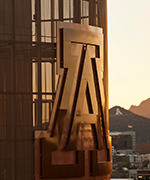
Janet Meiling Roveda
- Professor, Electrical and Computer Engineering
- Professor, Biomedical Engineering
- Professor, BIO5 Institute
- Member of the Graduate Faculty
- Professor, Nursing
Contact
- (520) 621-6182
- Electrical & Computer Engr, Rm. 256
- Tucson, AZ 85721
- wml@ece.arizona.edu
Biography
Janet M. Wang-Roveda is a Professor in the Department of Electrical and Computer Engineering at the University of Arizona in Tucson. She received her M.S. and Ph.D. degrees in Electrical Engineering and Computer Sciences from the University of California, Berkeley in 1998 and 2000, respectively. She was a recipient of the NSF career award and the Presidential Early Achievement Award for Science and Engineering at White House in 2005 and 2006, respectively. She was the recipient of the 2008 R. Newton Graduate Research Award from the EDA community, and the 2007 USS University of Arizona Outstanding Achievement Award. She received the best paper award in journal of clean energy in 2013, ISQED 2010 as well as best paper nominations in ASPDAC 2010, ICCAD 2007, and ISQED 2005. Her primary research interests focus on robust VLSI circuit design, biomedical instrument design, Smart grid, VLSI circuit modeling/design and analysis, and low power multi-core system design. She has over 120 publications. |
Awards
- ACABI Fellow
- October 2017 to October 2018, Fall 2018
- ICCD Best Paper Award
- October 2018, Fall 2018
- Graduate Student Interface Award
- The University of Arizona, Summer 2017
- Da Vinci fellow
- College of Engineering, Univ. of Arizona, Spring 2017
- TRIF Link Award
- Univ. of Arizona, Fall 2016
- Featured on Fox news Tucson
- Fox News, Fall 2015
- Featured on UA news
- UA News & ARIZONA Engineer Online, Fall 2015
- BIO5 fellowship
- Fall 2014
- Best paper award
- Journal of Clean Energy Technologies, Summer 2013
Interests
No activities entered.
Courses
2024-25 Courses
-
Analog Integrated Ckts
ECE 450 (Spring 2025) -
Analog Integrated Ckts
ECE 550 (Spring 2025) -
Dissertation
ECE 920 (Spring 2025) -
Research
ECE 900 (Spring 2025) -
Dissertation
ECE 920 (Fall 2024)
2023-24 Courses
-
Dissertation
ECE 920 (Spring 2024) -
Dissertation
ECE 920 (Fall 2023)
2022-23 Courses
-
Digital Vlsi Sys Design
ECE 407 (Spring 2023) -
Digital Vlsi Sys Design
ECE 507 (Spring 2023) -
Dissertation
BME 920 (Spring 2023) -
Dissertation
ECE 920 (Spring 2023) -
Research
ECE 900 (Spring 2023) -
Dissertation
BME 920 (Fall 2022) -
Dissertation
ECE 920 (Fall 2022) -
Internship
ECE 593 (Fall 2022)
2021-22 Courses
-
Internship
ECE 593 (Summer I 2022) -
Digital Vlsi Sys Design
ECE 407 (Spring 2022) -
Digital Vlsi Sys Design
ECE 507 (Spring 2022) -
Dissertation
BME 920 (Spring 2022) -
Dissertation
ECE 920 (Spring 2022) -
Dissertation
BME 920 (Fall 2021) -
Dissertation
ECE 920 (Fall 2021)
2020-21 Courses
-
Digital Vlsi Sys Design
ECE 407 (Spring 2021) -
Digital Vlsi Sys Design
ECE 507 (Spring 2021) -
Dissertation
BME 920 (Spring 2021) -
Dissertation
ECE 920 (Spring 2021) -
Analog Integrated Ckts
ECE 450 (Fall 2020) -
Analog Integrated Ckts
ECE 550 (Fall 2020) -
Directed Research
ECE 392 (Fall 2020) -
Dissertation
ECE 920 (Fall 2020) -
Research
BME 900 (Fall 2020) -
Thesis
ECE 910 (Fall 2020)
2019-20 Courses
-
Clinical/Indus Internshp
BME 693A (Summer I 2020) -
Digital Vlsi Sys Design
ECE 407 (Spring 2020) -
Digital Vlsi Sys Design
ECE 507 (Spring 2020) -
Dissertation
ECE 920 (Spring 2020) -
Meas&Data Anls Bio Eng
BME 517 (Spring 2020) -
Meas&Data Anls Bio Eng
ECE 517 (Spring 2020) -
Thesis
ECE 910 (Spring 2020) -
Analog Integrated Ckts
ECE 450 (Fall 2019) -
Analog Integrated Ckts
ECE 550 (Fall 2019) -
Dissertation
ECE 920 (Fall 2019)
2018-19 Courses
-
Digital Vlsi Sys Design
ECE 407 (Spring 2019) -
Digital Vlsi Sys Design
ECE 507 (Spring 2019) -
Dissertation
ECE 920 (Spring 2019) -
Research
ECE 900 (Spring 2019) -
Dissertation
ECE 920 (Fall 2018)
2017-18 Courses
-
Dissertation
ECE 920 (Spring 2018) -
Independent Study
ECE 599 (Spring 2018) -
Research
ECE 900 (Spring 2018) -
Dissertation
ECE 920 (Fall 2017) -
Thesis
ECE 910 (Fall 2017)
2016-17 Courses
-
Dissertation
ECE 920 (Spring 2017) -
Electronic Circuits
ECE 351C (Spring 2017) -
Independent Study
ECE 699 (Spring 2017) -
Research
ECE 900 (Spring 2017) -
Electronic Circuits
ECE 351C (Fall 2016) -
Research
ECE 900 (Fall 2016)
2015-16 Courses
-
Research
ECE 900 (Summer I 2016) -
Dissertation
ECE 920 (Spring 2016) -
Electronic Circuits
ECE 351C (Spring 2016) -
Research
ECE 900 (Spring 2016)
Scholarly Contributions
Chapters
- Roveda, J. M., Powers, L. S., & Ren, K. (2016). Pervasive Computing in Hospital. In Pervasive Computing in Medicine.More infoEditor Dr. Mary Mehrnoosh Eshaghian-Wilner, John Wiley & Sons, USA
Journals/Publications
- Coviello, G., Avitabile, G., Florio, A., Talarico, C., & Wang-Roveda, J. M. (2021). A Novel Low-Power Time Synchronization Algorithm Based on a Fractional Approach for Wireless Body Area Networks. IEEE Access, 9, 134916--134928.
