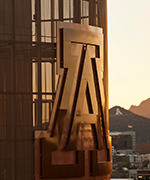
Clayton T Morrison
- Associate Professor, School of Information
- Associate Professor, Statistics-GIDP
- Associate Professor, Cognitive Science - GIDP
- Member of the Graduate Faculty
Contact
- Richard P. Harvill Building, Rm. 437A
- Tucson, AZ 85721
- claytonm@arizona.edu
Degrees
- M.S. Computer Science
- University of Massachusetts, Amherst, Massachusetts, United States
- Ph.D. Philosophy
- Binghamton University, Binghamton, New York, United States
- Situated Representation
- M.A. Philosophy
- Binghamton University, Binghamton, New York, United States
- B.A. Cognitive Science
- Occidental College, Los Angeles, California, United States
Work Experience
- University of Arizona, Tucson, Arizona (2014 - Ongoing)
- University of Arizona, Tucson, Arizona (2011 - 2014)
- SISTA, University of Arizona (2011 - 2013)
- Computer Science and SISTA, University of Arizona (2008 - 2011)
- USC Information Sciences Institute (2006 - 2008)
- USC Information Sciences Institute (2003 - 2006)
- Computer Science, University of Massachusetts (2001 - 2003)
- Computer Science, University of Massachusetts (1999 - 2001)
Interests
Research
Machine Learning, Causal Inference, Artificial Intelligence, Automated Planning, Knowledge Representation, Cognitive Science
Courses
2025-26 Courses
-
Intro to Machine Learning
INFO 521 (Fall 2025)
2024-25 Courses
-
Directed Research
INFO 692 (Spring 2025) -
Dissertation
INFO 920 (Spring 2025) -
Neural Networks
INFO 557 (Spring 2025) -
Neural Networks
ISTA 457 (Spring 2025) -
Directed Research
INFO 692 (Fall 2024) -
Dissertation
INFO 920 (Fall 2024) -
Neural Networks
INFO 557 (Fall 2024) -
Neural Networks
ISTA 457 (Fall 2024)
2023-24 Courses
-
Dissertation
CSC 920 (Spring 2024) -
Dissertation
INFO 920 (Spring 2024) -
Independent Study
INFO 699 (Spring 2024) -
Dissertation
CSC 920 (Fall 2023) -
Intro to Machine Learning
INFO 521 (Fall 2023) -
Intro to Machine Learning
ISTA 421 (Fall 2023)
2022-23 Courses
-
Dissertation
CSC 920 (Spring 2023) -
Dissertation
INFO 920 (Spring 2023) -
Directed Research
INFO 692 (Fall 2022) -
Dissertation
CSC 920 (Fall 2022) -
Dissertation
INFO 920 (Fall 2022)
2021-22 Courses
-
Directed Research
INFO 692 (Summer I 2022) -
Artificial Intelligence
INFO 550 (Spring 2022) -
Artificial Intelligence
ISTA 450 (Spring 2022) -
Research
CSC 900 (Spring 2022) -
Research
STAT 900 (Spring 2022) -
Thesis
STAT 910 (Spring 2022) -
Directed Research
INFO 692 (Fall 2021) -
Independent Study
CSC 699 (Fall 2021) -
Intro to Machine Learning
INFO 521 (Fall 2021) -
Intro to Machine Learning
ISTA 421 (Fall 2021) -
Thesis
STAT 910 (Fall 2021)
2020-21 Courses
-
Independent Study
STAT 599 (Spring 2021) -
Research
CSC 900 (Spring 2021) -
Thesis
STAT 910 (Spring 2021) -
Directed Research
INFO 492 (Fall 2020) -
Directed Research
INFO 692 (Fall 2020) -
Dissertation
INFO 920 (Fall 2020) -
Independent Study
INFO 699 (Fall 2020) -
Independent Study
STAT 599 (Fall 2020) -
Thesis
STAT 910 (Fall 2020)
2019-20 Courses
-
Artificial Intelligence
INFO 550 (Spring 2020) -
Artificial Intelligence
ISTA 450 (Spring 2020) -
Capstone
INFO 698 (Spring 2020) -
Dissertation
INFO 920 (Spring 2020) -
Dissertation
INFO 920 (Fall 2019) -
Independent Study
CSC 599 (Fall 2019) -
Independent Study
INFO 699 (Fall 2019) -
Independent Study
MATH 599 (Fall 2019) -
Intro to Machine Learning
INFO 521 (Fall 2019) -
Intro to Machine Learning
ISTA 421 (Fall 2019) -
Research
INFO 900 (Fall 2019)
2018-19 Courses
-
Directed Research
INFO 492 (Spring 2019) -
Dissertation
INFO 920 (Spring 2019) -
Independent Study
STAT 599 (Spring 2019) -
Dissertation
INFO 920 (Fall 2018) -
Independent Study
INFO 699 (Fall 2018) -
Intro to Machine Learning
INFO 521 (Fall 2018) -
Intro to Machine Learning
ISTA 421 (Fall 2018)
2017-18 Courses
-
Capstone
INFO 698 (Summer I 2018) -
Directed Research
INFO 492 (Summer I 2018) -
Artificial Intelligence
INFO 550 (Spring 2018) -
Artificial Intelligence
ISTA 450 (Spring 2018) -
Directed Research
INFO 692 (Spring 2018) -
Dissertation
INFO 920 (Spring 2018) -
Independent Study
INFO 499 (Spring 2018) -
Directed Research
INFO 692 (Fall 2017) -
Dissertation
LIS 920 (Fall 2017) -
Intro to Machine Learning
INFO 521 (Fall 2017) -
Intro to Machine Learning
ISTA 421 (Fall 2017)
2016-17 Courses
-
Artificial Intelligence
INFO 550 (Spring 2017) -
Artificial Intelligence
ISTA 450 (Spring 2017) -
Research
STAT 900 (Spring 2017) -
Dissertation
STAT 920 (Fall 2016) -
Honors Independent Study
ISTA 399H (Fall 2016) -
Independent Study
ISTA 599 (Fall 2016) -
Independent Study
LIS 699 (Fall 2016) -
Intro to Machine Learning
INFO 521 (Fall 2016) -
Intro to Machine Learning
ISTA 421 (Fall 2016)
2015-16 Courses
-
Honors Thesis
ISTA 498H (Summer I 2016) -
Independent Study
ISTA 499 (Summer I 2016) -
Bayesian Modeling & Inference
INFO 510 (Spring 2016) -
Bayesian Modeling & Inference
ISTA 410 (Spring 2016) -
Directed Research
ISTA 392 (Spring 2016) -
Honors Thesis
ISTA 498H (Spring 2016) -
Internship
ISTA 493 (Spring 2016) -
Research
LIS 900 (Spring 2016)
Scholarly Contributions
Books
- Surdeanu, M., Riloff, E., Chiticariu, L., Frietag, D., Hahn-Powell, G. V., Morrison, C. T., Noriega-Atala, E., Sharp, R., & Valenzuela-Escárcega, M. (2023). Proceedings of the 2nd Workshop on Pattern-based Approaches to NLP in the Age of Deep Learning, 2023. International Conference on Computation Linguistics.More infoIn the past year, the natural language processing (NLP) field (and the world at large!) has been hit by the large language model (LLM) "tsunami." This happened for the right reasons: LLMs perform extremely well in a multitude of NLP tasks, often with minimal training and, perhaps for the first time, have made NLP technology extremely approachable to non-expert users. However, LLMs are not perfect: they are not really explainable, they are not pliable, i.e., they cannot be easily modified to correct any errors observed, and they are not efficient due to the overhead of decoding. In contrast, rule-based methods are more transparent to subject matter experts; they are amenable to having a human in the loop through intervention, manipulation and incorporation of domain knowledge; and further the resulting systems tend to be lightweight and fast. This workshop focuses on all aspects of rule-based approaches, including their application, representation, and interpretability, as well as their strengths and weaknesses relative to state-of-the-art machine learning approaches.
- Chiticariu, L., Goldberg, Y., Hahn-Powell, G. V., Morrison, C. T., Naik, A., Sharp, R., Surdeanu, M., Valenzuela-Escárcega, M., & Noriega-Atala, E. (2022). Proceedings of the First Workshop on Pattern-based Approaches to NLP in the Age of Deep Learning. International Conference on Computational Linguistics.More infoWe all know that deep-learning methods have dominated the field of natural language processing in the past decade. However, these approaches usually rely on the availability of high-quality and highquantity data annotation. Furthermore, the learned models are difficult to interpret and incur substantial technical debt. As a result, these approaches tend to exclude users that lack the necessary machine learning background. In contrast, rule-based methods are easier to deploy and adapt; they support human examination of intermediate representations and reasoning steps; they are more transparent to subjectmatter experts; they are amenable to having a human in the loop through intervention, manipulation and incorporation of domain knowledge; and further the resulting systems tend to be lightweight and fast. This workshop focuses on all aspects of rule-based approaches, including their application, representation, and interpretability, as well as their strengths and weaknesses relative to state-of-the-art machine learning approaches.
- Pickett, M., Kuipers, B., Lecun, Y., & Morrison, C. T. (2013). Learning rich representations from low-level sensors: Papers from the AAAI Workshop, Technical Report WS-13-12. Elsevier.More infoA human-level artificially intelligent agent must be able to represent and reason about the world, at some level, in terms of high-level concepts such as entities and relations. The problem of acquiring these rich high-level representations, known as the "knowledge acquisition bottleneck," has long been an obstacle for achieving human-level AI. A popular approach to this problem is to handcraft these high-level representations, but this has had limited success. An alternate approach is for rich representations to be learned autonomously from low-level sensor data. Potentially, the latter approach may yield more robust representations, and should rely less on human knowledge-engineering.
