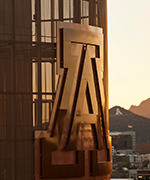
Francesca Vitali
- Assistant Professor, Neurology
- Member of the Graduate Faculty
- (520) 626-4551
- AHSC, Rm. 6205
- TUCSON, AZ 85724-5023
- francescavitali@arizona.edu
Biography
Francesca Vitali, PhD is a Research Assistant Professor and the Director of Bioinformatics in the Center for Innovation in Brain Sciences (CIBS).
After completing her Bachelor's Degree and Master's Degree in Biomedical Engineering at the University of Pavia, Dr. Vitali received her PhD in Bioinformatics and Biomedical Engineering from the University of Pavia, Italy. She was a postdoctoral researcher at the Bioinformatics, Mathematical Modelling and Synthetic Biology (BMS) Lab at the University of Pavia under the mentorship of prof. Riccardo Bellazzi. Most recently, she was a Research Assistant Professor for three years in the Lussier Research Group at the University of Arizona.
In her current role at CIBS, she focuses on the development of computational methods to support precision medicine, drug repurposing and poly-pharmacology. Her background includes data mining, statistics, graph theory, machine learning, artificial intelligence and data integration techniques. She has a long record of working in multidisciplinary collaborative teams, including collaborations with international laboratories and pharmaceutical companies (Sanofi and AstraZeneca). She is the organizer and instructor of a monthly workshop on the use of Orange, an open-source tool for data visualization, machine learning and data mining.
Degrees
- Ph.D. Bioinformatics and Biomedical Engineering
- University of Pavia, Pavia, Italy
- Network-based approaches for multi-target therapies: application to Triple Negative Breast Cancer
- M.A. Biomedical Engineering
- University of Pavia, Pavia, Italy
- A bionformatic method to support polypharmacological approaches
- B.A. Biomedical Engineering
- University of Pavia, Pavia, Italy
- Data import, data conversion and implementation ofan on-line system for the Stroke Unit Network Lombardia
Work Experience
- University of Arizona, Tucson, Arizona (2016 - Ongoing)
- University of Pavia (2015 - 2016)
Awards
- Pharmintech Award 2016
- Pharmintech, Spring 2016
- Young researcher prize
- Gruppo Nazionale di Bioingeneria (GNB), Italy, Spring 2014
Interests
Teaching
Artificial Intelligence, data mining, biostatistics, machine learning, biomedical informatics, programming and big data.
Research
Data mining, statistics, graph theory, machine learning, big data and data integration techniques.
Courses
2022-23 Courses
-
Independent Study
NEUR 599 (Spring 2023)
Scholarly Contributions
Journals/Publications
- Vitali, F., Wiegand, J. P., Parker-Halstead, L., Tucker, A., & Diaz Brinton, R. (2025). Weight trajectories in aging humanized APOE mice with translational validity to human Alzheimer's risk population: A retrospective analysis. PloS one, 20(1), e0314097.More infoTranslational validity of mouse models of Alzheimer's disease (AD) is variable. Because change in weight is a well-documented precursor of AD, we investigated whether diversity of human AD risk weight phenotypes was evident in a longitudinally characterized cohort of 1,196 female and male humanized APOE (hAPOE) mice, monitored up to 28 months of age which is equivalent to 81 human years. Autoregressive Hidden Markov Model (AHMM) incorporating age, sex, and APOE genotype was employed to identify emergent weight trajectories and phenotypes. In the hAPOE-AD mouse cohort, five distinct weight trajectories emerged: three trajectories were associated with a weight loss phenotype (36% of mice, n = 426), one with weight gain (13% of mice, n = 152), and one trajectory of no change in weight (34% of mice, n = 403). The AHMM model findings were validated with post-hoc survival analyses, revealing differences in survival rates across the five identified phenotypes. Further validation was performed using body composition and plasma β-amyloid data from mice within the identified gain, loss and stable weight trajectories. Weight gain trajectory was associated with elevated plasma β-amyloid levels, higher body fat composition, lower survival rates and a greater proportion of APOE4/4 carriers. In contrast, weight loss was associated with greater proportion of hAPOE3/4 carriers, better survival rates and was predominantly male. The association between weight change and AD risk observed in humans was mirrored in the hAPOE-AD mouse model. Weight trajectories of APOE3/3 mice were equally distributed across weight gain, loss and stability. Surprisingly, despite genetic uniformity, comparable housing, diet and handling, distinct weight trajectories and divergence points emerged for subpopulations. These data are consistent with the heterogeneity observed in the human population for change in body weight during aging and highlight the importance of longitudinal phenotypic characterization of mouse aging to advance the translational validity of preclinical AD mouse models.
- Vitali, F., Torrandell-Haro, G., Branigan, G., Arias Aristizabal, J., Reiman, E., Bedrick, E. J., Brinton, R. D., & Weinkauf, C. (2024). Asymptomatic carotid artery stenosis is associated with increased Alzheimer's disease and non-Alzheimer's disease dementia risk. Stroke and vascular neurology.More infoIn the absence of a cerebrovascular accident, whether asymptomatic extracranial carotid atherosclerotic disease (aECAD) affects Alzheimer's disease (AD) and non-AD dementia risk is not clear. Understanding whether aECAD is associated with an increased risk for AD is important as it is present in roughly 10% of the population over 60 and could represent a modifiable risk factor for AD and non-AD dementia.
