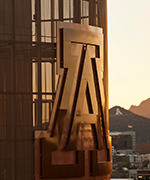
Yue Niu
- Associate Professor, Mathematics
- Associate Professor, Statistics-GIDP
- Member of the Graduate Faculty
Contact
- (520) 621-1961
- Environment and Natural Res. 2, Rm. S322
- Tucson, AZ 85719
- yueniu@arizona.edu
Degrees
- Ph.D. Operations Research and Financial Engineering
- Princeton University, Princeton, New Jersey, United States
Awards
- NSF-AWM Travel Grant
- National Science Foundation and Association for Women in Mathematics, Spring 2013
Interests
No activities entered.
Courses
2024-25 Courses
-
Intro to Statistical Computing
DATA 375 (Spring 2025) -
Capstone: Stats/Data Science
DATA 498A (Fall 2024) -
Theory of Probability
MATH 564 (Fall 2024) -
Theory of Probability
STAT 564 (Fall 2024)
2023-24 Courses
-
Independent Study
STAT 599 (Summer I 2024) -
Capstone: Stats/Data Science
DATA 498A (Spring 2024) -
Intro to Statistical Computing
DATA 375 (Spring 2024) -
Independent Study
DATA 499 (Fall 2023) -
Internship
MATH 593 (Fall 2023) -
Theory of Probability
MATH 564 (Fall 2023) -
Theory of Probability
STAT 564 (Fall 2023)
2022-23 Courses
-
Capstone: Stats/Data Science
DATA 498A (Spring 2023) -
Intro to Statistical Computing
DATA 375 (Spring 2023) -
Theory of Probability
MATH 564 (Fall 2022) -
Theory of Probability
STAT 564 (Fall 2022)
2021-22 Courses
-
Theory of Statistics
MATH 466 (Spring 2022) -
Theory of Probability
MATH 564 (Fall 2021) -
Theory of Probability
STAT 564 (Fall 2021)
2020-21 Courses
-
Intro to Statistical Computing
DATA 375 (Spring 2021) -
Intro to Statistical Computing
DATA 375 (Fall 2020)
2018-19 Courses
-
Theory of Statistics
MATH 466 (Spring 2019) -
Theory of Probability
MATH 564 (Fall 2018) -
Theory of Probability
STAT 564 (Fall 2018)
2017-18 Courses
-
Theory of Statistics
MATH 466 (Spring 2018) -
Theory of Probability
MATH 564 (Fall 2017) -
Theory of Probability
STAT 564 (Fall 2017)
2016-17 Courses
-
Theory of Statistics
MATH 466 (Spring 2017) -
Theory of Probability
MATH 564 (Fall 2016) -
Theory of Probability
STAT 564 (Fall 2016) -
Theory of Statistics
MATH 466 (Fall 2016) -
Thesis
STAT 910 (Fall 2016)
2015-16 Courses
-
Theory of Statistics
MATH 466 (Spring 2016)
Scholarly Contributions
Journals/Publications
- Xiao, H., Niu, Y., & Hao, N. (2023). Equivariant Variance Estimation for Multiple Change-point Model. Electronic Journal of Statistics, 17(2), 3811-3853. doi:10.1214/23-EJS2190
- Wang, Y., Niu, Y., Wang, Z., Vashisth, T., Li, J., Madden, R., & Livingston, T. S. (2022). Nontargeted metabolomics-based multiple machine learning modeling boosts early accurate detection for citrus Huanglongbing. Horticulture Research, 9. doi:10.1093/hr/uhac145
- Zhang, H., Xiao, F., Niu, Y. S., & Hao, N. (2021). A super scalable algorithm for short segment detection.. Statistics in biosciences, 13(1), 18-33. doi:10.1007/s12561-020-09278-zMore infoIn many applications such as copy number variant (CNV) detection, the goal is to identify short segments on which the observations have different means or medians from the background. Those segments are usually short and hidden in a long sequence, and hence are very challenging to find. We study a super scalable short segment (4S) detection algorithm in this paper. This nonparametric method clusters the locations where the observations exceed a threshold for segment detection. It is computationally efficient and does not rely on Gaussian noise assumption. Moreover, we develop a framework to assign significance levels for detected segments. We demonstrate the advantages of our proposed method by theoretical, simulation, and real data studies.