- Morrison, C. T., & Oates, T. (2007). Computational approaches to representation charge during learning and development : Papers from the AAAI Fall Symposium, Technical Report FS-07-03. AAAI Press.More infoIn nearly every subfield of artificial intelligence, it is both true and well known that using the right representation is crucial. For example, moving from state-space planning to plan-space planning can make previously intractable problems solvable. Representation change is likewise implicated in psychological accounts of learning and problem solving, ranging from the relatively mundane to more significant “aha! moments” where an insight leads to problem reorganization and a breakthrough. Representation change also features prominently in many accounts of perceptual and cognitive development, and in some cases, such as that of Jean Piaget's constructivism, it is the driver of cognitive development. The goal of this symposium was to bring together researchers from a diverse set of fields (artificial intelligence, machine learning, cognitive and developmental psychology, cognitive science, and philosophy) to survey the state of the art and establish a set of open problems and a research agenda in the area of automated development and, more specifically, change of representation. Submissions that emphasize computational mechanisms, present animal and human evidence of representation change, or propose learning and problem solving scenarios that require representation change are particularly encouraged.
- Morrison, C. T. (1998). Situated representation: solving the handcoding problem with emergent structured representation. State University of New York at Binghamton.More infoClayton T. Morrison PhD Dissertation, Binghamton University. Eric Dietrich Advisor.
Chapters
- Beal, C., Morrison, C. T., & Villegas, J. (2013). Human computation as an educational opportunity. In Handbook of Human Computation(pp 163-170). New York: Springer. doi:10.1007/978-1-4614-8806-4_15More info“Citizen science” refers to the emerging practice in which individuals in the community, often en masse, partner with researchers to assist with data collection, analysis or interpretation. Such partnerships benefit researchers through access to data at a scale not possible for individuals or small teams. To date, the benefits to the citizen scientists have been less apparent, although some have argued that participation increases critical thinking and appreciation for science methodologies. The present chapter reports a case study in which 12-year-old citizen scientists contributed to a major research investigation of evapotranspiration and, in turn, deepened their own understanding of the water cycle.
- Morrison, C. T., & Oates, T. (2012). Representation Changes in Learning. In The Encyclopedia of the Sciences of Learning. New York, NY: Springer. doi:10.1007/978-1-4419-1428-6More infoOver the past century, educational psychologists and researchers have posited many theories to explain how individuals learn, i.e. how they acquire, organize and deploy knowledge and skills. The 20th century can be considered the century of psychology on learning and related fields of interest (such as motivation, cognition, metacognition etc.) and it is fascinating to see the various mainstreams of learning, remembered and forgotten over the 20th century and note that basic assumptions of early theories survived several paradigm shifts of psychology and epistemology. Beyond folk psychology and its naïve theories of learning, psychological learning theories can be grouped into some basic categories, such as behaviorist learning theories, connectionist learning theories, cognitive learning theories, constructivist learning theories, and social learning theories. Learning theories are not limited to psychology and related fields of interest but rather we can find the topic of learning in various disciplines, such as philosophy and epistemology, education, information science, biology, and – as a result of the emergence of computer technologies – especially also in the field of computer sciences and artificial intelligence. As a consequence, machine learning struck a chord in the 1980s and became an important field of the learning sciences in general. As the learning sciences became more specialized and complex, the various fields of interest were widely spread and separated from each other; as a consequence, even presently, there is no comprehensive overview of the sciences of learning or the central theoretical concepts and vocabulary on which researchers rely. The Encyclopedia of the Sciences of Learning provides an up-to-date, broad and authoritative coverage of the specific terms mostly used in the sciences of learning and its related fields, including relevant areas of instruction,pedagogy, cognitive sciences, and especially machine learning and knowledge engineering. This modern compendium will be an indispensable source of information for scientists, educators, engineers, and technical staff active in all fields of learning. More specifically, the Encyclopedia provides fast access to the most relevant theoretical terms provides up-to-date, broad and authoritative coverage of the most important theories within the various fields of the learning sciences and adjacent sciences and communication technologies; supplies clear and precise explanations of the theoretical terms, cross-references to related entries and up-to-date references to important research and publications. The Encyclopedia also contains biographical entries of individuals who have substantially contributed to the sciences of learning; the entries are written by a distinguished panel of researchers in the various fields of the learning sciences.
Journals/Publications
- Noriega-Atala, E., Hein, P. D., Thumsi, S. S., Wong, Z., Wang, X., Hendryx, S., & Morrison, C. T. (2020). Extracting Inter-Sentence Relations for Associating Biological Context with Events in Biomedical Texts. IEEE/ACM Transactions of Computational Biology and Bioinformatics (TCBB).More infoWe present an analysis of the problem of identifying biological context and associating it with biochemical events described in biomedical texts. This constitutes a non-trivial, inter-sentential relation extraction task. We focus on biological context as descriptions of the species, tissue type, and cell type that are associated with biochemical events. We present a new corpus of open access biomedical texts that have been annotated by biology subject matter experts to highlight context-event relations. Using this corpus, we evaluate several classifiers for context-event association along with a detailed analysis of the impact of a variety of linguistic features on classifier performance. We find that gradient tree boosting performs by far the best, achieving an F1 of 0.865 in a cross-validation study.
- Savage, R., Palafox, L. F., Morrison, C. T., Rodriguez, J. J., Barnard, K. J., Byrne, S., & Hamilton, C. W. (2018). A Bayesian Approach to Sub-Kilometer Crater Shape Analysis using Individual HiRISE Images. IEEE Transactions on Geoscience and Remote Sensing, PP(99), 1-11. doi:10.1109/TGRS.2018.2825608
- Valenzuela-Escárcega, M. A., Babur, Ö., Hahn-Powell, G., Bell, D., Hicks, T., Noriega-Atala, E., Wang, X., Surdeanu, M., Demir, E., & Morrison, C. T. (2018). Large-scale Automated Reading with Reach Discovers New Cancer Driving Mechanisms. Database: The Journal of Biological Databases and Curation.
- Camillo Villegas, J., Espeleta, J. E., Morrison, C. T., Breshears, D. D., & Huxman, T. E. (2014). Factoring in canopy cover heterogeneity on evapotranspiration partitioning: Beyond big-leaf surface homogeneity assumptions. Journal of Soil and Water Conservation, 69(3), 78A-83A.
- Morrison, C. T., & Snodgrass, R. T. (2011). Computer science can use more science. Communications of The ACM, 54(6), 36-38. doi:10.1145/1953122.1953139More infoSoftware developers should use empirical methods to analyze their designs to predict how working systems will behave.
- Gerst, K. L., Adamson, M., Beal, C. R., Espeleta, J. E., Morrison, C. T., & Villegas, J. C. (2010). Impact of an Ecohydrology Classroom Activity on Middle School Students’ Understanding of Evapotranspiration. Journal of Natural Resources and Life Sciences Education, 39(1), 150-156. doi:10.4195/jnrlse.2009.0044kMore infoABSTRACT Current trends in ecological research emphasize interdisciplinary approaches for assessing effects of present and predicted environmental changes. One such emerging interdisciplinary field is the discipline of ecohydrology, which studies the feedbacks and interactions between ecological and hydrological processes. However, interdisciplinary science, which includes ecohydrology and other fields, has not yet been effectively translated into many K–12 curricula. We adapted an ecohydrological research project, originally conducted at the Biosphere 2 research apparatus, for use in a middle school classroom. The experiment focuses on describing the effects of changes in landscape vegetation cover on the partitioning of evapotranspiration, the major component of the water budget, into plant transpiration and soil evaporation. The 1-week long experiment was conducted by Grade 6 students ( n = 82) in classrooms in Oro Valley, AZ. Students completed pre- and post-experiment tests designed to assess their general understanding of the components of evapotranspiration as well as the scientific procedures that can be used to differ-entiate them. Our results show significant improvement between the pre- and post-experiment evaluations on the understanding of the water cycle concepts, particularly those associated with evapotranspiration. This improvement illustrates how the incorpo-ration of experimental knowledge can constitute a key instrument to successful delivery of scientific information in the classroom. We discuss how current scientific research can be effectively incorporated into the science curriculum, which in turn can be used as an instrumental tool to produce scientifically relevant data.
- Arney, C., Ball, J. T., Chella, A., Collins, S. G., Goebel, K., Li, J. H., Lyell, M., Magerko, B., Manzotti, R., Marcus, M., Morrison, C. T., Nirenburg, S., Oates, T., Riedl, M. O., Trajkovski, G., Truszkowski, W., & Uckun, N. S. (2008). AAAI Fall Symposium Reports. Ai Magazine, 29(1), 99-104. doi:10.1609/aimag.v29i1.2130More infoThe Association for the Advancement of Artificial Intelligence presented the 2007 Fall Symposium Series on Friday through Sunday, November 9–11, at the Westin Arlington Gateway, Arlington, Virginia. The titles of the seven symposia were (1) AI and Consciousness: Theoretical Foundations and Current Approaches, (2) Artificial Intelligence for Prognostics, (3) Cognitive Approaches to Natural Language Processing, (4) Computational Approaches to Representation Change during Learning and Development, (5) Emergent Agents and Socialities: Social and Organizational Aspects of Intelligence, (6) Intelligent Narrative Technologies, and (7) Regarding the “Intelligence” in Distributed Intelligent Systems.
- Land, W. H., Masters, T., Morrison, C. T., & Lo, J. Y. (1999). Application of a GRNN oracle to the intelligent combination of several breast cancer benign/malignant predictive paradigms. Intelligent Engineering Systems Through Artificial Neural Networks, 9, 803-808.More infoThe General Regression Neural Network (GRNN) is well known to be an extremely effective prediction model in a wide variety of problems. It has been recently established that in many prediction problems, the results obtained by intelligently combining the outputs of several different prediction models are generally superior to the results obtained by using any one of the models. An overseer model that combines predictions from other independently trained prediction models is often called an oracle. This paper describes how the GRNN is modified to serve as a powerful oracle for combining decisions from four different breast cancer benign/malignant prediction models using mammogram data. In all experiments conducted, the oracle consistently provided superior benign/malignant classification discrimination as measured by the receiver operator characteristic curve Az index values.
