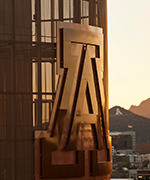
Jacobus J Barnard
- Professor, Computer Science
- Professor, Electrical and Computer Engineering
- Professor, BIO5 Institute
- Professor, Cognitive Science - GIDP
- Professor, Genetics - GIDP
- Professor, Statistics-GIDP
- Member of the Graduate Faculty
- Professor, Applied Mathematics - GIDP
Contact
- (520) 621-4632
- Gould-Simpson, Rm. 708
- Tucson, AZ 85721
- kobus@arizona.edu
Bio
No activities entered.
Interests
No activities entered.
Courses
2025-26 Courses
-
Dissertation
CSC 920 (Fall 2025) -
Intro To Computer Vision
CSC 477 (Fall 2025) -
Intro to Computer Vision
COGS 577 (Fall 2025) -
Intro to Computer Vision
CSC 577 (Fall 2025) -
Special Topics in Computer Sci
CSC 296 (Fall 2025)
2024-25 Courses
-
Dissertation
CSC 920 (Spring 2025) -
Honors Independent Study
CSC 499H (Spring 2025) -
Research
CSC 900 (Spring 2025) -
Intro To Computer Vision
CSC 477 (Fall 2024) -
Intro to Computer Vision
COGS 577 (Fall 2024) -
Intro to Computer Vision
CSC 577 (Fall 2024) -
Research
CSC 900 (Fall 2024) -
Special Topics in Computer Sci
CSC 296 (Fall 2024)
2023-24 Courses
-
Directed Research
CSC 392 (Spring 2024) -
Dissertation
CSC 920 (Spring 2024) -
Dissertation
CSC 920 (Fall 2023) -
Intro To Computer Vision
CSC 477 (Fall 2023) -
Intro to Computer Vision
CSC 577 (Fall 2023) -
Probabilistic Graphical Models
CSC 535 (Fall 2023) -
Research
CSC 900 (Fall 2023) -
Thesis
CSC 910 (Fall 2023)
2022-23 Courses
-
Directed Research
CSC 492 (Spring 2023) -
Dissertation
CSC 920 (Spring 2023) -
Research
CSC 900 (Spring 2023) -
Research
MATH 900 (Spring 2023) -
Directed Research
CSC 492 (Fall 2022) -
Dissertation
CSC 920 (Fall 2022) -
Research
CSC 900 (Fall 2022) -
Research
MATH 900 (Fall 2022) -
Thesis
CSC 910 (Fall 2022)
2021-22 Courses
-
Directed Research
CSC 492 (Spring 2022) -
Dissertation
CSC 920 (Spring 2022) -
Independent Study
CSC 399 (Spring 2022) -
Intro To Computer Vision
CSC 477 (Spring 2022) -
Intro to Computer Vision
CSC 577 (Spring 2022) -
Research
CSC 900 (Spring 2022) -
Research
MATH 900 (Spring 2022) -
AdvTpc Artificial Intelligence
CSC 696H (Fall 2021) -
Directed Research
CSC 492 (Fall 2021) -
Dissertation
CSC 920 (Fall 2021) -
Independent Study
CSC 399 (Fall 2021) -
Research
CSC 900 (Fall 2021)
2020-21 Courses
-
Dissertation
CSC 920 (Spring 2021) -
Independent Study
CSC 599 (Spring 2021) -
Intro To Computer Vision
CSC 477 (Spring 2021) -
Intro to Computer Vision
CSC 577 (Spring 2021) -
Research
CSC 900 (Spring 2021) -
AdvTpc Artificial Intelligence
CSC 696H (Fall 2020) -
Dissertation
CSC 920 (Fall 2020) -
Research
CSC 900 (Fall 2020)
2019-20 Courses
-
Adv Tpcs:Doctoral Colloq
CSC 695C (Spring 2020) -
Directed Research
CSC 492 (Spring 2020) -
Dissertation
CSC 920 (Spring 2020) -
Honors Thesis
CSC 498H (Spring 2020) -
Independent Study
CSC 399 (Spring 2020) -
Independent Study
CSC 599 (Spring 2020) -
Intro To Computer Vision
CSC 477 (Spring 2020) -
Intro to Computer Vision
CSC 577 (Spring 2020) -
Research
CSC 900 (Spring 2020) -
Adv Tpcs:Doctoral Colloq
CSC 695C (Fall 2019) -
Directed Research
CSC 392 (Fall 2019) -
Honors Thesis
CSC 498H (Fall 2019) -
Independent Study
CSC 499 (Fall 2019) -
Probabilistic Graphical Models
CSC 535 (Fall 2019) -
Research
CSC 900 (Fall 2019)
2018-19 Courses
-
Adv Tpcs:Doctoral Colloq
CSC 695C (Spring 2019) -
Directed Research
CSC 392 (Spring 2019) -
Dissertation
ECE 920 (Spring 2019) -
Honors Thesis
CSC 498H (Spring 2019) -
Intro To Computer Vision
CSC 477 (Spring 2019) -
Intro to Computer Vision
CSC 577 (Spring 2019) -
Adv Tpcs:Doctoral Colloq
CSC 695C (Fall 2018) -
Directed Research
CSC 392 (Fall 2018) -
Directed Research
CSC 492 (Fall 2018) -
Dissertation
ECE 920 (Fall 2018) -
Honors Thesis
CSC 498H (Fall 2018) -
Independent Study
CSC 399 (Fall 2018) -
Independent Study
CSC 499 (Fall 2018) -
Probabilistic Graphical Models
CSC 535 (Fall 2018)
2017-18 Courses
-
Directed Research
CSC 492 (Summer I 2018) -
Adv Tpcs:Doctoral Colloq
CSC 695C (Spring 2018) -
Directed Research
CSC 392 (Spring 2018) -
Directed Research
CSC 492 (Spring 2018) -
Dissertation
ECE 920 (Spring 2018) -
Honors Independent Study
CSC 499H (Spring 2018) -
Honors Thesis
CSC 498H (Spring 2018) -
Independent Study
CSC 399 (Spring 2018) -
Probabilistic Graphical Models
CSC 535 (Spring 2018) -
Adv Tpcs:Doctoral Colloq
CSC 695C (Fall 2017) -
Directed Research
CSC 392 (Fall 2017) -
Dissertation
ECE 920 (Fall 2017) -
Honors Independent Study
CSC 399H (Fall 2017) -
Independent Study
CSC 499 (Fall 2017) -
Intro To Computer Vision
CSC 477 (Fall 2017) -
Intro to Computer Vision
CSC 577 (Fall 2017)
2016-17 Courses
-
Adv Tpcs:Doctoral Colloq
CSC 695C (Spring 2017) -
Directed Research
CSC 392 (Spring 2017) -
Dissertation
ECE 920 (Spring 2017) -
Independent Study
CSC 399 (Spring 2017) -
Independent Study
CSC 599 (Spring 2017) -
Research
ECE 900 (Spring 2017) -
System Programming+Unix
CSC 352 (Spring 2017) -
Adv Tpcs:Doctoral Colloq
CSC 695C (Fall 2016) -
Intro To Computer Vision
CSC 477 (Fall 2016) -
Intro to Computer Vision
CSC 577 (Fall 2016)
2015-16 Courses
-
Dissertation
CSC 920 (Spring 2016) -
Research
STAT 900 (Spring 2016)
Scholarly Contributions
Books
- Barnard, J. J. (2016). Computational approaches for integrating vision and language. Morgan-Claypool.
Chapters
- Barnard, J. J., Simek, K., Brau, E., Predoehl, A., Guan, J., & Butler, E. A. (2017). RC1: * Counted as accepted last year: Computational interpersonal emotion systems. In Computational Models in Social Psychology.
- Barnard, J. J., Simek, K., Brau, E., Predoehl, A., Guan, J., & Butler, E. A. (2017). RC1: Counted as accepted last year: Computational interpersonal emotion systems. In Computational Models in Social Psychology.
- Butler, E. A., Guan, J., Predoehl, A., Brau, E., Simek, K., & Barnard, J. J. (2017). Computational interpersonal emotion systems. In Computational Models in Social Psychology.
Journals/Publications
- Dai, Y., Wu, Y., Zhou, F., & Barnard, J. J. (2021). Attentional Local Contrast Networks for Infrared Small Target Detection. IEEE Transactions on Geoscience and Remote Sensing (TGRS).More infoSoid journal
- Butler, E., & Barnard, K. (2019). Quantifying Interpersonal Dynamics for the Study of Socio-Emotional Processes and Health. Psychosomatic Medicine, 81(8).More infoTop non-CS venue
- Katib, A., Rao, P., Barnard, J. J., & Kamhoua, C. (2018). Fast Approximate Score Computation on Large-Scale Distributed Data for Learning Multinomial Bayesian Networks. ACM Transactions on Knowledge Discovery from Data.
- Katib, A., Rao, P., Barnard, K., & Kamhoua, C. (2019). Fast Approximate Score Computation on Large-Scale Distributed Data for Learning Multinomial Bayesian Networks. ACM Transactions on Knowledge Discovery from Data, 13(2).More infoCore B
- Morad, S., Nash, J., Higa, S., Smith, R. G., Parness, A., & Barnard, K. (2019). Improving Visual Feature Extraction in Glacial Environments. IEEE Robotics and Automation Letters, to appear.More infoSolid second tier
- Shao, J., Zhang, J., huang, X., Liang, R., & Barnard, K. (2019). Fiber bundle image restoration using deep learning. Optics Letters, 44(5), 1080-1083.More infoSolid second tier, paper got "editor pick"
- Shao, J., Zhang, J., huang, X., Liang, R., & Barnard, K. (2019). Fiber bundle imaging resolution enhancement using deep learning. Optics Express.More infoSolid second tier
- Hamilton, C. W., Byrne, S., Barnard, K. J., Rodriguez, J. J., Morrison, C. T., Palafox, L. F., & Savage, R. (2018). A Bayesian Approach to Sub-Kilometer Crater Shape Analysis using Individual HiRISE Images. IEEE Transactions on Geoscience and Remote Sensing, PP(99), 1-11. doi:10.1109/TGRS.2018.2825608
- Savage, R., Palafox, L. F., Morrison, C. T., Rodriguez, J. J., Barnard, K. J., Byrne, S., & Hamilton, C. W. (2018). A Bayesian Approach to Sub-Kilometer Crater Shape Analysis using Individual HiRISE Images. Transactions on Geoscience and Remote Sensing.