- Zhang, H., Hao, N., Xiao, F., Niu, Y., Xiao, F., Niu, Y., Zhang, H., & Hao, N. (2020). A super scalable algorithm for segment detection. Statistics in Biosciences.
- Zhang, H., Xiao, F., Amos, C. I., Luo, X., Cai, G., Hao, N., Xiao, X., Niu, Y., Xiao, X., Niu, Y., Cai, G., Hao, N., Amos, C. I., Luo, X., Zhang, H., & Xiao, F. (2019). An Accurate and Powerful Method for Copy Number Variation Detection. Bioinformatics, 35(17), 2891-2898. doi:https://doi.org/10.1093/bioinformatics/bty1041
- Niu, Y. S., Suh, J. H., Wang, Z., Gmitter, F. G., & Wang, Y. (2018). Metabolic Analysis Reveals Altered Long-Chain Fatty Acid Metabolism in the Host by Huanglongbing Disease. Journal of Agricultural and Food Chemistry, 66(5), 1296-1304. doi:10.1021/acs.jafc.7b05273
- Niu, Y., Hao, N., & Dong, B. (2018). A New Reduced-Rank Linear Discriminant Analysis Method and Its Applications. Statistica Sinica, 28, 189-202. doi:https://doi.org/10.5705/ss.202015.0387
- Niu, Y., Hao, N., & Zhang, H. (2018). Interaction Screening by Partial Correlation. Statistics and Its Interface, 11(2), 317-325. doi:http://dx.doi.org/10.4310/SII.2018.v11.n2.a9
- Niu, Y., Suh, J. H., Wang, Z., Gmitter Jr., F., & Wang, Y. (2018). Metabolic analysis reveals altered long-chain fatty acid metabolism in the host by Huanglongbing disease. Journal of Agricultural and Food Chemistry, 66, 1296-1304. doi:10.1021/acs.jafc.7b05273
- Hao, N., Niu, Y., Xiao, F., Xu, Y., Jin, Z., & Zhang, H. (2017). modSaRa: a computationally efficient R package for CNV identification. Bioinformatics, 33(15), 2384-2385. doi:10.1093/bioinformatics/btx212
- Suh, J. H., Niu, Y. S., Hung, W., Ho, C., & Wang, Y. (2017). Lipidomic analysis for carbonyl species derived from fish oil using liquid chromatography-tandem mass spectrometry. Talanta, 168, 31-42. doi:http://dx.doi.org/10.1016/j.talanta.2017.03.023
- Xiao, F., Niu, Y., Hao, N., Xu, Y., Jin, Z., & Zhang, H. (2017). modSaRa: a computationally efficient R package for CNV identification. Bioinformatics.
- Zhang, H., Niu, Y., Hao, N., Hao, N., Zhang, H., & Niu, Y. (2016). Multiple Change-Point Detection, a Selective Overview. Statistical Science, 31(4), 611-623.
- Hao, N., Niu, Y. S., & Zhang, H. (2013). Multiple change-point detection via a screening and ranking algorithm. Statistica Sinica, 23(4), 1553-1572.More infoAbstract: Let Y1; Yn be a sequence whose underlying mean is a step function with an unknown number of the steps and unknown change points. The detection of the change points, namely the positions where the mean changes, is an important problem in such fields as engineering, economics, climatology and bioscience. This problem has attracted a lot of attention in statistics, and a variety of solutions have been proposed and implemented. However, there is scant literature on the theoretical properties of those algorithms. Here, we investigate a recently developed algorithm called the Screening and Ranking algorithm (SaRa). We characterize the theoretical properties of SaRa and show its superiority over other commonly used algorithms. In particular, we develop a false discovery rate approach to the multiple change-point problem and show a strong sure coverage property for the SaRa.