- Li, A. o., Roveda, J. M., Powers, L. S., & Quan, S. F. (2021). Obstructive sleep apnea predicts 10-year cardiovascular disease related mortality in the Sleep Heart Health Study: a machine learning approach. Journal of Clinical Sleep Medicine, jcsm--9630.
- Li, A. o., Chen, S., Quan, S. F., Silva, G. E., Ackerman, C., Powers, L. S., Roveda, J. M., & Perfect, M. M. (2020). Sleep patterns and sleep deprivation recorded by actigraphy in 4th-grade and 5th-grade students. Sleep Medicine, 67, 191-199.
- Quan, S. F., Chen, S., Roveda, J. M., Powers, L. S., Roveda, J. M., Quan, S. F., Powers, L. S., Li, A., & Chen, S. (2020). A deep learning-based algorithm for detection of cortical arousal during sleep.. Sleep, 43(12). doi:10.1093/sleep/zsaa120More infoThe frequency of cortical arousals is an indicator of sleep quality. Additionally, cortical arousals are used to identify hypopneic events. However, it is inconvenient to record electroencephalogram (EEG) data during home sleep testing. Fortunately, most cortical arousal events are associated with autonomic nervous system activity that could be observed on an electrocardiography (ECG) signal. ECG data have lower noise and are easier to record at home than EEG. In this study, we developed a deep learning-based cortical arousal detection algorithm that uses a single-lead ECG to detect arousal during sleep..This study included 1,547 polysomnography records that met study inclusion criteria and were selected from the Multi-Ethnic Study of Atherosclerosis database. We developed an end-to-end deep learning model consisting of convolutional neural networks and recurrent neural networks which: (1) accepted varying length physiological data; (2) directly extracted features from the raw ECG signal; (3) captured long-range dependencies in the physiological data; and (4) produced arousal probability in 1-s resolution..We evaluated the model on a test set (n = 311). The model achieved a gross area under precision-recall curve score of 0.62 and a gross area under receiver operating characteristic curve score of 0.93..This study demonstrated the end-to-end deep learning approach with a single-lead ECG has the potential to be used to accurately detect arousals in home sleep tests.
- Li, A., Chen, S., Quan, S., Powers, L., & Roveda, J. M. (2019). A Deep Learning-based Algorithm for Detection of Cortical Arousal During Sleep. Sleep.
- Roveda, J. M., Chen, S., Li, A., & Powers, L. (2019). The potential capability of deep learning in cardiac arrhythmia classification. Journal of Diagnostic Techniques and Biomedical Analysis.
- Chen, K., Powers, L. S., & Roveda, J. M. (2018). Noise-Invariant Component Analysis for Wearable Sensor based Electrocardiogram Monitoring System. SMJ Biomed Eng.
- Chen, S., Roveda, J., Roveda, J., Li, A., & Chen, S. (2018). Artificial Intelligence in Cardiac Arrhythmia Classification. Research in Medical & Engineering Sciences, 4(4), 1-2. doi:10.31031/rmes.2018.04.000592
- Jeroh, O. E., Powers, L. S., & Roveda, J. M. (2018). Determination of Stress in Humans using Data Fusion of Off-the-Shelf Wearable Sensors Data for Electrocardiogram and Galvanic Skin Response. Journal of Biomedical Sciences. doi:10.4172/2254-609X.100090
- Quan, S. F., Silva, G. E., Roveda, J. M., Silva, G. E., Roveda, J. M., Quan, S. F., Perfect, M. M., & Li, A. (2018). A Novel Artificial Neural Network Based Sleep-Disordered Breathing Screening Tool.. Journal of clinical sleep medicine : JCSM : official publication of the American Academy of Sleep Medicine, 14(6), 1063-1069. doi:10.5664/jcsm.7182More infoThis study evaluated a novel artificial neural network (ANN) based sleep-disordered breathing (SDB) screening tool incorporating nocturnal pulse oximetry with demographic, anatomic, and clinical data. The tool was compatible with 6 categories of apnea-hypopnea index (AHI) with 4% oxyhemoglobin desaturation threshold, ≥ 5, 10, 15, 20, 25, and 30 events/h..Using a general population dataset, the training set included 2,280 subjects, whereas the test set included 470 subjects. The input of this tool was a set of 22 variables. The tool had six neural network models for each AHI threshold. Several metrics were explored to evaluate the performance of the tool: area under the receiver operating characteristic curve (AUC), sensitivity, specificity, positive predictive value, negative predictive value, and 95% confidence interval (CI)..The AUC was 0.904, 0.912, 0.913, 0.926, 0.930, and 0.954, respectively, with models of AHI ≥ 5, 10, 15, 20, 25, and 30 events/h thresholds. The sensitivities of all neural network models were higher than 95%. The AHI ≥ 30 events/h model had the maximum sensitivity: 98.31% (95% CI: 95.01%-100%)..The results of this study suggested that the ANN based SDB screening tool can be used to identify the presence or absence of SDB. Future validation should be performed in other populations to determine the practicability of this screening tool in sleep clinics and other at-risk populations.