- Beskin, J., Dietrich, E., Loren, L. A., & Morrison, C. T. (1998). WHAT IT MEANS TO BE "SITUATED". Cybernetics and Systems, 29(8), 751-777. doi:10.1080/019697298125399More infoSituated action is a new approach to artificial intelligence that has thus far functioned without any explicit underlying theoretical foundation. As a result, many researchers in artificial intelligence have misunderstood the goals and claims of situated action. In order to rectify this situation, we provide an explicit formulation of the theoretical foundations of situated action.
Proceedings Publications
- Dharma, K., Dayana, V., Wu, M., Cherukuri, V., Hwang, H., & Morrison, C. (2024). Towards Light Weight Object Detection System. In International Workshop on Advanced Image Technology (IWAIT) 2024.More infoTransformers are a popular choice for classification tasks and as backbones for object detection tasks. However, their high latency brings challenges in their adaptation to lightweight classification and object detection systems. We present an approximation of the self-attention layers used in the transformer architecture. This approximation significantly reduces the latency of the classification system while incurring minimal loss in accuracy. We also present a method that uses a transformer encoder layer for multi-resolution feature fusion for object detection. This feature fusion improves the accuracy of the state-of-the-art lightweight object detection system without significantly increasing the number of parameters. These modules can be easily integrated into existing CNN and Transformer architecture to reduce latency and increase the accuracy of the system. Finally, we provide an abstraction for the transformer architecture called Generalized Transformer (gFormer) that can guide the design of novel transformer-like architectures.
- Han, C., Leiffers, J., Morrison, C. T., & Isaacs, K. (2024). An Overview + Detail Layout for Visualizing Compound Graphs. In IEEE VIS 2024.More infoAbstract: Compound graphs are networks in which vertices can be grouped into larger subsets, with these subsets capable of further grouping, resulting in a nesting that can be many levels deep. In several applications, including biological workflows, chemical equations, and computational data flow analysis, these graphs often exhibit a tree-like nesting structure, where sibling clusters are disjoint. Common compound graph layouts prioritize the lowest level of the grouping, down to the individual ungrouped vertices, which can make the higher level grouped structures more difficult to discern, especially in deeply nested networks. Leveraging the additional structure of the tree-like nesting, we contribute an overview+detail layout for this class of compound graphs that preserves the saliency of the higher level network structure when groups are expanded to show internal nested structure. Our layout draws inner structures adjacent to their parents, using a modified tree layout to place substructures. We describe our algorithm and then present case studies demonstrating the layout's utility to a domain expert working on data flow analysis. Finally, we discuss network parameters and analysis situations in which our layout is well suited.
- Kwak, A. S., Morrison, C. T., Bambauer, D. E., & Surdeanu, M. (2024). Classify First, and Then Extract: Prompt Chaining Techniques for Information Extraction. In Proceedings of the Natural Legal Language Processing Workshop 2024 (Colocated with EMNLP 2024).More infoThis work presents a new task-aware prompt design and example retrieval approach for information extraction (IE) using a prompt chaining technique. Our approach divides IE tasks into two steps: (1) text classification to understand what information (e.g., entity or event types) is contained in the underlying text and (2) information extraction for the identified types. Initially, we use a large language model (LLM) in a few-shot setting to classify the contained information. The classification output is used to select the relevant prompt and retrieve the examples relevant to the input text. Finally, we ask a LLM to do the information extraction with the generated prompt. By evaluating our approach on legal IE tasks with two different LLMs, we demonstrate that the prompt chaining technique improves the LLM`s overall performance in a few-shot setting when compared to the baseline in which examples from all possible classes are included in the prompt. Our approach can be used in a low-resource setting as it does not require a large amount of training data. Also, it can be easily adapted to many different IE tasks by simply adjusting the prompts. Lastly, it provides a cost benefit by reducing the number of tokens in the prompt.
- Noriega-Atala, E., Vacareanu, R., Torres Ashton, S., Pyarelal, A., Morrison, C. T., & Surdeanu, M. (2024). When and Where Did it Happen? An Encoder-Decoder Model to Identify Scenario Context. In Findings of the Association for Computational Linguistics: EMNLP 2024.More infoAbstract: We introduce a neural architecture finetuned for the task of scenario context generation: The relevant location and time of an event or entity mentioned in text. Contextualizing information extraction helps to scope the validity of automated finings when aggregating them as knowledge graphs. Our approach uses a high-quality curated dataset of time and location annotations in a corpus of epidemiology papers to train an encoder-decoder architecture. We also explored the use of data augmentation techniques during training. Our findings suggest that a relatively small fine-tuned encoder-decoder model performs better than out-of-the-box LLMs and semantic role labeling parsers to accurate predict the relevant scenario information of a particular entity or event.
- Kc, D., Ferra, T., & Morrison, C. (2023). Neural Machine Translation for Recovering ASTs from Binaries. In The 3rd IEEE International Conference on Software Engineering and Artificial Intelligence (SEAI), 2023.More infoRecovering higher-level abstractions of source code from binaries is an important task underlying malware identification, program verification, debugging, program comparison, vulnerability detection, and helping subject matter experts understand compiled code. Existing approaches to extracting higher-level structures from lower-level binary code rely on hand-crafted rules and generally require great time and effort of domain experts to design and implement. We present Binary2AST, a framework for generating a structured representation of binary code in the form of an abstract syntax tree (AST) using neural machine translation (NMT). We use the Ghidra binary analysis tool to extract assembly instructions from binaries. A tokenized version of these instructions are then translated by our NMT system into a sequence of symbols that represent an AST. The NMT framework uses deep neural network models that can require a lot of training examples. To address this, we have developed a C source code generator for a restricted subset of the C language, from which we can sample an arbitrary number of syntactically correct C source code files that in turn can be used to create a parallel data set suitable for NMT training. We evaluate several variant NMT models on their ability to recover AST representations of the original source code from compiled binaries, where the best-performing attention-based model achieves a BLEU score of 0.99 on our corpus.
- Kwak, A., Jeong, C., Forte, G., Bambauer, D. E., Morrison, C. T., & Surdeanu, M. (2023). Information Extraction from Legal Wills: How Well Does GPT-4 Do?. In Findings of EMNLP (EMNLP), 2023.More infoThis work presents a manually annotated dataset for Information Extraction (IE) from legal wills, and relevant in-context learning experiments on the dataset. The dataset consists of entities, binary relations between the entities (e.g., relations between testator and beneficiary), and n-ary events (e.g., bequest) extracted from 45 legal wills from two US states. This dataset can serve as a foundation for downstream tasks in the legal domain. Another use case of this dataset is evaluating the performance of large language models (LLMs) on this IE task. We evaluated GPT-4 with our dataset to investigate its ability to extract information from legal wills. Our evaluation result demonstrates that the model is capable of handling the task reasonably well. When given instructions and examples as a prompt, GPT-4 shows decent performance for both entity extraction and relation extraction tasks. Nevertheless, the evaluation result also reveals that the model is not perfect. We observed inconsistent outputs (given a prompt) as well as prompt over-generalization.
- Pyarelal, A., Duong, E., Shibu, C. J., Soares, P., Boyd, S., Khosla, P., Pfeifer, V., Zhang, D., Andrews, E. S., Champlin, R., Raymond, V. P., Krishnaswamy, M., Morrison, C. T., Butler, E., & Barnard, K. (2023). The ToMCAT Dataset. In Advances in Neural Information Processing Systems 36 (NeurIPS 2023), Datasets and Benchmarks Track, 36.More infoAbstract:We present a rich, multimodal dataset consisting of data from 40 teams of three humans conducting simulated urban search-and-rescue (SAR) missions in a Minecraft-based testbed, collected for the Theory of Mind-based Cognitive Architecture for Teams (ToMCAT) project. Modalities include two kinds of brain scan data---functional near-infrared spectroscopy (fNIRS) and electroencephalography (EEG), as well as skin conductance, heart rate, eye tracking, face images, spoken dialog audio data with automatic speech recognition (ASR) transcriptions, game screenshots, gameplay data, game performance data, demographic data, and self-report questionnaires. Each team undergoes up to six consecutive phases: three behavioral tasks, one mission training session, and two collaborative SAR missions. As time-synchronized multimodal data collected under a variety of circumstances, this dataset will support studying a large variety of research questions on topics including teamwork, coordination, plan recognition, affective computing, physiological linkage, entrainment, and dialog understanding. We provide an initial public release of the de-identified data, along with analyses illustrating the utility of this dataset to both computer scientists and social scientists.
- KC, D., Morrison, C. T., & Walls, B. (2022). Texture Generation Using Graph Generative Adversarial Network And Differentiable Rendering. In Image and Vision Computing: 37th International Conference (IVCNZ), 2022.More infoNovel texture synthesis for existing 3D mesh models is an important step towards photo realistic asset generation for existing simulators. But existing methods inherently work in the 2D image space which is the projection of the 3D space from a given camera perspective. These methods take camera angle, 3D model information, lighting information and generate photorealistic 2D image. To generate a photorealistic image from another perspective or lighting, we need to make a computationally expensive forward pass each time we change the parameters. Also, it is hard to generate such images for a simulator that can satisfy the temporal constraints the sequences of images should be similar but only need to change the viewpoint of lighting as desired. The solution can not be directly integrated with existing tools like Blender and Unreal Engine. Manual solution is expensive and time consuming. We thus present a new system called a graph generative adversarial network (GGAN) that can generate textures which can be directly integrated into a given 3D mesh models with tools like Blender and Unreal Engine and can be simulated from any perspective and lighting condition easily.