- Branigan, G. L., Haro, G. T., Vitali, F., Brinton, R. D., & Soto, M. (2023). Implications for Age and Sex Differences on Anti-Hyperglycemic Therapy Exposure and Incidence of Newly Diagnosed Multiple Sclerosis in Propensity Score Matched Type 2 Diabetics (P9-3.007). Neurology, 100(17_supplement_2). doi:10.1212/wnl.0000000000202117
- Branigan, G. L., Torrandell-Haro, G., Chen, S., Shang, Y., Perez-Miller, S., Mao, Z., Padilla-Rodriguez, M., Cortes-Flores, H., Vitali, F., & Brinton, R. D. (2023). Breast cancer therapies reduce risk of Alzheimer's disease and promote estrogenic pathways and action in brain. iScience, 26(11), 108316.More infoWorldwide, an ever-increasing number of women are prescribed estrogen-modulating therapies (EMTs) for the treatment of breast cancer. In parallel, aging of the global population of women will contribute to risk of both breast cancer and Alzheimer's disease. To address the impact of anti-estrogen therapies on risk of Alzheimer's and neural function, we conducted medical informatic and molecular pharmacology analyses to determine the impact of EMTs on risk of Alzheimer's followed by determination of EMT estrogenic mechanisms of action in neurons. Collectively, these data provide both clinical and mechanistic data indicating that select EMTs exert estrogenic agonist action in neural tissue that are associated with reduced risk of Alzheimer's disease while simultaneously acting as effective estrogen receptor antagonists in breast.
- Branigan, G. L., Torrandell-Haro, G., Chen, S., Shang, Y., Perez-Miller, S., Mao, Z., Padilla-Rodriguez, M., Cortes-Flores, H., Vitali, F., & Brinton, R. D. (2023). Breast cancer therapies reduce risk of Alzheimer’s disease and promote estrogenic pathways and action in brain. Iscience, 26(11).
- Mi, Y., Qi, G., Vitali, F., Shang, Y., Raikes, A. C., Wang, T., Jin, Y., Brinton, R. D., Gu, H., & Yin, F. (2023). Loss of fatty acid degradation by astrocytic mitochondria triggers neuroinflammation and neurodegeneration. Nature Metabolism. doi:10.1038/s42255-023-00756-4
- Mi, Y., Qi, G., Vitali, F., Shang, Y., Raikes, A. C., Wang, T., Jin, Y., Brinton, R. D., Gu, H., & Yin, F. (2023). Loss of fatty acid degradation by astrocytic mitochondria triggers neuroinflammation and neurodegeneration. Nature metabolism, 5(3), 445-465.More infoAstrocytes provide key neuronal support, and their phenotypic transformation is implicated in neurodegenerative diseases. Metabolically, astrocytes possess low mitochondrial oxidative phosphorylation (OxPhos) activity, but its pathophysiological role in neurodegeneration remains unclear. Here, we show that the brain critically depends on astrocytic OxPhos to degrade fatty acids (FAs) and maintain lipid homeostasis. Aberrant astrocytic OxPhos induces lipid droplet (LD) accumulation followed by neurodegeneration that recapitulates key features of Alzheimer's disease (AD), including synaptic loss, neuroinflammation, demyelination and cognitive impairment. Mechanistically, when FA load overwhelms astrocytic OxPhos capacity, elevated acetyl-CoA levels induce astrocyte reactivity by enhancing STAT3 acetylation and activation. Intercellularly, lipid-laden reactive astrocytes stimulate neuronal FA oxidation and oxidative stress, activate microglia through IL-3 signalling, and inhibit the biosynthesis of FAs and phospholipids required for myelin replenishment. Along with LD accumulation and impaired FA degradation manifested in an AD mouse model, we reveal a lipid-centric, AD-resembling mechanism by which astrocytic mitochondrial dysfunction progressively induces neuroinflammation and neurodegeneration.
- Arias, J. C., Edwards, M., Vitali, F., Beach, T. G., Serrano, G. E., & Weinkauf, C. C. (2022). Extracranial carotid atherosclerosis is associated with increased neurofibrillary tangle accumulation. Journal of vascular surgery, 75(1), 223-228.More infoWe sought to determine whether extracranial carotid atherosclerotic disease (ECAD) is associated with increased key neurodegenerative pathology such as neurofibrillary tangle (NFT), beta-amyloid plaque, or cerebral amyloid angiopathy (CAA) accumulation, findings associated with Alzheimer's disease (AD) and other dementias.
- Branigan, G. L., Torrandell-Haro, G., Soto, M., Gelmann, E. P., Vitali, F., Rodgers, K. E., & Brinton, R. D. (2022). Androgen-targeting therapeutics mitigate the adverse effect of GnRH agonist on the risk of neurodegenerative disease in men treated for prostate cancer. Cancer medicine, 11(13), 2687-2698.More infoProstate cancer and multiple neurodegenerative diseases (NDD) share an age-associated pattern of onset. Therapy of prostate cancer is known to impact cognitive function. The objective of this study was to determine the impact of multiple classes of androgen-targeting therapeutics (ATT) on the risk of NDD.
- Branigan, G. L., Torrandell-Haro, G., Vitali, F., Brinton, R. D., & Rodgers, K. (2022). Age and sex differences on anti-hyperglycemic medication exposure and risk of newly diagnosed multiple sclerosis in propensity score matched type 2 diabetics. Heliyon, 8(10), e11196.More infoThe association between exposure to anti-hyperglycemic medications (A-HgM) for Type 2 Diabetes Mellitus (T2D) treatment and Multiple Sclerosis (MS) in T2D patients is unclear.