- Roveda, J. M., Powers, L. S., Smith, H. D., Kilungo, A. P., Ellis Jr, W., McKay, C. P., & Bonaccorsi, R. (2018). In situ Real-time Quantification of Microbial Communities: Applications to Cold and Dry Volcanic Habitats. Global Ecology and Conservation.More infoe00458
- Li, A., Quan, S., Silva, G. E., Perfect, M., & Roveda, J. M. (2017). A Novel Artificial Neural Network Based Sleep Disordered Breathing. Journal of Clinical Sleep Medicine.
- Powers, L. S., Roveda, J. M., Zhang, Y., Wu, W. T., Roveda, J. M., Powers, L. S., Pan, H., Nasibulin, R., Hall, P. W., Fairbanks, J. V., & Chen, K. (2017). Low power real-time data acquisition using compressive sensing. Proceedings of SPIE, 10194. doi:10.1117/12.2263220More infoNew possibilities exist for the development of novel hardware/software platforms havin g fast data acquisition capability with low power requirements. One application is a high speed Adaptive Design for Information (ADI) system that combines the advantages of feature-based data compression, low power nanometer CMOS technology, and stream computing [1]. We have developed a compressive sensing (CS) algorithm which linearly reduces the data at the analog front end, an approach which uses analog designs and computations instead of smaller feature size transistors for higher speed and lower power. A level-crossing sampling approach replaces Nyquist sampling. With an in-memory design, the new compressive sensing based instrumentation performs digitization only when there is enough variation in the input and when the random selection matrix chooses this input.
- Roveda, J. M., Chen, K. M., & Powers, L. S. (2017). Sweet Dreams: Review of a Sleep Tracking Framework. International Journal of Biosensors and Bioelectronics, 2.
- Fink, W., Roveda, J. M., Kincade, A. M., White, J. L., Wangusi, T. W., & TF, M. F. (2016). Self-Powering and Geo-Tagging Method for Pipeline Inspection Gauges to Enable Continuous High-Quality Data- Recording. Journal of Pipeline Engineering, 47-55.
- Fink, W., Roveda, J. M., Kincade, A. M., White, J. L., Wangusi, T. W., & TF, M. F. (2016). Self-Powering and Geo-Tagging Method for Pipeline Inspection Gauges to Enable Continuous High-Quality Data- Recording. Journal of Pipeline Engineering.
- Lysecky, S., Roveda, J., Sprinkle, J., Sprinkle, J., Son, Y. J., Roveda, J., Qin, X., Lysecky, S., & Lin, L. (2016). A modular framework to enable rapid evaluation and exploration of energy management methods in smart home platforms. Energy Systems, 7(2), 215-235. doi:10.1007/s12667-014-0121-9More infoNumerous efforts focus on developing smart grid and smart home platforms to provide monitoring, management, and optimization solutions. In order to more effectively manage energy resources, a holistic view is needed; however the involved platforms are complex and require integration of a multitude of parameters such as the end-user behavior, underlying hardware components, environment, etc., many of which operate on varying time scale at various levels of detail. A general and modular framework is presented to enable designers to focus on modeling, simulating, analyzing, or optimizing specific sub-components without requiring a detailed implementation across all levels. We incorporate two case studies in which the proposed framework is utilized to help an end user evaluate platform configurations given an energy usage model, as well as integrate an energy optimization module to investigate rescheduling of appliance usage times in an effort to lower cost.
- Roveda, J. M., Roveda, J. M., & Fink, W. (2016). System perspectives for mobile platform design in m-Health. Proceedings of SPIE, 9836. doi:10.1117/12.2219645More infoAdvances in integrated circuit technologies have led to the integration of medical sensor front ends with data processing circuits, i.e., mobile platform design for wearable sensors. We discuss design methodologies for wearable sensor nodes and their applications in m-Health. From the user perspective, flexibility, comfort, appearance, fashion, ease-of-use, and visibility are key form factors. From the technology development point of view, high accuracy, low power consumption, and high signal to noise ratio are desirable features. From the embedded software design standpoint, real time data analysis algorithms, application and database interfaces are the critical components to create successful wearable sensor-based products.
