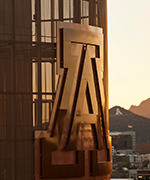
Jian Liu
- Associate Professor, Systems and Industrial Engineering
- Associate Professor, Statistics-GIDP
- Member of the Graduate Faculty
- (520) 621-6548
- Engineering, Rm. 221
- Tucson, AZ 85721
- jianliu@arizona.edu
Biography
Dr. Jian Liuis an Associate Professor in the Department of Systems & Industrial Engineering at the University of Arizona and an affiliated faculty member in the Graduate Interdisciplinary Degree Program at The University of Arizona. He received the B.S. and M.S. degrees in Precision Instruments & Mechanology from the Tsinghua University, China in 1999 and 2002, respectively; the M.S. degree in Industrial Engineering, the M.S. degree in Statistics and the Ph.D. in Mechanical Engineering and Industrial and Operation Engineering, all from the University of Michigan in 2005, 2006 and 2008, respectively.
Dr. Liu has more than ten years of research experience in data analytics and system informatics. His research specialty is in the fusion of multi-source, multi-scale and multi-level information in hierarchical and distributed systems for better system design, operation, and maintenance. By integrating engineering knowledge, optimization algorithms and the statistical analysis and learning of massive high-dimensional data, Dr. Liu and his research team advance the scientific research in system performance modeling, system prognostic/diagnostic, decision-making and risk management. These methodological advancements have been successfully applied in a variety of domains, such as manufacturing engineering systems, chemical and civil engineering systems and geographical information systems. His collaborations with domain experts have resulted in joint research projects in quality and reliability improvement for machining/assembly processes, mono-crystalline processes, and service improvement for water systems, software systems, and food assistance provision systems. Dr. Liu and his research team have published research papers in prestigious journals and conference proceedings, such as IISE-Transactions, IEEE Transactions, ASME Transactions and IISE annual conference proceedings. Dr. Liu’s research has been funded by US National Science Foundation, US Department of Homeland Security and US Air Force Office of Scientific Research.
Dr. Liu is a member of INFORMS and a member of IISE. He served as a Council Member of Quality, Statistics and Reliability Section of INFORMS from 2012 to 2014, a Board Director of the Quality Control and Reliability Engineering (QCRE) Division of IISE from 2013 to 2015, the President-Elect of QCRE from 2015 to 2016. Dr. Liu is currently the President of the QCRE of IISE.
Degrees
- Ph.D. Industrial and Operations Engineering and Mechanical Engineering
- The University of Michigan, Ann Arbor, Michigan, United States
- M.S. Statistics
- The University of Michigan, Ann Arbor, Michigan, United States
- M.S. Industrial and Operations Engineering
- The University of Michigan, Ann Arbor, Michigan, United States
- M.S. Mechanical Engineering
- Tsinghua University, Beijing, Beijing, China
- B.S. Precision Instruments & Mechanology
- Tsinghua University, Beijing, Beijing, China
Work Experience
- Department of Systems and Industrial Engineering, The University of Arizona (2014 - Ongoing)
- Statistics Graduate Interdisciplinary Degree Programs (2010 - Ongoing)
- Department of Systems and Industrial Engineering, The University of Arizona (2008 - 2014)
Awards
- Honorable Mention for the Best Paper in the 2020 IISE Transactions Focus Issue on Quality and Reliability Engineering
- Institute of Systems and Industrial Engineering, Spring 2021 (Award Finalist)
- Honorable Mention for the Best Paper Award
- International Conference on Industrial Engineering and Engineering Management, 2020, Fall 2020
- Outstanding Associate Editor Award
- Journal of Manufacturing Systems, Transactions of Society of Manufacturing Engineering, Spring 2019
Interests
Research
Data Analytics; Statistical Quality Control; System Reliability Modeling and Analysis; Manufacturing Process Improvement; Machine Learning and Image Processing.