- Niu, Y. S., & Zhang, H. (2012). The screening and ranking algorithm to detect DNA copy number variations. Annals of Applied Statistics, 6(3), 1306-1326.More infoAbstract: DNA Copy number variation (CNV) has recently gained considerable interest as a source of genetic variation that likely influences phenotypic differences. Many statistical and computational methods have been proposed and applied to detect CNVs based on data that generated by genome analysis platforms. However, most algorithms are computationally intensive with complexity at least O(n 2), where n is the number of probes in the experiments. Moreover, the theoretical properties of those existing methods are not well understood. A faster and better characterized algorithm is desirable for the ultra high throughput data. In this study, we propose the Screening and Ranking algorithm (SaRa) which can detect CNVs fast and accurately with complexity down to O(n). In addition, we characterize theoretical properties and present numerical analysis for our algorithm. © Institute of Mathematical Statistics 2012.
- Zhang, H., & Niu, Y. S. (2012). THE SCREENING AND RANKING ALGORITHM TO DETECT DNA COPY NUMBER VARIATIONS.. The annals of applied statistics, 6(3), 1306-1326. doi:10.1214/12-aoas539suppMore infoDNA Copy number variation (CNV) has recently gained considerable interest as a source of genetic variation that likely influences phenotypic differences. Many statistical and computational methods have been proposed and applied to detect CNVs based on data that generated by genome analysis platforms. However, most algorithms are computationally intensive with complexity at least O(n2), where n is the number of probes in the experiments. Moreover, the theoretical properties of those existing methods are not well understood. A faster and better characterized algorithm is desirable for the ultra high throughput data. In this study, we propose the Screening and Ranking algorithm (SaRa) which can detect CNVs fast and accurately with complexity down to O(n). In addition, we characterize theoretical properties and present numerical analysis for our algorithm.
- Bailey-Wilson, J. E., Brennan, J. S., Bull, S. B., Culverhouse, R., Kim, Y., Jiang, Y., Jung, J., Qing, L. i., Lamina, C., Liu, Y., Mägi, R., Niu, Y. S., Simpson, C. L., Wang, L., Yilmaz, Y. E., Zhang, H., & Zhang, Z. (2011). Regression and data mining methods for analyses of multiple rare variants in the Genetic Analysis Workshop 17 mini-exome data. Genetic Epidemiology, 35(SUPPL. 1), S92-S100.More infoPMID: 22128066;PMCID: PMC3360949;Abstract: Group 14 of Genetic Analysis Workshop 17 examined several issues related to analysis of complex traits using DNA sequence data. These issues included novel methods for analyzing rare genetic variants in an aggregated manner (often termed collapsing rare variants), evaluation of various study designs to increase power to detect effects of rare variants, and the use of machine learning approaches to model highly complex heterogeneous traits. Various published and novel methods for analyzing traits with extreme locus and allelic heterogeneity were applied to the simulated quantitative and disease phenotypes. Overall, we conclude that power is (as expected) dependent on locus-specific heritability or contribution to disease risk, large samples will be required to detect rare causal variants with small effect sizes, extreme phenotype sampling designs may increase power for smaller laboratory costs, methods that allow joint analysis of multiple variants per gene or pathway are more powerful in general than analyses of individual rare variants, population-specific analyses can be optimal when different subpopulations harbor private causal mutations, and machine learning methods may be useful for selecting subsets of predictors for follow-up in the presence of extreme locus heterogeneity and large numbers of potential predictors. © 2011 Wiley Periodicals, Inc.