- Zhou, H., Xiao, L., Tian, J., Sun, J., & Roveda, J. (2016). Surrogating circuit design solutions with robustness metrics. Integration, 52, 1-9. doi:10.1016/j.vlsi.2015.07.015More infoWith the increase in device variability, the performance uncertainty poses a daunting challenge to analog/mixed-signal circuit design. This situation requires a robust design approach to add large margins to the circuit and system-level specification to ensure correct operation and the overall yield. In this paper, we propose a new robust design approach by using norm metrics to quantify the robustness for both design parameters and performance uncertainty. In addition, we adopt a surrogating procedure to achieve robustness in design space and to reduce uncertainty in performance space. The end result of the proposed method is a Pareto-surface that provides the designer with trade-offs between design robustness and performance uncertainty. One advantage of this new approach is the ability to take into account the strong nonlinear relationship between performance and design parameters. Considering a set of highly nonlinear circuit performances, we demonstrate the effectiveness of this robust design framework on a fully CMOS operational amplifier circuit. HighlightsA new robustness model that estimates robustness of both design variability and performance uncertainty.A two-way mapping design framework that integrates design space robustness and performance space robustness.A surrogate-based optimization procedure for the proposed design methodology.Promising robustness improvements compared with the conventional robust design approach.
- Roveda, J. M., & Powers, L. S. (2015). Compressive Sensing: Real-Time Data Acquisition and Analysis for Biosensors and Biomedical Instrumentation (invited editorial article). Journal of Biosensors, 2015.
- Roveda, J., Powers, L. S., Roveda, J., & Powers, L. S. (2015). Secure and Compressive Acquisition for Telemedicine Applications. Journal of Bioengineering and Biomedical Science. doi:10.4172/2155-9538.s1.002
- Subramaniam, A. R., Roveda, J., & Cao, Y. (2015). Finite-point method for efficient timing characterization of sequential elements. Integration, 49, 104-113. doi:10.1016/j.vlsi.2014.11.004More infoTiming characterization of sequential elements, such as latches and flip-flops, is one of the critical steps for timing closure in the pipelined design. Traditional characterization of setup and hold time constraints is computationally intensive, due to the demand on high accuracy in monitoring the operation failure. To improve the efficiency, this work proposes a finite-point based method for the characterization of setup and hold time constraints. The finite-point method identifies several critical data points in the non-linear curve of timing characteristics, and abstracts the essential setup/hold information from them. Moreover, compact models are derived for each point, further reducing the computation cost. The proposed method is general for all sequential elements in the standard cell library. It is comprehensively validated using benchmark circuits at 45nm node. Experimental results demonstrate approximately 25i? reduction in characterization time, with the prediction error in setup and hold time within 9% of FO4 nominal delay, as compared to that of SPICE simulation results. An efficient method for setup / hold time constraints using Finite-Point approach.Critical data points abstracted from non-linear curve of timing characteristics.Significant reduction in computation cost and data volume achieved.Accuracy comparable to SPICE simulation data.Same approach can be extended for all sequential cells for all technology nodes.
- Subramaniam, A., Roveda, J. M., & Cao, Y. K. (2015). A Finite-Point Method for Efficient Gate Characterization under Multiple Input Switching. ACM Trans. Design Automation of Electronic System.
- Sun, J., Gupta, P., Talarico, C., & Roveda, J. M. (2015). Budget Uncertainty based Mixed Signal Design Using Conic Algorithms. ACM Trans. Design Automation of Electronic System, 2015.
- Sun, J., He, Z., & Roveda, J. M. (2015). Surrogating Circuit Design Solutions with Robustness Metrics. VLSI Journal, Elsevier, 2015.
- Talarico, n., Agarwal, G., & Roveda, J. M. (2015). Design Optimization of a Transimpedance Amplifier for a Fiber Optic Receiver. Circuits, Systems & Signal Processing, Springer, 34, 2785-2800.
- Muchherla, K. K., Kumar, J. G., & Roveda, J. M. (2014). Cluster based dynamic area-array I/O planning for flip chip technology. Microelectronic Engineering, 114, 57--69.
- Nguyen, D., Chang, H., Talarico, C., Annamalai, A., Zhou, H., & Roveda, J. M. (2014). Intelligent Connector (I-Connector) Design and Demand Response in Smart Grid. Journal of Clean Energy Technologies, 2(1).
- Qin, X., Lin, L., Lysecky, S., Roveda, J., Son, Y., & Sprinkle, J. (2014). A modular framework to enable rapid evaluation and exploration of energy management methods in smart home platforms. Energy Systems, 1--21.
- Subramaniam, A. R., Roveda, J., & Cao, Y. (2014). Finite-point method for efficient timing characterization of sequential elements. Integration, the VLSI Journal.
- Sun, J., Lysecky, R., Shankar, K., Kodi, A., Louri, A., & Roveda, J. (2014). Workload assignment considering NBTI degradation in multicore systems. ACM Journal on Emerging Technologies in Computing Systems (JETC), 10(1), 4.
- Talarico, C., Agrawal, G., & Roveda, J. M. (2014). Design Optimization of a TIA for High Speed Data Applications using gm/ID Based Methodology. Journal of Circuits, Systems and Signal Processing.
- Wang, C., Zhang, B., Ren, K., Roveda, J. M., Chen, C. W., & Xu, Z. (2014). A Privacy-aware Cloud-assisted Healthcare Monitoring System via Compressive Sensing. IEEE Infocom.
- sun, J., & Roveda, J. M. (2014). Robust Optimization for Gate Sizing Considering Non-Gaussian Local Variations. Optimization, Applied Mathematics.