Teaching
Engineering Statistics; Quality Engineering; Applied Multivariate Statistics; Design of Experiment; Reliability Engineering;
Courses
2024-25 Courses
-
Dissertation
SIE 920 (Fall 2024) -
Engineering Statistics
SIE 430 (Fall 2024) -
Engineering Statistics
SIE 530 (Fall 2024) -
Quality Engineering
SIE 406 (Fall 2024) -
Quality Engineering
SIE 506 (Fall 2024)
2023-24 Courses
-
Dissertation
SIE 920 (Spring 2024) -
Dissertation
STAT 920 (Spring 2024) -
Fund of Data Science for Engrs
SIE 433 (Spring 2024) -
Fund. of Data Science for Engr
SIE 533 (Spring 2024) -
Dissertation
SIE 920 (Fall 2023) -
Dissertation
STAT 920 (Fall 2023) -
Engineering Statistics
SIE 430 (Fall 2023) -
Engineering Statistics
SIE 530 (Fall 2023) -
Quality Engineering
SIE 406 (Fall 2023) -
Quality Engineering
SIE 506 (Fall 2023) -
Research
SIE 900 (Fall 2023)
2022-23 Courses
-
Dissertation
SIE 920 (Spring 2023) -
Dissertation
STAT 920 (Spring 2023) -
Fund of Data Science for Engrs
SIE 433 (Spring 2023) -
Fund. of Data Science for Engr
SIE 533 (Spring 2023) -
Research
SIE 900 (Spring 2023) -
Thesis
SIE 910 (Spring 2023) -
Dissertation
SIE 920 (Fall 2022) -
Dissertation
STAT 920 (Fall 2022) -
Engineering Statistics
SIE 430 (Fall 2022) -
Engineering Statistics
SIE 530 (Fall 2022) -
Intro SIE Mthd:Prob+Stat
SIE 500A (Fall 2022) -
Quality Engineering
SIE 406 (Fall 2022) -
Quality Engineering
SIE 506 (Fall 2022) -
Research
SIE 900 (Fall 2022)
2021-22 Courses
-
Internship
SIE 493 (Summer I 2022) -
Dissertation
SIE 920 (Spring 2022) -
Dissertation
STAT 920 (Spring 2022) -
Engineering Statistics
SIE 530 (Spring 2022) -
Intro SIE Mthd:Prob+Stat
SIE 500A (Spring 2022) -
Quality Engineering
SIE 406 (Spring 2022) -
Quality Engineering
SIE 506 (Spring 2022) -
Research
SIE 900 (Spring 2022) -
Dissertation
SIE 920 (Fall 2021) -
Dissertation
STAT 920 (Fall 2021) -
Engineering Statistics
SIE 430 (Fall 2021) -
Engineering Statistics
SIE 530 (Fall 2021) -
Fund of Data Science for Engrs
SIE 433 (Fall 2021) -
Fund. of Data Science for Engr
SIE 533 (Fall 2021) -
Independent Study
SIE 599 (Fall 2021) -
Intro SIE Mthd:Prob+Stat
SIE 500A (Fall 2021) -
Research
SIE 900 (Fall 2021)
2020-21 Courses
-
Internship
SIE 493 (Summer I 2021) -
Dissertation
SIE 920 (Spring 2021) -
Independent Study
SIE 599 (Spring 2021) -
Independent Study
SIE 699 (Spring 2021) -
Internship
SIE 593 (Spring 2021) -
Intro SIE Mthd:Prob+Stat
SIE 500A (Spring 2021) -
Master's Report
SIE 909 (Spring 2021) -
Quality Engineering
SIE 406 (Spring 2021) -
Quality Engineering
SIE 506 (Spring 2021) -
Research
SIE 900 (Spring 2021) -
Dissertation
SIE 920 (Fall 2020) -
Engineering Statistics
SIE 430 (Fall 2020) -
Engineering Statistics
SIE 530 (Fall 2020) -
Fund of Data Science for Engrs
SIE 433 (Fall 2020) -
Fund. of Data Science for Engr
SIE 533 (Fall 2020) -
Independent Study
SIE 699 (Fall 2020) -
Internship
SIE 493 (Fall 2020)
2019-20 Courses
-
Internship
SIE 493 (Summer I 2020) -
Dissertation
SIE 920 (Spring 2020) -
Independent Study
SIE 599 (Spring 2020) -
Internship
SIE 493 (Spring 2020) -
Intro SIE Mthd:Prob+Stat
SIE 500A (Spring 2020) -
Master's Report
SIE 909 (Spring 2020) -
Quality Engineering
SIE 406 (Spring 2020) -
Quality Engineering
SIE 506 (Spring 2020) -
Research
SIE 900 (Spring 2020) -
Dissertation
SIE 920 (Fall 2019) -
Engineering Statistics
SIE 430 (Fall 2019) -
Engineering Statistics
SIE 530 (Fall 2019) -
Fund of Data Science for Engrs
SIE 433 (Fall 2019) -
Fund. of Data Science for Engr
SIE 533 (Fall 2019) -
Independent Study
SIE 599 (Fall 2019) -
Internship
SIE 493 (Fall 2019) -
Intro SIE Mthd:Prob+Stat
SIE 500A (Fall 2019) -
Master's Report
SIE 909 (Fall 2019)
2018-19 Courses
-
Directed Research
SIE 492 (Summer I 2019) -
Independent Study
SIE 499 (Summer I 2019) -
Internship
SIE 493 (Summer I 2019) -
Adv Quality Engineering
SIE 606 (Spring 2019) -
Dissertation
SIE 920 (Spring 2019) -
Internship
SIE 493 (Spring 2019) -
Intro SIE Mthd:Prob+Stat
SIE 500A (Spring 2019) -
Master's Report
SIE 909 (Spring 2019) -
Quality Engineering
SIE 406 (Spring 2019) -
Quality Engineering
SIE 506 (Spring 2019) -
Research
SIE 900 (Spring 2019) -
Senior Dsgn Projects II
SIE 498B (Spring 2019) -
Dissertation
SIE 920 (Fall 2018) -
Engineering Statistics
SIE 430 (Fall 2018) -
Engineering Statistics
SIE 530 (Fall 2018) -
Internship
SIE 493 (Fall 2018) -
Master's Report
SIE 909 (Fall 2018) -
Research
SIE 900 (Fall 2018) -
Senior Design Projects I
SIE 498A (Fall 2018)
2017-18 Courses
-
Directed Research
SIE 492 (Summer I 2018) -
Independent Study
SIE 499 (Summer I 2018) -
Internship
SIE 493 (Summer I 2018) -
Adv Quality Engineering
SIE 606 (Spring 2018) -