- Niu, Y. S., Hao, N., Niu, Y. S., Hao, N., & An, L. (2011). Detection of rare functional variants using group ISIS.. BMC proceedings, 5 Suppl 9(9), S108. doi:10.1186/1753-6561-5-s9-s108More infoGenome-wide association studies have been firmly established in investigations of the associations between common genetic variants and complex traits or diseases. However, a large portion of complex traits and diseases cannot be explained well by common variants. Detecting rare functional variants becomes a trend and a necessity. Because rare variants have such a small minor allele frequency (e.g.,
- Fan, J., Feng, Y., & Niu, Y. S. (2010). Nonparametric estimation of genewise variance for microarray data. Annals of Statistics, 38(5), 2723-2750.More infoAbstract: Estimation of genewise variance arises from two important applications in microarray data analysis: selecting significantly differentially expressed genes and validation tests for normalization of microarray data. We approach the problem by introducing a two-way nonparametric model, which is an extension of the famous Neyman-Scott model and is applicable beyond microarray data. The problem itself poses interesting challenges because thenumber of nuisance parameters is proportional to the sample size and it is not obvious how the variance function can be estimated when measurements are correlated. In such a high-dimensional nonparametric problem, we proposed two novel nonparametric estimators for genewise variance function and semiparametric estimators for measurement correlation, via solving a system of nonlinear equations. Their asymptotic normality is established. The finite sample property is demonstrated by simulation studies. The estimators also improve the power of the tests for detecting statistically differentially expressed genes. The methodology is illustrated by the data from microarray quality control (MAQC) project. © Institute of Mathematical Statistics, 2010.
- Niu, Y. S., Feng, Y., & Fan, J. (2010). NONPARAMETRIC ESTIMATION OF GENEWISE VARIANCE FOR MICROARRAY DATA.. Annals of statistics, 38(5), 2723-2750. doi:10.1214/10-aos802More infoEstimation of genewise variance arises from two important applications in microarray data analysis: selecting significantly differentially expressed genes and validation tests for normalization of microarray data. We approach the problem by introducing a two-way nonparametric model, which is an extension of the famous Neyman-Scott model and is applicable beyond microarray data. The problem itself poses interesting challenges because the number of nuisance parameters is proportional to the sample size and it is not obvious how the variance function can be estimated when measurements are correlated. In such a high-dimensional nonparametric problem, we proposed two novel nonparametric estimators for genewise variance function and semiparametric estimators for measurement correlation, via solving a system of nonlinear equations. Their asymptotic normality is established. The finite sample property is demonstrated by simulation studies. The estimators also improve the power of the tests for detecting statistically differentially expressed genes. The methodology is illustrated by the data from MicroArray Quality Control (MAQC) project.
- Fan, J., & Niu, Y. (2007). Selection and validation of normalization methods for c-DNA microarrays using within-array replications. Bioinformatics, 23(18), 2391-2398.More infoPMID: 17660210;Abstract: Motivation: Normalization of microarray data is essential for multiple-array analyses. Several normalization protocols have been proposed based on different biological or statistical assumptions. A fundamental problem arises whether they have effectively normalized arrays. In addition, for a given array, the question arises how to choose a method to most effectively normalize the microarray data. Results: We propose several techniques to compare the effectiveness of different normalization methods. We approach the problem by constructing statistics to test whether there are any systematic biases in the expression profiles among duplicated spots within an array. The test statistics involve estimating the genewise variances. This is accomplished by using several novel methods, including empirical Bayes methods for moderating the genewise variances and the smoothing methods for aggregating variance information. P-values are estimated based on a normal or χ approximation. With estimated P-values, we can choose a most appropriate method to normalize a specific array and assess the extent to which the systematic biases due to the variations of experimental conditions have been removed. The effectiveness and validity of the proposed methods are convincingly illustrated by a carefully designed simulation study. The method is further illustrated by an application to human placenta cDNAs comprising a large number of clones with replications, a customized microarray experiment carrying just a few hundred genes on the study of the molecular roles of Interferons on tumor, and the Agilent microarrays carrying tens of thousands of total RNA samples in the MAQC project on the study of reproducibility, sensitivity and specificity of the data. © The Author 2007. Published by Oxford University Press. All rights reserved.
Presentations
- Niu, Y. (2024). Detecting Epidemic Changes through Shifted Maximum Subarray Analysis. ICSA China. Wuhan, China.
- Niu, Y. (2024). Detecting Epidemic Changes through Shifted Maximum Subarray Analysis. IWSM International Workshop in Sequential Methodologies. Utah Valley University.