- Mishra, A., Wang, Y., Yin, F., Vitali, F., Rodgers, K. E., Soto, M., Mosconi, L., Wang, T., & Brinton, R. D. (2022). A tale of two systems: Lessons learned from female mid-life aging with implications for Alzheimer's prevention & treatment. Ageing research reviews, 74, 101542.More infoNeurological aging is frequently viewed as a linear process of decline, whereas in reality, it is a dynamic non-linear process. The dynamic nature of neurological aging is exemplified during midlife in the female brain. To investigate fundamental mechanisms of midlife aging that underlie risk for development of Alzheimer's disease (AD) in late life, we investigated the brain at greatest risk for the disease, the aging female brain. Outcomes of our research indicate that mid-life aging in the female is characterized by the emergence of three phases: early chronological (pre-menopause), endocrinological (peri-menopause) and late chronological (post-menopause) aging. The endocrinological aging program is sandwiched between early and late chronological aging. Throughout the three stages of midlife aging, two systems of biology, metabolic and immune, are tightly integrated through a network of signaling cascades. The network of signaling between these two systems of biology underlie an orchestrated sequence of adaptative starvation responses that shift the brain from near exclusive dependence on a single fuel, glucose, to utilization of an auxiliary fuel derived from lipids, ketone bodies. The dismantling of the estrogen control of glucose metabolism during mid-life aging is a critical contributor to the shift in fuel systems and emergence of dynamic neuroimmune phenotype. The shift in fuel reliance, puts the largest reservoir of local fatty acids, white matter, at risk for catabolism as a source of lipids to generate ketone bodies through astrocytic beta oxidation. APOE4 genotype accelerates the tipping point for emergence of the bioenergetic crisis. While outcomes derived from research conducted in the female brain are not directly translatable to the male brain, the questions addressed in a female centric program of research are directly applicable to investigation of the male brain. Like females, males with AD exhibit deficits in the bioenergetic system of the brain, activation of the immune system and hallmark Alzheimer's pathologies. The drivers and trajectory of mechanisms underlying neurodegeneration in the male brain will undoubtedly share common aspects with the female in addition to factors unique to the male. Preclinical and clinical evidence indicate that midlife endocrine aging can also be a transitional bridge to autoimmune disorders. Collectively, the data indicate that endocrinological aging is a critical period "tipping point" in midlife which can initiate emergence of the prodromal stage of late-onset-Alzheimer's disease. Interventions that target both immune and metabolic shifts that occur during midlife aging have the potential to alter the trajectory of Alzheimer's risk in late life. Further, to achieve precision medicine for AD, chromosomal sex is a critical variable to consider along with APOE genotype, other genetic risk factors and stage of disease.
- Vitali, F. (2022). Translational potential of JAX humanized APOE mice: trajectories of resilient versus susceptible aging by sex and weight. Alzheimer's & Dementia, 18(S5). doi:10.1002/alz.068242
- Branigan, G. L., Vitali, F., & Brinton, R. D. (2021). Alzheimer’s disease prevention: Within reach by 2025. Alzheimer's & Dementia, 17(S10). doi:10.1002/alz.055621
- Vitali, F. (2021). Preventing Alzheimer's disease within reach by 2025: Targeted‐risk‐AD‐prevention (TRAP) strategy. Alzheimer's & Dementia: Translational Research & Clinical Interventions, 7(1). doi:10.1002/trc2.12190
- Vitali, F., Branigan, G. L., & Brinton, R. D. (2021). Preventing Alzheimer's disease within reach by 2025: Targeted-risk-AD-prevention (TRAP) strategy. Alzheimer's & dementia (New York, N. Y.), 7(1), e12190.More infoAlzheimer's disease (AD) is a progressive neurodegenerative disease that currently affects 6.2 million people in the United States and is projected to impact 12.7 million worldwide in 2050 with no effective disease-modifying therapeutic or cure. In 2011 as part of the National Alzheimer's Project Act, the National Plan to Address Alzheimer's Disease was signed into law which proposed to effectively prevent AD by 2025, which is rapidly approaching. The preclinical phase of AD can begin 20 years prior to diagnosis, which provides an extended window for preventive measures that would exert a transformative impact on incidence and prevalence of AD.
- Vitali, F., Torrandell-haro, G., Brinton, R. D., & Branigan, G. L. (2021). Statin therapies reduce risk of Alzheimer’s disease and dementia with increased protective therapeutic effect with increasing age. Alzheimer's & Dementia, 17(S10). doi:10.1002/alz.055735
- Balli, M., Vitali, F., Janiszewski, A., Caluwé, E., Cortés-Calabuig, A., Carpentier, S., Duelen, R., Ronzoni, F., Marcelis, L., Bosisio, F. M., Bellazzi, R., Luttun, A., De Angelis, M. G., Ceccarelli, G., Lluis, F., & Sampaolesi, M. (2020). Autologous micrograft accelerates endogenous wound healing response through ERK-induced cell migration. Cell death and differentiation, 27(5), 1520-1538.More infoDefective cell migration causes delayed wound healing (WH) and chronic skin lesions. Autologous micrograft (AMG) therapies have recently emerged as a new effective and affordable treatment able to improve wound healing capacity. However, the precise molecular mechanism through which AMG exhibits its beneficial effects remains unrevealed. Herein we show that AMG improves skin re-epithelialization by accelerating the migration of fibroblasts and keratinocytes. More specifically, AMG-treated wounds showed improvement of indispensable events associated with successful wound healing such as granulation tissue formation, organized collagen content, and newly formed blood vessels. We demonstrate that AMG is enriched with a pool of WH-associated growth factors that may provide the starting signal for a faster endogenous wound healing response. This work links the increased cell migration rate to the activation of the extracellular signal-regulated kinase (ERK) signaling pathway, which is followed by an increase in matrix metalloproteinase expression and their extracellular enzymatic activity. Overall we reveal the AMG-mediated wound healing transcriptional signature and shed light on the AMG molecular mechanism supporting its potential to trigger a highly improved wound healing process. In this way, we present a framework for future improvements in AMG therapy for skin tissue regeneration applications.