- Kwak, A. S., Israelsen, J. O., Morrison, C. T., Bambauer, D. E., & Surdeanu, M. (2022). Validity Assessment of Legal Will Statements as Natural Language Inference. In Empirical Methods in Natural Language Processing (EMNLP), 2022.More infoThis work introduces a natural language inference (NLI) dataset that focuses on the validity of statements in legal wills. This dataset is unique because: (a) each entailment decision requires three inputs: the statement from the will, the law, and the conditions that hold at the time of the testator’s death; and (b) the included texts are longer than the ones in current NLI datasets. We trained eight neural NLI models in this dataset. All the models achieve more than 80% macro F1 and accuracy, which indicates that neural approaches can handle this task reasonably well. However, group accuracy, a stricter evaluation measure that is calculated with a group of positive and negative examples generated from the same statement as a unit, is in mid 80s at best, which suggests that the models’ understanding of the task remains superficial. Further ablative analyses and explanation experiments indicate that all three text segments are used for prediction, but some decisions rely on semantically irrelevant tokens. This indicates that overfitting on these longer texts likely happens, and that additional research is required for this task to be solved.
- Liang, Z., Noriega-Atala, E., Morrison, C. T., & Surdeanu, M. (2022). Low Resource Causal Event Detection from Biomedical Literature. In Proceedings of the 21st Workshop on Biomedical Language Processing at ACL 2022.More infoAbstract Recognizing causal precedence relations among the chemical interactions in biomedical literature is crucial to understanding the underlying biological mechanisms. However, detecting such causal relation can be hard because: (1) many times, such causal relations among events are not explicitly expressed by certain phrases but implicitly implied by very diverse expressions in the text, and (2) annotating such causal relation detection datasets requires considerable expert knowledge and effort. In this paper, we propose a strategy to address both challenges by training neural models with in-domain pre-training and knowledge distillation. We show that, by using very limited amount of labeled data, and sufficient amount of unlabeled data, the neural models outperform previous baselines on the causal precedence detection task, and are ten times faster at inference compared to the BERT base model. Anthology ID:
- Noriega-Atala, E., Lovett, P. M., Morrison, C. T., & Surdeanu, M. (2022). Neural Architectures for Biological Inter-Sentence Relation Extraction. In AAAI-22 Workshop on Scientific Document Understanding (SDU@AAAI22, https://easychair.org/cfp/SDU_AAAI_22).More infoWe introduce a family of deep-learning architectures for inter-sentence relation extraction, i.e., relations where the participants are not necessarily in the same sentence. We apply these architectures to an important use case in the biomedical domain: assigning biological context to biochemical events. In this work, biological context is defined as the type of biological system within which the biochemical event is observed. The neural architectures encode and aggregate multiple occurrences of the same candidate context mentions to determine whether it is the correct context for a particular event mention. We propose two broad types of architectures: the first type aggregates multiple instances that correspond to the same candidate context with respect to event mention before emitting a classification; the second type independently classifies each instance and uses the results to vote for the final class, akin to an ensemble approach. Our experiments show that the proposed neural classifiers are competitive and some achieve better performance than previous state of the art traditional machine learning methods without the need for feature engineering. Our analysis shows that the neural methods particularly improve precision compared to traditional machine learning classifiers and also demonstrates how the difficulty of inter-sentence relation extraction increases as the distance between the event and context mentions increase.
- Noriega-Atala, E., Surdeanu, M., & Morrison, C. T. (2022). Learning Open Domain Multi-hop Search Using Reinforcement Learning. In NAACL 2022 Workshop SUKI: Structured and Unstructured Knowledge Integration,.More infoWe propose a method to teach an automated agent to learn how to search for multi-hop paths of relations between entities in an open domain. The method learns a policy for directing existing information retrieval and machine reading resources to focus on relevant regions of a corpus. The approach formulates the learning problem as a Markov decision process with a state representation that encodes the dynamics of the search process and a reward structure that minimizes the number of documents that must be processed while still finding multi-hop paths. We implement the method in an actor-critic reinforcement learning algorithm and evaluate it on a dataset of search problems derived from a subset of English Wikipedia. The algorithm finds a family of policies that succeeds in extracting the desired information while processing fewer documents compared to several baseline heuristic algorithms.
- Hendryx, S. M., Kc, D. R., Morrison, C. T., & Walls, B. (2021). Federated Reconnaissance: Efficient, Distributed, Class-Incremental Learning.. In Workshop on New Frontiers in Federated Learning: Privacy, Fairness, Robustness, Personalization and Data Ownership (https://neurips2021workshopfl.github.io/NFFL-2021/paper.html), held in conjunction with the Thirty-fifth Conference on Neural Information Processing Systems (NeurIPS), 2021.More infoWe describe federated reconnaissance, a class of learning problems in which distributed clients learn new concepts independently and communicate that knowledge efficiently. In particular, we propose an evaluation framework and methodological baseline for a system in which each client is expected to learn a growing set of classes and communicate knowledge of those classes efficiently with other clients, such that, after knowledge merging, the clients should be able to accurately discriminate between classes in the superset of classes observed by the set of clients. We compare a range of learning algorithms for this problem and find that prototypical networks are a strong approach in that they are robust to catastrophic forgetting while incorporating new information efficiently. Furthermore, we show that the online averaging of prototype vectors is effective for client model merging and requires only a small amount of communication overhead, memory, and update time per class with no gradient-based learning or hyperparameter tuning. Additionally, to put our results in context, we find that a simple, prototypical network with four convolutional layers significantly outperforms complex, state of the art continual learning algorithms, increasing the accuracy by over 22% after learning 600 Omniglot classes and over 33% after learning 20 mini-ImageNet classes incrementally. These results have important implications for federated reconnaissance and continual learning more generally by demonstrating that communicating feature vectors is an efficient, robust, and effective means for distributed, continual learning.
- Alexeeva, M., Sharp, R., Valenzuela, M. A., Kadowaki, J., Pyarelal, A., & Morrison, C. T. (2020, Spring). MathAlign: Linking Formula Identifiers to their Contextual Natural Language Descriptions. In 12th Edition of the Language Resources and Evaluation Conference (LREC).
- Hendryx, S. M., Leach, A. B., Hein, P. D., & Morrison, C. T. (2020, Fall). Meta-Learning Initializations for Image Segmentation. In Workshop on Meta-Learning (MetaLearn 2020; https://meta-learn.github.io/2020/) held in conjunction with the Thirty-fourth Conference on Neural Information Processing Systems (NeurIPS), 2020.
- Noriega-Atala, E., Liang, Z., Bachman, J., Morrison, C. T., & Surdeanu, M. (2019, Summer). Understanding the Polarities of Events in the Biomedical Literature: Deep Learning vs. Linguistically-informed Methods. In Workshop on Extracting Structured Knowledge from Scientific Publications (ESSP).
- Pyarelal, A., Sharp, R., Morrison, C. T., & Barnard, J. J. (2019, May). Interpreting Causal Expressions with Gradable Adjectives to Assembly Dynamics Models. In Modeling the World's Systems.
- Pyarelal, A., Valenzuela-Escárcega, M. A., Sharp, R., Hein, P. D., Stephens, J., Bhandari, P., Lim, H., Debray, S., & Morrison, C. T. (2019, May). AutoMATES: Automated Model Assembly from Text, Equations, and Software. In Modeling the World's Systems.More infoModels of complicated systems can be represented in different ways - inscientific papers, they are represented using natural language text as well asequations. But to be of real use, they must also be implemented as software,thus making code a third form of representing models. We introduce theAutoMATES project, which aims to build semantically-rich unifiedrepresentations of models from scientific code and publications to facilitatethe integration of computational models from different domains and allow formodeling large, complicated systems that span multiple domains and levels ofabstraction.
- Sharp, R., Pyarelal, A., Gyori, B. M., Alcock, K., Laparra, E., Valenzuela-Escárcega, M. A., Nagesh, A., Yadav, V., Bachman, J. A., Tang, Z., Lent, H., Luo, F., Paul, M., Bethard, S. J., Barnard, J. J., Morrison, C. T., & Surdeanu, M. (2019). Eidos & Delphi: From Free Text to Executable Causal Models. In Modeling the World’s Systems, 2019.
- Sharp, R., Pyarelal, A., Gyori, B. M., Alcock, K., Laparra, E., Valenzuela-Escárcega, M. A., Nagesh, A., Yadav, V., Bachman, J. A., Tang, Z., Lent, H., Luo, F., Paul, M., Bethard, S. J., Barnard, J. J., Morrison, C. T., & Surdeanu, M. (2019, Summer). Eidos, INDRA & Delphi: From Free Text to Executable Causal Models. In Annual Conference of the North American Chapter of the Association for Computational Linguistics (NAACL).
- Jansen, P. A., Wainwright, E., Marmorstein, S., & Morrison, C. T. (2018, May). WorldTree: A Corpus of Explanation Graphs for Elementary Science Questions supporting Multi-hop Inference. In Language Resources and Evaluation Conference (LREC).
- Noriega-Atala, E., Hein, P. D., Thumsi, S. S., Wong, Z., Wang, X., & Morrison, C. T. (2018, September). Inter-sentence Relation Extraction for Associating Biological Context with Events in Biomedical Texts. In The Sixth Workshop on Data Mining in Biomedical Informatics and Healthcare, held in conjunction with the IEEE International Conference on Data Mining (DMBIH@ICDM’18).