- Niu, Y. (2024). Detecting Epidemic Changes through Shifted Maximum Subarray Analysis. WNAR. Fort Collins, CO.
- Niu, Y. (2023). Inference for Gaussian Multiple Change-point Model via Bayesian Information Criterion. JSM 2023.
- Niu, Y. (2022). Equivariant Variance Estimation for Multiple Change-Point Model. 2022 International Symposium on Modern Data Science Application, Practice, and Theory. New Haven, CT.
- Niu, Y. (2022). Inference for Gaussian Multiple Change-point Model viaBayesian Information Criterion. ICSA 2022 Applied Statistics Symposium. Gainesville, FL.
- Niu, Y. (2022). Inference for Gaussian Multiple Change-point Model viaBayesian Information Criterion. ICSA 2022 China Conference. Virtual.
- Niu, Y. (2022). Inference for Gaussian Multiple Change-point Model viaBayesian Information Criterion. The Fifth ICSA-Canada Chapter Symposium. Banff, Canada.
- Niu, Y. (2021). A Super Scalable Algorithm for Short Segment Detection. Department of Biostatistics and Medical Informatics Seminar. Online: School of Medicine and Public Health, University of Wisconsin- Madison.
- Niu, Y. (2021). A Super Scalable Algorithm for Short Segment Detection. WNAR 2021 Conference. Online.
- Niu, Y. (2021). A super scalable algorithm for segment detection. ENAR 2021 Spring Meeting. Online.
- Niu, Y. (2021). A super scalable algorithm for segment detection. Machine Learning Day, New College. Online: Arizona State University.
- Niu, Y. (2019, July). Variance estimation for change-point model. 2019 ICSA China Conference. Tianjin, China.
- Niu, Y. (2019, June). Variance estimation for change-point model. Ecosta 2019. Taiwan, China.
- Niu, Y. (2019, March). A super scalable algorithm for short segment detection. Statistics and Data Science Seminar. Chicago, IL: Dept of Mathematics, Statistics and Computer Science, UIC.
- Niu, Y. (2019, May). A Super Scalable Algorithm for Short Segment Detection. 2019 Hangzhou International Conference on Frontiers of Data Science. Hangzhou, China.
- Niu, Y. (2018, July). A super scalable algorithm for short segment detection. 2018 ICSA China Conference with the Focus on Data Science. Qingdao, China: ICSA.
- Niu, Y. (2018, July). Reduced-Rank Linear Discriminant Analysis. MJU First International workshop on data science. Minjiang University, Fuzhou, China: Minjiang University.
- Niu, Y. (2018, June). A super scalable algorithm for short segment detection. EcoSta 2018. Hong Kong, China.
- Niu, Y. (2018, June). A super scalable algorithm for short segment detection. Statistics Colloquium. Nankai University, Tianjin, China.: Institute of Statistics.
- Niu, Y. (2018, June). Reduced-Rank Linear Discriminant Analysis. Statistics Colloquium. Shanghai University of Finance and Economics, Shanghai, China.: School of Statistics and Management.
- Niu, Y. (2016, June). Reduced-Rank Linear Discriminant Analysis. 2016 ICSA Applied Statistics Symposium. Atlanta, GA: ICSA.
- Niu, Y. (2015, July). Reduced Ranked Linear Discriminant Analysis. Invited seminar talk. Tianjin, China: Nankai University, Institute of Statistics.
- Niu, Y. (2015, June). Screening Interaction Effects in Quadratic Regression Model. ISBS/DIA Symposium on Biopharmaceutical Statistics. Beijing, China: The International Society for Biopharmaceutical Statistics (ISBS).
- Niu, Y. (2015, May). Reduced Ranked Linear Discriminant Analysis. Invited seminar talk. Statistics Department: Oregon State University.
Creative Productions
- Xiao, F., Luo, X., Hao, N., Niu, Y., Xiao, X., Cai, G., Amos, C. I., & Zhang, H. (2018. R Package: modSaRa2. https://publichealth.yale.edu/c2s2/software/modSaRa2/.