- Lysecky, R., Roveda, J., Zheng, R., Velamala, J., Sun, J., Shankar, K., Roveda, J., Lysecky, R., & Cao, Y. (2013). A self-tuning design methodology for power-efficient multi-core systems. ACM Transactions on Design Automation of Electronic Systems, 18(1), 1-24. doi:10.1145/2390191.2390195More infoThis article aims to achieve computational reliability and energy efficiency through codevelopment of algorithms, device, and circuit designs for application-specific, reconfigurable architectures. The new methodology characterizes aging-switching activity and aging-supply voltage relationships that are applicable for minimizing power consumption and task execution efficiency in order to achieve low bit energy ratio (BER). In addition, a new dynamic management algorithm (DMA) is proposed to alleviate device degradation and to extend system lifespan. In contrast to traditional workload balancing schemes in which cores are regarded as homogeneous, the new algorithm ranks cores as “highly competitive,” “less competitive,” and “not competitive” according to their various competitiveness. Core competitiveness is evaluated based upon their reliability, temperature, and timing requirements. Consequently, “competitive” cores will take charge of the majority of the tasks at relatively high voltage/frequency without violating power and timing budgets, while “not competitive” cores will have light workloads to ensure their reliability. The new approach combines intrinsic device characteristics (aging-switching activity and aging-supply voltage curves) into an integrated framework to achieve high reliability and low energy level with graceful degradation of system performance. Experimental results show that the proposed method has achieved up to 20p power reduction, with about 4p performance degradation (in terms of accomplished workload and system throughput), compared with traditional workload balancing methods. The new method also improves system mean-time-to-failure (MTTF) by up to 25p.
- Talarico, C., Sun, J., Gupta, P., & Roveda, j. M. (2013). A New Framework for the Robust Design of Analog Blocks using Conic Uncertainty Budgeting. Journal of Circuits, Systems and Signal Processing, 7, 143-150.
- Wang, C., Zhang, B., Ren, K., & Roveda, J. M. (2013). Privacy-assured Outsourcing of Image Reconstruction Service in Cloud. IEEE Transactions on Emerging Topics in Computing, 166-177.
- Zhou, H., Bhopalwala, M., & Roveda, J. (2013). On-Chip Interconnect Network Communication Management for Multi-Core Design. Journal of Advances in Computer Network, 1(3).
- Zhou, H., Powers, L. S., & Roveda, J. M. (2013). Data Intensive Design for Multi-core Era. IERI Procedia, 4, 275--281.
- Nguyen, D. C., Ma, D. B., & Roveda, J. M. (2012). Recent Design Development in Molecular Imaging for Breast Cancer Detection Using Nanometer CMOS Based Sensors. Journal of oncology, 2012.
- Sun, J., Zheng, R., Velamala, J., Cao, Y., Lysecky, R., Shankar, K., & Roveda, J. (2012). A self-tuning design methodology for power-efficient multi-core systems. ACM Transactions on Design Automation of Electronic Systems (TODAES), 18(1), 16.
Proceedings Publications
- Coviello, G., Avitabile, G., Florio, A., Talarico, C., & Roveda, J. (2021). Relationship between Timer Resolution and Synchronization Message Repetition Frequency in a Synchronized Wireless Sensor Network. In 2021 International Conference on Computer Engineering and Artificial Intelligence (ICCEAI).
- Coviello, G., Avitabile, G., Talarico, C., Roveda, J., & Florio, A. (2021). A Fractional Approach to Time Synchronization in Wireless Body Area Networks. In 2021 IEEE International Midwest Symposium on Circuits and Systems (MWSCAS).
- Chen, S., Li, A. o., & Roveda, J. (2020). Sequence-level Supervised Deep Neural Networks for Mitosis Event Detection in Time-Lapse Microscopy Images. In 2020 IEEE International Conference on Bioinformatics and Biomedicine (BIBM).
- Chen, K., Zhang, N., Powers, L., & Roveda, J. (2019). Cell Nuclei Detection and Segmentation for Computational Pathology Using Deep Learning. In 2019 Spring Simulation Conference (SpringSim).
- Chen, S., Li, A. o., Lasick, K., Huynh, J., Powers, L., Roveda, J., & Paek, A. (2019). Weakly Supervised Deep Learning for Detecting and Counting Dead Cells in Microscopy Images. In 2019 18th IEEE International Conference On Machine Learning And Applications (ICMLA).
- Liu, B., Chen, K., Seo, M., Roveda, J. M., & Lysecky, R. L. (2019, December). Evaluation of the Complexity of Automated Trace Alignment using Novel Power Obfuscation Methods. In ACM Great Lakes Symposium on VLSI, 467-470.
- Roveda, J. M., & Chen, S. (2019, Fall). High Yield and Precision Plant Production with Sensors and Microgrid Technologies. In Agriculture and Food Conference, Las Vegas, NV.
- Dai, C., Havey, E., Miller, J., Powers, L., & Roveda, J. M. (2018, August). A low power occlusion dose monitor for compliance to glasses wearing. In Biosensor Congress, Chicago IL.
- Geiger, J. M., Li, A., Chen, S., Roveda, J. M., & Perfect, M. M. (2018, Spring). Engaging Students Through STEM: A 21st Century Learning Approach. In Poster presented at the 2018 National Association of School Psychologists annual convention, Chicago, IL.
- Liu, B., Lysecky, R. L., & Roveda, J. M. (2018, October). Composable Template Attacks using Templates for Individual Architectural Components. In IEEE International Conference on Computer Design.More infoBozhi Liu, Roman Lysecky, and Janet Roveda, Best Paper Award.