- Shao, J., Liao, W. C., Liang, R., & Barnard, J. J. (2018). Resolution enhancement for fiber bundle imaging using maximum a posteriori estimation. Optics Letters, 43, 1906-1909.
- Gabbur, P., Hoying, J., & Barnard, K. (2015). Multimodal probabilistic generative models for time-course gene expression data and Gene Ontology (GO) tags. Mathematical biosciences, 268, 80-91.More infoWe propose four probabilistic generative models for simultaneously modeling gene expression levels and Gene Ontology (GO) tags. Unlike previous approaches for using GO tags, the joint modeling framework allows the two sources of information to complement and reinforce each other. We fit our models to three time-course datasets collected to study biological processes, specifically blood vessel growth (angiogenesis) and mitotic cell cycles. The proposed models result in a joint clustering of genes and GO annotations. Different models group genes based on GO tags and their behavior over the entire time-course, within biological stages, or even individual time points. We show how such models can be used for biological stage boundary estimation de novo. We also evaluate our models on biological stage prediction accuracy of held out samples. Our results suggest that the models usually perform better when GO tag information is included.
- Guan, J., Brau, E., Simek, K., Morrison, C. T., Butler, E. A., & Barnard, K. J. (2015). Moderated and Drifting Linear Dynamical Systems. International Conference on Machine Learning.More infoThis venue is a peer reviewed, competitive conference (acceptance rate: 26%) and the full paper is published as part of the conference proceedings [ CSRanking endorsed, A* ]
- Reed, R. G., Barnard, K., & Butler, E. A. (2015). Distinguishing emotional coregulation from codysregulation: an investigation of emotional dynamics and body weight in romantic couples. Emotion (Washington, D.C.), 15(1), 45-60.More infoWell-regulated emotions, both within people and between relationship partners, play a key role in facilitating health and well-being. The present study examined 39 heterosexual couples' joint weight status (both partners are healthy-weight, both overweight, 1 healthy-weight, and 1 overweight) as a predictor of 2 interpersonal emotional patterns during a discussion of their shared lifestyle choices. The first pattern, coregulation, is one in which partners' coupled emotions show a dampening pattern over time and ultimately return to homeostatic levels. The second, codysregulation, is one in which partners' coupled emotions are amplified away from homeostatic balance. We demonstrate how a coupled linear oscillator (CLO) model (Butner, Amazeen, & Mulvey, 2005) can be used to distinguish coregulation from codysregulation. As predicted, healthy-weight couples and mixed-weight couples in which the man was heavier than the woman displayed coregulation, but overweight couples and mixed-weight couples in which the woman was heavier showed codysregulation. These results suggest that heterosexual couples in which the woman is overweight may face formidable coregulatory challenges that could undermine both partners' well-being. The results also demonstrate the importance of distinguishing between various interpersonal emotional dynamics for understanding connections between interpersonal emotions and health.
- Butler, E. A., & Gross, J. J. (2014). Testing the effects of suppression and reappraisal on emotional concordance using a multivariate multilevel model. Biological psychology, 98, 6-18.More infoIn theory, the essence of emotion is coordination across experiential, behavioral, and physiological systems in the service of functional responding to environmental demands. However, people often regulate emotions, which could either reduce or enhance cross-system concordance. The present study tested the effects of two forms of emotion regulation (expressive suppression, positive reappraisal) on concordance of subjective experience (positive-negative valence), expressive behavior (positive and negative), and physiology (inter-beat interval, skin conductance, blood pressure) during conversations between unacquainted young women. As predicted, participants asked to suppress showed reduced concordance for both positive and negative emotions. Reappraisal instructions also reduced concordance for negative emotions, but increased concordance for positive ones. Both regulation strategies had contagious interpersonal effects on average levels of responding. Suppression reduced overall expression for both regulating and uninstructed partners, while reappraisal reduced negative experience. Neither strategy influenced the uninstructed partners' concordance. These results suggest that emotion regulation impacts concordance by altering the temporal coupling of phasic subsystem responses, rather than by having divergent effects on subsystem tonic levels.
- Butler, E. A., Gross, J. J., & Barnard, K. (2013). Testing the effects of suppression and reappraisal on emotional concordance using a multivariate multilevel model. Biological Psychology.More infoAbstract: In theory, the essence of emotion is coordination across experiential, behavioral, and physiological systems in the service of functional responding to environmental demands. However, people often regulate emotions, which could either reduce or enhance cross-system concordance. The present study tested the effects of two forms of emotion regulation (expressive suppression, positive reappraisal) on concordance of subjective experience (positive-negative valence), expressive behavior (positive and negative), and physiology (inter-beat interval, skin conductance, blood pressure) during conversations between unacquainted young women. As predicted, participants asked to suppress showed reduced concordance for both positive and negative emotions. Reappraisal instructions also reduced concordance for negative emotions, but increased concordance for positive ones. Both regulation strategies had contagious interpersonal effects on average levels of responding. Suppression reduced overall expression for both regulating and uninstructed partners, while reappraisal reduced negative experience. Neither strategy influenced the uninstructed partners' concordance. These results suggest that emotion regulation impacts concordance by altering the temporal coupling of phasic subsystem responses, rather than by having divergent effects on subsystem tonic levels. © 2013 Elsevier B.V. All rights reserved.
- Kraft, R., Kahn, A., Medina-Franco, J. L., Orlowski, M. L., Baynes, C., Loṕez-Vallejo, F., Barnard, K., Maggiora, G. M., & Restifo, L. L. (2013). A cell-based fascin bioassay identifies compounds with potential anti-metastasis or cognition-enhancing functions. DMM Disease Models and Mechanisms, 6(1), 217-235.More infoPMID: 22917928;PMCID: PMC3529353;Abstract: The actin-bundling protein fascin is a key mediator of tumor invasion and metastasis and its activity drives filopodia formation, cell-shape changes and cell migration. Small-molecule inhibitors of fascin block tumor metastasis in animal models. Conversely, fascin deficiency might underlie the pathogenesis of some developmental brain disorders. To identify fascin-pathway modulators we devised a cell-based assay for fascin function and used it in a bidirectional drug screen. The screen utilized cultured fascin-deficient mutant Drosophila neurons, whose neurite arbors manifest the 'filagree' phenotype. Taking a repurposing approach, we screened a library of 1040 known compounds, many of them FDA-approved drugs, for filagree modifiers. Based on scaffold distribution, molecular-fingerprint similarities, and chemical-space distribution, this library has high structural diversity, supporting its utility as a screening tool. We identified 34 fascin-pathway blockers (with potential anti-metastasis activity) and 48 fascin-pathway enhancers (with potential cognitive-enhancer activity). The structural diversity of the active compounds suggests multiple molecular targets. Comparisons of active and inactive compounds provided preliminary structure-activity relationship information. The screen also revealed diverse neurotoxic effects of other drugs, notably the 'beads-on-a-string' defect, which is induced solely by statins. Statin-induced neurotoxicity is enhanced by fascin deficiency. In summary, we provide evidence that primary neuron culture using a genetic model organism can be valuable for early-stage drug discovery and developmental neurotoxicity testing. Furthermore, we propose that, given an appropriate assay for target-pathway function, bidirectional screening for brain-development disorders and invasive cancers represents an efficient, multipurpose strategy for drug discovery. © 2012. Published by The Company of Biologists Ltd.
- Peralta, R. T., Rebguns, A., Fasel, I. R., & Barnard, K. (2013). Learning a policy for gesture-based active multi-touch authentication. Lecture Notes in Computer Science (including subseries Lecture Notes in Artificial Intelligence and Lecture Notes in Bioinformatics), 8030 LNCS, 59-68.More infoAbstract: Multi-touch tablets can offer a large, collaborative space where several users can work on a task at the same time. However, the lack of privacy in these situations makes standard password-based authentication easily compromised. This work presents a new gesture-based authentication system based on users' unique signature of touch motion when drawing a combination of one-stroke gestures following two different policies, one fixed for all users and the other selected by a model of control to maximize the expected long-term information gain. The system is able to achieve high user recognition accuracy with relatively few gestures, demonstrating that human touch patterns have a distinctive "signature" that can be used as a powerful biometric measure for user recognition and personalization. © 2013 Springer-Verlag Berlin Heidelberg.
- Pero, L. D., Bowdish, J., Kermgard, B., Hartley, E., & Barnard, K. (2013). Understanding bayesian rooms using composite 3D object models. Proceedings of the IEEE Computer Society Conference on Computer Vision and Pattern Recognition, 153-160.More infoAbstract: We develop a comprehensive Bayesian generative model for understanding indoor scenes. While it is common in this domain to approximate objects with 3D bounding boxes, we propose using strong representations with finer granularity. For example, we model a chair as a set of four legs, a seat and a backrest. We find that modeling detailed geometry improves recognition and reconstruction, and enables more refined use of appearance for scene understanding. We demonstrate this with a new likelihood function that rewards 3D object hypotheses whose 2D projection is more uniform in color distribution. Such a measure would be confused by background pixels if we used a bounding box to represent a concave object like a chair. Complex objects are modeled using a set or re-usable 3D parts, and we show that this representation captures much of the variation among object instances with relatively few parameters. We also designed specific data-driven inference mechanisms for each part that are shared by all objects containing that part, which helps make inference transparent to the modeler. Further, we show how to exploit contextual relationships to detect more objects, by, for example, proposing chairs around and underneath tables. We present results showing the benefits of each of these innovations. The performance of our approach often exceeds that of state-of-the-art methods on the two tasks of room layout estimation and object recognition, as evaluated on two bench mark data sets used in this domain. © 2013 IEEE.
- Predoehl, A., Morris, S., & Barnard, K. (2013). A statistical model for recreational trails in aerial images. Proceedings of the IEEE Computer Society Conference on Computer Vision and Pattern Recognition, 337-344.More infoAbstract: We present a statistical model of aerial images of recreational trails, and a method to infer trail routes in such images. We learn a set of textons describing the images, and use them to divide the image into super-pixels represented by their text on. We then learn, for each text on, the frequency of generating on-trail and off-trail pixels, and the direction of trail through on-trail pixels. From these, we derive an image likelihood function. We combine that with a prior model of trail length and smoothness, yielding a posterior distribution for trails, given an image. We search for good values of this posterior using a novel stochastic variation of Dijkstra's algorithm. Our experiments, on trail images and ground truth collected in the western continental USA, show substantial improvement over those of the previous best trail-finding method. © 2013 IEEE.