Dissertation
SIE 920 (Spring 2018) -
Intro SIE Mthd:Prob+Stat
SIE 500A (Spring 2018) -
Master's Report
SIE 909 (Spring 2018) -
Quality Engineering
SIE 406 (Spring 2018) -
Quality Engineering
SIE 506 (Spring 2018) -
Research
SIE 900 (Spring 2018) -
Dissertation
SIE 920 (Fall 2017) -
Engineering Statistics
SIE 430 (Fall 2017) -
Engineering Statistics
SIE 530 (Fall 2017) -
Intro SIE Mthd:Prob+Stat
SIE 500A (Fall 2017) -
Master's Report
SIE 909 (Fall 2017)
2016-17 Courses
-
Adv Quality Engineering
SIE 606 (Spring 2017) -
Dissertation
SIE 920 (Spring 2017) -
Quality Engineering
SIE 406 (Spring 2017) -
Quality Engineering
SIE 506 (Spring 2017) -
Research
SIE 900 (Spring 2017) -
Dissertation
SIE 920 (Fall 2016) -
Engineering Statistics
SIE 430 (Fall 2016) -
Engineering Statistics
SIE 530 (Fall 2016) -
Intro SIE Mthd:Prob+Stat
SIE 500A (Fall 2016) -
Research
SIE 900 (Fall 2016)
2015-16 Courses
-
Research
SIE 900 (Spring 2016)
Scholarly Contributions
Journals/Publications
- Frantziskonis, G. N., Liu, J., Zhang, Y., & Nikravesh, Y. (2022). A partition and microstructure based method applicable to large-scale topology optimization. Mechanics of Materials, 166(104234), 104234.
- Liu, J., Son, Y., & Yuan, Y. (2022). Bayesian Modeling of Crowd Dynamics by Aggregating Multiresolution Observations From UAVs and UGVs. IEEE Transactions on Systems, Man, and Cybernetics: Systems, 52(10), 6406-6417. doi:10.1109/tsmc.2022.3146455
- Wang, C., Liu, J., Yang, Q., Hu, Q., & Yu, D. (2022). Recoverability effects on reliability assessment for accelerated degradation testing. IISE Transactions, 1-13. doi:10.1080/24725854.2022.2089784
- Baldwin, E., Han, J., Luo, W., Zhou, J., An, L., Liu, J., Zhang, H. H., & Li, H. (2020). On fusion methods for knowledge discovery from multi-omics datasets. Computational and structural biotechnology journal, 18, 509-517.More infoRecent years have witnessed the tendency of measuring a biological sample on multiple omics scales for a comprehensive understanding of how biological activities on varying levels are perturbed by genetic variants, environments, and their interactions. This new trend raises substantial challenges to data integration and fusion, of which the latter is a specific type of integration that applies a uniform method in a scalable manner, to solve biological problems which the multi-omics measurements target. Fusion-based analysis has advanced rapidly in the past decade, thanks to application drivers and theoretical breakthroughs in mathematics, statistics, and computer science. We will briefly address these methods from methodological and mathematical perspectives and categorize them into three types of approaches: data fusion (a narrowed definition as compared to the general data fusion concept), model fusion, and mixed fusion. We will demonstrate at least one typical example in each specific category to exemplify the characteristics, principles, and applications of the methods in general, as well as discuss the gaps and potential issues for future studies.
- Baldwin, E., Han, J., Luo, W., Zhou, J., An, L., Liu, J., Zhang, H., & Li, H. (2020). On fusion methods for knowledge discovery from multi-omics datasets. Computational and structural biotechnology journal, 18, 509–517. doi:https://doi.org/10.1016/j.csbj.2020.02.011
- Li, Z., Yu, D., Liu, J., & Hu, Q. (2020). Higher-order normal approximation approach for highly reliable system assessment. IISE Transactions, 52(5), 555-567.
- Minaeian, S., Liu, J., & Son, Y. (2018). Effective and Efficient Detection of Moving Targets From a UAV's Camera. IEEE TRANSACTIONS ON INTELLIGENT TRANSPORTATION SYSTEMS, 19(2), 497-506.
- Liu, J., Han, J., & Li, M. (2017). Bayesian nonparametric modeling of heterogeneous time-to-event data with an unknown number of sub-populations. IISE Transactions, 49(5), 481-492. doi:10.1080/0740817x.2016.1234732
- Li, M., & Liu, J. (2016). Bayesian hazard modeling based on lifetime data with latent heterogeneity. RELIABILITY ENGINEERING & SYSTEM SAFETY, 145, 183-189.
- Minaeian, S., Liu, J., & Son, Y. (2016). Vision-Based Target Detection and Localization via a Team of Cooperative UAV and UGVs. IEEE TRANSACTIONS ON SYSTEMS MAN CYBERNETICS-SYSTEMS, 46(7), 1005-1016.
- Wang, L., Hu, Q., & Liu, J. (2016). Software reliability growth modeling and analysis with dual fault detection and correction processes. IIE TRANSACTIONS, 48(4), 359-370.
- Jung, D., Kang, D., Liu, J., & Lansey, K. (2015). Improving the rapidity of responses to pipe burst in water distribution systems: a comparison of statistical process control methods. JOURNAL OF HYDROINFORMATICS, 17(2), 307-328.