- Dawson, C. R., Huang, C., & Morrison, C. T. (2017, August). An infinite hidden Markov model with similarity-based transitions. In The Thirty-Fourth International Conference on Machine Learning (ICML 2017).More infoShort paper at competitive conference, double-blind review with acceptance rate of 25.46% (433 accepted out of 1701 submissions)
- Noriega-Atala, E., Valenzuela-Escárcega, M. A., Morrison, C. T., & Surdeanu, M. (2017, August). Focused Reading: Reinforcement Learning for What Documents to Read. In ICML 2017 Workshop on Interactive Machine Learning and Semantic Information Retrieval (IMLSIR@ICML 2017).
- Noriega-Atala, E., Valenzuela-Escárcega, M. A., Morrison, C. T., & Surdeanu, M. (2017, September). Learning what to read: Focused machine reading. In Conference on Empirical Methods in Natural Language Processing (EMNLP 2017).More infoShort paper at competitive conference, double-blind review with acceptance rate of 18% (107 accepted out of 582 submissions)
- Quick, D., & Morrison, C. T. (2017, October). Composition by Conversation. In The Forty-Third International Computer Music Conference (ICMC 2017).More infoFull paper at competitive conference, double-blind review.
- Valenzuela-Escárcega, M. A., Babur, Ö., Hahn-Powell, G., Bell, D., Hicks, T., Noriega-Atala, E., Wang, X., Surdeanu, M., Demir, E., & Morrison, C. T. (2017, September). Large-scale Automated Reading with Reach Discovers New Cancer Driving Mechanisms. In BioCreative VI Workshop (BioCreative6 2017).
- Brau, E., Dawson, C. R., Carillo, A., Sidi, D., & Morrison, C. T. (2016, February). Bayesian inference of recursive sequences of group activities from tracks. In The Thirteenth AAAI Conference on Artificial Intelligence (AAAI 2016).More infoFull paper at competitive conference, double-blind review with acceptance rate of 26% (549 accepted out of 2132 submissions)
- Gorji-Sefidmazgi, M., & Morrison, C. T. (2016, September). Spatiotemporal analysis of seasonal precipitation over US using co-clustering. In The 6th International Workshop on Climate Informatics (IC 2016).
- Guan, J., Simek, K., Brau, E., Morrison, C. T., Butler, E. A., & Barnard, J. J. (2016). Bayesian generative modeling for complex dynamical systems. In The 11th Annual Women in Machine Learning Workshop (WiML), 2016.
- Shiaun Peng, K., & Morrison, C. T. (2016, July). Model predictive prior reinforcement learning for a heat pump thermostat. In The 11th International Workshop on Feedback Computing (FC 2016).
- Dykhuis, N. J., Rossi, F., & Morrison, C. T. (2015, June). Contributions to Teams Formed in Dynamic Networks. In The Multi-disciplinary Conference on Reinforcement Learning and Decision Making (RLDM 2015).
- Guan, J., Brau, E., Simek, K., Morrison, C. T., Butler, E. A., & Barnard, K. J. (2015, July). Moderated and Drifting Linear Dynamical Systems. In Proceedings of the Thirty-second International Conference on Machine Learning (ICML 2015).More infoThis venue is a peer reviewed, competitive conference (acceptance rate: 26%) and the full paper is published as part of the conference proceedings.Abstract We consider linear dynamical systems, particularly coupled linear oscillators, where the parameters represent meaningful values in a domain theory and thus learning what affects them contributes to explanation. Rather than allow perturbations of latent states, we assume that temporal variation beyond noise is explained by parameter drift, and variation across coupled systems is a function of moderating variables. This change of focus reduces opportunities for efficient inference, and we propose sampling procedures to learn and fit the models. We test our approach on a real dataset of physiological measures of heterosexual couples engaged in a conversation about a potentially emotional topic, with body mass index (BMI) being considered as a moderator. We evaluate several models on their ability to predict future conversation dynamics (the last 20% of the data for each test couple), with shared parameters being learned using held out data. As proof of concept, we validate the hypothesis that BMI affects the conversation dynamic in the experimentally chosen topic.
- Hamilton, C. W., Palafox, L. F., & Morrison, C. T. (2015, June-July). Automated detection of geologic changes on Mars using Bayesian models. In International Union of Geodesy and Geophysics, General Assembly, 26.
- Dawson, C. R., Del Pero, L., Morrison, C. T., Surdeanu, M., Hahn-Powell, G., Chapman, Z., & Barnard, K. (2013). Bayesian Modeling of Scenes and Captions. In The 2013 Conference of the North American Chapter of the Association for Computational Linguistics: Human Language Technologies Workshop on Vision and Language (WVL@NAACL-HLT), 2013.
- Kaochar, T., Peralta, R. T., Morrison, C. T., Walsh, T. J., Fasel, I., Beyon, S., Tran, A., Wright, J. B., & Cohen, P. R. (2011). Human Natural Instruction of a Simulated Electronic Student. In AAAI Spring Symposium Series Workshop: Help Me Help You: Bridging the Gaps in Human-Agent Collaboration, 2011.More infoHumans naturally use multiple modes of instruction while teaching one another. We would like our robots and artificial agents to be instructed in the same way, rather than programmed. In this paper, we review prior work on human instruction of autonomous agents and present observations from two exploratory pilot studies and the results of a full study investigating how multiple instruction modes are used by humans. We describe our Bootstrapped Learning User Interface, a prototype multi-instruction interface informed by our human-user studies.
- Kaochar, T., Torres Peralta, R., Morrison, C. T., Fasel, I. R., Walsh, T. J., & Cohen, P. R. (2011). Towards understanding how humans teach robots. In Proceedings of 19th Conference on User Modeling, Adaptation and Personalization (UMAP), 2011.More infoOur goal is to develop methods for non-experts to teach complex behaviors to autonomous agents (such as robots) by accommodating "natural" forms of human teaching. We built a prototype interface allowing humans to teach a simulated robot a complex task using several techniques and report the results of 44 human participants using this interface. We found that teaching styles varied considerably but can be roughly categorized based on the types of interaction, patterns of testing, and general organization of the lessons given by the teacher. Our study contributes to a better understanding of human teaching patterns and makes specific recommendations for future human-robot interaction systems. © 2011 Springer-Verlag.
- Torres Peralta, R., Kaochar, T., Fasel, I. R., Morrison, C. T., Walsh, T. J., & Cohen, P. R. (2011). Challenges to Decoding the Intenttion Behind Natural Instruction. In IJCAI 2011 Workshop on Agents Learning Interactively from Human Teachers (ALIHT).More infoAbstract: Currently, most systems for human-robot teaching allow only one mode of teacher-student interaction (e.g., teaching by demonstration or feedback), and teaching episodes have to be carefully set-up by an expert. To understand how we might integrate multiple, interleaved forms of human instruction into a robot learner, we performed a behavioral study in which 44 untrained humans were allowed to freely mix interaction modes to teach a simulated robot (secretly controlled by a human) a complex task. Analysis of transcripts showed that human teachers often give instructions that require considerable interpretation and are not easily translated into a form useable by machine learning algorithms. In particular, humans often use implicit instructions, fail to clearly indicate the boundaries of procedures, and tightly interleave testing, feedback, and new instruction. In this paper, we detail these teaching patterns and discuss the challenges they pose to automatic teaching interpretation as well as the machine-learning algorithms that must ultimately process these instructions. We highlight the challenges by demonstrating the difficulties of an initial automatic teacher interpretation system.
- Torres Peralta, R., Kaochar, T., Fasel, I. R., Morrison, C. T., Walsh, T. J., & Cohen, P. R. (2011). Challenges to decoding the intention behind natural instruction. In Proceedings of the 20th IEEE International Symposium on Robot and Human Interactive Communication (Ro-Man), 2011.More infoCurrently, most systems for human-robot teaching allow only one mode of teacher-student interaction (e.g., teaching by demonstration or feedback), and teaching episodes have to be carefully set-up by an expert. To understand how we might integrate multiple, interleaved forms of human instruction into a robot learner, we performed a behavioral study in which 44 untrained humans were allowed to freely mix interaction modes to teach a simulated robot (secretly controlled by a human) a complex task. Analysis of transcripts showed that human teachers often give instructions that are nontrivial to interpret and not easily translated into a form useable by machine learning algorithms. In particular, humans often use implicit instructions, fail to clearly indicate the boundaries of procedures, and tightly interleave testing, feedback, and new instruction. In this paper, we detail these teaching patterns and discuss the challenges they pose to automatic teaching interpretation as well as the machine-learning algorithms that must ultimately process these instructions. We highlight the challenges by demonstrating the difficulties of an initial automatic teacher interpretation system. © 2011 IEEE.
- Walsh, T. J., Hewlett, D., & Morrison, C. T. (2011). Blending Autonomous and Apprenticeship Learning. In Robots: Science and Systems (RSS), Workshop on Imitation Learning, 2011.
- Walsh, T. J., Morrison, C. T., & Hewlett, D. (2011). Blending Autonomous Exploration and Apprenticeship Learning. In Advances in Neural Information Processing Systems 24 (NIPS 2011), 24, 2258-2266.More infoWe present theoretical and empirical results for a framework that combines the benefits of apprenticeship and autonomous reinforcement learning. Our approach modifies an existing apprenticeship learning framework that relies on teacher demonstrations and does not necessarily explore the environment. The first change is replacing previously used Mistake Bound model learners with a recently proposed framework that melds the KWIK and Mistake Bound supervised learning protocols. The second change is introducing a communication of expected utility from the student to the teacher. The resulting system only uses teacher traces when the agent needs to learn concepts it cannot efficiently learn on its own.
- Fasel, I. R., Wilt, A., Mafi, N., & Morrison, C. T. (2010). Intrinsically motivated information foraging. In International Conference on Development and Learning (ICDL), 2010.More infoWe treat information gathering as a POMDP in which the goal is to maximize an accumulated intrinsic reward at each time step based on the negative entropy of the agent's beliefs about the world state. We show that such information foraging agents can discover intelligent exploration policies that take into account the long-term effects of sensor and motor actions, and can automatically adapt to variations in sensor noise, different amounts of prior information, and limited memory conditions. © 2010 IEEE.