- Pero, L. D., Bowdish, J., Fried, D., Kermgard, B., Hartley, E., & Barnard, K. (2012). Bayesian geometric modeling of indoor scenes. Proceedings of the IEEE Computer Society Conference on Computer Vision and Pattern Recognition, 2719-2726.More infoAbstract: We propose a method for understanding the 3D geometry of indoor environments (e.g. bedrooms, kitchens) while simultaneously identifying objects in the scene (e.g. beds, couches, doors). We focus on how modeling the geometry and location of specific objects is helpful for indoor scene understanding. For example, beds are shorter than they are wide, and are more likely to be in the center of the room than cabinets, which are tall and narrow. We use a generative statistical model that integrates a camera model, an enclosing room box, frames (windows, doors, pictures), and objects (beds, tables, couches, cabinets), each with their own prior on size, relative dimensions, and locations. We fit the parameters of this complex, multi-dimensional statistical model using an MCMC sampling approach that combines discrete changes (e.g, adding a bed), and continuous parameter changes (e.g., making the bed larger). We find that introducing object category leads to state-of-the-art performance on room layout estimation, while also enabling recognition based only on geometry. © 2012 IEEE.
- Yanai, K., Kawakubo, H., & Barnard, K. (2012). Entropy-Based Analysis of Visual and Geolocation Concepts in Images. Multimedia Information Extraction: Advances in Video, Audio, and Imagery Analysis for Search, Data Mining, Surveillance, and Authoring, 63-80.
- Brau, E., Barnard, J. J., Palanivelu, R. -., Dunatunga, D., Tsukamoto, T., & Lee, P. (2011). A generative statistical model for tracking multiple smooth trajectories of pollen tubes. Proceedings of the IEEE Computer Vision and Pattern Recognition, 1137-1144.More infodoi: 10.1109/CVPR.2011.5995736
- Brau, E., Dunatunga, D., Barnard, K., Tsukamoto, T., Palanivelu, R., & Lee, P. (2011). A generative statistical model for tracking multiple smooth trajectories. Proceedings of the IEEE Computer Society Conference on Computer Vision and Pattern Recognition, 1137-1144.More infoAbstract: We present a general model for tracking smooth trajectories of multiple targets in complex data sets, where tracks potentially cross each other many times. As the number of overlapping trajectories grows, exploiting smoothness becomes increasingly important to disambiguate the association of successive points. However, in many important problems an effective parametric model for the trajectories does not exist. Hence we propose modeling trajectories as independent realizations of Gaussian processes with kernel functions which allow for arbitrary smooth motion. Our generative statistical model accounts for the data as coming from an unknown number of such processes, together with expectations for noise points and the probability that points are missing. For inference we compare two methods: A modified version of the Markov chain Monte Carlo data association (MCMCDA) method, and a Gibbs sampling method which is much simpler and faster, and gives better results by being able to search the solution space more efficiently. In both cases, we compare our results against the smoothing provided by linear dynamical systems (LDS). We test our approach on videos of birds and fish, and on 82 image sequences of pollen tubes growing in a petri dish, each with up to 60 tubes with multiple crossings. We achieve 93% accuracy on image sequences with up to ten trajectories (35 sequences) and 88% accuracy when there are more than ten (42 sequences). This performance surpasses that of using an LDS motion model, and far exceeds a simple heuristic tracker. © 2011 IEEE.
- Fan, Q., Barnard, K., Amir, A., & Efrat, A. (2011). Robust spatiotemporal matching of electronic slides to presentation videos. IEEE transactions on image processing : a publication of the IEEE Signal Processing Society, 20(8), 2315-28.More infoWe describe a robust and efficient method for automatically matching and time-aligning electronic slides to videos of corresponding presentations. Matching electronic slides to videos provides new methods for indexing, searching, and browsing videos in distance-learning applications. However, robust automatic matching is challenging due to varied frame composition, slide distortion, camera movement, low-quality video capture, and arbitrary slides sequence. Our fully automatic approach combines image-based matching of slide to video frames with a temporal model for slide changes and camera events. To address these challenges, we begin by extracting scale-invariant feature-transformation (SIFT) keypoints from both slides and video frames, and matching them subject to a consistent projective transformation (homography) by using random sample consensus (RANSAC). We use the initial set of matches to construct a background model and a binary classifier for separating video frames showing slides from those without. We then introduce a new matching scheme for exploiting less distinctive SIFT keypoints that enables us to tackle more difficult images. Finally, we improve upon the matching based on visual information by using estimated matching probabilities as part of a hidden Markov model (HMM) that integrates temporal information and detected camera operations. Detailed quantitative experiments characterize each part of our approach and demonstrate an average accuracy of over 95% in 13 presentation videos.
- Pero, L. D., Guan, J., Brau, E., Schlecht, J., & Barnard, K. (2011). Sampling bedrooms. Proceedings of the IEEE Computer Society Conference on Computer Vision and Pattern Recognition, 2009-2016.More infoAbstract: We propose a top down approach for understanding indoor scenes such as bedrooms and living rooms. These environments typically have the Manhattan world property that many surfaces are parallel to three principle ones. Further, the 3D geometry of the room and objects within it can largely be approximated by non overlapping simple structures such as single blocks (e.g. the room boundary), thin blocks (e.g. picture frames), and objects that are well modeled by single blocks (e.g. simple beds). We separately model the 3D geometry, the imaging process (camera parameters), and edge likelihood, to provide a generative statistical model for image data. We fit this model using data driven MCMC sampling. We combine reversible jump Metropolis Hastings samples for discrete changes in the model such as the number of blocks, and stochastic dynamics to estimate continuous parameter values in a particular parameter space that includes block positions, block sizes, and camera parameters. We tested our approach on two datasets using room box pixel orientation. Despite using only bounding box geometry and, in particular, not training on appearance, our method achieves results approaching those of others. We also introduce a new evaluation method for this domain based on ground truth camera parameters, which we found to be more sensitive to the task of understanding scene geometry. © 2011 IEEE.
- Pero, L. D., Lee, P., Magahern, J., Hartley, E., & Barnard, K. (2011). Fusing object detection and region appearance for image-text alignment. MM'11 - Proceedings of the 2011 ACM Multimedia Conference and Co-Located Workshops, 1113-1116.More infoAbstract: We present a method for automatically aligning words to image regions that integrates specific object classifiers (e.g., "car"detectors) with weak models based on appearance features. Previous strategies have largely focused on the latter, and thus have not exploited progress on object category recognition. Hence, we augment region labeling with object detection, which simplifies the problem by reliably identifying a subset of the labels, and thereby reducing correspondence ambiguity overall. Comprehensive testing on the SAIAPR TC dataset shows that principled integration of object detection improves the region labeling task. Copyright 2011 ACM.
- Taralova, E. H., Schlecht, J., Barnard, K., & Pryor, B. M. (2011). Modelling and visualizing morphology in the fungus Alternaria. Fungal Biology, 115(11), 1163-1173.More infoPMID: 22036294;Abstract: Alternaria is one of the most cosmopolitan fungal genera encountered and impacts humans and human activities in areas of material degradation, phytopathology, food toxicology, and respiratory disease. Contemporary methods of taxon identification rely on assessments of morphology related to sporulation, which are critical for accurate diagnostics. However, the morphology of Alternaria is quite complex, and precise characterization can be laborious, time-consuming, and often restricted to experts in this field. To make morphology characterization easier and more broadly accessible, a generalized statistical model was developed for the three-dimensional geometric structure of the sporulation apparatus. The model is inspired by the widely used grammar-based models for plants, Lindenmayer-systems, which build structure by repeated application of rules for growth. Adjusting the parameters of the underlying probability distributions yields variations in the morphology, and thus the approach provides an excellent tool for exploring the morphology of Alternaria under different assumptions, as well as understanding how it is largely the consequence of local rules for growth. Further, different choices of parameters lead to different model groups, which can then be visually compared to published descriptions or microscopy images to validate parameters for species-specific models. The approach supports automated analysis, as the models can be fit to image data using statistical inference, and the explicit representation of the geometry allows the accurate computation of any morphological quantity. Furthermore, because the model can encode the statistical variation of geometric parameters for different species, it will allow automated species identification from microscopy images using statistical inference. In summary, the approach supports visualization of morphology, automated quantification of phenotype structure, and identification based on form. © 2011 British Mycological Society.
- Gabbur, P., Hua, H., & Barnard, K. (2010). A fast connected components labeling algorithm and its application to real-time pupil detection. Machine Vision and Applications, 21(5), 779-787.More infoAbstract: We describe a fast connected components labeling algorithm using a region coloring approach. It computes region attributes such as size, moments, and bounding boxes in a single pass through the image. Working in the context of real-time pupil detection for an eye tracking system, we compare the time performance of our algorithm with a contour tracing-based labeling approach and a region coloring method developed for a hardware eye detection system. We find that region attribute extraction performance exceeds that of these comparison methods. Further, labeling each pixel, which requires a second pass through the image, has comparable performance. © Springer-Verlag 2009.
- Yanai, K., & Barnard, K. (2010). Region-based automatic web image selection. MIR 2010 - Proceedings of the 2010 ACM SIGMM International Conference on Multimedia Information Retrieval, 305-312.More infoAbstract: We propose a new Web image selection method which employs the region-based bag-of-features representation. The contribution of this work is (1) to introduce the region-based bag-of-features representation into an Web image selection task where training data is incomplete, and (2) to prove its effectiveness by experiments with both generative and discriminative machine learning methods. In the experiments, we used a multiple-instance learning SVM and a standard SVM as discriminative methods, and pLSA and LDA mixture models as probabilistic generative methods. Several works on Web image filtering task with bag-of-features have been proposed so far. However, in case that the training data includes much noise, sufficient results could not be obtained. In this paper, we divide images into regions and classify each region instead of classifying whole images. By this region-based classification, we can separate foreground regions from background regions and achieve more effective image training from incomplete training data. By the experiments, we show that the results by the proposed methods outperformed the results by the whole-image-based bag-of-features. Copyright 2010 ACM.