- Brinton, R. D., Vitali, F., Branigan, G. L., Torrandell‐Haro, G., Geifman, N., & Zissimopoulos, J. M. (2020). Statin therapy and risk of Alzheimer's and age‐related neurodegenerative diseases. Alzheimer's & Dementia: Translational Research & Clinical Interventions, 6(1). doi:10.1002/trc2.12108
- Nicora, G., Vitali, F., Dagliati, A., Geifman, N., & Bellazzi, R. (2020). Integrated Multi-Omics Analyses in Oncology: A Review of Machine Learning Methods and Tools. Frontiers in oncology, 10, 1030.More infoIn recent years, high-throughput sequencing technologies provide unprecedented opportunity to depict cancer samples at multiple molecular levels. The integration and analysis of these multi-omics datasets is a crucial and critical step to gain actionable knowledge in a precision medicine framework. This paper explores recent data-driven methodologies that have been developed and applied to respond major challenges of stratified medicine in oncology, including patients' phenotyping, biomarker discovery, and drug repurposing. We systematically retrieved peer-reviewed journals published from 2014 to 2019, select and thoroughly describe the tools presenting the most promising innovations regarding the integration of heterogeneous data, the machine learning methodologies that successfully tackled the complexity of multi-omics data, and the frameworks to deliver actionable results for clinical practice. The review is organized according to the applied methods: Deep learning, Network-based methods, Clustering, Features Extraction, and Transformation, Factorization. We provide an overview of the tools available in each methodological group and underline the relationship among the different categories. Our analysis revealed how multi-omics datasets could be exploited to drive precision oncology, but also current limitations in the development of multi-omics data integration.
- Sauta, E., Demartini, A., Vitali, F., Riva, A., & Bellazzi, R. (2020). A Bayesian data fusion based approach for learning genome-wide transcriptional regulatory networks. BMC bioinformatics, 21(1), 219.More infoReverse engineering of transcriptional regulatory networks (TRN) from genomics data has always represented a computational challenge in System Biology. The major issue is modeling the complex crosstalk among transcription factors (TFs) and their target genes, with a method able to handle both the high number of interacting variables and the noise in the available heterogeneous experimental sources of information.
- Torrandell-Haro, G., Branigan, G. L., Vitali, F., Geifman, N., Zissimopoulos, J. M., & Brinton, R. D. (2020). Statin therapy and risk of Alzheimer's and age-related neurodegenerative diseases. Alzheimer's & dementia (New York, N. Y.), 6(1), e12108.More infoEstablishing efficacy of and molecular pathways for statins has the potential to impact incidence of Alzheimer's and age-related neurodegenerative diseases (NDD).
- Weinkauf, C., Weinkauf, C., Vitali, F., Reiman, E. M., Brinton, R. D., Beach, T. G., Arias, J. A., Altbach, M. I., & Alexander, G. E. (2020). Carotid artery stenosis as a risk factor for Alzheimer’s disease: Human neuropathology/vascular. Alzheimers & Dementia, 16. doi:10.1002/alz.046162
- Balli, M., Vitali, F., Janiszewski, A., Caluwé, E., Cortés-Calabuig, A., Carpentier, S., Duelen, R., Ronzoni, F., Marcelis, L., Bosisio, F. M., Bellazzi, R., Luttun, A., De, A., Ceccarelli, G., Lluis, F., & Sampaolesi, M. (2019). Autologous micrograft accelerates endogenous wound healing response through ERK-induced cell migration. Cell Death & Differentiation.
- Berghout, J., Lussier, Y. A., Vitali, F., Bulyk, M. L., Kann, M. G., & Moore, J. H. (2019). Workshop during the Pacific Symposium of Biocomputing, Jan 3-7, 2019: Reading between the genes: interpreting non-coding DNA in high-throughput. Pacific Symposium of Biocomputing.
- Berghout, J., Lussier, Y. A., Vitali, F., Bulyk, M. L., Kann, M. G., & Moore, J. H. (2019). Workshop during the Pacific Symposium of Biocomputing, Jan 3-7, 2019: Reading between the genes: interpreting non-coding DNA in high-throughput. Pacific Symposium on Biocomputing. Pacific Symposium on Biocomputing, 24, 444-448.More infoIdentifying functional elements and predicting mechanistic insight from non-coding DNA and noncoding variation remains a challenge. Advances in genome-scale, high-throughput technology, however, have brought these answers closer within reach than ever, though there is still a need for new computational approaches to analysis and integration. This workshop aims to explore these resources and new computational methods applied to regulatory elements, chromatin interactions, non-protein-coding genes, and other non-coding DNA.
