Graduate Students, Applied Math GIDP.. Tucson, UA.: Applied Math GIDP.
- Zhang, H. (2016, July). Structured functional additive regression in reproducing kernel Hilbert spaces.. The 4th IBS-China International Biostatistic Conference. Shanghai, China..
- Zhang, H. (2016, June). Modern statistics methods for genomics and optimal treatment.. The Second International Workshop on Statistical Genetics and Genomics, Shanxi Medical University.. Taiyuan, China..
- Zhang, H. (2016, June). Probability-enhanced sufficient dimension reduction for binary classification. Third Conference of the International Society for Nonparametric Statistics. Avignon, France.
- Zhang, H. (2016, June). Structured functional additive regression in reproducing kernel Hilbert spaces.. Workshop on Probability and Statistics, Beijing University,. Beijing, China..
- Zhang, H. (2016, May). Probability-enhanced sufficient dimension reduction for binary classification. International Statistics Forum, Renmin University of China. Beijing, China.
- Zhang, H. (2016, September). Identify Interactions for Ultra-high Dimensional Data.. SAMSI DPDA Workshop: Reinforcing the Importance of Statistics and Applied Mathematics in Distributed Computing.. Raleigh, NC.: SAMSI..
- Zhang, H. (2015, April). Interaction Selection for High Dimensional Data. Colloquium, Department of Statistics, Iowa State University. Ames, Iowa: Iowa State University.
- Zhang, H. (2015, December). Conquering Cancer. The Second Mathematical Science Cafes Series. Borderlands Brewing Company: College of Science UA Cafes Series, University of Arizona..
- Zhang, H. (2015, Jan.). Variable Selection for Optimal Treatment Decision. Colloquium, Department of Epidemiology and Biostatistics.
- Zhang, H. (2015, June). Identify Interactions for Ultra-high Dimensional Data. Second International Workshop on Statistical Genetics and Genomics. Taiyuan, China: Shanxi Medical University.
- Zhang, H. (2015, May). Identify Interactions for Ultra-high Dimensional Data. Colloquium, School of Mathematical and Statistical Sciences. Phoenix, AZ: Arizona State University.
- Zhang, H. (2014, April). Identify Interactions for Ultra-high Dimensional Data.. Department of Statistics Colloquium. Columbus, OH: Ohio State University.
- Zhang, H. (2014, February). Identify Interactions for Ultra-high Dimensional Data.. SAMSI Low-dimensional Structure in High-dimensional Systems (LDHD) Workshop.. RTP, NC: Statistical and Applied Mathematical Sciences Institute (SAMSI).
- Zhang, H. (2014, June). Structured functional additive regression in reproducing kernel Hilbert spaces.. ASA Section Meeting on Statistical Learning and Data Mining. Durham, NC: ASA, Statistical Learning and Data Mining Section.
- Zhang, H. (2014, June). Structured functional additive regression in reproducing kernel Hilbert spaces.. ICSA/KISS Joint Applied Statistics Symposium. Portland, OR: International Chinese Statistical Association (ICSA) and the Korean International Statistical Society (KISS).
- Zhang, H. (2014, March). Structured functional additive regression in reproducing kernel Hilbert spaces. 2014 ENAR International Biometric Society Spring Meeting.. Baltimore, MD.: Eastern North American Region. International Biometric Society..
- Zhang, H. (2014, May). Rising Stars: Women Making Waves, Panel Discussion on NSF Career Award.. Woman in Statistics Conference.
- Zhang, H. (2014, November). Structured functional additive regression in reproducing kernel Hilbert spaces.. Big Data Statistics Workshop. Shanghai, China.: Shanghai University of Finance and Economics, Shanghai Center for Mathematical Sciences..
- Zhang, H. (2014, October). Identify Interactions for Ultra-high Dimensional Data.. Department of Statistics Colloquium. Tallahassee, FL: Florida State University.
Creative Productions
- Feng, Y., Hao, N., & Zhang, H. (2015. R Package "RAMP".