- Schlecht, J., & Barnard, K. (2009). Learning models of object structure. Advances in Neural Information Processing Systems 22 - Proceedings of the 2009 Conference, 1615-1623.More infoAbstract: We present an approach for learning stochastic geometric models of object categories from single view images. We focus here on models expressible as a spatially contiguous assemblage of blocks. Model topologies are learned across groups of images, and one or more such topologies is linked to an object category (e.g. chairs). Fitting learned topologies to an image can be used to identify the object class, as well as detail its geometry. The latter goes beyond labeling objects, as it provides the geometric structure of particular instances. We learn the models using joint statistical inference over category parameters, camera parameters, and instance parameters. These produce an image likelihood through a statistical imaging model. We use trans-dimensional sampling to explore topology hypotheses, and alternate between Metropolis-Hastings and stochastic dynamics to explore instance parameters. Experiments on images of furniture objects such as tables and chairs suggest that this is an effective approach for learning models that encode simple representations of category geometry and the statistics thereof, and support inferring both category and geometry on held out single view images.
- Barnard, K., Fan, Q., Swaminathan, R., Hoogs, A., Collins, R., Rondot, P., & Kaufhold, J. (2008). Evaluation of localized semantics: Data, methodology, and experiments. International Journal of Computer Vision, 77(1-3), 199-217.More infoAbstract: We present a new data set of 1014 images with manual segmentations and semantic labels for each segment, together with a methodology for using this kind of data for recognition evaluation. The images and segmentations are from the UCB segmentation benchmark database (Martin et al., in International conference on computer vision, vol. II, pp. 416-421, 2001). The database is extended by manually labeling each segment with its most specific semantic concept in WordNet (Miller et al., in Int. J. Lexicogr. 3(4):235-244, 1990). The evaluation methodology establishes protocols for mapping algorithm specific localization (e.g., segmentations) to our data, handling synonyms, scoring matches at different levels of specificity, dealing with vocabularies with sense ambiguity (the usual case), and handling ground truth regions with multiple labels. Given these protocols, we develop two evaluation approaches. The first measures the range of semantics that an algorithm can recognize, and the second measures the frequency that an algorithm recognizes semantics correctly. The data, the image labeling tool, and programs implementing our evaluation strategy are all available on-line (kobus.ca//research/data/IJCV_2007). We apply this infrastructure to evaluate four algorithms which learn to label image regions from weakly labeled data. The algorithms tested include two variants of multiple instance learning (MIL), and two generative multi-modal mixture models. These experiments are on a significantly larger scale than previously reported, especially in the case of MIL methods. More specifically, we used training data sets up to 37,000 images and training vocabularies of up to 650 words. We found that one of the mixture models performed best on image annotation and the frequency correct measure, and that variants of MIL gave the best semantic range performance. We were able to substantively improve the performance of MIL methods on the other tasks (image annotation and frequency correct region labeling) by providing an appropriate prior. © 2007 Springer Science+Business Media, LLC.
- Morris, S., & Barnard, K. (2008). Finding trails. 26th IEEE Conference on Computer Vision and Pattern Recognition, CVPR.More infoAbstract: We present a statistical learning approach for finding recreational trails in aerial images. While the problem of recognizing relatively straight and well defined roadways in digital images has been well studied in the literature, the more difficult problem of extracting trails has received no attention. However, trails and rough roads are less likely to be adequately mapped, and change more rapidly over time. Automated tools for finding trails will be useful to cartographers, recreational users and governments. In addition, the methods developed here are applicable to the more general problem of finding linear structure. Our approach combines local estimates for image pixel trail probabilities with the global constraint that such pixels must link together to form a path. For the local part, we present results using three classification techniques. To construct a global solution (a trail) from these probabilities, we propose a global cost function that includes both global probability and path length. We show that the addition of a length term significantly improves trail finding ability. However, computing the optimal trail becomes intractable as known dynamic programming methods do not apply. Thus we describe a new splitting heuristic based on Dijkstra's algorithm. We then further improve upon the results with a trail sampling scheme. We test our approach on 500 challenging images along the 2500 mile continental divide mountain bike trail, where assumptions prevalent in the road literature are violated. ©2008 IEEE.
- Schlecht, J., Kaplan, M. E., Barnard, K., Karafet, T., Hammer, M. F., & Merchant, N. C. (2008). Machine-learning approaches for classifying haplogroup from Y chromosome STR data. PLoS Computational Biology, 4(6).More infoPMID: 18551166;PMCID: PMC2396484;Abstract: Genetic variation on the non-recombining portion of the Y chromosome contains information about the ancestry of male lineages. Because of their low rate of mutation, single nucleotide polymorphisms (SNPs) are the markers of choice for unambiguously classifying Y chromosomes into related sets of lineages known as haplogroups, which tend to show geographic structure in many parts of the world. However, performing the large number of SNP genotyping tests needed to properly infer haplogroup status is expensive and time consuming. A novel alternative for assigning a sampled Y chromosome to a haplogroup is presented here. We show that by applying modern machine-learning algorithms we can infer with high accuracy the proper Y chromosome haplogroup of a sample by scoring a relatively small number of Y-linked short tandem repeats (STRs). Learning is based on a diverse ground-truth data set comprising pairs of SNP test results (haplogroup) and corresponding STR scores. We apply several independent machine-learning methods in tandem to learn formal classification functions. The result is an integrated high-throughput analysis system that automatically classifies large numbers of samples into haplogroups in a cost-effective and accurate manner. © 2008 Schlecht et al.
- Barnard, K., & Fan, Q. (2007). Reducing correspondence ambiguity in loosely labeled training data. Proceedings of the IEEE Computer Society Conference on Computer Vision and Pattern Recognition.More infoAbstract: We develop an approach to reduce correspondence ambiguity in training data where data items are associated with sets of plausible labels. Our domain is images annotated with keywords where it is not known which part of the image a keyword refers to. In contrast to earlier approaches that build predictive models or classifiers despite the ambiguity, we argue that that it is better to first address the correspondence ambiguity, and then build more complex models from the improved training data. This addresses difficulties of fitting complex models in the face of ambiguity while exploiting all the constraints available from the training data. We contribute a simple and flexible formulation of the problem, and show results validated by a recently developed comprehensive evaluation data set and corresponding evaluation methodology. © 2007 IEEE.
- Schlecht, J., Barnard, K., & Pryor, B. (2007). Statistical inference of biological structure and point spread functions in 3D microscopy. Proceedings - Third International Symposium on 3D Data Processing, Visualization, and Transmission, 3DPVT 2006, 373-380.More infoAbstract: We present a novel method for detecting and quantifying 3D structure in stacks of microscopic images captured at incremental focal lengths. We express the image data as stochastically generated by an underlying model for biological specimen and the effects of the imaging system. The method simultaneously fits a model for proposed structure and the imaging system's parameters, which include a model of the point spread function. We demonstrate our approach by detecting spores in image stacks of Alternaria, a microscopic genus of fungus. The spores are modeled as opaque ellipsoids and fit to the data using statistical inference. Since the number of spores in the data is not known, model selection is incorporated into the fitting process. Thus, we develop a reversible jump Markov chain Monte Carlo sampler to explore the parameter space. Our results show that simultaneous statistical inference of specimen and imaging models is useful for quantifying biological structures in 3D microscopic images. In addition, we show that inferring a model of the imaging system improves the overall fit of the specimen model to the data. © 2006 IEEE.
- Schlecht, J., Barnard, K., Spriggs, E., & Pryor, B. (2007). Inferring grammar-based structure models from 3D microscopy data. Proceedings of the IEEE Computer Society Conference on Computer Vision and Pattern Recognition.More infoAbstract: We present a new method to fit grammar-based stochastic models for biological structure to stacks of microscopic images captured at incremental focal lengths. Providing the ability to quantitatively represent structure and automatically fit it to image data enables important biological research. We consider the case where individuals can be represented as an instance of a stochastic grammar, similar to L-systems used in graphics to produce realistic plant models. In particular, we construct a stochastic grammar of Alternaria, a genus of fungus, and fit instances of it to microscopic image stacks. We express the image data as the result of a generative process composed of the underlying probabilistic structure model together with the parameters of the imaging system. Fitting the model then becomes probabilistic inference. For this we create a reversible-jump MCMC sampler to traverse the parameter space. We observe that incorporating spatial structure helps fit the model parts, and that simultaneously fitting the imaging system is also very helpful. © 2007 IEEE.
- Kraft, R., Escobar, M. M., Narro, M. L., Kurtis, J. L., Efrat, A., Barnard, K., & Restifo, L. L. (2006). Phenotypes of Drosophila brain neurons in primary culture reveal a role for fascin in neurite shape and trajectory. The Journal of neuroscience : the official journal of the Society for Neuroscience, 26(34), 8734-47.More infoSubtle cellular phenotypes in the CNS may evade detection by routine histopathology. Here, we demonstrate the value of primary culture for revealing genetically determined neuronal phenotypes at high resolution. Gamma neurons of Drosophila melanogaster mushroom bodies (MBs) are remodeled during metamorphosis under the control of the steroid hormone 20-hydroxyecdysone (20E). In vitro, wild-type gamma neurons retain characteristic morphogenetic features, notably a single axon-like dominant primary process and an arbor of short dendrite-like processes, as determined with microtubule-polarity markers. We found three distinct genetically determined phenotypes of cultured neurons from grossly normal brains, suggesting that subtle in vivo attributes are unmasked and amplified in vitro. First, the neurite outgrowth response to 20E is sexually dimorphic, being much greater in female than in male gamma neurons. Second, the gamma neuron-specific "naked runt" phenotype results from transgenic insertion of an MB-specific promoter. Third, the recessive, pan-neuronal "filagree" phenotype maps to singed, which encodes the actin-bundling protein fascin. Fascin deficiency does not impair the 20E response, but neurites fail to maintain their normal, nearly straight trajectory, instead forming curls and hooks. This is accompanied by abnormally distributed filamentous actin. This is the first demonstration of fascin function in neuronal morphogenesis. Our findings, along with the regulation of human Fascin1 (OMIM 602689) by CREB (cAMP response element-binding protein) binding protein, suggest FSCN1 as a candidate gene for developmental brain disorders. We developed an automated method of computing neurite curvature and classifying neurons based on curvature phenotype. This will facilitate detection of genetic and pharmacological modifiers of neuronal defects resulting from fascin deficiency.