- Li, Q., Zaim, S. R., Aberasturi, D., Berghout, J., Li, H., Vitali, F., Kenost, C., Zhang, H. H., & Lussier, Y. A. (2019). Interpretation of 'Omics dynamics in a single subject using local estimates of dispersion between two transcriptomes. AMIA ... Annual Symposium proceedings. AMIA Symposium, 2019, 582-591.More infoCalculating Differentially Expressed Genes (DEGs) from RNA-sequencing requires replicates to estimate gene-wise variability, a requirement that is at times financially or physiologically infeasible in clinics. By imposing restrictive transcriptome-wide assumptions limiting inferential opportunities of conventional methods (edgeR, NOISeq-sim, DESeq, DEGseq), comparing two conditions without replicates (TCWR) has been proposed, but not evaluated. Under TCWR conditions (e.g., unaffected tissue vs. tumor), differences of transformed expression of the proposed individualized DEG (iDEG) method follow a distribution calculated across a local partition of related transcripts at baseline expression; thereafter the probability of each DEG is estimated by empirical Bayes with local false discovery rate control using a two-group mixture model. In extensive simulation studies of TCWR methods, iDEG and NOISeq are more accurate at 5%75%, false_positive_rate
- Lussier, Y. A., Zhang, H., Vitali, F., Berghout, J., Kenost, C., & Rachid Zaim, S. (2019). Evaluating single-subject study methods for personal transcriptomic interpretation to advance precision medicine. BMC Medical Genomics, 12(Suppl 5), 96. doi:doi:10.1186/s12920-019-0513-8
- Marini, S., Vitali, F., Rampazzi, S., Demartini, A., & Akutsu, T. (2019). Protease target prediction via matrix factorization. Bioinformatics (Oxford, England), 35(6), 923-929.More infoProtein cleavage is an important cellular event, involved in a myriad of processes, from apoptosis to immune response. Bioinformatics provides in silico tools, such as machine learning-based models, to guide the discovery of targets for the proteases responsible for protein cleavage. State-of-the-art models have a scope limited to specific protease families (such as Caspases), and do not explicitly include biological or medical knowledge (such as the hierarchical protein domain similarity or gene-gene interactions). To fill this gap, we present a novel approach for protease target prediction based on data integration.
- Rachid Zaim, S., Kenost, C., Berghout, J., Vitali, F., Zhang, H. H., & Lussier, Y. A. (2019). Evaluating single-subject study methods for personal transcriptomic interpretations to advance precision medicine. BMC medical genomics, 12(Suppl 5), 96.More infoGene expression profiling has benefited medicine by providing clinically relevant insights at the molecular candidate and systems levels. However, to adopt a more 'precision' approach that integrates individual variability including 'omics data into risk assessments, diagnoses, and therapeutic decision making, whole transcriptome expression needs to be interpreted meaningfully for single subjects. We propose an "all-against-one" framework that uses biological replicates in isogenic conditions for testing differentially expressed genes (DEGs) in a single subject (ss) in the absence of an appropriate external reference standard or replicates. To evaluate our proposed "all-against-one" framework, we construct reference standards (RSs) with five conventional replicate-anchored analyses (NOISeq, DEGseq, edgeR, DESeq, DESeq2) and the remainder were treated separately as single-subject sample pairs for ss analyses (without replicates).
- Vitali, F., Berghout, J., Fan, J., Li, J., Li, Q., Li, H., & Lussier, Y. A. (2019). Precision drug repurposing via convergent eQTL-based molecules and pathway targeting independent disease-associated polymorphisms. Pacific Symposium on Biocomputing. Pacific Symposium on Biocomputing, 24, 308-319.More infoRepurposing existing drugs for new therapeutic indications can improve success rates and streamline development. Use of large-scale biomedical data repositories, including eQTL regulatory relationships and genome-wide disease risk associations, offers opportunities to propose novel indications for drugs targeting common or convergent molecular candidates associated to two or more diseases. This proposed novel computational approach scales across 262 complex diseases, building a multi-partite hierarchical network integrating (i) GWAS-derived SNP-to-disease associations, (ii) eQTL-derived SNP-to-eGene associations incorporating both cis- and trans-relationships from 19 tissues, (iii) protein target-to-drug, and (iv) drug-to-disease indications with (iv) Gene Ontology-based information theoretic semantic (ITS) similarity calculated between protein target functions. Our hypothesis is that if two diseases are associated to a common or functionally similar eGene - and a drug targeting that eGene/protein in one disease exists - the second disease becomes a potential repurposing indication. To explore this, all possible pairs of independently segregating GWAS-derived SNPs were generated, and a statistical network of similarity within each SNP-SNP pair was calculated according to scale-free overrepresentation of convergent biological processes activity in regulated eGenes (ITSeGENE-eGENE) and scale-free overrepresentation of common eGene targets between the two SNPs (ITSSNP-SNP). Significance of ITSSNP-SNP was conservatively estimated using empirical scale-free permutation resampling keeping the node-degree constant for each molecule in each permutation. We identified 26 new drug repurposing indication candidates spanning 89 GWAS diseases, including a potential repurposing of the calcium-channel blocker Verapamil from coronary disease to gout. Predictions from our approach are compared to known drug indications using DrugBank as a gold standard (odds ratio=13.1, p-value=2.49x10-8). Because of specific disease-SNPs associations to candidate drug targets, the proposed method provides evidence for future precision drug repositioning to a patient's specific polymorphisms.
- Li, H., Fan, J., Vitali, F., Berghout, J., Aberasturi, D., Li, J., Wilson, L., Chiu, W., Pumarejo, M., Han, J., Kenost, C., Koripella, P. C., Pouladi, N., Billheimer, D., Bedrick, E. J., & Lussier, Y. A. (2018). Novel disease syndromes unveiled by integrative multiscale network analysis of diseases sharing molecular effectors and comorbidities. BMC medical genomics, 11(Suppl 6), 112.More infoForty-two percent of patients experience disease comorbidity, contributing substantially to mortality rates and increased healthcare costs. Yet, the possibility of underlying shared mechanisms for diseases remains not well established, and few studies have confirmed their molecular predictions with clinical datasets.
- Lussier, Y. A., Berghout, J., Vitali, F., Ramos, K. S., Kann, M., & Moore, J. H. (2018). Reading Between the Genes: Computational Models to Discover Function from Noncoding DNA. Pacific Symposium on Biocomputing. Pacific Symposium on Biocomputing, 23, 507-511.More infoNoncoding DNA - once called "junk" has revealed itself to be full of function. Technology development has allowed researchers to gather genome-scale data pointing towards complex regulatory regions, expression and function of noncoding RNA genes, and conserved elements. Variation in these regions has been tied to variation in biological function and human disease. This PSB session tackles the problem of handling, analyzing and interpreting the data relating to variation in and interactions between noncoding regions through computational biology. We feature an invited speaker to how variation in transcription factor coding sequences impacts on sequence preference, along with submitted papers that span graph based methods, integrative analyses, machine learning, and dimension reduction to explore questions of basic biology, cancer, diabetes, and clinical relevance.