- Powers, L., & Roveda, J. M. (2018, August). A new prototype for real time, time resolved nanosecond fluorescence lifetime measurements. In Biosensor Congress, Chicago IL.
- Valdez, H., Reeves, K., Zhang, N., Rahman, T., Powers, L., Martins, T., & Roveda, J. M. (2018, August). Using wearable sensors and music to build a stress intervention system. In Biosensor Congress, Chicago IL.
- Li, A., Roveda, J. M., Quan, S. F., Chen, S., Powers, L. S., Perfect, M., & Quan, S. (2017, Spring). Dream Sweet Dreams: A New Framework for Sleep Tracking and Body Change Prediction”, Keynote, Simulation and Modeling for Medical Systems. In ACM simulation series; Virginia Beach, VA, 49, 106-107.
- Li, A., Roveda, J., Powers, L. S., Powers, L. S., Perfect, M. M., Quan, S. F., Quan, S. F., & Roveda, J. M. (2017). Dream sweet dreams: a new framework for sleep tracking and body change prediction. In MSM '17: Proceedings of the Symposium on Modeling and Simulation in Medicine.More infoThis paper addresses a real-world issue, sleep insufficiency, and its health consequences, as an innovative vehicle to improve elementary education in Science, Technology, Engineering and Math (STEM). Partnering with the Catalina Foothills School District, researchers at the University of Arizona developed, implemented, and evaluated a new STEM education program that includes a database, a set of prediction and simulation models, and a set of sleep science lessons (developed in collaboration with Biological Sciences Curriculum Study). The new "MySleep" program allows for tracking of sleep patterns in the students' natural environment, and provides real-time feedback, options for communications with teachers and parents, and data analysis capabilities.
- Powers, L. S., Hall, P., Fairbanks, J., Nasibulin, R., Zhang, Y., & Roveda, J. M. (2017, April 17-21). Low Power Real-time Data Acquisition using Compressive Sensing. In SPIE Defense+Commercial Sensing.
- Roveda, J. M., Slepian, M. J., Perfect, M. M., Quan, S. F., & Rahman, F. (2017, Feb). Embedded systems in Medical devices and their integration with medical data, EMR and Big Data. In International Conference on Biomedical and Health Informatics (BHI).
- Fink, W., & Roveda, J. M. (2016, Aug. 14). Spatial and Temporal Model for Infectious Disease Prediction Considering Human Mobility. In Military Health System Research Symposium.
- Roveda, J. M., & Fink, W. (2016, April 17-21). System Perspectives for Mobile Platform Design in m-Health. In SPIE Defense+Commercial Sensing.
- Roveda, J. M., & Fink, W. (2016, Aug 14). Modular Low Power Wearable Sensors for Physiological Monitoring Throughout the Continuum of Combat Care. In Military Health System Research Symposium.
- Roveda, J. M., & Fink, W. (2016, Aug. 9-12). Real-time Dynamic Data Driven System for Stress Management. In Dynamic Data Driven Applications Systems.
- Roveda, J. M., Fink, W., Chen, W., Chen, K., & Wu, W. (2016, n/a). Psychological Health Monitoring for Pilots and Astronauts by Tracking Sleep-Stress-Emotion Changes. In IEEE Aerospace Conference, Big Sky Montana.
- Roveda, J. M., Perfect, M. M., & Quan, S. F. (2016, April 3-6). Modeling and Simulation for pediatric sleep and education performance correlations. In Spring Simulation Multi-Conference.
- Roveda, J. M., Powers, L. S., & Groth, C. (2016, Aug. 9-12). Dynamic Data Driven System for Real-time Streamline Processing on UAVs. In Dynamic Data Driven Applications Systems.
- Chen, K., Fink, W., Lane, R., Allen, J., Vanuk, J., & Roveda, J. M. (2015, April). Identify Invariant Patterns in ECG and Respiratory Waveforms (Invited Talk). In Biomedical Engineering Conference 2015 by Omics International group, Philadelphia PA.More infoAbstract and oral presentation
- Chen, K., Fink, W., Lane, R., Allen, J., Vanuk, J., & Roveda, J. M. (2015, June). Wearable Sensor Based Stress Management Using Integrated Respiratory and ECG Waveforms. In International Conference on Body Sensor Network, Boston, MA.
- Chen, K., Fink, W., Lane, R., Allen, J., Vanuk, J., & Roveda, J. M. (2015, June). Wearable Sensor Based Stress Management Using Integrated Respiratory and ECG Waveforms. In International Conference on Body Sensor Network, Cambridge, MA.
- Fink, W., & Roveda, J. M. (2015, n/a). Environmental Impact of Infectious Disease Propagation. In 2015 Defense Energy Innovation Summit.More infoAbstract and poster presentation
- Fink, W., & Roveda, J. M. (2015, n/a). Stress Management Using Wearable Sensors Using Integrated Data Information. In 2015 Defense Energy Innovation Summit.
- Jung, S., & Roveda, J. M. (2015, April). Design of Low Jitter Phase-Locked Loop with Closed Loop Voltage Controlled Oscillator. In IEEE Wireless and Microwave Technology Conference.
- Jung, S., & Roveda, J. M. (2015, June). Design of a Feedback Digitally Controlled Oscillator for Linear Enhancement. In IEEE Conference on Electronic Designs and Solid-State Circuits.