- Ramanan, D., Forsyth, D. A., & Barnard, K. (2006). Building models of animals from video. IEEE Transactions on Pattern Analysis and Machine Intelligence, 28(8), 1319-1333.More infoPMID: 16886866;Abstract: This paper argues that tracking, object detection, and model building are all similar activities. We describe a fully automatic system that builds 2D articulated models known as pictorial structures from videos of animals. The learned model can be used to detect the animal in the original video - in this sense, the system can be viewed as a generalized tracker (one that is capable of modeling objects while tracking them). The learned model can be matched to a visual library; here, the system can be viewed as a video recognition algorithm. The learned model can also be used to detect the animal in novel images - in this case, the system can be seen as a method for learning models for object recognition. We find that we can significantly improve the pictorial structures by augmenting them with a discriminative texture model learned from a texture library. We develop a novel texture descriptor that outperforms the state-of-the-art for animal textures. We demonstrate the entire system on real video sequences of three different animals. We show that we can automatically track and identify the given animal. We use the learned models to recognize animals from two data sets; images taken by professional photographers from the Corel collection, and assorted images from the Web returned by Google. We demonstrate quite good performance on both data sets. Comparing our results with simple baselines, we show that, for the Google set, we can detect, localize, and recover part articulations from a collection demonstrably hard for object recognition. © 2006 IEEE.
- Yanai, K., & Barnard, K. (2006). Finding visual concepts by web image mining. Proceedings of the 15th International Conference on World Wide Web, 923-924.More infoAbstract: We propose measuring "visualness" of concepts with images on the Web, that is, what extent concepts have visual characteristics. This is a new application of "Web image mining". To know which concept has visually discriminative power is important for image recognition, since not all concepts are related to visual contents. Mining image data on the Web with our method enables it. Our method performs probabilistic region selection for images and computes an entropy measure which represents "visualness" of concepts. In the experiments, we collected about forty thousand images from the Web for 150 concepts. We examined which concepts are suitable for annotation of image contents.
- Barnard, K., & Johnson, M. (2005). Word sense disambiguation with pictures. Artificial Intelligence, 167(1-2), 13-30.More infoAbstract: We introduce using images for word sense disambiguation, either alone, or in conjunction with traditional text based methods. The approach is based on a recently developed method for automatically annotating images by using a statistical model for the joint probability for image regions and words. The model itself is learned from a data base of images with associated text. To use the model for word sense disambiguation, we constrain the predicted words to be possible senses for the word under consideration. When word prediction is constrained to a narrow set of choices (such as possible senses), it can be quite reliable. We report on experiments using the resulting sense probabilities as is, as well as augmenting a state of the art text based word sense disambiguation algorithm. In order to evaluate our approach, we developed a new corpus, ImCor, which consists of a substantive portion of the Corel image data set associated with disambiguated text drawn from the SemCor corpus. Our experiments using this corpus suggest that visual information can be very useful in disambiguating word senses. It also illustrates that associated non-textual information such as image data can help ground language meaning. © 2005 Elsevier B.V. All rights reserved.
- Kim, D., Chung, C., & Barnard, K. (2005). Relevance feedback using adaptive clustering for image similarity retrieval. Journal of Systems and Software, 78(1), 9-23.More infoAbstract: Research has been devoted in recent years to relevance feedback as an effective solution to improve performance of image similarity search. However, few methods using the relevance feedback are currently available to perform relatively complex queries on large image databases. In the case of complex image queries, images with relevant concepts are often scattered across several visual regions in the feature space. This leads to adapting multiple regions to represent a query in the feature space. Therefore, it is necessary to handle disjunctive queries in the feature space. In this paper, we propose a new adaptive classification and cluster-merging method to find multiple regions and their arbitrary shapes of a complex image query. Our method achieves the same high retrieval quality regardless of the shapes of query regions since the measures used in our method are invariant under linear transformations. Extensive experiments show that the result of our method converges to the user's true information need fast, and the retrieval quality of our method is about 22% in recall and 20% in precision better than that of the query expansion approach, and about 35% in recall and about 31% in precision better than that of the query point movement approach, in MARS. © 2005 Elsevier Inc. All rights reserved.
- Morris, S., Gimblett, R., & Barnard, K. (2005). Probabilistic travel modeling using GPS. MODSIM05 - International Congress on Modelling and Simulation: Advances and Applications for Management and Decision Making, Proceedings, 149-155.More infoAbstract: Recreation simulation modeling, when combined with intelligent monitoring, is becoming a valuable tool for natural resource managers. The goal of recreation simulation is to accurately model recreational use, both current and future. Models are applied to gain a thorough understanding of the characteristics of recreation. Indicator variables such as visitor experience, carrying capacity and impact on resources can be computed. If the model is valid it can be used to predict future use as well as to investigate the effect of new scenarios and management decisions. Recent research has focused on agent-based modeling techniques. Recreators are represented by autonomous, intelligent agents that travel across the landscape. A central issue is the model used for agent travel decisions. Current techniques range from replicating trips exactly to making local, intersection level decisions based on probability. But little attention has been paid to justifying these models. In this work we examine a range of probabilistic models. The models differ in the length of the Markov chain used to compute agent decisions. The length of the chain ranges from zero (local decisions only) to infinity (exact trip replication). We test the length of the chain on held out data for validation. We show that the choice of model strongly influences the validity and results of the simulation. To test these models we present a framework for automatically constructing agent-based models from an input set of GPS tracklogs. The GPS tracklogs are collected by volunteers as they recreate in natural areas. Traditionally, data on where recreators travel is collected in the form of trip diaries, filled out on paper by visitors or by interview. Other demographic and attitudinal data is also collected along with the actual route traveled. Although the additional information is valuable, the data must be collected and entered by hand. Paper diaries also place a significant time burden on visitors, reducing the compliance rate as well as skewing the results (ensuring only visitors with excess time participate). Using GPS devices to record visitor trips helps alleviate these problems. The framework for processing GPS trips and automatically building a model presented in this work significantly reduces the time required to build a model, lowers the cost and widens the applicability of recreation simulation modeling to new areas. GPS devices automatically record their data, requiring only that visitors turn the unit on and carry it with a marginal view of the sky. GPS use is also becoming more widespread among recreators. As more recreators use GPS to record their trips, data useful to modeling is becoming increasingly available. The steps in GPS driven model generation are as follows. First, the set of GPS tracklogs is combined to form the underlying travel network along which agents will travel. Each GPS tracklog is then traced along the network in order to determine what choices were made as the recreator traveled across the network. This produces a list of trip itineraries. Model parameters (probability tables) can then be computed from the trips. The length of the Markov chain used in the probability tables is a parameter to the model. The optimal value is found by testing the likelihood of heldout data for different chain lengths. This step is done automatically. Once the optimal length of the chain is chosen the model is complete and agent-based simulation can proceed. The entire framework for automatically producing GPS driven agent-based models is implemented in our TopoFusion GPS mapping software. We present results from two collections of GPS tracklogs from different trail systems. The first is from Tucson Mountain Park and is the result of a volunteer collection effort by the authors. A trails master plan is underway at the park, with input from our model. The second is a collection of tracks from mountain bike rides in the Finger Rock Wash area, collected by the author. Testing by held-out data on both GPS datasets indicates that current modeling methods are insufficient to model recreator travel decisions. The middle ground (neither exact replication nor local decisions) consistently performs better.
- Ramanan, D., Forsyth, D. A., & Barnard, K. (2005). Detecting, localizing and recovering kinematics of textured animals. Proceedings of the IEEE Computer Society Conference on Computer Vision and Pattern Recognition, 2, 635-642.More infoAbstract: We develop and demonstrate an object recognition system capable of accurately detecting, localizing, and recovering the kinematic configuration of textured animals in real images. We build a deformation model of shape automatically from videos of animals and an appearance model of texture from a labeled collection of animal images, and combine the two models automatically. We develop a simple texture descriptor that outperforms the state of the art. We test our animal models on two datasets; images taken by professional photographers from the Corel collection, and assorted images from the web returned by Google. We demonstrate quite good performance on both datasets. Comparing our results with simple baselines, we show that for the Google set, we can recognize objects from a collection demonstrably hard for object recognition. © 2005 IEEE.
- Shirahatti, N. V., & Barnard, K. (2005). Evaluating image retrieval. Proceedings - 2005 IEEE Computer Society Conference on Computer Vision and Pattern Recognition, CVPR 2005, I, 955-961.More infoAbstract: We present a comprehensive strategy for evaluating image retrieval algorithms. Because automated image retrieval is only meaningful in its service to people, performance characterization must be grounded in human evaluation. Thus we have collected a large data set of human evaluations of retrieval results, both for query by image example and query by text. The data is independent of any particular image retrieval algorithm and can be used to evaluate and compare many such algorithms without further data collection. The data and calibration software are available on-line (http://kobus.ca/research/data). We develop and validate methods for generating sensible evaluation data, calibrating for disparate evaluators, mapping image retrieval system scores to the human evaluation results, and comparing retrieval systems. We demonstrate the process by providing grounded comparison results for several algorithms. © 2005 IEEE.
- Yanai, K., & Barnard, K. (2005). Image region entropy: A measure of "visualness" of web images associated with one concept. Proceedings of the 13th ACM International Conference on Multimedia, MM 2005, 419-422.More infoAbstract: We propose a new method to measure "visualness" of concepts, that is, what extent concepts have visual characteristics. To know which concept has visually discriminative power is important for image annotation, especially automatic image annotation by image recognition system, since not all concepts are related to visual contents. Our method performs probabilistic region selection for images which are labeled as concept "X" or "non-X", and computes an entropy measure which represents "visualness" of concepts. In the experiments, we collected about forty thousand images from the World-Wide Web using the Google Image Search for 150 concepts. We examined which concepts are suitable for annotation of image contents. Copyright © 2005 ACM.
- Carbonetto, P., Freitas, N. D., & Barnard, K. (2004). A statistical model for general contextual object recognition. Lecture Notes in Computer Science (including subseries Lecture Notes in Artificial Intelligence and Lecture Notes in Bioinformatics), 3021, 350-362.More infoAbstract: We consider object recognition as the process of attaching meaningful labels to specific regions of an image, and propose a model that learns spatial relationships between objects. Given a set of images and their associated text (e.g. keywords, captions, descriptions), the objective is to segment an image, in either a crude or sophisticated fashion, then to find the proper associations between words and regions. Previous models are limited by the scope of the representation. In particular, they fail to exploit spatial context in the images and words. We develop a more expressive model that takes this into account. We formulate a spatially consistent probabilistic mapping between continuous image feature vectors and the supplied word tokens. By learning both word-to-region associations and object relations, the proposed model augments scene segmentations due to smoothing implicit in spatial consistency. Context introduces cycles to the undirected graph, so we cannot rely on a straightforward implementation of the EM algorithm for estimating the model parameters and densities of the unknown alignment variables. Instead, we develop an approximate EM algorithm that uses loopy belief propagation in the inference step and iterative scaling on the pseudo-likelihood approximation in the parameter update step. The experiments indicate that our approximate inference and learning algorithm converges to good local solutions. Experiments on a diverse array of images show that spatial context considerably improves the accuracy of object recognition. Most significantly, spatial context combined with a nonlinear discrete object representation allows our models to cope well with over-segmented scenes. © Springer-Verlag 2004.