- Taira, M., Imamura, M., Takahashi, A., Kamatani, Y., Yamauchi, T., Araki, S., Tanaka, N., Zuydam, N. R., Ahlqvist, E., Toyoda, M., & others, . (2018). A variant within the FTO confers susceptibility to diabetic nephropathy in Japanese patients with type 2 diabetes. PloS one, 13(12), e0208654.
- Vella, D., Marini, S., Vitali, F., Di Silvestre, D., Mauri, G., & Bellazzi, R. (2018). MTGO: PPI Network Analysis Via Topological and Functional Module Identification. Scientific reports, 8(1), 5499.More infoProtein-protein interaction (PPI) networks are viable tools to understand cell functions, disease machinery, and drug design/repositioning. Interpreting a PPI, however, it is a particularly challenging task because of network complexity. Several algorithms have been proposed for an automatic PPI interpretation, at first by solely considering the network topology, and later by integrating Gene Ontology (GO) terms as node similarity attributes. Here we present MTGO - Module detection via Topological information and GO knowledge, a novel functional module identification approach. MTGO let emerge the bimolecular machinery underpinning PPI networks by leveraging on both biological knowledge and topological properties. In particular, it directly exploits GO terms during the module assembling process, and labels each module with its best fit GO term, easing its functional interpretation. MTGO shows largely better results than other state of the art algorithms (including recent GO-based ones) when searching for small or sparse functional modules, while providing comparable or better results all other cases. MTGO correctly identifies molecular complexes and literature-consistent processes in an experimentally derived PPI network of Myocardial infarction. A software version of MTGO is available freely for non-commercial purposes at https://gitlab.com/d1vella/MTGO .
- Vitali, F. (2018). Patient similarity by joint matrix trifactorization to identify subgroups in acute myeloid leukemia. JAMIA Open, 1(1), 75-86. doi:10.1093/jamiaopen/ooy008
- Vitali, F. (2018). Protease target prediction via matrix factorization. Bioinformatics, 35(6), 923-929. doi:10.1093/bioinformatics/bty746
- Vitali, F., Marini, S., Pala, D., Demartini, A., Montoli, S., Zambelli, A., & Bellazzi, R. (2018). Patient similarity by joint matrix trifactorization to identify subgroups in acute myeloid leukemia. JAMIA Open.
- Vitali, F., Marini, S., Rampazzi, S., Demartini, A., & Akutsu, T. (2018). Protease target prediction via matrix factorization. Bioinformatics. doi:1367-4803
- Vitali, F. (2017). Developing a ‘personalome’ for precision medicine: emerging methods that compute interpretable effect sizes from single-subject transcriptomes. Briefings in Bioinformatics, 20(3), 789-805. doi:10.1093/bib/bbx149
- Vitali, F., Li, Q., Schissler, A. G., Berghout, J., Kenost, C., & Lussier, Y. A. (2017). Developing a 'personalome' for precision medicine: emerging methods that compute interpretable effect sizes from single-subject transcriptomes. Briefings in bioinformatics. doi:https://doi.org/10.1093/bib/bbx149More infoThe development of computational methods capable of analyzing -omics data at the individual level is critical for the success of precision medicine. Although unprecedented opportunities now exist to gather data on an individual's -omics profile ('personalome'), interpreting and extracting meaningful information from single-subject -omics remain underdeveloped, particularly for quantitative non-sequence measurements, including complete transcriptome or proteome expression and metabolite abundance. Conventional bioinformatics approaches have largely been designed for making population-level inferences about 'average' disease processes; thus, they may not adequately capture and describe individual variability. Novel approaches intended to exploit a variety of -omics data are required for identifying individualized signals for meaningful interpretation. In this review-intended for biomedical researchers, computational biologists and bioinformaticians-we survey emerging computational and translational informatics methods capable of constructing a single subject's 'personalome' for predicting clinical outcomes or therapeutic responses, with an emphasis on methods that provide interpretable readouts.
- Vitali, F., Marini, S., Balli, M., Grosemans, H., Sampaolesi, M., Lussier, Y. A., Cusella De Angelis, M. G., & Bellazzi, R. (2017). Exploring Wound-Healing Genomic Machinery with a Network-Based Approach. Pharmaceuticals (Basel, Switzerland), 10(2).More infoThe molecular mechanisms underlying tissue regeneration and wound healing are still poorly understood despite their importance. In this paper we develop a bioinformatics approach, combining biology and network theory to drive experiments for better understanding the genetic underpinnings of wound healing mechanisms and for selecting potential drug targets. We start by selecting literature-relevant genes in murine wound healing, and inferring from them a Protein-Protein Interaction (PPI) network. Then, we analyze the network to rank wound healing-related genes according to their topological properties. Lastly, we perform a procedure for in-silico simulation of a treatment action in a biological pathway. The findings obtained by applying the developed pipeline, including gene expression analysis, confirms how a network-based bioinformatics method is able to prioritize candidate genes for in vitro analysis, thus speeding up the understanding of molecular mechanisms and supporting the discovery of potential drug targets.