- Kim, B. U., Goodman, D., Li, M., Liu, J., & Li, J. (2015). Improved Reliability-Based Decision Support Methodology Applicable in System-Level Failure Diagnosis and Prognosis (vol 50, pg 2630, 2014). IEEE TRANSACTIONS ON AEROSPACE AND ELECTRONIC SYSTEMS, 51(3), 2519-2524.
- Mingyang, L. i., Liu, J., Jing, L. i., & Kim, B. U. (2014). Bayesian modeling of multi-state hierarchical systems with multi-level information aggregation. Reliability Engineering and System Safety, 124, 158-164.More infoAbstract: Reliability modeling of multi-state hierarchical systems is challenging because of the complex system structures and imbalanced reliability information available at different system levels. This paper proposes a Bayesian multi-level information aggregation approach to model the reliability of multi-level hierarchical systems by utilizing all available reliability information throughout the system. Cascading failure dependency among components and/or sub-systems at the same level is explicitly considered. The proposed methodology can significantly improve the accuracy of system-level reliability modeling. A case study demonstrates the effectiveness of the proposed methodology. © 2013 Elsevier Ltd.
- Mingyang, L. i., Qingpei, H. u., & Liu, J. (2014). Proportional hazard modeling for hierarchical systems with multi-level information aggregation. IIE Transactions (Institute of Industrial Engineers), 46(2), 149-163.More infoAbstract: Reliability modeling of hierarchical systems is crucial for their health management in many mission-critical industries. Conventional statistical modeling methodologies are constrained by the limited availability of reliability test data, especially when the system-level reliability tests of such systems are expensive and/or time-consuming. This article presents a semi-parametric approach to modeling system-level reliability by systematically and explicitly aggregating lower-level information of system elements; i.e., components and/or subsystems. An innovative Bayesian inference framework is proposed to implement information aggregation based on the known multi-level structure of hierarchical systems and interaction relationships among their composing elements. Numerical case study results demonstrate the effectiveness of the proposed method. ©"IIE".
- Abellán, J. V., & Liu, J. (2013). Variation propagation modelling for multi-station machining processes with fixtures based on locating surfaces. International Journal of Production Research, 51(15), 4667-4681.More infoAbstract: Modelling the dimensional variation propagation in multi-station machining processes (MMPs) has been studied intensively in the past decade to understand and reduce the variation of product quality characteristics. Among others, the Stream-of-Variation (SoV) model has been successfully applied in a variety of applications, such as fault diagnosis, process planning and process-oriented tolerancing. However, the current SoV model is limited to the MMPs where only fixtures with punctual locators are applied. Other types of fixtures, such as those based on locating surfaces, have not been investigated. In this paper, the derivation of the SoV model is extended to model the effect of fixture-and datum-induced variations when fixtures with locating surfaces are applied. Due to the hyperstatic nature of these fixtures, different workholding configurations can be adopted. This will increase the dimension of the SoV model exponentially and thus may make the model-based part quality prediction extremely complex. This paper presents a method of reducing the complexity of the SoV model when fixtures based on locating surfaces are applied and evaluates the worst-case approach of the resulting part quality. © 2013 Taylor and Francis Group, LLC.
- Abellán-Nebot, J. V., Liu, J., & Subirón, F. R. (2013). Process-oriented tolerancing using the extended stream of variation model. Computers in Industry, 64(5), 485-498.More infoAbstract: Current works on process-oriented tolerancing for multi-station manufacturing processes (MMPs) have been mainly focused on allocating fixture tolerances to ensure part quality specifications at a minimum manufacturing cost. Some works have also included fixture maintenance policies into the tolerance allocation problem since they are related to both manufacturing cost and final part quality. However, there is a lack of incorporation of other factors that lead to increase of manufacturing cost and degrade of product quality, such as cutting-tool wear and machine-tool thermal state. The allocation of the admissible values of these process variables may be critical due to their impact on cutting-tool replacement and quality loss costs. In this paper, the process-oriented tolerancing is expanded based on the recently developed extended stream of variation (SoV) model which explicitly represents the influence of machining process variables in the variation propagation along MMPs. In addition, the probability distribution functions (pdf) for some machining process variables are analyzed, and a procedure to derive part quality constraints according to GD&T specifications is also shown. With this modeling capability extension, a complete process-oriented tolerancing can be conducted, reaching a real minimum manufacturing cost. In order to demonstrate the advantage of the proposed methodology over a conventional method, a case study is analyzed in detail. © 2013 Elsevier B.V.
- Chen, H., Cheng, H., Liu, J., Zhang, B., Zhang, G., & Fuhlbrigge, T. (2013). Performance improvement for high accuracy assembly process in manufacturing automation. IEEE International Conference on Automation Science and Engineering, 540-545.More infoAbstract: High precision assembly processes using industrial robots require the process parameters to be tuned in order to achieve desired performance such as cycle time and First Time Through (FTT) rate. Some researchers proposed methods such as Design-of-Experiment (DOE) to obtain optimal parameters. However, these methods only discuss how to find the optimal parameters if the part and/or workpiece location errors are in a certain range. In real assembly processes, the part and/or workpiece location errors could be different from batch to batch. Therefore the existing methods have some limitations. In this paper, the parameter optimization process based on DOE with different part and/or workpiece location errors are investigated. Experimental results demonstrate that the optimal parameters for different initial conditions are different and larger initial part and/or workpiece location errors will cause longer cycle time. Therefore, in order to improve the assembly process performance, the initial part and/or workpiece location errors should be compensated first and the optimal parameters in production should be changed once the initial tool position is compensated. An online parameter optimization method is also proposed. Experiments were performed to validate the proposed method and the results show that the proposed method is very promising in reducing the cycle time in assembly processes. © 2013 IEEE.