- Morris, S., Morris, A., & Barnard, K. (2004). Digital trail libraries. Proceedings of the ACM IEEE International Conference on Digital Libraries, JCDL 2004, 63-71.More infoAbstract: We propose the idea of an online, user submitted digital library of recreation trails. Digital libraries of trails offer advantages over paper guidebooks in that they are more accurate, dynamic and not limited to the experience of the author(s). The basic representation of a trail is a GPS track log, recorded as recreators travel on trails. As users complete trips, the GPS track logs of their trips are submitted to the central library voluntarily. A major problem is that track logs will overlap and intersect each other. We present a method for the combination of overlapping and intersecting GPS track logs to create a network of GPS trails. Each trail segment in the network can then be characterized by automatic and manual means, producing a digital library of trails. We also describe the TopoFusion system which creates, manages and visualizes GPS data, including GPS networks.
- Barnard, K., & Gabbur, P. (2003). Color and Color Constancy in a Translation Model for Object Recognition. Final Program and Proceedings - IS and T/SID Color Imaging Conference, 364-369.More infoAbstract: Color is of interest to those working in computer vision largely because it is assumed to be helpful for recognition. This assumption has driven much work in color based image indexing, and computational color constancy. However, in many ways, indexing is a poor model for recognition. In this paper we use a recently developed statistical model of recognition which learns to link image region features with words, based on a large unstructured data set. The system is general in that it learns what is recognizable given the data. It also supports a principled testing paradigm which we exploit here to evaluate the use of color. In particular, we look at color space choice, degradation due to illumination change, and dealing with this degradation. We evaluate two general approaches to dealing with this color constancy problem. Specifically we address whether it is better to build color variation due to illumination into a recognition system, or, instead, apply color constancy preprocessing to images before they are processed by the recognition system.
- Barnard, K., & Shirahatti, N. V. (2003). A method for comparing content based image retrieval methods. Proceedings of SPIE - The International Society for Optical Engineering, 5018, 1-8.More infoAbstract: We assume that the goal of content based image retrieval is to find images which are both semantically and visually relevant to users based on image descriptors. These descriptors are often provided by an example image - the query by example paradigm. In this work we develop a very simple method for evaluating such systems based on large collections of images with associated text. Examples of such collections include the Corel image collection, annotated museum collections, news photos with captions, and web images with associated text based on heuristic reasoning on the structure of typical web pages (such as used by Google(tm)). The advantage of using such data is that it is plentiful, and the method we propose can be automatically applied to hundreds of thousands of queries. However, it is critical that such a method be verified against human usage, and to do this we evaluate over 6000 query/result pairs. Our results strongly suggest that at least in the case of the Corel image collection, the automated measure is a good proxy for human evaluation. Importantly, our human evaluation data can be reused for the evaluation of any content based image retrieval system and/or the verification of additional proxy measures.
- Barnard, K., Duygulu, P., & Forsyth, D. (2003). Recognition as translating images into text. Proceedings of SPIE - The International Society for Optical Engineering, 5018, 168-178.More infoAbstract: We present an overview of a new paradigm for tackling long standing computer vision problems. Specifically our approach is to build statistical models which translate from a visual representations (images) to semantic ones (associated text). As providing optimal text for training is difficult at best, we propose working with whatever associated text is available in large quantities. Examples include large image collections with keywords, museum image collections with descriptive text, news photos, and images on the web. In this paper we discuss how the translation approach can give a handle on difficult questions such as: What counts as an object? Which objects are easy to recognize and which are hard? Which objects are indistinguishable using our features? How to integrate low level vision processes such as feature based segmentation, with high level processes such as grouping. We also summarize some of the models proposed for translating from visual information to text, and some of the methods used to evaluate their performance.
- Barnard, K., Duygulu, P., Forsyth, D., Freitas, N. D., Blei, D. M., & Jordan, M. I. (2003). Matching Words and Pictures. Journal of Machine Learning Research, 3(6), 1107-1135.More infoAbstract: We present a new approach for modeling multi-modal data sets, focusing on the specific case of segmented images with associated text. Learning the joint distribution of image regions and words has many applications. We consider in detail predicting words associated with whole images (auto-annotation) and corresponding to particular image regions (region naming). Auto-annotation might help organize and access large collections of images. Region naming is a model of object recognition as a process of translating image regions to words, much as one might translate from one language to another. Learning the relationships between image regions and semantic correlates (words) is an interesting example of multi-modal data mining, particularly because it is typically hard to apply data mining techniques to collections of images. We develop a number of models for the joint distribution of image regions and words, including several which explicitly learn the correspondence between regions and words. We study multi-modal and correspondence extensions to Hofmann's hierarchical clustering/aspect model, a translation model adapted from statistical machine translation (Brown et al.), and a multi-modal extension to mixture of latent Dirichlet allocation (MoM-LDA). All models are assessed using a large collection of annotated images of real scenes. We study in depth the difficult problem of measuring performance. For the annotation task, we look at prediction performance on held out data. We present three alternative measures, oriented toward different types of task. Measuring the performance of correspondence methods is harder, because one must determine whether a word has been placed on the right region of an image. We can use annotation performance as a proxy measure, but accurate measurement requires hand labeled data, and thus must occur on a smaller scale. We show results using both an annotation proxy, and manually labeled data.
- Barnard, K., Duygulu, P., Guru, R., Gabbur, P., & Forsyth, D. (2003). The effects of segmentation and feature choice in a translation model of object recognition. Proceedings of the IEEE Computer Society Conference on Computer Vision and Pattern Recognition, 2, II/675-II/682.More infoAbstract: We work with a model of object recognition where words must be placed on image regions. This approach means that large scale experiments are relatively easy, so we can evaluate the effects of various early and mid-level vision algorithms on recognition performance. We evaluate various image segmentation algorithms by determining word prediction accuracy for images segmented in various ways and represented by various features. We take the view that good segmentations respect object boundaries, and so word prediction should be better for a better segmentation. However, it is usually very difficult in practice to obtain segmentations that do not break up objects, so most practitioners attempt to merge segments to get better putative object representations. We demonstrate that our paradigm of word prediction easily allows us to predict potentially useful segment merges, even for segments that do not look similar (for example, merging the black and white halves of a penguin is not possible with feature-based segmentation; the main cue must be "familiar configuration"). These studies focus on unsupervised learning of recognition. However, we show that word prediction can be markedly improved by providing supervised information for a relatively small number of regions together with large quantities of unsupervised information. This supervisory information allows a better and more discriminative choice of features and breaks possible symmetries.
- Barnard, K., & Funt, B. (2002). Camera characterization for color research. Color Research and Application, 27(3), 152-163.More infoAbstract: In this article we introduce a new method for estimating camera sensitivity functions from spectral power input and camera response data. We also show how the procedure can be extended to deal with camera nonlinearities. Linearization is an important part of camera characterization, and we argue that it is best to jointly fit the linearization and the sensor response functions. We compare our method with a number of others, both on synthetic data and for the characterization of a real camera. All data used in this study is available online at www.cs.sfu.ca/~colour/data. © 2002 Wiley Periodicals, Inc. Col. Res. Appl.
- Barnard, K., Cardei, V., & Funt, B. (2002). A comparison of computational color constancy algorithms - Part I: Methodology and experiments with synthesized data. IEEE Transactions on Image Processing, 11(9), 972-984.More infoPMID: 18249720;Abstract: We introduce a context for testing computational color constancy, specify our approach to the implementation of a number of the leading algorithms, and report the results of three experiments using synthesized data. Experiments using synthesized data are important because the ground truth is known, possible confounds due to camera characterization and pre-processing are absent, and various factors affecting color constancy can be efficiently investigated because they can be manipulated individual and precisely. The algorithms chosen for close study include two gray world methods, a limiting case of a version of the Retinex method, a number of variants of Forsyth's gamut-mapping method, Cardei et al.'s neural net method, and Finlayson et al.'s Color by Correlation method. We investigate the ability of these algorithms to make estimates of three different color constancy quantities: the chromaticity of the scene illuminant, the overall magnitude of that illuminant, and a corrected, illumination invariant, image. We consider algorithm performance as a function of the number of surfaces in scenes generated from reflectance spectra, the relative effect on the algorithms of added specularities, and the effect of subsequent clipping of the data. All data is available on-line at http://www.cs.sfu.ca/∼color/data, and implementations for most of the algorithms are also available (http://www.cs.sfu.ca/∼color/code).
- Barnard, K., Duygulu, P., & Forsyth, D. (2002). Modeling the statistics of image features and associated text. Proceedings of SPIE - The International Society for Optical Engineering, 4670, 1-11.More infoAbstract: We present a methodology for modeling the statistics of image features and associated text in large datasets. The models used also serve to cluster the images, as images are modeled as being produced by sampling from a limited number of combinations of mixing components. Furthermore, because our approach models the joint occurrence image features and associated text, it can be used to predict the occurrence of either, based on observations or queries. This supports an attractive approach to image search as well as novel applications such a suggesting illustrations for blocks of text (auto-illustrate) and generating words for images outside the training set (auto-annotate). In this paper we illustrate the approach on 10,000 images of work from the Fine Arts Museum of San Francisco. The images include line drawings, paintings, and pictures of sculpture and ceramics. Many of the images have associated free text whose nature varies greatly, from physical description to interpretation and mood. We incorporate statistical natural language processing in order to deal with free text. We use WordNet to provide semantic grouping information and to help disambiguate word senses, as well as emphasize the hierarchical nature of semantic relationships.