- Vitali, F., Cohen, L. D., Demartini, A., Amato, A., Eterno, V., Zambelli, A., & Bellazzi, R. (2016). A Network-Based Data Integration Approach to Support Drug Repurposing and Multi-Target Therapies in Triple Negative Breast Cancer. PloS one, 11(9), e0162407.More infoThe integration of data and knowledge from heterogeneous sources can be a key success factor in drug design, drug repurposing and multi-target therapies. In this context, biological networks provide a useful instrument to highlight the relationships and to model the phenomena underlying therapeutic action in cancer. In our work, we applied network-based modeling within a novel bioinformatics pipeline to identify promising multi-target drugs. Given a certain tumor type/subtype, we derive a disease-specific Protein-Protein Interaction (PPI) network by combining different data-bases and knowledge repositories. Next, the application of suitable graph-based algorithms allows selecting a set of potentially interesting combinations of drug targets. A list of drug candidates is then extracted by applying a recent data fusion approach based on matrix tri-factorization. Available knowledge about selected drugs mechanisms of action is finally exploited to identify the most promising candidates for planning in vitro studies. We applied this approach to the case of Triple Negative Breast Cancer (TNBC), a subtype of breast cancer whose biology is poorly understood and that lacks of specific molecular targets. Our "in-silico" findings have been confirmed by a number of in vitro experiments, whose results demonstrated the ability of the method to select candidates for drug repurposing.
- Vitali, F., Mulas, F., Marini, P., & Bellazzi, R. (2013). Network-based target ranking for polypharmacological therapies. Journal of biomedical informatics, 46(5), 876-81.More infoWith the growing understanding of complex diseases, the focus of drug discovery has shifted from the well-accepted "one target, one drug" model, to a new "multi-target, multi-drug" model, aimed at systemically modulating multiple targets. In this context, polypharmacology has emerged as a new paradigm to overcome the recent decline in productivity of pharmaceutical research. However, finding methods to evaluate multicomponent therapeutics and ranking synergistic agent combinations is still a demanding task. At the same time, the data gathered on complex diseases has been progressively collected in public data and knowledge repositories, such as protein-protein interaction (PPI) databases. The PPI networks are increasingly used as universal platforms for data integration and analysis. A novel computational network-based approach for feasible and efficient identification of multicomponent synergistic agents is proposed in this paper. Given a complex disease, the method exploits the topological features of the related PPI network to identify possible combinations of hit targets. The best ranked combinations are subsequently computed on the basis of a synergistic score. We illustrate the potential of the method through a study on Type 2 Diabetes Mellitus. The results highlight its ability to retrieve novel target candidates, which role is also confirmed by the analysis of the related literature.
Proceedings Publications
- Demartini, A., Vitali, F., Sauta, E., Della Porta, M. G., Bellazzi, R., & Marini, S. (2019). Gene interaction discovery in myelodysplastic syndromes. In EUROPEAN JOURNAL OF HUMAN GENETICS, 27.
- Li, Q., Lussier, Y. A., Lussier, Y. A., Zaim, S., Zhang, H., Zhang, H., Kenost, C., Aberasturi, D., Kenost, C., Vitali, F., Vitali, F., Berghout, J., Li, H., Li, H., Li, H., Berghout, J., Berghout, J., Vitali, F., Aberasturi, D., , Kenost, C., et al. (2019, Nov). Interpretation of ‘Omics dynamics in a single subject using local estimates of dispersion between two transcriptomes. In AMIA Annual Symposium.
- Vitali, F., Berghout, J., Fan, J., Li, J., Li, Q., Li, H., & Lussier, Y. A. (2019). Precision drug repurposing via convergent eQTL-based molecules and pathway targeting independent disease-associated polymorphisms. In Pac Symp Biocomput.
- Berghout, J., Vitali, F., Moore, J. H., Lussier, Y. A., Kann, M. G., Bulyk, M. L., & Berghout, J. (2018). Workshop during the pacific symposium of biocomputing, Jan 3-7, 2019: Reading between the genes: Interpreting non-coding DNA in high-throughput. In Biocomputing 2019, 24, 444-448.More infoIdentifying functional elements and predicting mechanistic insight from non-coding DNA and noncoding variation remains a challenge. Advances in genome-scale, high-throughput technology, however, have brought these answers closer within reach than ever, though there is still a need for new computational approaches to analysis and integration. This workshop aims to explore these resources and new computational methods applied to regulatory elements, chromatin interactions, non-protein-coding genes, and other non-coding DNA.
- Fan, J., Vitali, F., Lussier, Y. A., Li, Q., Li, J., Li, H., Fan, J., & Berghout, J. (2018). Precision drug repurposing via convergent eQTL-based molecules and pathway targeting independent disease-associated polymorphisms.. In Biocomputing 2019, 24, 308-319.More infoRepurposing existing drugs for new therapeutic indications can improve success rates and streamline development. Use of large-scale biomedical data repositories, including eQTL regulatory relationships and genome-wide disease risk associations, offers opportunities to propose novel indications for drugs targeting common or convergent molecular candidates associated to two or more diseases. This proposed novel computational approach scales across 262 complex diseases, building a multi-partite hierarchical network integrating (i) GWAS-derived SNP-to-disease associations, (ii) eQTL-derived SNP-to-eGene associations incorporating both cis- and trans-relationships from 19 tissues, (iii) protein target-to-drug, and (iv) drug-to-disease indications with (iv) Gene Ontology-based information theoretic semantic (ITS) similarity calculated between protein target functions. Our hypothesis is that if two diseases are associated to a common or functionally similar eGene - and a drug targeting that eGene/protein in one disease exists - the second disease becomes a potential repurposing indication. To explore this, all possible pairs of independently segregating GWAS-derived SNPs were generated, and a statistical network of similarity within each SNP-SNP pair was calculated according to scale-free overrepresentation of convergent biological processes activity in regulated eGenes (ITSeGENE-eGENE) and scale-free overrepresentation of common eGene targets between the two SNPs (ITSSNP-SNP). Significance of ITSSNP-SNP was conservatively estimated using empirical scale-free permutation resampling keeping the node-degree constant for each molecule in each permutation. We identified 26 new drug repurposing indication candidates spanning 89 GWAS diseases, including a potential repurposing of the calcium-channel blocker Verapamil from coronary disease to gout. Predictions from our approach are compared to known drug indications using DrugBank as a gold standard (odds ratio=13.1, p-value=2.49x10-8). Because of specific disease-SNPs associations to candidate drug targets, the proposed method provides evidence for future precision drug repositioning to a patient's specific polymorphisms.