- Morrison, C. T., Bryce, D., Fasel, I. R., & Rebguns, A. (2009). Augmenting Instructable Computing with Planning Technology. In ICAPS'09 Workshop on the International Competition for Knowledge Engineering in Planning and Scheduling (ICKEPS), 2009.
- Villegas, J. C., Morrison, C. T., Gerst, K. L., Beal, C. R., & Quirós, A. (2009). The partitioning of evapotranspiration into evaporation and transpiration: an experimental design assessing the effects of changes in vegetation cover. In Ecological Society of America (ESA)’s Digital Teaching Library (EcoEd), Millennium Conference 2009: Drought & Water-Ecosystem Services Teaching Collection, 2009.
- Chang, Y., Cohen, P. R., & Morrison, C. T. (2007). Learning and transferring action schemas. In Proceedings of Twentieth International Joint Conference on Artificial Intelligence (IJCAI), 720-725.More infoJean is a model of early cognitive development based loosely on Piaget's theory of sensorimotor and pre-operational thought. Like an infant, Jean repeatedly executes schemas, gradually transferring them to new situations and extending them as necessary to accommodate new experiences. We model this process of accommodation with the Experimental State Splitting (ESS) algorithm. ESS learns elementary action schemas, which comprise controllers and maps of the expected dynamics of executing controllers in different conditions. ESS also learns compositions of action schemas called gists. We present tests of the ESS algorithm in three transfer learning experiments, in which Jean transfers learned gists to new situations in a real time strategy military simulator.
- Morrison, C. T., & Cohen, P. R. (2007). Designing Experiments to Test Planning Knowledge about Plan-step Order Constraints. In Proceedings of the Workshop on Artificial Intelligence Planning and Learning, in conjunction with the International Conference on Automated Planning and Scheduling (ICAPS-07), 2007.More infoA number of techniques have been developed to effectively extract and generalize planning knowledge based on expert demonstration. In this paper we consider a complementary approach to learning in which we may execute experiments designed to test hypothesized planning knowledge. In particular, we describe an algorithm that automatically generates experiments to test assertions about plan-step ordering, under the assumption that order constraints between two steps are independent of other step orderings. Experimenting with plan-step ordering can help identify asserted ordering constraints that are in fact not necessary, as well as uncover necessary ordering constraints not represented previously. The algorithm consists of three parts: identifying the space of step-ordering hypotheses, efficiently generating ordering tests, and planning experiments that use the tests to identify order constraints that are not currently represented. This method is implemented in the CMAX experiment design module and is part of the POIROT integrated learning system.
- Morrison, C. T., & Cohen, P. R. (2007). Designing experiments to test and improve hypothesized planning knowledge derived from demonstration. In Proceedings of the 2007 AAAI Workshop on Acquiring Planning Knowledge via Demonstration. AAAI Press, Technical Report WS-07-02, 2007.More infoA number of techniques have been developed to effectively extract and generalize planning knowledge based on expert demonstration. In this paper we consider a complementary approach to learning in which experiments are designed to test hypothesized planning knowledge. In particular, we describe an algorithm that automatically generates experiments to test assertions about plan-step ordering. Experimenting with plan-step ordering can identify asserted ordering constraints that are in fact not necessary, as well as uncover necessary ordering constraints previously not represented. The algorithm consists of three parts: identifying the space of step-ordering hypotheses, efficiently generating ordering tests, and planning experiments that use the tests to identify order constraints that are not currently represented. This method is implemented in the CMAX experiment design module and is part of the POIROT integrated learning system. We discuss the role of experimentation in planning knowledge refinement and some future directions for CMAX’s development.
- Amant, R. S., Morrison, C. T., Chang, Y., Mu, W., Cohen, P. R., & Beal, C. R. (2006). An Image Schema Language. In Proceedings of the International Conference on Cognitive Modeling (ICCM).More infoAbstract : This paper introduces ISL, a language for representing and manipulating image schemas. ISL supports the representation of symbolic as well as quantitative dynamic properties of objects and relationships. We have encoded a number of the image schemas commonly covered in the cognitive linguistics literature and tested them in three domains: patterns in chess, tactics in military scenarios, and behavior in a simple robot arm simulation. This paper discusses the design of the language and demonstrates its representational capabilities with examples from these domains.
- Chang, Y., Cohen, P., Morrison, C., Amant, R., & Beal, C. (2006). Piagetian adaptation meets image schemas: The jean system. In From Animals to Animats 9.More infoJean is a model of early cognitive development based loosely on Piaget's theory of sensori-motor and pre-operational thought [1]. Like an infant, Jean repeatedly executes schemas, gradually extending its schemas to accommodate new experiences. Jean's environment is a simulated "playpen" in which Jean and other objects move about and interact. Jean's cognitive development depends on several integrated functions: a simple perceptual system, an action-selection system, a motivational system, a long-term memory, and learning methods. This paper provides an overview of Jean's architecture and schemas, and it focuses on how Jean learns schemas and transfers them to new situations. © Springer-Verlag Berlin Heidelberg 2006.
- Chang, Y., Morrison, C. T., Kerr, W., Galstyan, A., Cohen, P. R., Beal, C., St. Amant, R., & Oates, T. (2006). The Jean System. In Proceedings of the 5th International Conference on Development and Learning (ICDL), 2006.
- Cohen, P. R., & Morrison, C. T. (2006). The Hats Information Fusion Challenge Problem. In 2006 9th International Conference on Information Fusion, 1-7.More infoWe describe the Hats Simulator as an information fusion challenge problem. Hats is a virtual world in which many agents engage in individual and collective activities. Most agents are benign, some intend harm. Agent activities are planned by a generative planner. Playing against the simulator, the goal of the analyst is to identify and arrest harmful agents before they carry out their plans. The simulator provides both scalar and categorical information. Information fusion tasks in the Hats domain include assessing information value, choosing information collection strategies, tracking individuals and resources, identifying events, hypothesizing group membership, ascribing suspicion, and identifying plans. After each game, the analyst is assessed a set of scores including the cost of acquiring information, the cost of falsely accusing benign agents, and the cost of failing to detect harmful agents. The simulator is implemented and currently manages hundreds of thousands of agents.
- Morrison, C. T., Chang, Y., Cohen, P. R., & Moody, J. (2006). Experimental State Splitting for Transfer Learning. In Proceedings of the ICML 2006 Workshop on Structural Knowledge Transfer for Machine Learning (ICML SKTML), 2006.
- Morrison, C., & Cohen, P. (2006). The Colab mixed-initiative analysis enviroment. In Proceedings of the 9th International Conference on Information Fusion (FUSION-06), 2006.More infoCOLAB is an analysis environment in which multiple human analysts in different physical locations can collaborate to build hypotheses of un-folding scenarios. COLAB consists of two components: an instrumented analysis working environment built on a blackboard system, and a web-based user interface that integrates the Trellis hypothesis authoring and management tool with a query language. On the blackboard, analysts collect data and perform analyses using relational database queries and a set of analysis tools that include group identification and suspicion scoring algorithms. Evidence extracted from data analysis on the blackboard may then be incorporated in hierarchical argument structures in the Trellis tool, combining evidence sources with user-supplied free text. Trellis arguments may then be shared between analysts and collaboratively authored. COLAB has been integrated with the Hats Simulator challenge domain and serves as a prototype mixed-initiative information fusion system.
- Morrison, C., & Cohen, P. (2006). The hats simulator and colab: An integrated information fusion challenge problem and collaborative analysis environment. In Proceedings of the IEEE International Conference on Intelligence and Security Informatics (ISI 2006), published in the Springer Lecture Notes in Computer Sciences (LNCS), number 3975.More infoWe present an overview of our work in information fusion for intelligence analysis. This work includes the Hats Simulator and the COLAB system. The Hats Simulator is a parameterized model of a virtual world in which hundreds of thousands of agents engage in individual and collective activities. Playing against the simulator, the goal of the analyst is to identify and stop harmful agents before they carry out terrorist attacks on simulated landmarks. The COLAB system enables multiple human analysts in different physical locations to conduct collaborative intelligence analysis. COLAB consists of two components: an instrumented analysis working environment built on a blackboard system, and a web-based user interface that integrates the Trellis hypothesis authoring and management tool with a query language. COLAB is integrated with the Hats Simulator to provide a complete end-to-end analysis environment with challenging problem domain. © Springer-Verlag Berlin Heidelberg 2006.
- Cannon, E. N., Cohen, P. R., & Morrison, C. T. (2005). Maps for Verbs: the Relation Between Interaction Dynamics and Verb Use. In Proceedings of the Nineteenth International Conference on Artificial Intelligence (IJCAI), 1022-1027.More infoWe report a study of word meaning that tests whether dynamical aspects of movies predict word use. The movies were based on a novel representation of verb semantics called maps for verbs. We asked preschool-school-age children to describe the movies, and demonstrated that their distributions of words could be predicted by the dynamical aspects of the movies. These results lend support to the empiricist position that word meanings are learned associatively.
- Cohen, P. R., & Morrison, C. T. (2005). COLAB: A Laboratory Environment for Studying Analyst Sensemaking and Collaboration. In Proceedings of the Tenth International Command and Control Research and Technology Symposium (10th ICCRTS), 2005.More infoAbstract : COLAB is a laboratory for studying tools that facilitate collaboration and sensemaking among groups of human analysts as they build interpretations of unfolding situations based on accruing intelligence data. The laboratory has three components. The Hats Simulator provides a challenging problem domain involving thousands to millions of agents engaged in individual and collective behaviors, a small portion of which are terrorist. The second component, the AIID Bayesian blackboard, is an instrumented working environment within which analysts collaborate to build their interpretation of the problem domain. The third component is a web based user interface that integrates the Trellis hypothesis authoring and management tool with a query language to allow human analysts to interact with AIID and each other. Looking to the big picture, COLAB is not merely a laboratory for studying collaboration and shared knowledge creation. COLAB is a tool to explore and develop the analyst working environment of the future, in which analyst tools and methods for collaboration in edge organizations are developed and tested. We present COLAB and its components and outline our plans for the system.