- Barnard, K., Martin, L., Coath, A., & Funt, B. (2002). A comparison of computational color constancy algorithms - Part II: Experiments with image data. IEEE Transactions on Image Processing, 11(9), 985-996.More infoPMID: 18249721;Abstract: We test a number of the leading computational color constancy algorithms using a comprehensive set of images. These were of 33 different scenes under 11 different sources representative of common illumination conditions. The algorithms studied include two gray world methods, a version of the Retinex method, several variants of Forsyth's gamut-mapping method, Cardei et al,'s neural net method, and Finlayson et al.'s Color by Correlation method. We discuss a number of issues in applying color constancy ideas to image data, and study in depth the effect of different preprocessing strategies. We compare the performance of the algorithms on image data with their performance on synthesized data. All data used for this study is available online at http://www.cs.sfu.ca/~color/data, and implementations for most of the algorithms are also available (http://www.cs.sfu.ca/∼color/code). Experiments with synthesized data (part one of this paper) suggested that the methods which emphasize the use of the input data statistics, specifically Color by Correlation and the neural net algorithm, are potentially the most effective at estimating the chromaticity of the scene illuminant. Unfortunately, we were unable to realize comparable performance on real images. Here exploiting pixel intensity proved to be more beneficial than exploiting the details of image chromaticity statistics, and the three-dimensional (3-D) gamut-mapping algorithms gave the best performance.
- Barnard, K., Martin, L., Funt, B., & Coath, A. (2002). A data set for color research. Color Research and Application, 27(3), 147-151.More infoAbstract: We present an extensive data set for color research that has been made available online (www.cs.sfu.ca/̃colour/data). The data are especially germane to research into computational color constancy, but we have also aimed to make the data as general as possible, and we anticipate a wide range of benefits to research into computational color science and computer vision. Because data are useful only in context, we provide the details of the collection process, including the camera characterization, and the data used to determine that characterization. The most significant part of the data is 743 images of scenes taken under a carefully chosen set of 11 illuminants. The data set also has several standardized sets of spectra for synthetic data experiments, including some data for fluorescent surfaces. © 2002 Wiley Periodicals, Inc. Col. Res. Appl.
- Cardei, V. C., Funt, B., & Barnard, K. (2002). Estimating the scene illumination chromaticity by using a neural network. Journal of the Optical Society of America A: Optics and Image Science, and Vision, 19(12), 2374-2386.More infoPMID: 12469731;Abstract: A neural network can learn color constancy, defined here as the ability to estimate the chromaticity of a scene's overall illumination. We describe a multilayer neural network that is able to recover the illumination chromaticity given only an image of the scene. The network is previously trained by being presented with a set of images of scenes and the chromaticities of the corresponding scene illuminants. Experiments with real images show that the network performs better than previous color constancy methods. In particular, the performance is better for images with a relatively small number of distinct colors. The method has application to machine vision problems such as object recognition, where illumination-independent color descriptors are required, and in digital photography, where uncontrolled scene illumination can create an unwanted color cast in a photograph. © 2002 Optical Society of America.
- Barnard, K., & Forsyth, D. (2001). Exploiting image semantics for picture libraries. Proceedings of First ACM/IEEE-CS Joint Conference on Digital Libraries, 469-.More infoAbstract: A system for learning the semantics of collections of images from features and associated text is discussed. The idea of the application of this system to the digital image libraries is explored. The nature of search and browsing is considered and it is argued that for many applications these should be used together.
- Barnard, K., & Forsyth, D. (2001). Learning the semantics of words and pictures. Proceedings of the IEEE International Conference on Computer Vision, 2, 408-415.More infoAbstract: We present a statistical model for organizing image collections which integrates semantic information provided by associated text and visual information provided by image features. The model is very promising for information retrieval tasks such as database browsing and searching for images based on text and/or image features. Furthermore, since the model learns relationships between text and image features, it can be used for novel applications such as associating words with pictures and unsupervised learning for object recognition.
- Barnard, K., Ciurea, F., & Funt, B. (2001). Sensor sharpening for computational color constancy. Journal of the Optical Society of America A: Optics and Image Science, and Vision, 18(11), 2728-2743.More infoPMID: 11688863;Abstract: Sensor sharpening [J. Opt. Soc. Am. A 11, 1553 (1994)] has been proposed as a method for improving computational color constancy, but it has not been thoroughly tested in practice with existing color constancy algorithms. In this paper we study sensor sharpening in the context of viable color constancy processing, both theoretically and empirically, and on four different cameras. Our experimental findings lead us to propose a new sharpening method that optimizes an objective function that includes terms that minimize negative sensor responses as well as the sharpening error for multiple illuminants instead of a single illuminant. Further experiments suggest that this method is more effective for use with several known color constancy algorithms. © 2001 Optical Society of America.
- Barnard, K., Duygulu, P., & Forsyth, D. (2001). Clustering art. Proceedings of the IEEE Computer Society Conference on Computer Vision and Pattern Recognition, 2, II434-II441.More infoAbstract: We extend a recently developed method for learning the semantics of image databases using text and pictures. We incorporate statistical natural language processing in order to deal with free text. We demonstrate the current system on a difficult dataset, namely 10,000 images of work from the Fine Arts Museum of San Francisco. The images include line drawings, paintings, and pictures of sculpture and ceramics. Many of the images have associated free text whose varies greatly, from physical description to interpretation and mood. We use WordNet to provide semantic grouping information and to help disambiguate word senses, as well as emphasize the hierarchical nature of semantic relationships. This allows us to impose a natural structure on the image collection, that reflects semantics to a considerable degree. Our method produces a joint probability distribution for words and picture elements. We demonstrate that this distribution can be used (a) to provide illustrations for given captions and (b) to generate words for images outside the training set. Results from this annotation process yield a quantitative study of our method. Finally, our annotation process can be seen as a form of object recognizer that has been learned through a partially supervised process.
- Barnard, K., & Finlayson, G. (2000). Shadow identification using colour ratios. Final Program and Proceedings - IS and T/SID Color Imaging Conference, 97-101.More infoAbstract: In this paper we present a comprehensive method for identifying probable shadow regions in an image. Doing so is relevant to computer vision, colour constancy, and image reproduction, specifically dynamic range compression. Our method begins with a segmentation of the image into regions of the same colour. Then the edges between the regions are analyzed with respect to the possibility that each is due to an illumination change as opposed to a material boundary. We then integrate the edge information to produce an estimate of the illumination field.
- Barnard, K. (1999). Color constancy with fluorescent surfaces. Final Program and Proceedings - IS and T/SID Color Imaging Conference, 257-261.More infoAbstract: Fluorescent surfaces are common in the modern world, but they present problems for machine color constancy because fluorescent reflection typically violates the assumptions needed by most algorithms. The complexity of fluorescent reflection is likely one of the reasons why fluorescent surfaces have escaped the attention of computational color constancy researchers. In this paper we take some initial steps to rectify this omission. We begin by introducing a simple method for characterizing fluorescent surfaces. It is based on direct measurements, and thus has low error and avoids the need to develop a comprehensive and accurate physical model. We then modify and extend several modern color constancy algorithms to address fluorescence. The algorithms considered are CRULE and derivatives, Color by Correlation, and neural net methods. Adding fluorescence to Color by Correlation and neural net methods is relatively straight forward, but CRULE requires modification so that its complete reliance on diagonal models can be relaxed. We present results for both synthetic and real image data for fluorescent capable versions of CRULE and Color by Correlation, and we compare the results with the standard versions of these and other algorithms.
- Barnard, K., & Funt, B. (1999). Camera calibration for color research. Proceedings of SPIE - The International Society for Optical Engineering, 3644, 576-585.More infoAbstract: In this paper we introduce a new method for determining the relationship between signal spectra and camera RGB which is required for many applications in color. We work with the standard camera model, which assumes that the response is linear. We also provide an example of how the fitting procedure can be augmented to include fitting for a previously estimated non-linearity. The basic idea of our method is to minimize squared error subject to linear constraints, which enforce positivity and range of the result. It is also possible to constrain the smoothness, but we have found that it is better to add a regularization expression to the objective function to promote smoothness. With this method, smoothness and error can be traded against each other without being restricted by arbitrary bounds. The method is easily implemented as it is an example of a quadratic programming problem, for which there are many software solutions available. In this paper we provide the results using this method and others to calibrate a Sony DXC-930 CCD color video camera. We find that the method gives low error, while delivering sensors which are smooth and physically realizable. Thus we find the method superior to methods which ignore any of these considerations.
- Barnard, K., & Funt, B. (1999). Color constancy with specular and non-specular surfaces. Final Program and Proceedings - IS and T/SID Color Imaging Conference, 114-119.More infoAbstract: There is a growing trend in machine color constancy research to use only image chromaticity information, ignoring the magnitude of the image pixels. This is natural because the main purpose is often to estimate only the chromaticity of the illuminant. However, the magnitudes of the image pixels also carry information about the chromaticity of the illuminant. One such source of information is through image specularities. As is well known in the computational color constancy field, specularities from inhomogeneous materials (such as plastics and painted surfaces) can be used for color constancy. This assumes that the image contains specularities, that they can be identified, and that they do not saturate the camera sensors. These provisos make it important that color constancy algorithms which make use of specularities also perform well when the they are absent. A further problem with using specularities is that the key assumption, namely that the specular component is the color of the illuminant, does not hold in the case of colored metals. In this paper we investigate a number of color constancy algorithms in the context of specular and non-specular reflection. We then propose extensions to several variants of Forsyth's CRULE algorithm1-4 which make use of specularities if they exist, but do not rely on their presence. In addition, our approach is easily extended to include colored metals, and is the first color constancy algorithm to deal with such surfaces. Finally, our method provides an estimate of the overall brightness, which chromaticity-based methods cannot do, and other RGB based algorithms do poorly when specularities are present.
- Cardei, V. C., Funt, B., & Barnard, K. (1999). White point estimation for uncalibrated images. Final Program and Proceedings - IS and T/SID Color Imaging Conference, 97-100.More infoAbstract: Color images often must be color balanced to remove unwanted color casts. We extend previous work on using a neural network for illumination, or white-point, estimation from the case of calibrated images to that of uncalibrated images of unknown origin. The results show that the chromaticity of the ambient illumination can be estimated with an average CIE Lab error of 5ΔE. Comparisons are made to the grayworld and white patch methods.
- Barnard, K., & Funt, B. (1998). Experiments in sensor sharpening for color constancy. Final Program and Proceedings - IS and T/SID Color Imaging Conference, 43-46.More infoAbstract: Sensor sharpening has been proposed as a method for improving color constancy algorithms but it has not been tested in the context of real color constancy algorithms. In this paper we test sensor sharpening as a method for improving color constancy algorithms in the case of three different cameras, the human cone sensitivity estimates, and the XYZ response curves. We find that when the sensors are already relatively sharp, sensor sharpening does not offer much improvement and can have a detrimental effect However, when the sensors are less sharp, sharpening can have a substantive positive effect. The degree of improvement is heavily dependent on the particular color constancy algorithm. Thus we conclude that using sensor sharpening for improving color constancy can offer a significant benefit, but its use needs to be evaluated with respect to both the sensors and the algorithm.