- Jung, S., & Roveda, J. M. (2015, March). A Radiation-Hardened-By-Design Phase-Locked Loop Using Feedback Voltage Controlled Oscillator. In International Symposium on Quality Electronic Design.
- Jung, S., & Roveda, J. M. (2015, n/a). A 320MHz-2.56GHz Low Jitter Phase-Locked Loop with Adaptive-Bandwidth Technique. In IEEE International System-On-Chip Conference (IEEE SOCC), 2015.
- Jung, S., & Roveda, J. M. (2015, n/a). A Low Jitter Digital Phase-Locked Loop with a Hybrid Analog/Digital PI Control. In IEEE International New Circuits and Systems Conference (NEWCAS).
- Roveda, J. M., & Powers, L. S. (2015, April). Secure and Compressive Acquisition for Telemedicine Applications (Invited talk). In Biomedical Engineering Conference 2015 by Omics International group.
- Roveda, J. M., & Powers, L. S. (2015, December). Compressive Sensing based Real Time Data Acquisition and Identification (RAID). In Defense Innovation Summit, Austin, Texas.
- Zhou, H., Yoo, S., Melde, K. L., Sun, J., & Roveda, J. M. (2015, June). HyNoC: An All-Direction Millimetre-Wave Wireless and Wired Reconfigurable Communication Fabric Design for Network-on-Chip. In Work-in- Progress, Design Automation Conference.
- Chen, K., Lane, R., & Roveda, J. M. (2014, december). Data Calibration and Dimensionality reduction for Health Care Systems. In International Conference on Electronics, Communications, and Networks.
- Nguyen, D., Roehrig, H., Borders, M. H., Fitzpatrick, K. A., & Roveda, J. (2014). Innovative visualization and segmentation approaches for telemedicine. In SPIE Optical Engineering+ Applications, 92140F--92140F.
- Nguyen, D., Roehrig, H., Borders, M. H., Fitzpatrick, K. A., & Roveda, J. (2014, August). Innovative visualization and segmentation approaches for telemedicine. In SPIE Optical Engineering+ Applications, 92140F--92140F.
- Shaikh, F., He, W., Sprinkle, J., & Roveda, J. M. (2014, december). A Heterogeneity Based Method to Identify Major Variability Components. In International Conference on Electronics, Communications, and Networks.
- Talarico, C., Agrawal, G., & Roveda, J. M. (2014, August). A 60dBΩ 2.9 GHz 0.18 μm CMOS Transimpedance Amplifier for a Fiber Optic Receiver Application. In IEEE 57th Int’l Midwest Symposium Circuits and Systems.
- Wang, C., Zhang, B., Ren, K., Roveda, J. M., Chen, C. W., & Xu, Z. (2014, April 29). A Privacy-aware Cloud-assisted Healthcare Monitoring System via Compressive Sensing. In IEEE Infocom.
- Zhou, H., Zhou, H., Powers, L. S., Powers, L. S., Roveda, J. M., & Roveda, J. M. (2014). Collision array based workload assignment for Network-on-Chip concurrency. In System-on-Chip Conference (SOCC), 2014 27th IEEE International, 188--191.
- Zhou, H., Zhou, H., Powers, L. S., Powers, L. S., Roveda, J., & Roveda, J. (2014). Increase the concurrency for multi-core systems through collision array based workload assignment. In Information Science, Electronics and Electrical Engineering (ISEEE), 2014 International Conference on, 2, 1209--1215.
- Gupta, P., Akoglu, A., Melde, K., & Roveda, J. (2013). FPGA based single cycle, reconfigurable router for NoC applications. In Circuits and Systems (ISCAS), 2013 IEEE International Symposium on, 2428--2431.
- Nguyen, D., Ren, K., & Roveda, J. (2013). A new data acquisition design for breast cancer detection system. In SOC Conference (SOCC), 2013 IEEE 26th International, 61--66.
- Nguyen, D., Roehrig, H., Borders, M. H., Fitzpatrick, K. A., & Roveda, J. (2013). Efficient image acquisition design for a cancer detection system. In SPIE Optical Engineering+ Applications, 88530K--88530K.
- Roveda, J., Nguyen, D., Powers, L., Ren, K., & Fairbanks, J. (2013). Effective signal region based analog mixed signal design considering variations and applications. In SOC Conference (SOCC), 2013 IEEE 26th International, 233--238.
- Talarico, C., Chang, H., Annamalai, A., & Roveda, J. (2013). Energy management design for smart homes using green technology. In Circuits and Systems (MWSCAS), 2013 IEEE 56th International Midwest Symposium on, 517--520.
- Nguyen, D., & Roveda, J. M. (2012, June). Low Current Laser Diode Driver and High Sensitivity Piezoelectric Transducer Design for Real Time Blood Glucose Monitoring. In Nano Technology.
- Nguyen, D., Nguyen, D., Nguyen, H., Nguyen, H., Roveda, J., & Roveda, J. (2012). A non-invasive blood glucose meter design using multi-type sensors. In SPIE Optical Engineering+ Applications, 85080E--85080E.
- Roveda, J. M., Lysecky, S., & Son, Y. J. (2012, April). A New Way to Look at Energy and Data Flow for Smart Grid. In IEEE Smart Grid Newsletter.