- Chen, H., Cheng, H., Zhang, B., Wang, J., Fuhlbrigge, T., & Liu, J. (2013). Semiautonomous industrial mobile manipulation for industrial applications. 2013 IEEE International Conference on Cyber Technology in Automation, Control and Intelligent Systems, IEEE-CYBER 2013, 361-366.More infoAbstract: The mobile industrial manipulator(MIM) which combines the advantages of both the mobile robot and industrial robot and owns powerful mobility, flexibility and functionality will be the next generation of robots. However fully autonomous MIM performing various tasks in unstructured or semi-structured environment is very challenging in autonomous localization & navigation, object identification, control and coordination. However, there is a demand in industry for MIMs to be deployed in hazardous, dangerous and/or remotely environments. Therefore, a semi-autonomous MIM is investigated to perform industrial operations. An MIM prototype using a mobile platform and an industrial robot is developed. Manual and autonomous methods are proposed and implemented to control the prototype manually and autonomously. Experiments using the prototype were performed. Experimental results demonstrate that the robot can run autonomously if there is no ambiguity and the operators can interrupt the autonomous mode at any time if needed. The developed prototype and proposed methods have big potential in industrial applications. © 2013 IEEE.
- Jung, D., Kang, D., Liu, J., & Lansey, K. (2013). Improving resilience of water distribution system through burst detection. World Environmental and Water Resources Congress 2013: Showcasing the Future - Proceedings of the 2013 Congress, 768-776.More infoAbstract: A burst in a water distribution system (WDS) results from the pipe rupture resulting in loss of water and disruptions to customer service. Rapid burst detection increases resilience by reducing the time to identify that a break has occurred to isolate the failed pipes and to recover service. This study compares three univariate and three multivariate Statistical Process Control (SPC) methods with respect to their detectability. The univariate SPC methods are the Western Electric Company (WEC) rules, the Cumulative Sum method (CUSUM), and the Exponentially Weighted Moving Average (EWMA) method, while three multivariate methods are Hotelling T2 method and the multivariate versions of CUSUM and EWMA. A method's detectability is determined by detection efficiency and effectiveness. To test the methods, nodal pressures and pipe flow rates were generated from a real hydraulic model and detectability is computed for flow and pressure meters in alternative configurations. © 2013 American Society of Civil Engineers.
- Liu, J., & Jin, J. (2013). Diagnosing Multistage Manufacturing Processes With Engineering-Driven Factor Analysis Considering Sampling Uncertainty. Journal of Manufacturing Science and Engineering, 135(4). doi:10.1115/1.4024661
- Liu, J., & Jin, J. (2013). Diagnosing multistage manufacturing processes with engineering-driven factor analysis considering sampling uncertainty. Journal of Manufacturing Science and Engineering, Transactions of the ASME, 135(4).More infoAbstract: A new engineering-driven factor analysis (EDFA) method has been developed to assist the variation source identification for multistage manufacturing processes (MMPs). The proposed method investigated how to fully utilize qualitative engineering knowledge of the spatial variation patterns to guide the factor rotation. It is shown that ideal identification can be achieved by matching the rotated factor loading vectors with the qualitative indicator vectors (IV) that are defined according to spatial variation patterns based on the design constraints. However, the random sampling variability may significantly affect the estimation of the rotated factor loading vectors, leading to the deviations from their true values. These deviations may change the matching results and cause misidentification of the actual variation sources. By using implicit differentiation approach, this paper derives the asymptotic distribution and the associated variance-covariance matrix of the rotated factor loading vectors. Therefore, by considering the effect of sample estimation variability, the variation sources identification problem is reformulated as an asymptotic statistical test of the hypothesized match between the rotated factor loading vectors and the indicator vectors. A real-world case study is provided to demonstrate the effectiveness of the proposed matching method and its robustness to the sample uncertainty. Copyright © 2013 by ASME.
- Wang, Z., Dessureault, S., & Liu, J. (2013). Quality-driven workforce performance evaluation based on robust regression and ANOMR/ANOMRV chart. IIE Transactions (Institute of Industrial Engineers), 45(6), 644-657.More infoAbstract: The integration of quality improvement and manufacturing system management has emerged as a promising research topic in recent years. Since operators performance variation can be reflected in product quality, workforce performance evaluation should be conducted with quality-based metrics to improve product quality as well as manufacturing system productivity. In this article, a methodology incorporating regression modeling and multiple comparisons is proposed to aid the performance evaluation. The effects of other impacting factors that contribute to operators performance variation are quantified with a robust zero-inflated Poisson regression model. The model coefficients are analyzed with multiple hypothesis tests to identify underperforming machines. Two statistical charts used in multiple comparisons are adopted for identifying underperforming operators. A case study with data from a real-world production system and a simulation experiment are presented to demonstrate the proposed methodology. © 2013 Copyright Taylor and Francis Group, LLC.