- Barnard, K., & Funt, B. (1997). Analysis and improvement of multi-scale retinex. Final Program and Proceedings - IS and T/SID Color Imaging Conference, 221-226.More infoAbstract: The main thrust of this paper is to modify the multi-scale retinex (MSR) approach to image enhancement so that the processing is more justified from a theoretical standpoint. This leads to a new algorithm with fewer arbitrary parameters that is more flexible, maintains color fidelity, and still preserves the contrast-enhancement benefits of the original MSR method. To accomplish this we identify the explicit and implicit processing goals of MSR. By decoupling the MSR operations from one another, we build an algorithm composed of independent steps that separates out the issues of gamma adjustment, color balance, dynamic range compression, and color enhancement, which are all jumbled together in the original MSR method. We then extend MSR with color constancy and chromaticity-preserving contrast enhancement.
- Barnard, K., & Funt, B. (1997). Analysis and improvement of multi-scale retinex. Proceedings of the Color Imaging Conference: Color Science, Systems, and Applications, 221-225.More infoAbstract: The main thrust of this paper is to modify the multi-scale retinex (MSR) approach to image enhancement so that the processing is more justified from a theoretical standpoint. This leads to a new algorithm with fewer arbitrary parameters that is more flexible, maintains color fidelity, and still preserves the contrast-enhancement benefits of the original MSR method. To accomplish this we identify the explicit and implicit processing goals of MSR. By decoupling the MSR operations from one another, we build an algorithm composed of independent steps that separates out the issues of gamma adjustment, color balance, dynamic range compression, and color enhancement, which are all jumbled together in the original MSR method. We then extend MSR with color constancy and chromaticity-preserving contrast enhancement.
- Barnard, K., Finlayson, G., & Funt, B. (1997). Color Constancy for Scenes with Varying Illumination. Computer Vision and Image Understanding, 65(2), 311-321.More infoAbstract: We present an algorithm which uses information from both surface reflectance and illumination variation to solve for color constancy. Most color constancy algorithms assume that the illumination across a scene is constant, but this is very often not valid for real images. The method presented in this work identifies and removes the illumination variation, and in addition uses the variation to constrain the solution. The constraint is applied conjunctively to constraints found from surface reflectances. Thus the algorithm can provide good color constancy when there is sufficient variation in surface reflectances, or sufficient illumination variation, or a combination of both. We present the results of running the algorithm on several real scenes, and the results are very encouraging. © 1997 Academic Press.
- Funt, B., Cardei, V., & Barnard, K. (1997). Learning color constancy. Proceedings of the Color Imaging Conference: Color Science, Systems, and Applications, 58-60.More infoAbstract: We decided to test a surprisingly simple hypothesis; namely, that the relationship between an image of a scene and the chromaticity of scene illumination could be learned by a neural network. The thought was that if this relationship could be extracted by a neural network, then the trained network would be able to determine a scene's Illuminant from its image, which would then allow correction of the image colors to those relative to a standard illuminance, thereby providing color constancy. Using a database of surface reflectances and illuminants, along with the spectral sensitivity functions of our camera, we generated thousands of images of randomly selected illuminants lighting `scenes' of 1 to 60 randomly selected reflectances. During the learning phase the network is provided the image data along with the chromaticity of its illuminant. After training, the network outputs (very quickly) the chromaticity of the illumination given only the image data. We obtained surprisingly good estimates of the ambient illumination lighting from the network even when applied to scenes in our lab that were completely unrelated to the training data.
- Funt, B., Cardei, V., & Barnard, K. (1996). Learning color constancy. Final Program and Proceedings - IS and T/SID Color Imaging Conference, 58-60.More infoAbstract: We decided to test a surprisingly simple hypothesis; namely, that the relationship between an image of a scene and the chromaticity of scene illumination could be learned by a neural network. The thought was that if this relationship could be extracted by a neural network, then the trained network would be able to determine a scene's Illuminant from its image, which would then allow correction of the image colors to those relative to a standard illuminance, thereby providing color constancy. Using a database of surface reflectances and illuminants, along with the spectral sensitivity functions of our camera, we generated thousands of images of randomly selected illuminants lighting 'scenes' of 1 to 60 randomly selected reflectances. During the learning phase the network is provided the image data along with the chromaticity of its illuminant. After training, the network outputs (very quickly) the chro-maticity of the illumination given only the image data. We obtained surprisingly good estimates of the ambient illumination lighting from the network even when applied to scenes in our lab that were completely unrelated to the training data.
- Finlayson, G. D., Funt, B. V., & Barnard, K. (1995). Color constancy under varying illumination. IEEE International Conference on Computer Vision, 720-725.More infoAbstract: Illumination is rarely constant in intensity or color throughout a scene. Multiple light sources with different spectra - sun and sky, direct and interreflected light - are the norm. Nonetheless, almost all color constancy algorithms assume that the spectrum of the incident illumination remains constant across the scene. We assume the converse, that illumination does vary, in developing a new algorithm for color constancy. Rather than creating difficulties, varying illumination is in fact a very powerful constraint. Indeed tests of our algorithm using real images of an office scene show excellent results.
Proceedings Publications
- Dai, Y., Oehmcke, S., Gieseke, F., Wu, Y., & Barnard, J. J. (2021, Fall). Attentional Feature Fusion. In Winter Conference on Applications of Computer Vision (WACV).More infoVery solid second tier venue
- Dai, Y., Oehmcke, S., Wu, Y., & Barnard, J. J. (2021, Fall). Asymmetric Contextual Modulation for Infrared Small Target Detection. In Winter Conference on Applications of Computer Vision (WACV).More infoVery solid second tier venue
- Pyarelal, A., Banerjee, A., & Barnard, J. J. (2021). Modular Procedural Generation for Voxel Maps. In AAAI 2021 Fall Symposium on Computational Theory of Mind for Human-Machine Teams.
- Soares, P., Pyarelal, A., & Barnard, J. J. (2021). Probabilistic Modeling of Human Intents and Beliefs to Predict Actions Under False Belief,. In AAAI 2021 Fall Symposium on Computational Theory of Mind for Human-Machine Teams..
- Dai, Y., Oehmcke, S., Gieseke, F., Wu, Y., & Barnard, J. J. (2020, Fall). Attention As Activation. In International Conference on Pattern Recognition (ICPR).
- Morad, S., Nash, J., Smith, R. G., Parness, A., & Barnard, J. J. (2020, June). Improving Visual Feature Extraction in Glacial Environments. In IEEE Conference on Robotics and Automation (ICRA).More infoUsed in CS rankings
- Barnard, J. J., Morrison, C. T., Sharp, R., & Pyarelal, A. (2019, May). Interpreting Causal Expressions with Gradable Adjectives to Assembly Dynamics Models. In Modeling the World's Systems.
- Basavaraj, C., Reimann, M., Barnard, K., & Norton, M. I. (2019). Predicting experiential (vs. monetary) risk preferences from consumersâ memory: A behavioral and neuroimaging experiment. In Association for Consumer Research Annual Conference.
- Surdeanu, M., Morrison, C. T., Barnard, J. J., Bethard, S. J., Paul, M., Luo, F., Lent, H., Tang, Z., Bachman, J. A., Yadav, V., Nagesh, A., Valenzuela-Escárcega, M. A., Laparra, E., Alcock, K., Gyori, B. M., Pyarelal, A., & Sharp, R. (2019, Summer). Eidos, INDRA & Delphi: From Free Text to Executable Causal Models. In Annual Conference of the North American Chapter of the Association for Computational Linguistics (NAACL).
- Zachariah, A., Senapati, M., Katib, A., Rao, P., Barnard, K., & Kamhoua, C. (2019). A Gossip-Based System for Fast Approximate Score Computation in Multinomial Bayesian Networks. In ICDE Demo.
- Brau, E., Gaun, J., Jeffries, T., & Barnard, J. J. (2018, October). Multiple-gaze geometry: Inferring novel 3D locations from gazes observed in monocular video. In European Conference on Computer Vision (ECCV).More infoThis is CS ranking approved and "A" (I disagree with "A"; this venue is an equivalence class with two others which have A*).
- Rao, P., Katib, A., Barnard, J. J., Kamhoua, C., Kwiat, K., & Njilla, L. (2017, January). Scalable Score Computation for Learning Multinomial Bayesian Networks over Distributed Data. In AAAI 2017 Workshop on Distributed Machine Learning.
- Simek, K., Palanivelu, R., & Barnard, J. J. (2016, October). Branching Gaussian Processes with Applications to Spatiotemporal Reconstruction of 3D Trees. In Computer Vision – ECCV 2016, 177-193.More infoIn computer sciences, submission to conference proceedings are only published after a peer-review. This is a primary research article that was published in the conference proceedings after a peer-review.[ CSRankings endorsed, A ](I contest the A as being US centric. ECCV is in an equivalence class with two A* venues).
- Barnard, J. J., & Simek, K. (2015, September). Gaussian Process Shape Models for Bayesian Segmentation of Plant Leaves. In Computer Vision Problems in Plant Phenotyping (CVPPP).
- Guan, J., Barnard, K. J., Brau, E., Butler, E. A., Simek, K., Morrison, C. T., Simek, K., Morrison, C. T., Brau, E., Butler, E. A., Guan, J., & Barnard, K. J. (2015, July). Moderated and Drifting Linear Dynamical Systems. In International Conference on Machine Learning.
- Guan, J., Brau, E., Simek, K., Morrison, C. T., Butler, E. A., & Barnard, K. J. (2015, July). Moderated and Drifting Linear Dynamical Systems. In International Conference on Machine Learning (ICML 2015).More infoThis venue is a peer reviewed, competitive conference (acceptance rate: 26%) and the full paper is published as part of the conference proceedings
Presentations
- Lall, U., Barnard, J. J., Melancon, A., Gurung, I., Molthan, A., Muhkerjee, R., & Tellman Sullivan, E. M. (2021). High resolution imagery to train and validate deep learning models of inundation extent for multiple satellite sensors. American Geophysical Union.
- Barnard, J. J., Butler, E. A., & Guan, J. (2016, August). Dynamic system modeling infrastructure (DSMI): Past, present and future. Dynamic Systems Modeling Expert Meeting. Aberdeen, U.K.