- Vitali, F., Ramos, K. S., Moore, J. H., Lussier, Y. A., Kann, M. G., & Berghout, J. (2018). Reading Between the Genes: Computational Models to Discover Function from Noncoding DNA.. In Biocomputing 2018, 23, 507-511.More infoNoncoding DNA - once called "junk" has revealed itself to be full of function. Technology development has allowed researchers to gather genome-scale data pointing towards complex regulatory regions, expression and function of noncoding RNA genes, and conserved elements. Variation in these regions has been tied to variation in biological function and human disease. This PSB session tackles the problem of handling, analyzing and interpreting the data relating to variation in and interactions between noncoding regions through computational biology. We feature an invited speaker to how variation in transcription factor coding sequences impacts on sequence preference, along with submitted papers that span graph based methods, integrative analyses, machine learning, and dimension reduction to explore questions of basic biology, cancer, diabetes, and clinical relevance.
- Vitali, F., Sauta, E., Riva, A., Demartini, A., & Bellazzi, R. (2017). Data Fusion Approach for Learning Transcriptional Bayesian Networks. In Lecture Notes in Computer Science book series, 76-80.More infoThe complexity of gene expression regulation relies on the synergic nature underlying the molecular interplay among its principal actors, transcription factors (TFs). Exerting a spatiotemporal control on their target genes, they define transcriptional programs across the genome, which are strongly perturbed in a disease context. In order to gain a more comprehensive picture of these complex dynamics, a data fusion approach, aimed at performing the integration of heterogeneous -omics data is fundamental.
Presentations
- Vitali, F. (2019, 01). Novel and emerging data fusion strategies for integrating health and biomolecular data. Pacific Symposium on Biocomputing 2019. Big Island, Hawaii.
- Vitali, F., Berghout, J., Fun, J., Li, J., Li, Q., Li, H., & Lussier, Y. A. (2019, 01). Precision drug repositioning via convergent eQTL molecules and pathways targeting indipendent distinct disease-associated polymorphisms. Pacific Symposium on Biocomputing 2019. Big Island, Hawaii.
Poster Presentations
- Vitali, F., Raikes, A. C., Hernandez, G. D., & Yin, F. (2023). Sex-and APOE-Specific Transcriptomic Signatures in Alzheimer’s Disease: An AZ-ADRC-Research Education Scholars Team Science Project. Alzheimer's & Dementia.
- Vitali, F., Zhang, J., Wang, T., & Brinton, R. D. (2023). Computational identification of precision therapeutics for Alzheimer’s disease specific to endocrine and APOE genotype status. Alzheimer's & Dementia.
- Berghout, J., Thompson, G. R., Bays, D., Waldman, S., Stewart, E., Hsu, A. P., Powell, D. P., Chaput, A. L., Pouladi, N., Vitali, F., Shubitz, L., Holland, S. M., Frelinger, J. A., Galgiani, J. N., & Lussier, Y. A. (2019, Nov). Collaborative Arizona and California combined cohort to investigate the role of rare genetic variants in susceptibility to disseminated coccidioidomycosis (Valley fever). reimagine Health: Is my fate in my genes? 2nd Annual University of Arizona Research Symposium. Phoenix, AZ: University of Arizona.
- Berghout, J., Thompson, G. R., Bays, D., Waldman, S., Stewart, E., Hsu, A. P., Powell, D. P., Chaput, A. L., Pouladi, N., Vitali, F., Shubitz, L., Holland, S. M., Frelinger, J. A., Galgiani, J. N., & Lussier, Y. A. (2019, Oct). Identification of rare variants contributing to disseminated coccidioidomycosis after infection. American Society of Human Genetics. Houston, TX.More infoASHG Abstract ID 30302128
- Berghout, J., Vitali, F., Fan, J., Li, J., Li, Q., Li, H., Lussier, Y. A., Lussier, Y. A., Li, H., Li, Q., Li, J., Fan, J., Vitali, F., & Berghout, J. (2018, Dec 2018). Precision drug repurposing via convergent eQTL-based molecules and pathway targeting independent disease-associated polymorphisms. Biomarkers: From Specimen to Clinical Impact - University of Arizona Research Symposium. University of Arizona COM - Phoenix: University of Arizona.
- Vitali, F., Marini, S., Pala, D., Zambelli, A., & Bellazzi, R. (2017, 11). A matrix trifactorization algorithm to identify patient similarities and subgroups in acute myeloid leukemia. Biomarkers: From Specimen to Clinical Impact - University of Arizona Research Symposium. Phoenix: University of Arizona.
Others
- Branigan, G., Haro, G. T., Vitali, F., Brinton, R., & Rodgers, K. (2023). Implications for Age and Sex Differences on Anti-Hyperglycemic Therapy Exposure and Incidence of Newly Diagnosed Multiple Sclerosis in Propensity Score Matched Type 2 Diabetics (P9-3.007).
- Lee, S. H., Pouladi, N., Kenost, C., Vitali, F., & Lussier, Y. A. (2019). MicroRNAs response to concurrent chemoradiotherapy predicts oligo-and polymetastatic progression in patients with Stage IV colorectal adenocarcinoma.