- Abellan-Nebot, J. V., Liu, J., & Subirón, F. R. (2012). Quality prediction and compensation in multi-station machining processes using sensor-based fixtures. Robotics and Computer-Integrated Manufacturing, 28(2), 208-219.More infoAbstract: New fixture technologies, such as sensor-based fixtures, will significantly improve part quality through cutting-tool path compensations in multi-station machining processes (MMPs). Successful application of sensor-based fixtures depends on the development of new variation reduction methodologies to predict part quality in MMPs and detect the critical machining stations whose critical manufacturing variations can be estimated by installing a suitable sensor-based fixture. In this paper, a methodology is proposed to facilitate the implementation of sensor-based fixtures in MMPs. This methodology involves three key steps: (1) an identification of station-induced variations; (2) a sensor placement optimization method for designing sensor-based fixtures; and (3) a compensability analysis. A case study is conducted to demonstrate the effectiveness of the methodology. © 2011 Elsevier Ltd. All rights reserved.
- Abellan-Nebot, J. V., Liu, J., Subirn, F. R., & Shi, J. (2012). State space modeling of variation propagation in multistation machining processes considering machining-induced variations. Journal of Manufacturing Science and Engineering, Transactions of the ASME, 134(2).More infoAbstract: In spite of the success of the stream of variation (SoV) approach to modeling variation propagation in multistation machining processes (MMPs), the absence of machining-induced variations could be an important factor that limits its application in accurate variation prediction. Such machining-induced variations are caused by geometric-thermal effects, cutting-tool wear, etc. In this paper, a generic framework for machining-induced variation representation based on differential motion vectors is presented. Based on this representation framework, machining-induced variations can be explicitly incorporated in the SoV model. An experimentation is designed and implemented to estimate the model coefficients related to spindle thermal-induced variations and cutting-tool wear-induced variations. The proposed model is compared with the conventional SoV model resulting in an average improvement on quality prediction of 67%. This result verifies the advantage of the proposed extended SoV model. The application of the new model can significantly extend the capability of SoV-model-based methodologies in solving more complex quality improvement problems for MMPs, such as process diagnosis and process tolerance allocation, etc. © 2012 American Society of Mechanical Engineers.
- Abellan-Nebot, J. V., Liu, J., & Subiron, F. R. (2011). Design of multi-station manufacturing processes by integrating the stream-of-variation model and shop-floor data. Journal of Manufacturing Systems, 30(2), 70-82.More infoAbstract: Process design has been intensively studied to reduce dimensional variability of products produced in multi-station manufacturing processes (MMPs). Most of the existing studies focus on predicting variation propagation and evaluating process robustness. However, these studies overlook the potential use of historical shop-floor quality data of existing MMPs in order to extract the actual manufacturing operation capabilities from each station, and then, to evaluate more accurately the expected dimensional variability of new candidate process plans. This paper proposes a methodology to improve process plan selection based on three components: (i) based on historical shop floor data, an inference on the process capabilities of the stations in an existing MMP, which will be used to produce the new product; (ii) a sensitivity analysis of candidate process plans to identify critical fixtures and manufacturing stations/operations; and (iii) an optimal selection of candidate process plans. A case study is presented to demonstrate the effectiveness of the methodology. © 2011 The Society of Manufacturing Engineers. Published by Elsevier Ltd. All rights reserved.
- Kim, B. U., Vohnout, S., Mikkola, E., Mingyang, L. i., & Liu, J. (2011). Causal analysis for troubleshooting and decision support system. 2011 IEEE International Conference on Prognostics and Health Management, PHM 2011 - Conference Proceedings.More infoAbstract: Troubleshooting and decision support system with reasoning is an active research topic, and causal analysis for complex component interactions in complex systems has remained a critical challenge to be overcome. We developed an innovative, constraint-based causal analysis to better detect, isolate, and troubleshoot complex systems. The feasibility of the causal Bayesian network (CBN) approach has been proven with implementation using test data acquired from electromechanical actuator (EMA) systems. The validation step is facilitated by comparing the trained CBN with original structure and shows the flexibility and extensibility of our solutions. This causal analysis processing in integrated system health management (ISHM) will enable enhancements in flight safety and condition-based maintenance (CBM) by increasing availability and mission-effectiveness while reducing maintenance costs. © 2011 IEEE.
- Liu, J., Jing, L. i., & Kim, B. U. (2011). Bayesian reliability modeling of multi-level system with interdependent subsystems and components. Proceedings of 2011 IEEE International Conference on Intelligence and Security Informatics, ISI 2011, 252-257.More infoAbstract: Modeling the reliability of multi-level engineering systems is critically important yet technically challenging. Existing methods have limited consideration of the failure interdependency caused by the interactions among subsystems and components. In this paper, a new Bayesian Network (BN) representation of system structure and component interactions is proposed. Based on the BN representation, a Bayesian framework is developed to fuse the information from different levels of a system for modeling the reliability of the components, subsystems and the system as a whole. A case study is conducted to demonstrate the effectiveness of the proposed methodology. © 2011 IEEE.