- Roveda, J. M., Nguyen, D., & Gupta, P. (2012, november). Application Driven, Analog Mixed Signal Design Considering Variations. In International Workshop on Design Automation for Analog and Mixed-Signal Circuits.
- Shaikh, F., He, W., Sprinkle, j., & Roveda, J. M. (2012, November). Identifying Key Components of Variability Using Energy based Control. In IEEE/ACM Workshop on Variability Modeling and Characterization (VMC).
- Sun, J., Gupta, P., & Roveda, J. (2012). A new uncertainty budgeting based method for robust analog/mixed-signal design. In Proceedings of the 49th Annual Design Automation Conference, 529--535.
- wang, c., nguyen, d., ren, k., & Wang, M. (2012, september). Reliable, Secure and Compressed Storage for Medical Image Data with Cloud Computing. In International Conference on New Energy, Bio-Medical Engineering and Food Security.
- Roveda, J., Lysecky, S., Son, Y. J., Roveda, J., Qin, X., Lysecky, S., Chang, H., & Annamalai, A. (2011). Interface model based cyber-physical energy system design for smart grid. In 2011 IEEE/IFIP 19th International Conference on VLSI and System-on-Chip, 368-373.More infoEnergy harvesting is becoming one of the most important issues today due to the fast depletion of conventional energy resources. Current research efforts focus on efficient and reliable use of renewable resources such as solar, hydro, wind, and thermal resources to provide environmentally friendly solutions while minimizing the cost. However, an efficient and effective delivery of green energy to individual households remains a challenging issue. One key roadblock is the high complexity of the smart grid system. In this paper, we propose a new interface theory based energy-timing model for smart homes and smart grid management and design. This model allows simple yet accurate computations for energy supply and consumptions involved with photovoltaic (PV) cells, storage units, and load appliances for each home. At community, and city or state level (or nationwide), this model also has the potential to facilitate fast and effective energy management, optimization, and load scheduling. The end result is the possibility of managing smart grid with millions of households to achieve low cost and high energy savings
- Roveda, J., Lysecky, R., Zheng, R., Velamala, J., Sun, J., Shankar, K., Roveda, J., Lysecky, R., & Cao, Y. (2010). A self-evolving design methodology for power efficient multi-core systems. In 2010 IEEE/ACM International Conference on Computer-Aided Design (ICCAD), 264-268.More infoThis paper introduces a new methodology that characterizes aging-duty cycle and aging-supply voltage relationships that are applicable to minimizing power consumption and task execution time to achieve low Bit-Energy-Ratio (BER). In contrast to the traditional workload balancing scheme where cores are regarded as homogeneous, we proposed a new task scheduler that ranks cores according to their various competitiveness evaluated based upon their reliability, temperature and timing requirements. Consequently, the new approach combines internal characteristics (aging-duty cycle and aging-supply voltage curves) into an integrated framework to achieve system performance improvement or graceful degradation with high reliability and low power. Experimental results show that the proposed method has achieved 18% power reduction with about 4% performance degradation (in terms of accomplished workload) compared with traditional workload balancing methods.
Presentations
- Fink, W., & Roveda, J. M. (2016, August). Real-time Dynamic Data Driven System for Stress Management. InfoSymbiotics/DDDAS Conference. Hartford, CT: DDDAS Conference.More infoProc. SPIE 9836, Micro- and Nanotechnology Sensors, Systems, and Applications VIII, 983610 (17 May 2016); doi: 10.1117/12.2219645
- Chen, K., Fink, W., Lane, R., Allen, J., Vanuk, J., & Roveda, J. M. (2015, April). Identify Invariant Patterns in ECG and Respiratory Waveforms (Invited Talk). Biomedical Engineering Conference 2015 by Omics International group, Philadelphia PA.More infoAbstract and oral presentation
- Wang, C., Xu, Z., Ren, K., & Roveda, J. M. (2013, march). OIRS: Outsourced Image Recovery Service from Compressive Sensing with Privacy Assurance. Annual Network & Distributed System Security Symposium.
- Wang, M., & Perfect, M. (2012, september). Measured Health and Life: VLSI Designs for Future Mobile Health. Mhealth and Mobile Matters Symposium.
Poster Presentations
- Fink, W., & Roveda, J. M. (2016, Aug. 14). Spatio-Temporal Model for Infectious Disease Prediction Considering Human Mobility. Military Health System Research Symposium.
- Roveda, J. M., Neifeld, M. A., & Thamvichai, R. (2016, Sept). The Subthreshold Design for the Computation in Graphic Interface. MURI Optical Science. Tucson Arizona: ONR.
- Fink, W., & Roveda, J. M. (2015, n/a). Environmental Impact of Infectious Disease Propagation. 2015 Defense Energy Innovation Summit.More infoAbstract and poster presentation
- Fink, W., & Tarbell, M. A. (2015, May). Smart Ophthalmics: A Smart Service Platform for Tele-‐Ophthalmology. 2015 Association for Research in Vision and Ophthalmology. Denver, Colorado: Association for Research in Vision and Ophthalmology.
Others
- Chen, S. (2020). Machine Learning Methods for Drug Evaluation and Treatment Assessment.
- Harvey, E. M., Miller, J. M., Mohler, M. J., & Roveda, J. M. (2020). Glasses compliance monitor and associated method.
- Li, A. o., Quan, S. F., & Roveda, J. M. (2019). Artificial Neural Network Based Sleep Disordered Breathing Screening Tool.