- Hannon, A., King, G., Morrison, C., Galstyan, A., & Cohen, P. (2005). Population generation for large-scale simulation. In Proceedings Volume 5805, Enabling Technologies for Simulation Science IX, 2005.More infoComputer simulation is used to research phenomena ranging from the structure of the space-time continuum to population genetics and future combat.1-3 Multi-agent simulations in particular are now commonplace in many fields.4, 5 By modeling populations whose complex behavior emerges from individual interactions, these simulations help to answer questions about effects where closed form solutions are difficult to solve or impossible to derive.6 To be useful, simulations must accurately model the relevant aspects of the underlying domain. In multi-agent simulation, this means that the modeling must include both the agents and their relationships. Typically, each agent can be modeled as a set of attributes drawn from various distributions (e.g., height, morale, intelligence and so forth). Though these can interact - for example, agent height is related to agent weight - they are usually independent. Modeling relations between agents, on the other hand, adds a new layer of complexity, and tools from graph theory and social network analysis are finding increasing application.7, 8 Recognizing the role and proper use of these techniques, however, remains the subject of ongoing research. We recently encountered these complexities while building large scale social simulations.9-11 One of these, the Hats Simulator, is designed to be a lightweight proxy for intelligence analysis problems. Hats models a “society in a box” consisting of many simple agents, called hats. Hats gets its name from the classic spaghetti western, in which the heroes and villains are known by the color of the hats they wear. The Hats society also has its heroes and villains, but the challenge is to identify which color hat they should be wearing based on how they behave. There are three types of hats: benign hats, known terrorists, and covert terrorists. Covert terrorists look just like benign hats but act like terrorists. Population structure can make covert hat identification significantly more difficult. Investigators using the Hats Simulator must be able to control population parameters, and population generation must be fast enough to support studies that vary these parameters. This paper reports our experiences developing algorithms to generate populations whose structure is dependent on experimenter controlled parameters. In the next section, we outline the general problem of population generation and the details of building populations for the Hats Simulator. This is followed by a brief description of our initial, brute force algorithm (section 3). It was sufficient for small populations, but scaled horribly. We realized that the Law of Large Numbers would allow randomized methods to generate large populations that satisfied our constraints within acceptable bounds (section 5). It was while developing this algorithm that we discovered the connection between our population generation problem and recent results from the theory of random bipartite graphs (section 4). Finally, section 6 examines the properties and performance of our randomized algorithm. Though we will describe our results in terms of the Hats Simulator, these methods apply to any modeling situation in which populations of agents share multiple, overlapping structures, each of which is independent from the others. Our hope is that our experience will benefit others facing the task of generating large populations that require similar overlapping group structure.
- Morrison, C. T., Cohen, P. R., & King, G. W. (2005). Simulating Terrorist Threat in the Hats Simulator. In Proceedings of the First International Conference on Intelligence Analysis (IA), 2005.
- Morrison, C., & Cohen, P. (2005, August). Noisy information value in utility-based decision making. In UBDM '05: Proceedings of the 1st international workshop on Utility-based data mining, 34-38.More infoHow much is information worth? In the context of decisions, the value of information is the expected increase in utility of the decision as a result of having the information. When the information might be noisy, the model is slightly more complicated. We develop a model of the value of noisy information in the context of a plausible intelligence information gathering and decision making scenario. Copyright 2005 ACM.
- Adibi, J., Cohen, P. R., & Morrison, C. T. (2004). Measuring Confidence Intervals in Link Discovery: A Bootstrap Approach. In Proceedings of the ACM Special Interest Group on Knowledge Discovery and Data Mining (SIGKDD), 2004.More infoRecently there has been great interest in the development of information technology for Link Discovery (LD). LD is relevant to a wide range of research topics, including social network analysis, fraud detection, graph theory, pattern analysis and link analysis. The main goal of this research is the development of techniques for mining large amounts of data to find hidden patterns, extract valuable knowledge and discover hidden links among sparse pieces of evidence. In LD many such algorithms are deterministic and the constructed hypotheses are not qualified by probabilities. However, for nearly all applications the data available are sampled from a population. Hence, the discovered knowledge and implied hypotheses is probabilistic in nature and such uncertainty has to be measured. Due to the nature of the LD problems many of the current techniques and methods lack such measurement. We are interested in addressing this methodological problem and provide a general method for measuring the confidence intervals of hypotheses generated by LD algorithms. In this paper, we examine the bootstrap resampling method to measure the uncertainty in LD hypotheses. We study and analyze an example of of this method applied to the problem of discovering group membership, and discuss the effect such evaluation has on the generated hypotheses and their interpretation. Our preliminary results are encouraging and indicate that the bootstrap confidences are correlated with derived hypothesis.
- Adibi, J., Cohen, P. R., Moody, J., Morrison, C. T., & Sutton, C. (2004). A Bayesian Blackboard for Information Fusion. In Proceedings of the 7th International Conference on Information Fusion (FUSION-04), 2004.More infoAbstract : A Bayesian blackboard is just a conventional, knowledge-based blackboard system in which knowledge sources modify Bayesian networks on the blackboard. As an architecture for intelligence analysis and data fusion this has many advantages: the blackboard is a shared workspace or corporate memory for collaborating analysts; analyses can be developed over long periods of time with information that arrives in dribs and drabs; the computer's contribution to analysis can range from data-driven statistical algorithms up to domain-specific, knowledge-based inference; and perhaps most important, the control of intelligence-gathering in the world and inference on the blackboard can be rational, that is, grounded in probability and utility theory. The Bayesian blackboard architecture presented here, called AIID, serves both as a prototype system for intelligence analysis and as a laboratory for testing mathematical models of the economics of intelligence analysis.
- Cannon, E. N., Cohen, P. R., & Morrison, C. T. (2004). When push comes to shove: A study of the relation between interaction dynamics and verb use. In Working Notes of the AAAI Spring Symposium Workshop: Language Learning: An Interdisciplinary Perspective, 2004.More infoA significant portion of our language is devoted to referring to, expressing, and representing the temporally extended dynamics of our world. Following Tomasello (1992), we label the class of words used to refer to such dynamics as verbs. Cohen (1998) presents a framework for distinguishing interactions involving whole-body objects by considering the dynamics of the before, during (contact), and after phases of interaction. These phases are characterized using dynamic maps to plot various measures of the physical interaction. The maps-for-verbs framework proposes that a representation based on the dynamics of before, during and after interaction are a foundation for the semantics of verbs describing physical interactions between objects. Here we report a preliminary study of the use of this framework to predict the verb use of adults describing simple whole-body interactions.
- Cohen, P. R., & Morrison, C. T. (2004). The Hats Simulator. In Proceedings of the Winter Simulation Conference (WSC-04), 1, 849-856.More infoThe Hats Simulator is designed to be a lightweight proxy for many intelligence analysis problems, and thus a test environment for analysts' tools. It is a virtual world in which many agents engage in individual and collective activities. Most agents are benign, some intend harm. Agent activities are planned by a generative planner. Playing against the simulator, the job of the analyst is to find harmful agents before they carry out their plans. The simulator maintains information about all agents. However, information is hidden from the analyst and some is expensive. After each game, the analyst is assessed a set of scores including the cost of acquiring information about agents, the cost of falsely accusing benign agents, and the cost of failing to detect harmful agents. The simulator is implemented and currently manages the activities of up to one hundred thousand agents.DOI: 10.1109/WSC.2004.1371399
- Barker, K., Blythe, J., Borchardt, G., Chaudhri, V. K., Clark, P., Cohen, P. R., Fitzgerald, J., Gil, Y., Katz, B., Kim, J., King, G., Mishra, S., Morrison, C. T., Murray, K., Porter, B., Schrag, R. C., Uribe, T. E., Usher, J., & Yeh, P. Z. (2003). A Knowledge Acquisition Tool for Course of Action Analysis. In Proceedings of Innovative Applications of Artificial Intelligence (IAAI), 2003, 15.More infoWe present the novel application of a general-purpose knowledge-based system, SHAKEN, to the specific task of acquiring knowledge for military Course of Action (COA) analysis. We show how SHAKEN can capture and reuse expert knowledge for COA critiquing, which can then be used to produce high-level COA assessments through declarative inference and simulation. The system has been tested and evaluated by domain experts, and we report on the results. The generality of the approach makes it applicable to task analysis and knowledge capture in other domains. The primary objective of this work is to demonstrate the application of the knowledge acquisition technology to the task of COA analysis. Developing a system deployable in an operational environment is the subject of future work.
- Burns, B. P., & Morrison, C. T. (2003). Temporal Abstraction in Bayesian Networks. In Working Notes of AAAI Spring Symposium Workshop: Foundation and Applications of Spatio-Temporal Reasoning (FASTR), 2003.More infoA current popular approach to representing time in Bayesian belief networks is through Dynamic Bayesian Networks (DBNs) (Dean & Kanazawa 1989). DBNs connect sequences of entire Bayes networks, each representing a situation at a snapshot in time. We present an alternative method for incorporating time into Bayesian belief networks that utilizes abstractions of temporal representation. This method maintains the principled Bayesian approach to reasoning under uncertainly, providing explicit representation of sequence and potentially complex temporal relationships, while also decreasing overall network complexity compared to DBNs.