- Liu, J. (2010). Variation reduction for multistage manufacturing processes: A comparison survey of statistical-process-control vs stream-of-variation methodologies. Quality and Reliability Engineering International, 26(7), 645-661.More infoAbstract: The performance of Multistage Manufacturing Processes (MMPs) can be measured by quality, productivity and cost, which are inversely related to the variation of key product characteristics (KPCs). Therefore, it is crucial to reduce KPCs' variations by not only detecting the changes of process parameters, but also identifying the variation sources and eliminating them with corrective actions. Recent developments in the Stream-of-Variation (SoV) and Statistical-Process-Control (SPC) methodologies significantly improve the variation reduction for MMPs. This paper provides a review on the reported methodologies by comparing these two categories of methodologies in terms of their variation propagation modeling, process monitoring and diagnostic capability. With an illustrative case study, it is concluded that the recent advancements of SoV and SPC methodologies significantly improve the effectiveness of variation reduction. The discussion on the drawbacks of both methodologies also suggests the future research directions. © 2010 John Wiley & Sons, Ltd.
- Liu, J., Jin, J., & Shi, J. (2010). State space modeling for 3-D variation propagation in rigid-body multistage assembly processes. IEEE Transactions on Automation Science and Engineering, 7(2), 274-290.More infoAbstract: Dimensional variation propagation modeling is a critical enabling technique for product quality variation reduction in a multistage assembly process (MAP). However, the complex inter-stage correlations make the modeling extremely difficult. This paper aims to improve the existing techniques by developing a generic state space approach to modeling 3-D variation propagation induced by various types of variation sources in general MAPs. A concept of differential motion vector (DMV) is adopted to represent deviations with respect to four types of coordinate systems and to formulate the variation propagation as a series of homogeneous transformation among different coordinate systems. Based on this representation and formulation strategy, a novel generic mechanism is proposed to model the effect of variations induced by part fabrication processes and a MAP. A case study on 3-D variation propagation in a panel fitting process is presented to demonstrate the modeling and analysis capability of the proposed methodology. © 2006 IEEE.
- Zhong, J., Liu, J., & Shi, J. (2010). Predictive control considering model uncertainty for variation reduction in multistage assembly processes. IEEE Transactions on Automation Science and Engineering, 7(4), 724-735.More infoAbstract: Active control for dimensional variation reduction in multistage assembly processes (MAPs) is a challenging issue for quality assurance. It is desirable to implement a system-level control strategy to minimize the end-of-line product variance, which is propagated from upstream manufacturing stages. Research has been conducted to realize such objective, based on the variation propagation models derived from the nominal parameters of product and process design. However, due to the uncertainties induced by the significant changes of process parameters, such designated model will be different from that of the actual process, and will not precisely represent the actual physics of the process. This model discrepancy may lead to the performance deterioration of the controllers. This paper proposed a feed-forward MAP control strategy that explicitly takes into account the uncertainties of model coefficients. The case study demonstrates that, when the model uncertainties are significant, the controller derived from the proposed approach outperforms that derived without considering the model uncertainty. © 2010 IEEE.
- Abellán-Nebot, J., Liu, J., & Romero, F. (2009). Limitations of the current state space modelling approach in multistage machining processes due to operation variations. AIP Conference Proceedings, 1181, 231-243.More infoAbstract: The State Space modelling approach has been recently proposed as an engineering-driven technique for part quality prediction in Multistage Machining Processes (MMP). Current State Space models incorporate fixture and datum variations in the multi-stage variation propagation, without explicitly considering common operation variations such as machine-tool thermal distortions, cutting-tool wear, cutting-tool deflections, etc. This paper shows the limitations of the current State Space model through an experimental case study where the effect of the spindle thermal expansion, cutting-tool flank wear and locator errors are introduced. The paper also discusses the extension of the current State Space model to include operation variations and its potential benefits. © 2009 American Institute of Physics.
- Liu, J., Shi, J., & Jack, H. u. (2009). Quality-assured setup planning based on the stream-of-variation model for multi-stage machining processes. IIE Transactions (Institute of Industrial Engineers), 41(4), 323-334.More infoAbstract: Setup planning is a set of activities used to arrange manufacturing features into an appropriate sequence for processing. It has significant impact on the product quality, which is often measured in terms of dimensional variation in key product characteristics. Current approaches to setup planning are experience-based and tend to be conservative due to the selection of unnecessarily precise machines and fixtures to ensure final product quality. This is especially true in multi-stage machining processes (MMPs) since it is difficult to predict variation propagation and its impact on the quality of the final product. In this paper, a methodology is proposed to realize cost-effective, quality-assured setup planning for MMPs. Setup planning is formulated as an optimization problem based on quantitative evaluation of variation propagations. The optimal setup plan minimizes the cost related to process precision and satisfies the quality specifications. The proposed approach can significantly improve the effectiveness as well as the efficiency of the setup planning for MMPs.