- Burns, B., Sutton, C., Morrison, C. T., & Cohen, P. R. (2003). Information Theory and Representation in Associative Word Learning. In Proceedings of the Third International Workshop on Epigenetic Robotics (EpiRob), 2003.More infoA significant portion of early language learning can be viewed as an associative learning problem. We investigate the use of associative language learning based on the principle that words convey Shannon information about the environment. We discuss the shortcomings in representation used by previous associative word learners and propose a functional representation that not only denotes environmental categories, but serves as the basis for activities and interaction with the environment. We present experimental results with an autonomous agent acquiring language.
- Cohen, P. R., King, G. W., & Morrison, C. T. (2003, December). Action Models. In Proceedings of the 35th Winter Simulation Conference: Driving Innovation, 944-951.More infoBuilding military simulations requires bridging the gap between the knowledge of commanders and computer representations of that knowledge. A significant part of this knowledge concerns military tasks, their interactions, and an understanding of how to grade their achievement. Action Models describe the complex spatial and temporal dynamics of goal directed tasks with a graphical notation. Commanders can understand the notation and Knowledge Engineers can convert it into declarative or procedural forms. The conversion makes possible automated After Action reviews of plans writen in terms of these tasks (Center for Army Lessons Learned (CALL) 1993). We describe Action Models, their conversion into Tapir, a declarative executable action language, and their use in the DARPA Rapid Knowledge Formation (RKF) Program.
- King, G., Morrison, C., Westbrook, D., & Cohen, P. (2003). Bridging the gap: Simulations meet Knowledge Bases. In Proceedings Volume 5091, Enabling Technologies for Simulation Science VII, 5091.More infoTapir and Krill are declarative languages for specifying actions and agents, respectively, that can be executed in simulation. As such, they bridge the gap between strictly declarative knowledge bases and strictly executable code. Tapir and Krill components can be combined to produce models of activity which can answer questions about mechanisms and processes using conventional inference methods and simulation. Tapir was used in DARPA's Rapid Knowledge Formation (RKF) project to construct models of military tactics from the Army Field Manual FM3-90. These were then used to build Courses of Actions (COAs) which could be critiqued by declarative reasoning or via Monte Carlo simulation. Tapir and Krill can be read and written by non-knowledge engineers making it an excellent vehicle for Subject Matter Experts to build and critique knowledge bases.
- Sutton, C., Burns, B., Morrison, C., & Cohen, P. (2003). Guided incremental construction of belief networks. In Advances in Intelligent Data Analysis V. IDA 2003. Lecture Notes in Computer Science, vol 2810, 2810.More infoBecause uncertain reasoning is often intractable, it is hard to reason with a large amount of knowledge. One solution to this problem is to specify a set of possible models, some simple and some complex, and choose which to use based on the problem. We present an architecture for interpreting temporal data, called AIID, that incrementally constructs belief networks based on data that arrives asynchronously. It synthesizes the opportunistic control of the blackboard architecture with recent work on constructing belief networks from fragments. We have implemented this architecture in the domain of military analysis. © Springer-Verlag Berlin Heidelberg 2003.
- Morrison, C. T., Cohen, P. R., & Sebastiani, P. (2002). On the development of visual object memory: the stay/go decision problem. In Proceedings 2nd International Conference on Development and Learning. ICDL 2002, 239-244.More infoA developing memory requires a mechanism for deciding how much information to gather, based on what is currently represented in memory. That is, we need to know when we have seen enough to say we have or have not seen this before, or that we need to continue collecting data. We present a statistical approach to this decision mechanism. This serves as the foundation for a simple visual object memory. We present results from simulations showing that the statistical measure can serve as the basis of the stay/go decision process.
- Morrison, C. T., Oates, T., & King, G. (2001). Grounding the Unobservable in the Observable: The Role and Representation of Hidden State in Concept Formation and Refinement. In Working Notes of AAAI Spring Symposium Workshop: Learning Grounded Representations (AAAI SSS LGR).More infoAbstract : One of the great mysteries of human cognition is how we learn to discover meaningful and useful categories and concepts about the world. Why do very young children acquire concepts like rather than with red and green dots? One answer to this question is that categories are created, refined and maintained to support accurate prediction. Knowing that an entity is animate is generally more useful for the purpose of predicting how it will behave than knowing that it is blue with red and green dots. The idea of using predictability, or a lack thereof, as the driving force behind the creation and refinement of knowledge structures has been applied in a variety of context. Virtually all of the work in this vein is based on two key assumptions. First, an assumption is made that the world is in principle deterministic; that given enough knowledge, outcomes can be predicted. Given this, an agent's failure to predict implies that it is either missing information or incorrectly representing information. Second, it is assumed that knowledge structures sufficient for the task can be created by combining raw perceptual information in various ways. That is, everything the agent needs to make accurate predictions is available in its percepts, and the problem facing the agent is to find the right combination of elements. Our position is that the first of these assumptions represents a useful mechanism for driving unsupervised concept acquisition, whereas blind adherence to the second makes it difficult or impossible to discover some of the most fundamental concepts. To explain observed phenomena, scientists often posit the existence of unobservable entities. No one has ever seen gravity or black holes, but they explain a wide range of observable phenomena. Scientific progress would come to a standstill if not for the ability to posit and collect evidence for the existence of causally efficacious entities that do not manifest themselves directly in our percepts.
- Land, W. H., Land, J. W., Lo, J. Y., & Morrison, C. T. (2000, June). Evolutionary programming technique for reducing complexity of artifical neural networks for breast cancer diagnosis. In Proceedings Volume 3979, Medical Imapaging 2000: Image Processing, 3979, 153-158.More infoAn evolutionary programming (EP) technique was investigated to reduce the complexity of artificial neural network (ANN) models that predict the outcome of mammography-induced breast biopsy. By combining input variables consisting of mammography lesion descriptors and patient history data, the ANN predicted whether the lesion was benign or malignant, which may aide in reducing the number of unnecessary benign biopsies and thus the cost of mammography screening of breast cancer. The EP has the ability to optimize the ANN both structurally and parametrically. An EP was partially optimized using a data set of 882 biopsy-proven cases from Duke University Medical Center. Although many different architectures were evolved, the best were often perceptrons with no hidden nodes. A rank ordering of the inputs was performed using twenty independent EP runs. This confirmed the predictive value of the mass margin and patient age variables, and revealed the unexpected usefulness of the history of previous breast cancer. Further work is required to improve the performance of the EP over all cases in general and calcification cases in particular.
- Land, W. H., Lo, J. Y., & Morrison, C. T. (1999, July). Application of evolutionary programming and probabilistic neural networks to breast cancer diagnosis. In IJCNN'99. International Joint Conference on Neural Networks. Proceedings (Cat. No.99CH36339), 5, 3712-3716.More infoTwo novel artificial neural network techniques, evolutionary programming (EP) and probabilistic neural networks (PNN), were applied to the problem of breast cancer diagnosis. The EP is a stochastic optimization technique with the ability to mutate both network connections and weight values. The PNN has the ability to produce optimal Bayesian decision making given sufficient training data. Both techniques offer potential improvements over the well-studied, classic backpropagation networks. Preliminary performances of these new techniques were comparable to but slightly worse than the classic networks. In on-going work, these new techniques will be optimized further and should produce results greater than or equal to the classic networks, but with more information content and confidence.
- Morrison, C. T. (1996). Conceptual Change as Change of Inner Perspective. In Working Notes of the 1996 AAAI Fall Symposium Workshop: Embodied Cognition & Action.More infoTo be a genuinely useful methodology for cognitive psychology, situated action must be extended to explain higher-level human cognition. Yet there is a tension between the requirements for cognitive explanations and the kind of explanations situated action supplies. It is just not clear how to useembodied interaction with an external environment to explain processes that are so internal and that seem to crucially involve representations and concepts. In short, there is nothing for concepts to interact with. Perhaps they interact with each other. This is the idea we explore here. We give a situated action style explanation for conceptual change in the context of analogical reminding. We have two central results: 1) analogical reminding changes concepts in long-term memory, and 2) concepts have implicit as well as explicit structure.
- Morrison, C. T., & Dietrich, E. S. (1995). Structure-mapping vs. high-level perception: The mistaken fight over analogy making. In Proceedings of the Seventeenth Annual Conference of the Cognitive Science Society (CogSci), 1995.More infoThere is currently a competition between two theories that propose to explain the cognitive phenomenon of analogy: Dedre Gentner’s Structure-Mapping Theory and Douglas Hofstadter’s theory of Analogy as High-level Perception. We argue that the competition between the two theories is ill-founded because they are after two different aspects of analogy: structure-mapping seeks a “horizontal” view of analogy where the phenomena is examined at the level of already existing psychological representations, and where the task is to identify what processes are common to all or most analogy function; High-level Perception, on the other hand, seeks a “vertical” view of analogy in which the goal is to explain the processes that make up the construction of representations. An integrated theory of analogy should encompass both horizontal and vertical views.
Poster Presentations
- Hoogenboom, G., Morrison, C. T., Porter, C., Hein, P. D., & Pyarelal, A. (2020, Spring). Tools to Support Computational Crop Model Analysis and Comparison. The Second International Crop Modeling Symposium (iCROPM).
- Dudding, K. M., Carrington, J. M., & Morrison, C. T. (2019, Summer). Detecting Neonatal Pain Through the Connection of Neonate-Nurse Communication. Career, Connection, Community, Communicating Nursing Research. San Diego, CA.
- Hamilton, C. W., Palafox Novack, L. F., & Morrison, C. T. (2015, June-July). Automated detection of geologic changes on Mars using Bayesian models. The 26th General Assembly of the International Union of Geodesy and Geophysics (IUGG). Prague, the Czech Republic.
- Cannon, E. N., Morrison, C. T., & Cohen, P. R. (2005). “Bonk!” Children’s Spontaneous Production of Verbs for Object Interactions. 2005 Biennial Meeting of the Society for Research in Child Development (SRCD).