- Liu, J., Shi, J., & Hu, S. J. (2008). Engineering-driven factor analysis for variation source identification in multistage manufacturing processes. Journal of Manufacturing Science and Engineering, Transactions of the ASME, 130(4), 0410091-04100910.More infoAbstract: Variation source identification is an important task of quality assurance in multistage manufacturing processes (MMPs). However, existing approaches, including the quantitative engineering-model-based methods and the data-driven methods, provide limited capabilities in variation source identification. This paper proposes a new methodology that does not depend on accurate quantitative engineering models. Instead, engineering domain knowledge about the interactions between potential variation sources and product quality variables is represented as qualitative indicator vectors. These indicator vectors guide the rotation of the factor loading vectors that are derived from factor analysis of the multivariate measurement data. Based on this engineering-driven factor analysis, a procedure is presented to identify multiple variation sources that are present in a MMP. The effectiveness of the proposed methodology is demonstrated in a case study of a three-stage assembly process. Copyright © 2008 by ASME.
- Liu, J., Shi, J., & Hu, S. J. (2006). Quality assured setup planning based on the stream-of-variation model for multi-stage machining processes. Proceedings of the International Conference on Manufacturing Science and Engineering, 2006.More infoAbstract: Setup planning is a set of activities to arrange manufacturing features into an appropriate sequence for processing. As such, setup planning can significantly impact the product quality in terms of dimensional variation in the Key Product Characteristics (KPC's). Current approaches in setup planning are experience-based and tend to be conservative by selecting unnecessarily precise machines and fixtures to ensure final product quality. This is especially true in multi-stage manufacturing processes because it has been difficult to predict the variation propagation and its impact on KPC quality. In this paper, a new methodology is proposed to realize cost-effective, quality ensured setup planning for multi-stage manufacturing processes. Setup planning is formulated as an optimization problem based on quantitative evaluation with the Stream-of-Variation (SoV) models. The optimal setup plan minimizes the cost related to process precision and satisfies the quality specifications. The effectiveness of the proposed approach is demonstrated through setup planning for a multi-stage machining process. Copyright © 2006 by ASME.
- Liu, J., Wang, W., Yip-Hoi, D. M., & Tang, L. (2006). Optimal part family and production module planning for reconfigurable manufacturing systems. Proceedings of the International Conference on Manufacturing Science and Engineering, 2006.More infoAbstract: Manufactures are adopting Reconfigurable Manufacturing Systems (RMS) to better cope with frequently changing market conditions, which place tremendous demands on a system's flexibility as well as its cost-effectiveness. Considerable efforts have been devoted to the development of necessary tools for the system level design and performance improvement, resulting in approaches to designing a single RMS. In this paper, a methodology for cost-effective reconfiguration planning for multi-module-multi-product RMS's that best reflect the market demand changes is proposed. Formulated as an optimization procedure, reconfiguration planning is defined as the best reallocation of part families to production modules in an RMS and the best rebalancing of the whole system and each individual module to achieve minimum related cost and simultaneously satisfy the market demand. A Genetic Algorithm (GA) approach is proposed to overcome the computational difficulties caused by the problem complexity. Effectiveness of the proposed methodology is demonstrated with a case study. Copyright © 2006 by ASME.
- Liu, J., Jing, L. i., & Shi, J. (2005). Engineering driven cause-effect modeling and statistical analysis for multi-operational machining process diagnosis. Transactions of the North American Manufacturing Research Institute of SME, 33, 65-72.More infoAbstract: Process fault identification for product quality improvement is a critical issue in both design and manufacturing, especially for multistage manufacturing processes. In this paper, an integrated approach is proposed to develop cause-effect models from engineering knowledge and to conduct associated statistical analysis of the measurement data. First, a cause-effect diagram and predicted symptom vectors (PSV) are formulated to recognize the cause-effect relationship between process variables and product qualities. Then factor analysis and factor rotating technique are employed to extract the symptoms reflected from measurement data. Finally the potential process faults are identified by comparing predicted symptoms and extracted symptoms. A case study is conducted to demonstrate the effectiveness of the proposed methodology.
Proceedings Publications
- Pan, F., Zhang, Y., Head, L., Liu, J., Elli, M., & Alvarez, I. (2022, December). Quantifying Error Propagation in Multi-Stage Perception System of Autonomous Vehicles via Physics-Based Simulation. In 2022 Winter Simulation Conference.
- Zhang, Y., Liu, J., Lansey, K. E., Lansey, K. E., Zhang, Y., & Liu, J. (2020, December). Detecting Burst in Water Distribution System via Penalized Functional Decomposition. In IEEE International Conference on Industrial Engineering and Engineering Management, 205-209.
- Phillips, L. R., Beverly, H., Zhang, Y., May, J., Lacasse, C. L., Martin Plank, L. M., Liu, J., Jessica, P., Loque, K., Peterson, R., Shea, K. D., & Crist, J. D. (2019, Fall). Sensor Technology: Mexican American Caregiving Families. In Council for the Advancement of Nursing Science.
- Phillips, L. R., Liu, J., Beverly, H., Martin Plank, L. M., Lacasse, C. L., Rachel, P., Crist, J. D., & Shea, K. D. (2019, November). Symposium The Tipping Point Study, Digital Detection and Decision Support for Older Adults and Families. In Gerontological Society of America.
Poster Presentations
- Liu, J., Son, Y., Yuan, Y., Minaeian, S., & Lee, S. (2017, September). DDDAMS-based Border Surveillance and Crowd Control via Aerostats, UAVs, and Ground Sensors. InfoSymbiotic:DDDAS17. University of Arizona.More infoFAC 2017 conference presentation/paper submission.